T-test, ANOVA and Chi Squared test made easy.
Summary
TLDRThis educational video explores various statistical tests and their appropriate applications. It delves into the t-test, covering single sample, two-tailed, one-tailed, and paired tests, using real-world examples to elucidate the concepts. The video then moves on to ANOVA, demonstrating how it allows for comparing means across three or more populations. Additionally, the chi-squared test is introduced, addressing both goodness of fit and test of independence scenarios, enabling analyses of categorical variables and proportions. Throughout the video, the presenter emphasizes the importance of understanding the research question and selecting the appropriate statistical test accordingly, making the material highly accessible and engaging.
Takeaways
- 🔑 The key to understanding statistical tests is to first understand the question being asked.
- 👤 For t-tests, we are examining the difference in means between two populations, or between one population at different points in time.
- 🤔 The null hypothesis assumes there is no difference in means, and we calculate the probability of observing our sample data if the null hypothesis were true.
- ✅ If this probability is below a predetermined threshold (typically 0.05), we reject the null hypothesis and conclude the observed difference is statistically significant.
- 📐 There are different types of t-tests: single sample, two-tailed, one-tailed, and paired t-tests, depending on the specific question being asked.
- ⚖️ ANOVA (Analysis of Variance) is used when comparing means across three or more populations.
- 🔍 After an ANOVA identifies a significant difference, multiple comparisons can pinpoint which populations differ from each other.
- 📊 Chi-squared tests are used to test for differences in proportions of categorical variables.
- ✔️ The chi-squared goodness-of-fit test examines if observed proportions match expected proportions.
- 🔗 The chi-squared test of independence checks if the proportions of one categorical variable depend on the values of another variable.
Q & A
What are the three main statistical tests covered in the video?
-The three main statistical tests covered in the video are the t-test, ANOVA (analysis of variance), and the chi-squared test.
What is the purpose of the t-test?
-The t-test is used to test the difference in means or averages between two populations, between one population at different points in time, or between a sample mean and a presumed or hypothesized mean.
What is the difference between a one-tailed and two-tailed t-test?
-A two-tailed t-test is used when we are asking if there is a difference in any direction, while a one-tailed t-test is used when we are asking if there is a difference in a particular direction.
When is a paired t-test used?
-A paired t-test is used when there are paired observations in each population, meaning that for each observation in one population, there is a corresponding observation in the other population.
What is the purpose of ANOVA?
-ANOVA (analysis of variance) is used to compare the means of three or more populations. It tests the null hypothesis that there are no differences in the means of these populations.
How can you determine which specific populations are driving the difference in means after conducting an ANOVA?
-After conducting an ANOVA and rejecting the null hypothesis, a multiple comparison of means (such as Tukey's test) can be performed to identify which specific populations have statistically significant differences in their means.
What is the chi-squared goodness of fit test used for?
-The chi-squared goodness of fit test is used to determine if the observed proportions of a categorical variable are significantly different from the expected proportions based on a hypothesized distribution.
What is the purpose of the chi-squared test of independence?
-The chi-squared test of independence is used to determine if there is a significant relationship between two categorical variables, i.e., if the proportions of one variable are independent of the other variable.
What is the null hypothesis being tested in a chi-squared test?
-In a chi-squared test, the null hypothesis is that there is no difference in proportions (for goodness of fit) or that the variables are independent of each other (for test of independence).
How is the decision to reject or accept the null hypothesis made in hypothesis testing?
-The decision to reject or accept the null hypothesis is made by comparing the calculated p-value to a predetermined significance level (typically 0.05). If the p-value is less than the significance level, the null hypothesis is rejected, indicating a statistically significant result.
Outlines
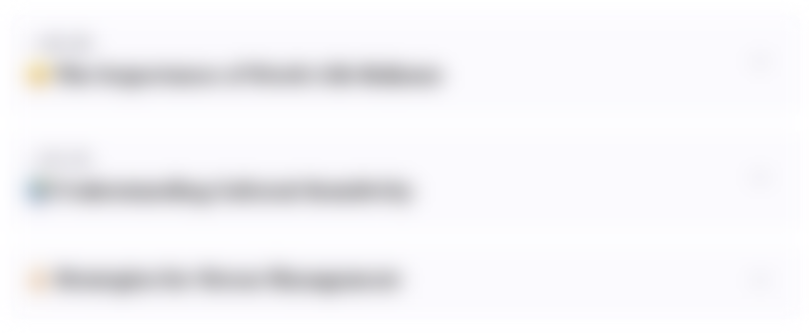
Cette section est réservée aux utilisateurs payants. Améliorez votre compte pour accéder à cette section.
