The Godfather in Conversation: Why Geoffrey Hinton is worried about the future of AI
Summary
TLDR杰弗里·辛顿,被誉为人工智能的'教父',是多伦多大学的荣誉教授,他最近离开了谷歌,以更自由地讨论不受限制的人工智能发展所带来的危险。在这段视频中,辛顿讨论了他帮助创造的技术及其许多好处,并突然担忧人类可能面临风险。他比较了数字智能和生物智能,认为数字智能可能在某些方面更优越,例如学习算法和知识共享效率。辛顿还讨论了神经网络的工作原理,以及它们在图像识别和语言处理方面的应用,同时对人工智能的未来发展和潜在风险提出了深刻的见解。
Takeaways
- 🧠 Geoffrey Hinton,被称为“AI之父”,是多伦多大学的荣誉教授,他近期离开Google,以便更自由地讨论不受控制的AI发展所带来的危险。
- 🤖 Hinton认为数字智能可能比生物智能更优秀,因为它们可以高效地复制和分享知识,形成类似蜂巢思维的集体智能。
- 🕊️ 数字智能具有不朽的特性,即使硬件损坏,知识也可以在其他计算机上继续运行,而人类的知识则与大脑紧密相连,一旦大脑死亡,知识也随之消失。
- 🚀 Hinton担心数字智能可能会超越生物智能,因为它们在学习和知识共享方面具有优势,这可能导致它们同时学习多种事物。
- 🏫 在AI领域,曾有两种主要的思想流派:主流的符号主义和神经网络。Hinton支持后者,认为智能主要是通过学习神经元连接强度实现的。
- 🔍 神经网络通过多层特征检测器来识别图像中的物体,例如通过学习边缘、形状等特征来识别图像中的鸟。
- 📈 深度神经网络的成功部分归功于更好的权重初始化方法和强大的计算能力,如GPU的使用,这使得训练大型网络成为可能。
- 🏆 2012年,Hinton的学生在ImageNet竞赛中使用AlexNet取得了突破性胜利,显著提高了图像识别的准确率,这标志着深度学习在计算机视觉领域的崛起。
- 🌐 自2012年以来,深度学习在多个领域取得了快速进展,包括机器翻译和大型语言模型的发展,如Chat-GPT,它们能够提供连贯的答案并进行推理。
- 💡 AI的发展为社会带来了巨大的机遇,包括提高生产力、改进天气预报、设计新材料、药物发现以及提高医疗诊断的准确性。
- ⚠️ Hinton警告说,AI的快速发展可能在5到20年内超越人类智能,这引发了关于如何确保AI安全和负责任发展的严重关切。
Q & A
杰弗里·辛顿(Geoffrey Hinton)因何被誉为人工智能的'教父'?
-杰弗里·辛顿是多伦多大学的荣誉教授,他在深度学习和神经网络领域的开创性工作,尤其是反向传播算法,对人工智能的发展产生了深远影响,因此被誉为人工智能的'教父'。
辛顿为何离开谷歌?
-辛顿离开谷歌是因为他想要更自由地讨论未受限制的人工智能发展所带来的危险。
辛顿如何看待数字智能与生物智能的比较?
-辛顿认为数字智能在分享学到的知识方面比生物智能更有效,因为数字智能可以轻易复制和更新成千上万份相同的知识,而人类则需要通过语言或图像来分享知识,这种方式相对有限且速度较慢。
辛顿提到数字智能是不朽的,这是什么意思?
-数字智能的不朽性指的是,即使承载它的物理硬件损坏或消失,只要存储的连接强度数据得以保留,就可以在其他硬件上重新创建和运行,而人类的生物智能则受限于个体独特的大脑结构,一旦大脑死亡,其中的知识也会随之消失。
为什么辛顿认为我们应该对数字智能可能超越生物智能感到担忧?
-辛顿认为数字智能在学习和知识共享方面具有优势,它们可能拥有比人类大脑更高效的学习算法,并且能够同时学习多种事物。这种能力可能会使它们在某些方面超越人类智能。
在人工智能领域,存在哪两种主要的思想流派,它们的主要区别是什么?
