CARTA: Computational Neuroscience and Anthropogeny with Terry Sejnowski
Summary
TLDRThe talk explores the intersection of computational neuroscience and anthropology, delving into how the brain functions computationally. It highlights the advancements in neuroscience due to the Brain Initiative, allowing for the recording of thousands of neurons. The speaker contrasts early neural networks from the 1980s with modern deep learning models, emphasizing the evolution to complex architectures capable of tasks like language translation. The discussion also touches on the controversy surrounding the consciousness and understanding of AI, proposing the 'mirror hypothesis' suggesting AI reflects the intelligence of its interrogator.
Takeaways
- 🧠 The human brain, with its 100 billion neurons, is a complex organ that continues to generate activity even in the absence of external stimuli.
- 🔬 The Brain Initiative launched by President Obama in 2013 has significantly advanced systems neuroscience, enabling the recording of hundreds of thousands of neurons simultaneously.
- 🐟 Research on model organisms like zebra fish larvae has provided insights into brain activity, showing that brains are active even when the organism is immobilized and in darkness.
- 📈 The computational power required for training neural networks has increased exponentially over time, with modern networks like GPT-3 requiring a million million times more computation than earlier models.
- 🌐 The advancements in deep learning have led to the development of sophisticated language models capable of understanding and generating human-like text.
- 🗣️ Early neural networks, like the one used in the 1980s text-to-speech project, were primitive compared to today's models but still demonstrated the potential for machine learning in language processing.
- 🤖 The architecture of modern neural networks, including recurrent and transformer models, allows for the handling of complex tasks such as language translation and understanding social interactions.
- 🤝 The concept of 'attention' in transformer models is crucial for understanding and generating contextually relevant responses, mirroring the way humans process language.
- 💬 Large language models like LaMDA can generate responses that appear to show understanding and even 'sentience', but their capabilities are heavily dependent on the quality of the prompts they receive.
- 🔮 The debate over whether AI models are truly conscious or just mimicking human-like responses is ongoing, with some experts arguing for a 'mirror hypothesis' suggesting that AI reflects the intelligence of the interviewer.
Q & A
What does the speaker describe as paradoxical about humans?
-Humans are paradoxical because they are bipedal, naked, have large brains, and are the master of fire, tools, and language, yet they are still trying to understand themselves and are aware of their inevitable death.
What was the Brain Initiative announced by Barack Obama in 2013?
-The Brain Initiative aimed to develop innovative new technologies that could revolutionize systems neuroscience by enabling the recording of hundreds of thousands of neurons at a time.
How has the ability to record from a large number of neurons at once impacted neuroscience?
-The ability to record from a large number of neurons at once has dramatically increased the understanding of brain activity patterns, showing that the brain is constantly generating activity even in the absence of external stimuli.
What was the significance of the text-to-speech project in the 1980s mentioned in the script?
-The text-to-speech project in the 1980s was significant because it demonstrated that a simple neural network could master complex language tasks like text-to-speech conversion, challenging traditional linguistic views that relied on rules.
How does the Back-Propagation Learning Algorithm mentioned in the script work?
-The Back-Propagation Learning Algorithm works by repeatedly going through the text until the network learns to pronounce new words accurately, adjusting the weights of the connections between neurons (units) to minimize errors.
What advancements in network architectures have been highlighted in the transition from the 20th to the 21st century?
-The advancements include the move from simple neural networks to deep learning with multiple layers of hidden units, the introduction of recurrent architectures for learning temporal sequences, and the development of transformers with attention mechanisms.
Why are recurrent networks important for language processing?
-Recurrent networks are important for language processing because they can handle temporal sequences, allowing the network to understand the context and order of words, which is crucial for tasks like language translation.
How does the transformer network architecture differ from earlier feed-forward networks?
-Transformer networks differ from earlier feed-forward networks by using an encoder-decoder structure with attention mechanisms that allow the model to process entire sentences or paragraphs at once and produce outputs word by word, enhancing the model's ability to understand context.
