AWS re:Invent 2023 - Transforming the consumer packaged goods industry with generative AI (CPG203)
Summary
TLDRMichael Connor, leading the generative AI practice for a global CPG retail team, shares insights from engaging with 1,000 C-suite and board members on the transformative impact of generative AI on business. He discusses the technology's potential $7 trillion GDP impact, use cases in marketing, sales, and product R&D, and the importance of a robust data strategy. Connor also highlights innovative applications like advertising personalization and product concept generation, emphasizing the need for change management and responsible use frameworks to leverage generative AI effectively.
Takeaways
- 📈 Michael Connor leads the generative AI practice for the global CPG retail team and has observed significant interest and adoption of generative AI in the last six months.
- 💡 The technology is predicted to have a $7 trillion impact on GDP, indicating a massive potential for business transformation.
- 🔍 Connor has engaged with over 1,000 C-suite and board members, noting a significant shift in business strategies to incorporate generative AI.
- 🌐 Investments in generative AI are prominent, with examples such as the $4 billion investment in Anthropic, highlighting the technology's potential.
- 📢 Advertising is being revolutionized by generative AI, with the ability to create personalized content and engage consumers in new ways.
- 🛠️ Use cases for generative AI span across various departments including marketing, sales, customer operations, product R&D, and software engineering.
- 📈 There's a clear progression in customer engagement with generative AI, moving from understanding the technology to executing and integrating it into business operations.
- 🧑💻 Generative AI differs from traditional machine learning by utilizing a vast array of internet data to create models capable of multiple tasks, such as code generation and image creation.
- 💼 A robust data strategy is essential for leveraging generative AI, emphasizing the need for data hygiene, governance, and a chief data officer role.
- 🔧 Tools like ComfyUI and LangChain enable the creation of AI-driven solutions without coding, facilitating rapid development and deployment of generative AI applications.
- 📱 The future of retail may involve conversational interfaces with product catalogs, allowing for more personalized and dynamic shopping experiences.
Q & A
What is Michael Connor's role at the company?
-Michael Connor leads the generative AI practice for the CPG retail team globally.
What is the estimated impact of generative AI on GDP according to the script?
-The estimated impact of generative AI on GDP is $7 trillion.
What is the significance of the investment in Anthropic mentioned in the script?
-The company invested $4 billion in Anthropic, which makes Claude, Michael Connor's favorite model.
How is generative AI changing advertising according to Michael Connor?
-Generative AI is changing advertising by allowing for the creation of personalized ads and product concepts, potentially leading to a permanent shift in the industry.
What is the role of generative AI in product development according to the transcript?
-Generative AI is used in product development for creating product concepts, generating 2D images, 3D CAD models, and even drafting press releases and product descriptions.
What are the three phases customers go through when engaging with generative AI as described in the script?
-The three phases are: understanding what the technology is, discovering use cases, and executing on the technology by organizing as a business and considering ethics and bias.
What is the 'zero-ETL era' mentioned by Michael Connor?
-The 'zero-ETL era' refers to a future state where the need for ETL jobs, which involve transferring data from one system to another, will be eliminated due to advancements in technology.
How does Michael Connor describe the difference between machine learning and generative AI?
-Machine learning involves training a model on one task with a dataset, whereas generative AI uses data from the internet to train a model capable of doing many different tasks.
What is the importance of a good data strategy for leveraging generative AI, as emphasized in the script?
-A good data strategy is crucial for taking advantage of generative AI because it ensures that the data is well-organized, clean, and accessible for the AI to generate accurate and relevant outputs.
What is LangChain and how does it relate to generative AI?
-LangChain is a framework that helps glue various systems together, allowing generative AI models to interact with databases, knowledge bases, product catalogs, and other resources to provide more informed and context-aware responses.
What is the recommended approach for companies to start utilizing generative AI according to the script?
-The recommended approach is to start with high-impact, low-effort use cases, such as call center applications with a human-in-the-loop, and to develop a responsible use framework that considers ethics, bias, and legal implications.