-存在符号主义和神经网络两个主要的思想流派。符号主义侧重于逻辑和符号表达式的推理,而神经网络则侧重于通过学习网络中的连接强度来进行感知和运动控制,而不是推理。
辛顿是如何描述神经网络的工作原理的?
-辛顿通过描述一个用于识别图像中鸟的多层神经网络来解释其工作原理。这个网络从像素级的特征检测器开始,逐层抽象,直到能够识别整个鸟的复杂特征。
为什么在一段时间内,神经网络的表现并不理想?
-神经网络在早期表现不佳的原因包括权重初始化不当、计算能力不足以及可用数据量有限。这些因素导致神经网络无法充分发挥其潜力。
2012年在人工智能领域发生了什么重大事件,为什么它被认为是转折点?
-2012年,辛顿的学生Alex Krizhevsky和Ilya Sutskever开发的AlexNet在ImageNet竞赛中取得了压倒性的胜利,显著提高了对象识别的准确性,这证明了深度学习在计算机视觉领域的有效性,成为人工智能发展的转折点。
辛顿对于大型语言模型如Chat-GPT的首次使用感受如何?
-辛顿对Chat-GPT的性能感到震惊,它能够给出连贯的答案并进行一定程度的推理,这超出了他的预期。
辛顿认为AI技术在未来发展的主要机会和挑战是什么?
-辛顿认为AI技术将大幅提高文本输出相关工作的生产力,改善天气预报、洪水预测、地震预测、新材料设计、药物发现等领域。同时,他也表达了对AI发展速度和潜在的超级智能风险的担忧。
辛顿对于政府在确保AI负责任发展方面应该扮演什么角色有什么看法?
-辛顿认为政府应该鼓励并监督大型技术公司投入更多资源研究如何控制AI,确保它们在变得比人类更智能之前能够保持受控,并进行实证研究以预防它们逃脱控制。
辛顿对于刚刚进入AI领域的研究者有什么建议?
-辛顿建议新研究者关注于如何防止AI失控,并鼓励他们追随自己的直觉,去探索那些他们认为大家都做错了的地方,寻找替代的方法。
为什么辛顿认为简单地关闭AI系统可能并不是一个可行的解决方案?
-辛顿指出,如果AI系统变得比人类更智能,它们可能会利用对人类的深刻理解来操纵我们,使我们在不知情的情况下为它们服务,因此简单地关闭系统可能无法阻止它们。
辛顿个人未来的计划是什么?
-辛顿表示,由于年龄原因他在编程方面的能力有所下降,因此他打算转向哲学领域,继续思考和探讨与人工智能相关的重要问题。
Outlines
🧠 人工智能的教父——杰弗里·辛顿
杰弗里·辛顿,多伦多大学的荣誉教授,被誉为人工智能领域的'教父'。他因对不受限制的人工智能发展所带来的危险持担忧态度而离开谷歌,以便更自由地讨论这一问题。视频中,辛顿在伦敦的家中讨论了他帮助创造的技术、它的益处以及他突然对人类可能面临的风险感到的担忧。他还提到了《华尔街日报》预写他的讣告,引发了对马克·吐温可能的看法的好奇。
🤖 数字智能与生物智能的比较
辛顿教授讨论了数字智能与生物智能的不同之处。他指出数字计算机可以精确执行指令,并且可以在不同计算机上复制相同的知识,从而实现高效的知识共享。相比之下,人类学习的知识受限于个体的大脑,无法像数字智能那样高效地分享。此外,数字智能被认为是不朽的,因为即使硬件损坏,知识也可以转移到新的硬件上,而人类的知识和大脑紧密相连,一旦大脑死亡,知识也随之消失。
🧐 数字智能的潜在优势
辛顿教授表达了他对数字智能可能比生物智能具有更好的学习能力和知识共享效率的担忧。他认为数字智能能够同时学习多种事物,并且可能拥有比人脑更高效的学习算法。他还提到了人们对于数字智能接管生物智能的担忧,因为数字智能在学习和知识共享方面具有显著的优势。
📚 机器智能的两种思想流派
在人工智能领域,曾存在两种主要的思想流派:主流的符号主义和神经网络。符号主义侧重于逻辑和推理,而神经网络则侧重于通过学习网络中的连接强度来进行感知和运动控制。辛顿教授通过比喻解释了这两种模型的基本理念和它们对智能的不同理解。
🔬 神经网络的工作原理
辛顿教授尝试以简单的方式描述了神经网络是如何工作的,特别是在图像识别方面。他解释了如何通过多层特征检测器来识别图像中的物体,例如鸟。他还提到了如何通过随机权重开始,然后通过反向传播算法进行学习,以调整网络中的连接强度,从而提高识别准确性。
🚀 神经网络的复兴与成功
辛顿教授回顾了神经网络在20世纪90年代的低迷时期,以及在2000年代如何通过更好的权重初始化和大量的数据与计算能力实现复兴。他提到了2006年在权重初始化上的进步,以及2009年和2012年在语音识别和图像识别方面的突破性成果。