Améliorer maintenantMindmap
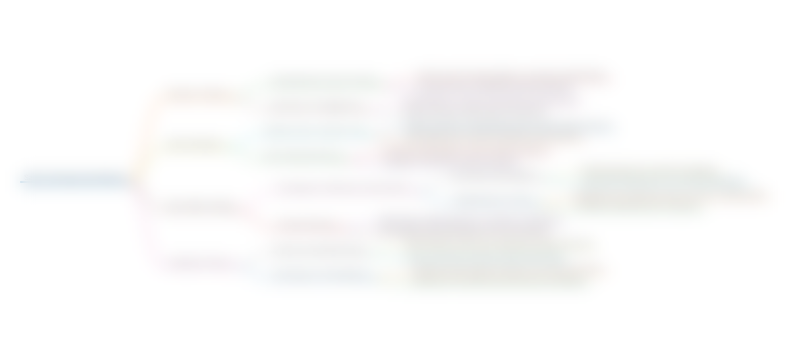
Cette section est réservée aux utilisateurs payants. Améliorez votre compte pour accéder à cette section.
Améliorer maintenantKeywords
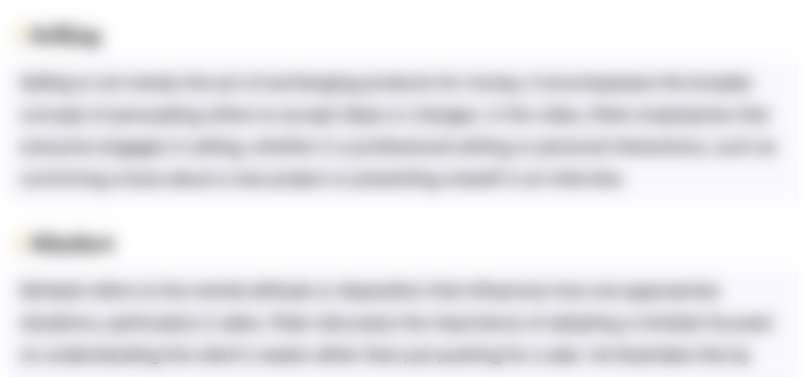
Cette section est réservée aux utilisateurs payants. Améliorez votre compte pour accéder à cette section.
Améliorer maintenantHighlights
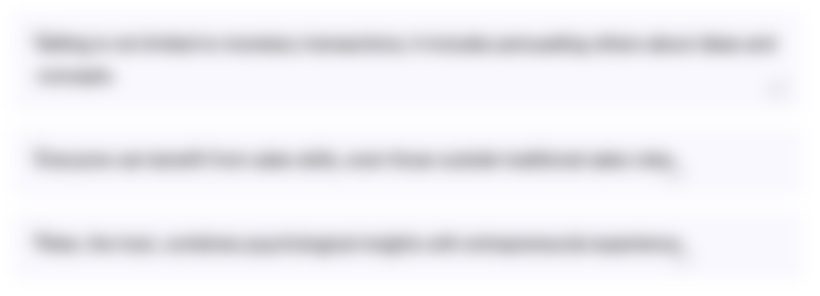
Cette section est réservée aux utilisateurs payants. Améliorez votre compte pour accéder à cette section.
Améliorer maintenantTranscripts
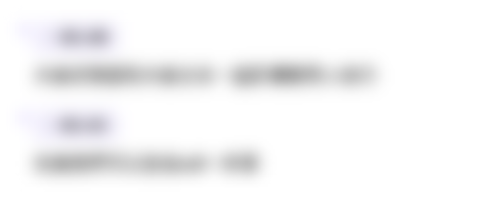
Cette section est réservée aux utilisateurs payants. Améliorez votre compte pour accéder à cette section.