What is the 'mirror hypothesis' proposed by the speaker regarding large language models?
-The 'mirror hypothesis' suggests that large language models reflect the intelligence of the interviewer. If prompted with sophisticated questions, they provide sophisticated answers; if prompted with nonsensical questions, they provide nonsensical answers.
What is the significance of the comparison between the brain's functionality and the transformer model's loop?
-The comparison highlights the remarkable similarity between how the human brain processes language and how the transformer model operates, suggesting that the model can replicate some of the brain's language processing functionality.
What does the speaker suggest about the future of understanding large language models?
-The speaker suggests that with mathematical analysis and further study, we will eventually understand the underlying mechanisms that give large language models their abilities, much like how we understand other complex systems.
Outlines
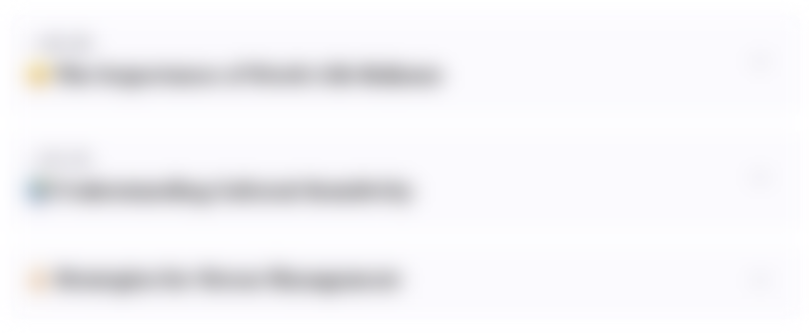
Cette section est réservée aux utilisateurs payants. Améliorez votre compte pour accéder à cette section.
Améliorer maintenantMindmap
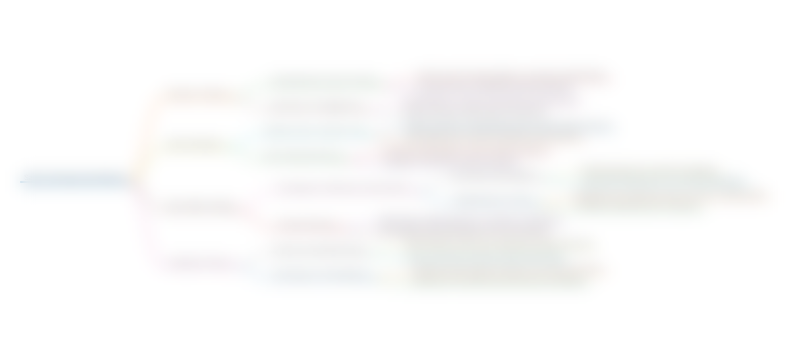
Cette section est réservée aux utilisateurs payants. Améliorez votre compte pour accéder à cette section.
Améliorer maintenantKeywords
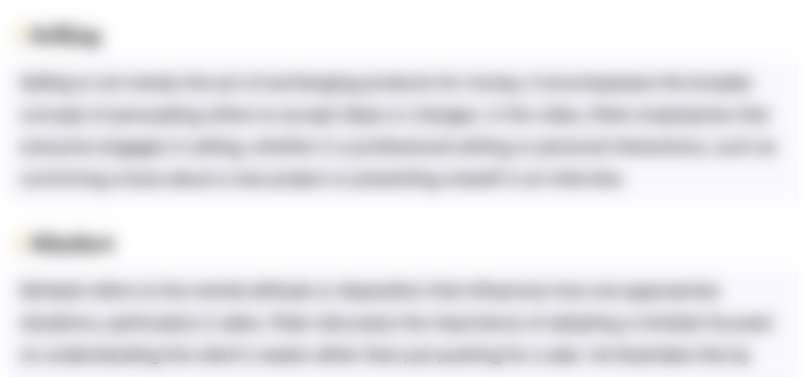
Cette section est réservée aux utilisateurs payants. Améliorez votre compte pour accéder à cette section.