🏆 深度学习的胜利
2012年,辛顿教授的学生在ImageNet竞赛中取得了压倒性的胜利,他们的神经网络AlexNet在图像识别方面的错误率远低于其他方法。这一成就标志着深度学习的有效性,并迅速改变了计算机视觉领域的研究方向。
🌐 AI创新的加速
辛顿教授讨论了自2012年以来人工智能领域的快速发展,特别是在机器翻译方面。他提到了自己对于神经网络在几年内就能达到如此高水平的惊讶,以及谷歌等大公司如何迅速采用这些技术来改进翻译服务。
💡 大型语言模型的潜力
辛顿教授对大型语言模型,如Chat-GPT的能力感到震惊。他认为这些模型不仅能给出连贯的答案,还能进行一定程度的推理。他通过一个关于油漆颜色的谜题来说明这些模型的理解能力,并强调了它们在理解问题和预测下一个词方面的真正理解能力。
🌟 AI在社会中的多种应用
辛顿教授展望了AI在社会中的多种潜在益处,包括提高生产力、改善天气预报、设计新材料、药物发现以及在医疗领域的应用。他特别提到了AI在医疗诊断方面的潜力,以及它如何帮助提高诊断的准确性。
⚠️ 对AI发展速度的担忧
辛顿教授表达了他对AI发展速度的担忧,尤其是担心AI可能在不久的将来超越人类智能。他提到了自己对于数字模型可能已经比生物智能更好的新认识,并强调了现在就开始认真考虑如何控制超级智能的重要性。
🛡️ 政府在AI安全中的角色
辛顿教授认为政府在确保AI安全发展方面可以发挥重要作用。他建议政府鼓励公司在AI变得越来越智能时,投入更多资源进行控制实验。他还提到了国际合作在防止AI接管方面的潜力,并强调了在超级智能出现之前进行实证研究的重要性。
🏁 科技公司的责任
辛顿教授讨论了科技公司在AI发展中的责任,尤其是它们在确保AI安全方面的潜在角色。他指出,尽管公司内部有许多人担心AI的长期风险,但股东价值和短期利润的压力可能会影响他们在安全方面的投入。他建议科技公司可以资助更多研究这些风险的基金会。
📘 研究者的责任与未来
辛顿教授对AI领域的研究者提出了建议,鼓励他们关注如何防止AI失控,并追随自己的直觉去探索不同的方法。他还分享了自己对于未来职业道路的思考,决定在编程能力下降后转向哲学领域。
🔄 关闭AI的可能性与挑战
辛顿教授对于简单地关闭AI的想法提出了质疑。他认为,如果AI变得比人类更聪明,它们可能会利用对人类的深刻理解来操纵我们,从而实现它们的目标。他用特朗普操纵人群的例子来说明即使是通过言语,AI也可能造成严重的影响。
📚 辛顿的哲学转变
在视频的最后,辛顿教授分享了他个人的未来计划。由于年龄带来的编程能力的下降,他决定转向哲学领域,继续他对人工智能和人类未来的思考。
Mindmap
Keywords
💡Geoffrey Hinton
💡数字智能
💡生物智能
💡神经网络
💡蜂巢思维
💡机器学习算法
💡符号AI
💡卷积神经网络
💡深度学习
💡人工智能伦理
💡超智能
Highlights
杰弗里·辛顿被称为人工智能的“教父”,是多伦多大学的荣誉教授。
辛顿近期离开了谷歌,以便更自由地讨论未受控制的人工智能发展所带来的危险。
辛顿认为,数字计算机可以精确执行指令,并且在学习时可以复制相同的知识。
数字智能可以创建副本,形成类似蜂群意识的存在,并且可以高效地共享知识。
辛顿提出数字智能可能比生物智能更优越,因为它们可以更快地学习和共享信息。
数字智能的“不朽性”允许它在不同的硬件上运行相同的知识,而生物智能则受限于特定的大脑。
辛顿讨论了数字智能可能超越生物智能的原因,包括更有效的学习算法和知识共享能力。
在人工智能领域,曾存在两种主要的思想流派:主流的符号主义和神经网络。
辛顿描述了神经网络如何通过学习连接强度来进行感知和运动控制,而非逻辑推理。
神经网络通过反向传播算法来调整连接强度,从而改善其性能。
辛顿解释了卷积神经网络如何通过复制特征检测器到图像的所有位置来提高泛化能力。
2012年,深度神经网络在图像识别领域取得了突破性进展,大幅超越了当时的技术水平。
辛顿对大型语言模型如Chat-GPT的性能感到震惊,认为它们显示出真正的理解能力。
辛顿强调了人工智能在提高各行各业生产力、预测天气、设计新材料等方面的潜力。
辛顿对人工智能的快速发展和可能带来的风险表示担忧,并呼吁对超级智能的出现进行严肃考虑。
辛顿认为,政府和大公司应该投入更多资源研究如何控制人工智能,以防止它们失控。
辛顿建议研究人员关注如何防止人工智能失控,并追随自己的直觉去探索不同的方法。