Améliorer maintenantVoir Plus de Vidéos Connexes
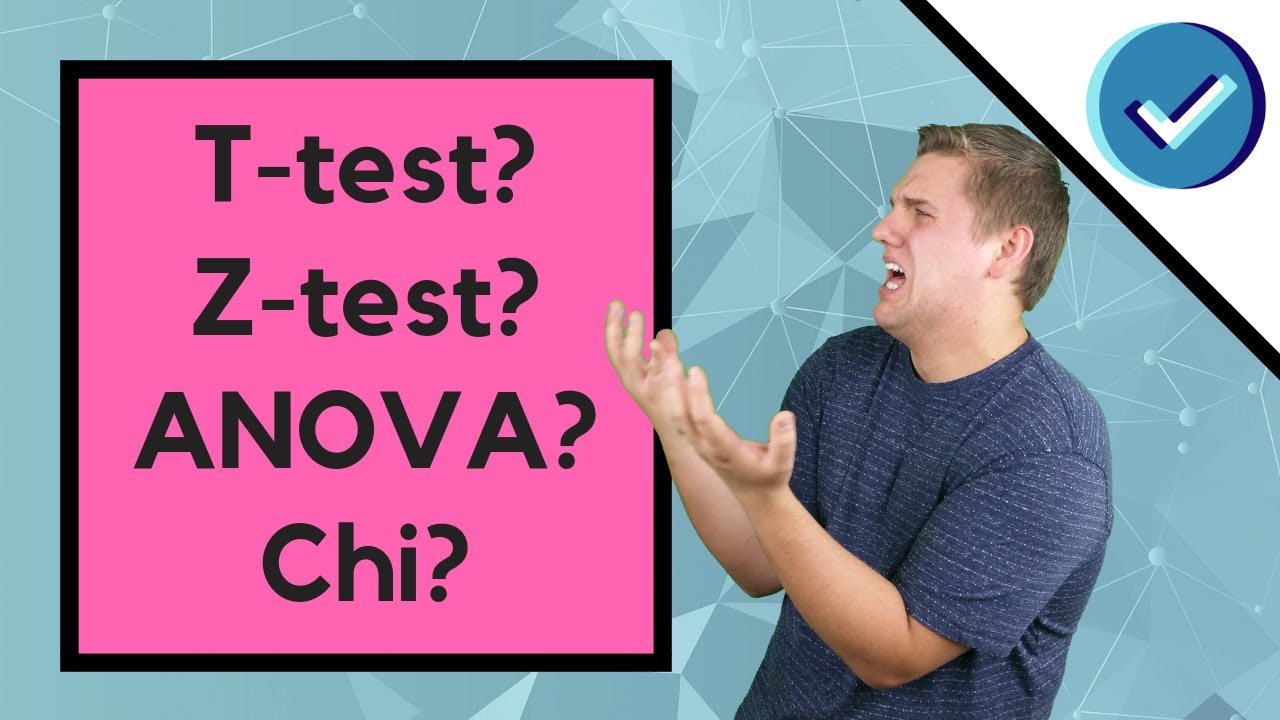
How To Know Which Statistical Test To Use For Hypothesis Testing
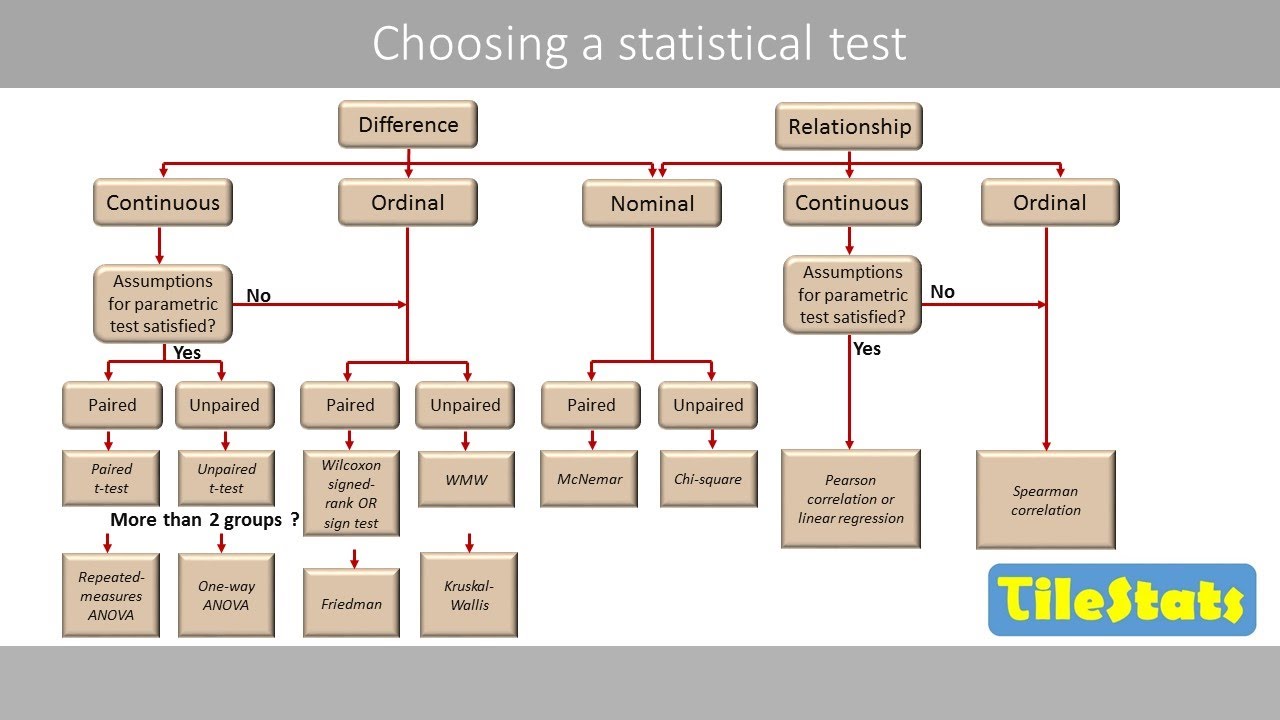