Améliorer maintenantHighlights
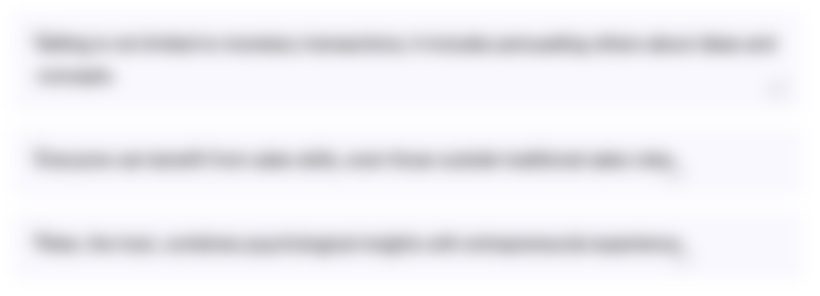
Cette section est réservée aux utilisateurs payants. Améliorez votre compte pour accéder à cette section.
Améliorer maintenantTranscripts
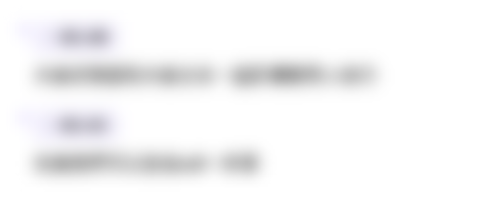