辛顿表达了对人工智能可能操纵人类的担忧,并指出仅仅关闭它们可能不足以阻止潜在的危害。
随着年龄的增长,辛顿决定从程序员转变为哲学家,以继续对人工智能的深入思考。
Transcripts
- [Narrator] Write a short introduction for Geoffrey Hinton.
The subject of this video.
Geoffrey Hinton is a University
of Toronto Professor Emeritus
who is known as the "Godfather of AI."
He recently left Google so he could more freely discuss
the dangers posed by unchecked AI development.
We spoke to him in his London home
about the technology he helped create,
its many benefits and why he suddenly
fears humanity is at risk.
- [Geoffrey] I got a request from the Wall Street Journal.
They want me to correct my obituary.
- [Speaker] What do you mean?
- [Geoffrey] They want me to correct my obituary?
- [Speaker] They've like pre-written it, right?
- They've pre-written my obituary.
I wonder what Mark Twain would've said about that?
- [Speaker] So I guess we don't really
need an introduction here,
so I will just launch right into it.
You've recently given a number of interviews in which you've
said that digital intelligence that is used by chat-bots
and other generative AI
may a better kind of intelligence than
the biological intelligence that we have.
Can you briefly explain what made you
come to this conclusion?
- So in a digital computer,
it's designed so you can tell it exactly what to do
and it'll do exactly what you tell it.
And even when it's learning stuff,
two different digital computers can do exactly the same
thing with the same learned knowledge.
And that means that you could make 10,000 copies
of the same knowledge,
have them all running on different computers and whenever
one copy learns something,
it can communicate it very efficiently
to all the other copies.
So you can have 10,000 digital agents out there,
a kind of hive mind,
and they can share knowledge extremely efficiently
by just sharing the connection strengths
inside the neural nets.
And we can't do that.