How to choose an appropriate statistical test
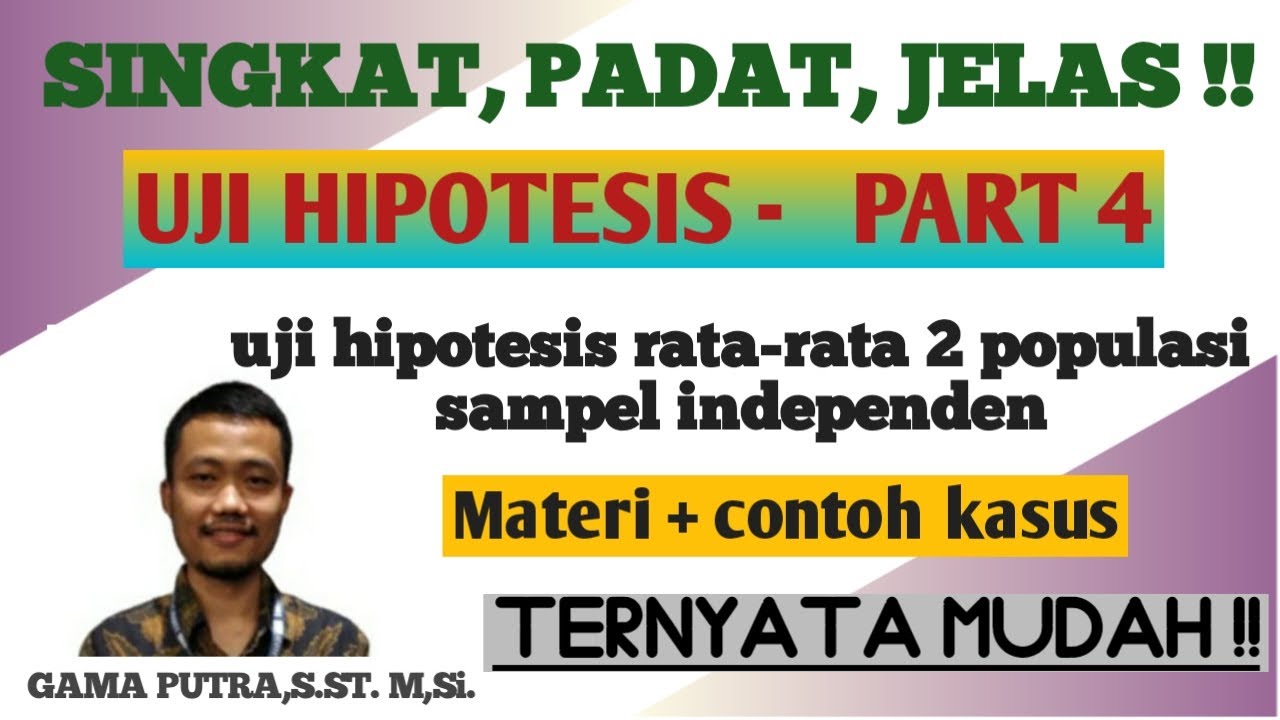
uji hipotesis rata-rata 2 populasi (sampel independen)

UJI BEDA LEBIH DARI 2 MEAN BERPASANGAN DAN TIDAK BERPASANGAN