Cette section est réservée aux utilisateurs payants. Améliorez votre compte pour accéder à cette section.
Améliorer maintenantVoir Plus de Vidéos Connexes
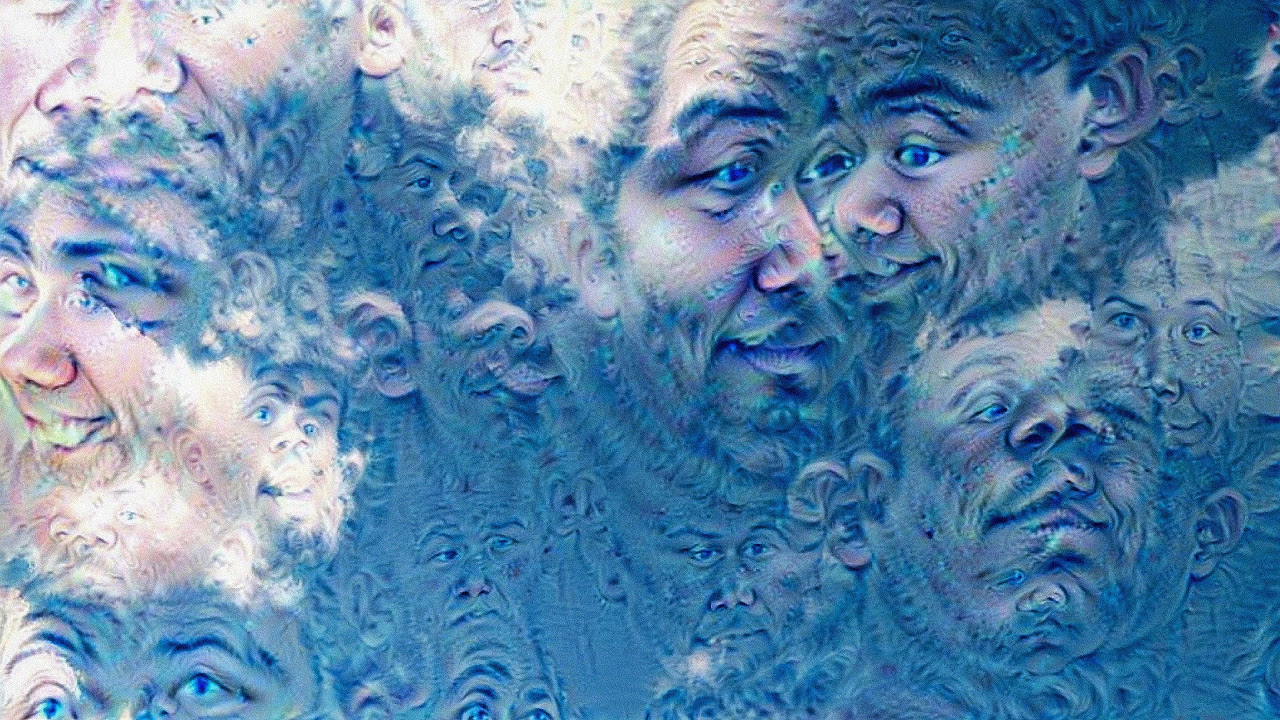