Outlines
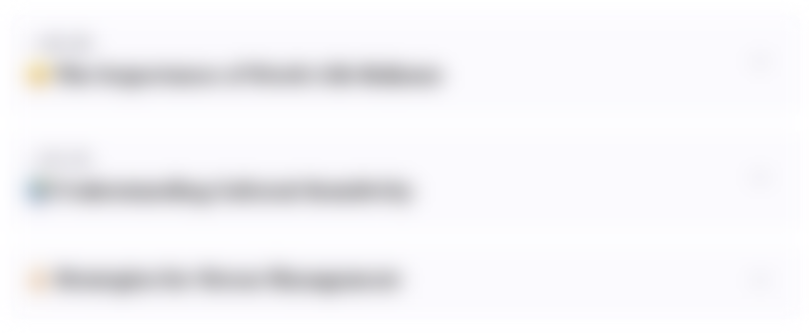
Cette section est réservée aux utilisateurs payants. Améliorez votre compte pour accéder à cette section.
Améliorer maintenantMindmap
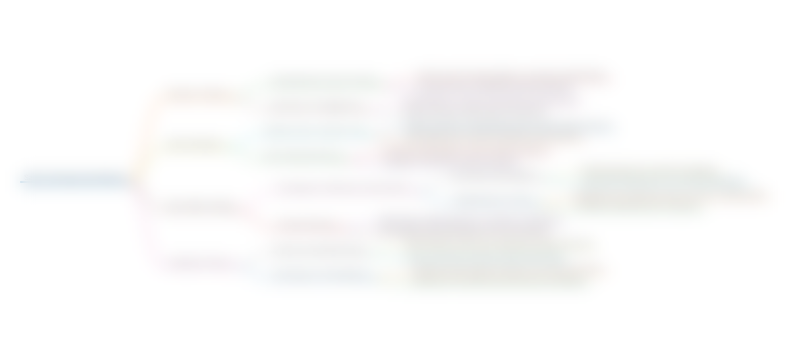
Cette section est réservée aux utilisateurs payants. Améliorez votre compte pour accéder à cette section.
Améliorer maintenantKeywords
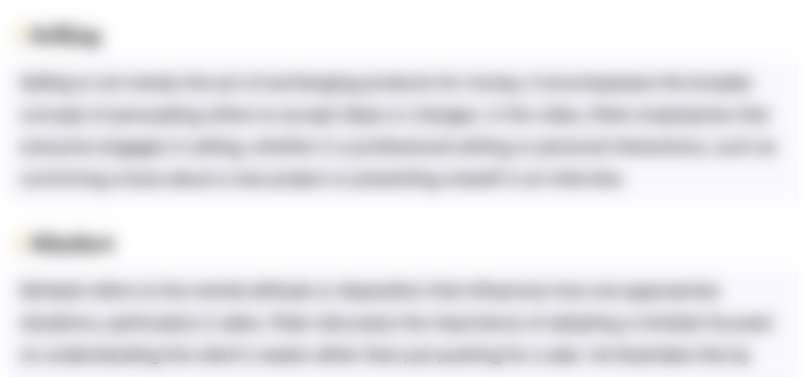
Cette section est réservée aux utilisateurs payants. Améliorez votre compte pour accéder à cette section.
Améliorer maintenantHighlights
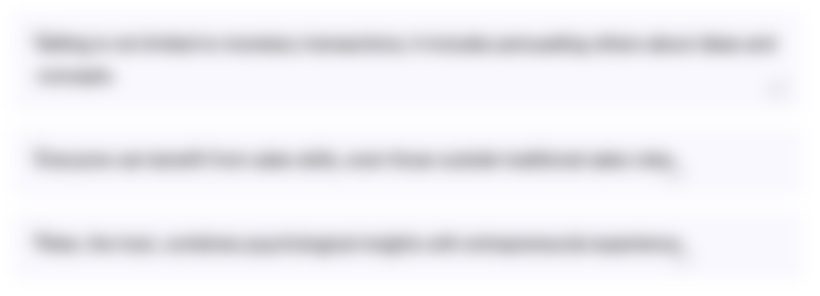
Cette section est réservée aux utilisateurs payants. Améliorez votre compte pour accéder à cette section.
Améliorer maintenantTranscripts
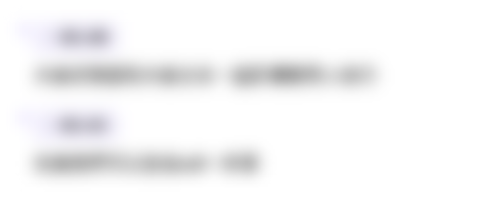
Cette section est réservée aux utilisateurs payants. Améliorez votre compte pour accéder à cette section.
Améliorer maintenant5.0 / 5 (0 votes)