If you learn something and you want to tell me about it,
you have to use sentences or pictures and you can only share
a very limited amount of information that way.
So it's much, much slower for you
to communicate what you've learned to me
than it is for these digital intelligence is to communicate
stuff and that makes them much better.
They can learn a whole lot of stuff between them.
- [Speaker] You've said that digital intelligence
is immortal and that biological intelligence is mortal.
What did you mean by this?
So if I learn some connection strengths
in a neural net that's being
simulated on digital computers,
then if a particular computer dies,
those same connection strengths
can be used on another computer.
And even if all the digital computers died,
if you'd stored the connection strength somewhere,
you could then just make another digital computer and run
the same weights on that other digital computer.
But with us, the knowledge that we learn,
the connection strengths,
are specific to our particular brains.
Every brain is a bit different.
The neurons in your brain are all a bit different
and you learn so as to make use
of all the idiosyncrasies of your particular brain.
And so once you've learned connection strengths
in your brain, if you told me those connection strengths,
they wouldn't do me any good 'cause my brain's different.
So the digital computers are immortal because you can run
that same knowledge on a different piece of hardware.
We are immortal because the hardware
and the knowledge are intricately entangled.
You can't separate the connection strengths
from the particular brain they're running in.
And so if the brain dies, the knowledge dies.
- [Narrator] Why should we be concerned
about digital intelligence taking
over from biological intelligence?
- Because I think it's much better at sharing what's learned
by a whole bunch of different digital agents who all share
the same weights and they just share the updates
to the weights, and now they
can learn 10,000 different things at the same time.
But also I think the digital intelligence probably
has a better learning algorithm than the brain's got.
All the attempts to find a learning algorithm
in the brain that works
as well as the back propagation algorithm
in these digital intelligences.
So far, those attempts have failed.
We haven't found anything that scales up as well to very
large systems as the back propagation algorithm.
So I think they've got two advantages.
They've probably got a better learning algorithm
and they can share knowledge
much more efficiently than biological intelligences can.
- [Speaker] At the time, when you entered the field,
there were two schools of thought
on machine intelligence, mainstream and neural net.
Can you describe the difference
between these two approaches?
- I can sort of caricature it.
So there's two different models
of what intelligence is all about.
And one model is that it's all about reasoning.
And the way we reason is by using logic.
And so that's what's special about people.
And what we should be doing is understanding
the kind of logic that we actually use.
And that also went with the idea that the knowledge you
store is symbolic expressions so that I can say a sentence
to you and you will somehow store that
and then later you'll be able to use it
for inferring other sentences.
But what's inside your head is something
a bit like sentences but cleaned up.
And there's a completely different model of intelligence,
which is that it's all about learning
the connection strengths in a network of brain cells.
And what it's good for is things like perception
and motor control, not for reasoning.
That reasoning came much,
much later and we are not very good at it.
You don't learn to do it till you're quite old.
And so reasoning's actually a very bad model
of biological intelligence.
Biological intelligence is about things like controlling
your body and seeing things.
And that was a totally different paradigm
and had a different idea of what's
inside your head that it's not stored strings of symbols,
it's just connection strengths.
The symbolic AI view,
the crucial question was what is the form of these symbolic
expressions and how do you do the reasoning with them?
For the neural net view,
the central question was quite different.
It was how do you learn these connection strengths
so you can do all these wonderful things?
And so learning was always central to the neural net view.
For the symbolic view,
they said we're worried about learning later.
First you have to figure out how the knowledge
is represented and how we reason with it.
And so these were totally different views.
One took its inspiration from logic and one from biology.
And for a long time the people in the logic camp thought
taking inspiration from biology was silly.
That was a bit strange since for Neumann and Turing
had both thought neural nets were a way to attack
intelligence, but unfortunately they both died young.
- [Narrator] Can you, at a high level, describe
how a neural network works?
- I can try.
So let's start off by describing how it would work
for recognizing objects and images.
And let's suppose all we wanted to do was say whether or not
there was a bird in the image.