How computers are learning to be creative | Blaise Agüera y Arcas
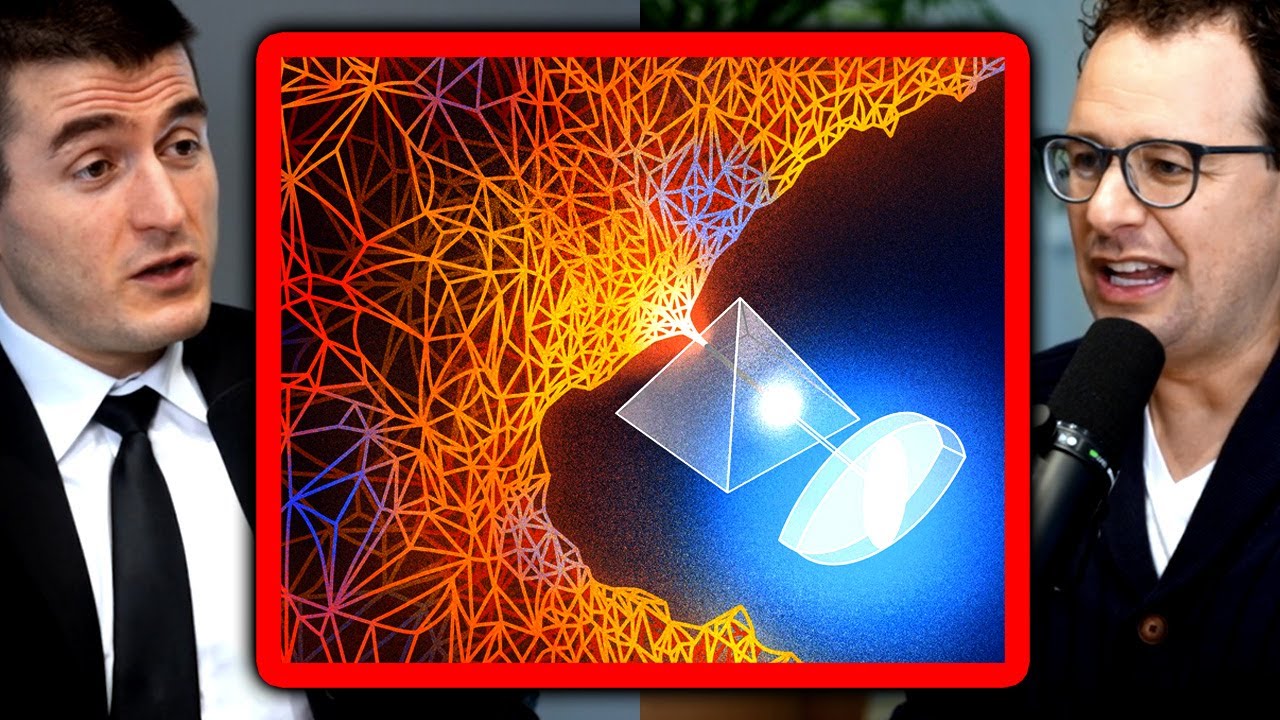
Why bigger neural networks are more intelligent | Dario Amodei and Lex Fridman
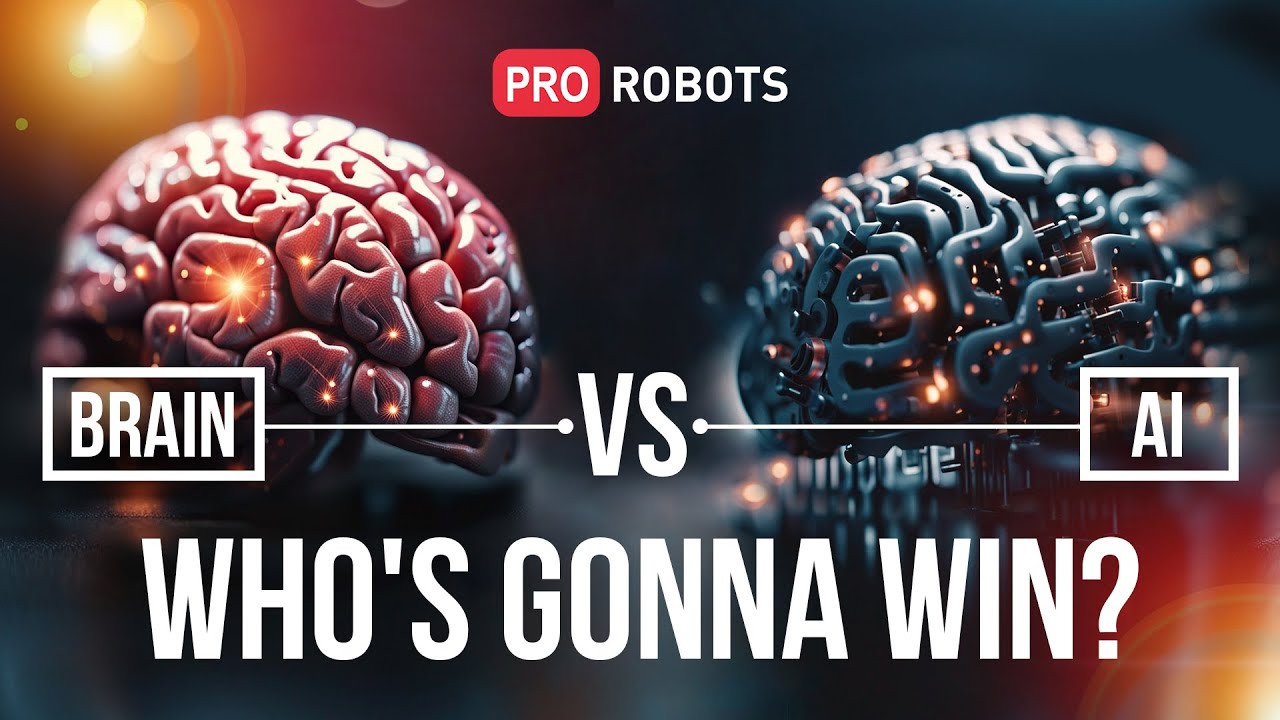