Uji Hipotesis
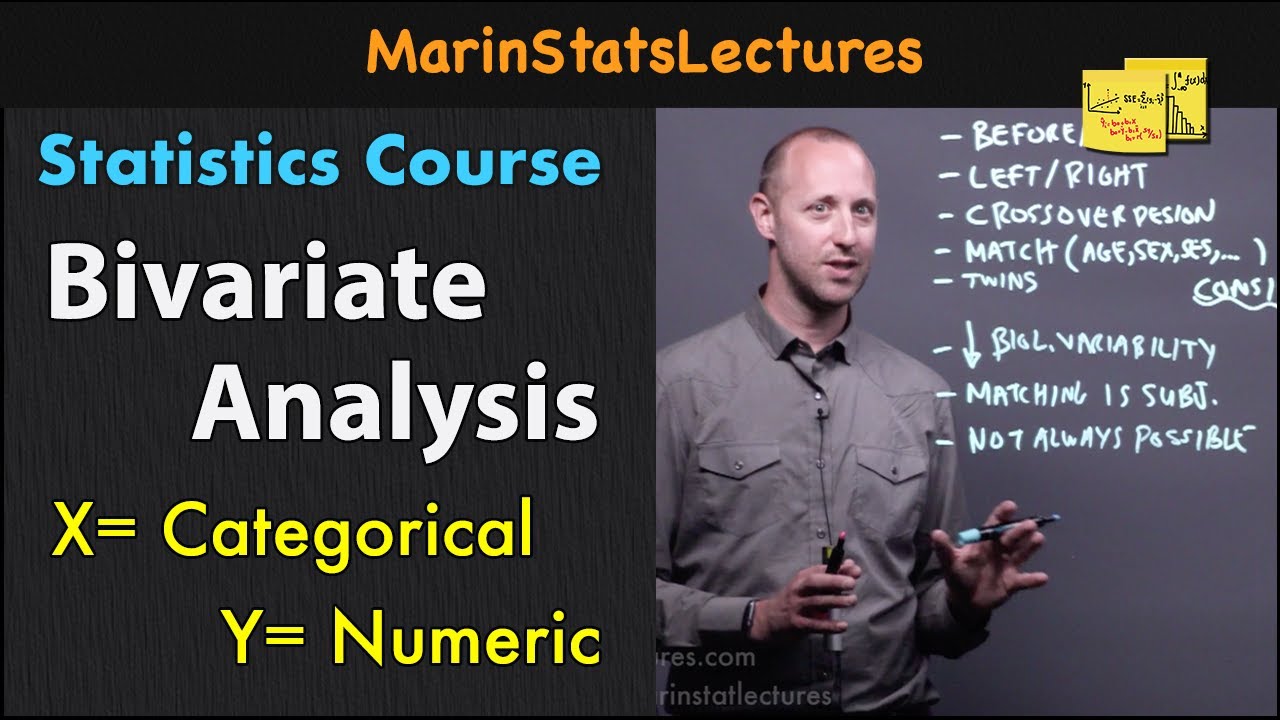
Bivariate Analysis for Categorical & Numerical | Statistics Tutorial #20 | MarinStatsLectures
5.0 / 5 (0 votes)