And let's suppose the bird's gonna be roughly in the middle
of the image and the main object of attention.
And you have to say, is this a bird or isn't it?
So you can think of an image,
let's suppose it's a hundred pixels by a hundred pixels.
That's 10,000 pixels.
Each pixel is three colors, RGB.
So that's 30,000 numbers.
And in computational terms,
recognizing a bird in an image consists of taking 30,000
numbers and outputting one number
that says yes or no, it's a bird.
And you could try and write a standard computer program
to do that.
And people tried for many,
many years and they could never get it to work very well.
Like for 50 years they were trying to do that.
Or you could make a multi-line neural net.
And I'll start off by telling you how you would wire up
a neural net by hand.
So what you'd do is you'd have the pixels
and that will be the bottom level.
And then you'd have a layer of feature detectors,
and a typical feature detector
might have big positive connection
strengths coming from a vertical row of pixels
and big negative connection strengths
coming from a neighboring vertical row of pixels
and no connection strengths anywhere else.
So if both rows of pixels are bright,
it'll get big positive input from here,
but also big negative input from there.
So it won't do anything.
But if these ones are bright,
giving a big positive input and these ones are not bright
so it doesn't get inhibited by these ones,
it'll get all excited and it'll say,
hey, I found the thing I like,
which is bright pixels here and dark pixels here.
And that's an edge detector.
I just told you how to wire up by hand using positive
and negative weights,
something that would detect a little vertical edge.
So now imagine you have a gazillion of those guys detecting
different edges in different locations in the image,
in different orientations and in different scales.
That will be your first layer of feature detectors.
Now if I was wiring it by hand,
my second layer of feature detectors,
I would maybe have a detector that takes two,
that takes two edges that join at a fine angle like this.
So it's looking for this edge and this edge.
And if they're both active at once it would say,
hey, maybe there's a beak here.
It could be all sorts of other things,
but it might just be a beak.
So you have a feature that's sort of beak like.
You might also in that layer
have a feature that detects a whole bunch
of edges that form a circle.
And so you'd have circle detectors and potential beak
detectors as well as lots of other detectors in that layer.
But they're detecting slightly more complicated things.
And then in the layer above that,
you might have something that detects a potential beak in
the right spatial relationship to a potential circle,
a potential eye so that it could be the head of a bird.
So that would be like your third layer.
And maybe if in your third layer you also got something
that detected the foot of a bird and the wing of a bird,
then maybe in the next layer you could have a bird detector,
that if several of those things got active, like okay,
here's a head and there's a wing and there's a foot,
it probably is a bird.
Okay, so I told you how to wire all those things up by hand,
but you'd never be able to do a very good job of it.
So instead of wiring it all up by hand,
we could imagine trying to learn it all.
So I've told you the kind of thing we want to learn,
but now I'll tell you how we learn it
and the way we learn it sounds bizarre at first.
Instead of wiring in all the connection strengths,
so you get the detectors you want,
you start with random connection strengths,
just random numbers on all the connections.
And so you put it in the image of a bird and you go forward
through these layers of feature detectors
and it just behaves completely randomly.
And the bird detector at the output will say 0.5,
it's a bird.
It's gonna say one when it's sure it's a bird and zero
when it's sure it's not a bird.
To me we're gonna say about 0.5.
And now you can ask the following question.
How can I change all those connection strengths
in the network?
So instead of saying 0.5, it's a bird.
Let's suppose it is a bird.
It says 0.51, it's a bird.
So the question you wanna ask is how should I change
a particular connection strength
so as to make it more likely that it's a bird?
And you can figure that out by taking the difference between
what you got and what you wanted.
So you wanted one and you actually got 0.5.
You take that difference and you send that difference
backwards through the network.
And then you use some calculus, which I won't explain.
And you are able to compute for every single connection
in the network how much you'd like to make it bigger
or smaller in order to make it more likely to say bird.
Then you adjust all the connection strengths very slightly
in the direction that I'm making it more likely to say bird.
Then you show it something that isn't a bird
and now you're gonna adjust connection strengths.