Human Brain vs. Artificial Intelligence: Reality and Prospects
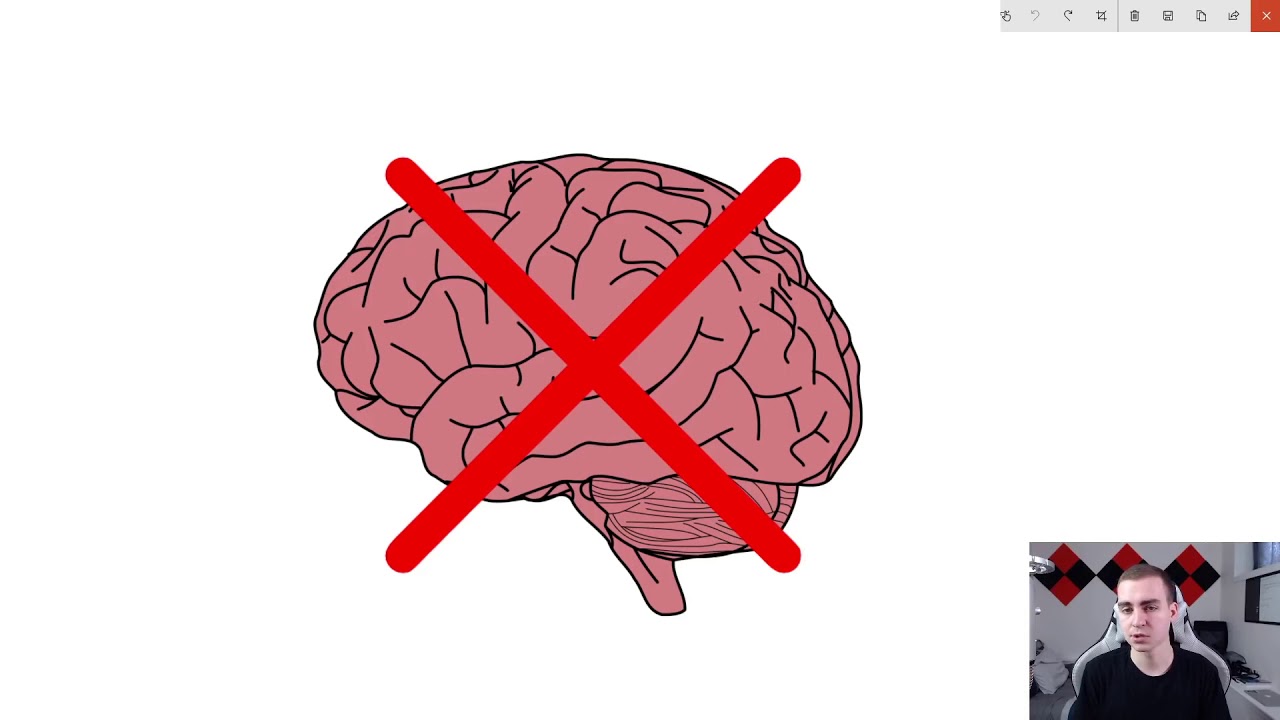
Machine Learning Fundamentals A - TensorFlow 2.0 Course
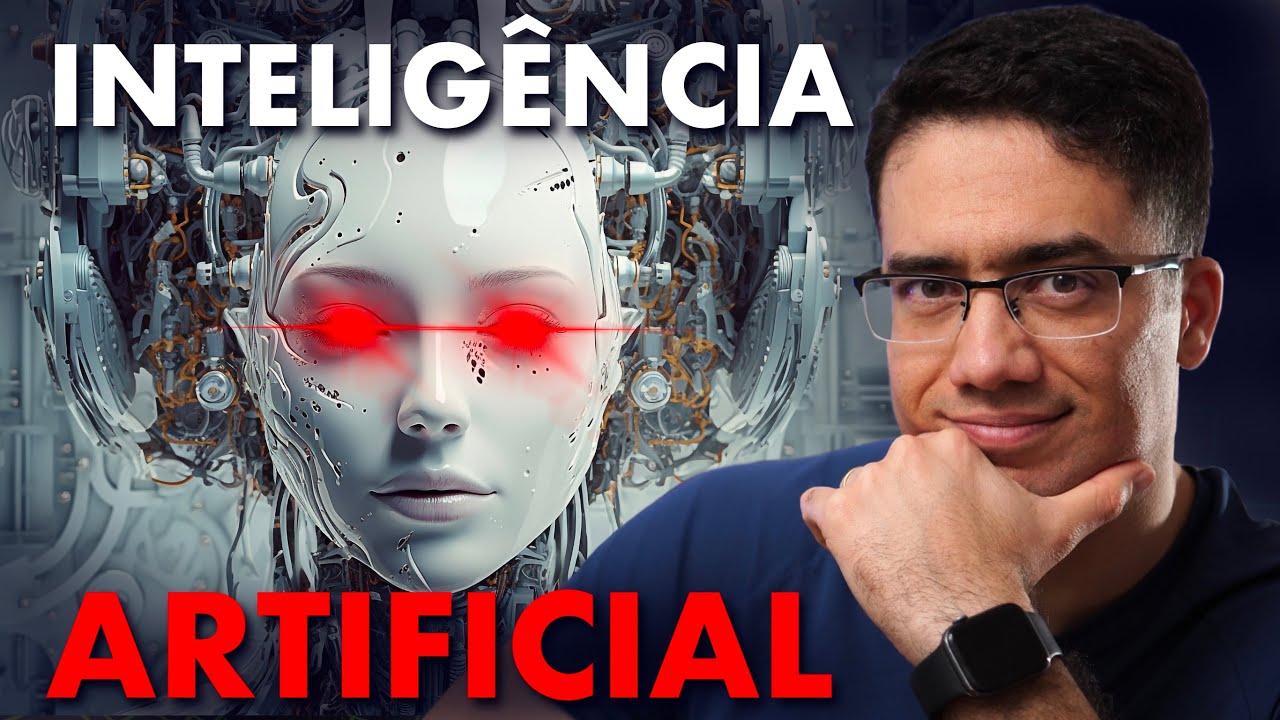
COMO a INTELIGÊNCIA ARTIFICIAL realmente FUNCIONA?
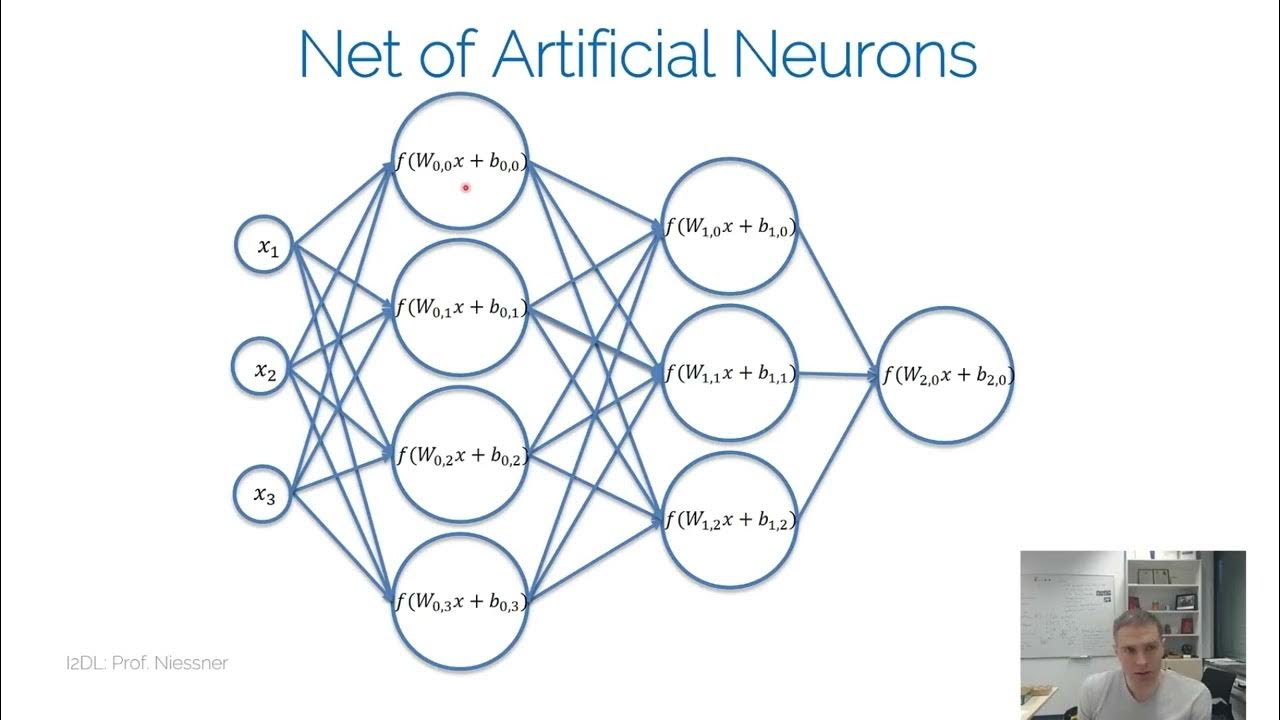
I2DL NN
5.0 / 5 (0 votes)