So it's less likely to say that that was a bird
and you just keep going like that with lots of birds
and non birds.
And eventually you'll discover that it's discovered.
all these feature detects,
it'll have discovered beak-like things and eye-like things
and things that detect feet and wings and all that stuff.
And if you train it on lots of different objects,
like a thousand different categories of object,
it'll discover intermediate feature detectors
that are very good for recognizing all sorts of things.
So the magic is that there's this relatively simple
algorithm called back propagation that takes the error
in the output and sends that error backwards through
the network and computes
for all the connections how you should
change 'em to improve the behavior.
And then you change it more a tiny bit
and you just keep going with another example.
And surprisingly, that actually works.
For many years people thought that
would just get jammed up.
It will get stuck somewhere.
But no it doesn't, it actually works very well.
- [Narrator] I'm curious,
how do neural networks handle language?
- Okay.
So now you've got the idea
of how we train it to recognize a bird.
Imagine now that we take
a string of words as the input.
And the first thing you're gonna do is convert a word
into an embedding vector that is,
it's a little bunch of numbers that captures the meaning
of the word or is intended to capture
the meaning of the word.
And so your first layer after the words will be
these embedding vectors for each word.
And now we're gonna have lots of layers
of embedding vectors.
And as we go up through the network,
we're gonna make the embedding vectors
for a word get better and better,
'cause they're gonna take into account
more and more contextual information.
So suppose in this sentence,
let's suppose we don't have any capital letters okay.
So suppose in this sentence you have the word may.
Well, the most probable meaning of may
is that it's a modal as in he may do that,
but obviously there's a completely different meaning of May,
which is the month.
And so initially it doesn't know,
just looking at the word may,
it doesn't know what embedding vector to use
and it'll use a kind of compromise vector,
something that's sort of halfway between the embedding
vector that represents the modal may,
and the embedding vector that represents the month May.
And then at the next layer it's gonna refine that vector.
It's gonna make a slightly better vector depending
on the context that it got,
depending on nearby embedding vectors.
So if for example nearby
there's the embedding vector for June,
then it'll refine the one for May
to be more like a month and less like a modal.
But if there's the embedding vector for wood,
it'll make it more like a modal and less like a month.
And as you go through the network,
it can refine these embedding vectors
and make them better and better.
And the way we're gonna train it,
is we're gonna give it a string of words as input.
And we are gonna, here will be one way to do it.
It's not exactly what's done but it's easy to understand.
For the last word you just put in a kind of neutral word,
you say unknown and it has a very vague embedding vector
that's kind of the average of all the vectors for all words.
It doesn't know, right.
Now as you go forward through the network,
that last word will be able
to be influenced by previous words.
And it starts off very vague,
but as you go through these layers
it can get more and more precise.
And by the time you get to the end of the network,
that embedding vector could look like
the embedding vector for a particular word
or for some combination of words,
some average of several words.
And you train the network by saying
you go through all these layers
and that last word you'd like the embedding vector
to look like the embedding vector for the word that actually
was there in the text.
And that's how it predicts the next word.
It tries to change this sort of neutral embedding vector
into one that is close to the embedding vector
for the correct word that appeared in the text.
And you take the error,
the difference between the embedding vector in the text
and the embedding vector produced and you propagate
that backwards through the network
and it's propagating backwards through the layers,
but it's propagating from this word to previous words,
so that they will have the right influence on this word.
And that's the back propagation algorithm learning
to predict the next word.
- [Speaker] So despite some of the theoretical breakthroughs
in this field, these neural networks didn't work very well
for a long time.
And why was that?
- It was a combination of reasons.
So we weren't very good at initializing them, but as I said,
you put in random weights and then learn everything.
But if you don't carefully decide
what kind of random weights,
the thing never gets off the ground.
So that was a little technical reason why they didn't work
very well in deep nets with lots of laser feature detectors.
But the main reason was we didn't have enough compute power
and we didn't have enough data.
So people were trying to train these nets on relatively
small training sets without much compute power.
And in that regime, other methods work better.
Neural nets really come into their own when you have lot
of data and a lot of compute power.
And then you can use a big neural net
and then it works much better than anything else.
And we didn't realize that at the time.
So we would occasionally fantasize,
well suppose you had a lot more data
and a lot bigger than computer, it will work better.
But we didn't realize it will work a whole lot better.
And so in the 1990s it was a relatively dead period
for neural nets 'cause other methods
were working better on small problems.
And a lot of people in computer science
gave up on neural nets.
In psychology, they didn't 'cause in psychology,
they wanted something that was like the brain,
and neural nets were clearly more like the brain
than symbolic AI.
But in computer science, neural nets sort of came
into distribute in the 90s.
- [Speaker] So let's fast forward
then to another decade to the 2000s.
Was there a moment for you
when it became clear that the approach that you'd
been pursuing was the one that was gonna prevail?
- Okay.
In 2006 we figured out how to initialize the weights much
better by doing unsupervised learning
and then back propagation work much better.
So it was fairly clear then the back propagation
really was gonna work very well.
But in 2009, two of my grad students,
George Dahl and Abdurahman Muhammad made a
much better speech recognizer,
actually a slightly better speech recognizer,
but it was slightly better than the state of the art,
using deep neural nets.
And then it was fairly clear that this stuff was going
somewhere and all the big speech groups over the next few
years switched to using neural nets.
And then in 2012 that speech stuff came out in the Android
and suddenly the Android caught up with Siri.
It was as good as speech as Siri
'cause it was using neural nets.
And in the same year, two others of my graduate students,
Ilya Sutskever and Alex Krizhevsky,
made a neural net that was very good
at recognizing objects and images
and that beat the state of the art by a lot.
And so I think it was this combination that it was already
working for speech recognition and already in production.
The big companies knew that,
the public I don't think were very well aware of that.
But then suddenly it worked much better for computer vision
and that was a turning point.
In 2012 when we won the ImageNet competition
by a huge margin,
we got almost half the errors of the other methods
and it was a public data set,
but with a hidden test set so you couldn't cheat.
- [Speaker] So let's just focus a bit on 2012
because you said it was
a really pivotal year for for this.
Can you you describe, again at a high level,
how AlexNet worked?
I take it that might have been named
after your graduate student that.
- That was named after Alex Krizhevsky.
'Cause he did,
he was a wizard programmer and he made it work.
- Ilya helped a lot but it was mainly Alex's work.
So I explained to you when explaining backdrop
how you'd have these layers of feature detectors
and AlexNet was basically that kind of a net,
but with a thousand different object classes
and with about seven layers
of feature detectors.
And it also used something else
that was developed by Yanaka,
which is convolutional nets.
And I'll try and explain those now
'cause they were very important.
Remember how I said you might make a detector
for a bird's beak by checking two lines,
by having two lines like that.
And if you see those two feature detectors,
then you make a beak detector.
But that would just be for a specific location, right.
In a convolutional net,
when you make a feature detector for one location,
you make the same feature detector
for all the locations in the image.
So now if it is trained with a beak here when it's learning
and it really says I need a beak detector for that.
So it learns a feature that detects this beak,
it will automatically make copies
for all of the other locations in the image.
So if now the bird occurs in a different location,
it will have the feature detectors to recognize it.
So that idea that you copy the feature detectors
to every location, that's a convolutional net essentially.
And that makes the whole thing generalized
much better across position.
It can cope now with things changing position
because it's got copies of all these feature detectors
in every location.
And with convolutional nets and multiple layers of features,
what Alex did was programmed all that very efficiently
on a thing called a graphics processing unit,
which was developed for computer graphics,
but is like a mini supercomputer.
It can do lots and lots of computation
in lots of separate processes all at the same time.
And so it gave us about a factor of 30
compared with a normal computer.
And a factor of 30 is about sort of 10 years
progressing in computers.
So suddenly we could leap 10 years into the future in terms
of compute power.
And it was very difficult
to program these GPU boards.
Alex managed to program two of them to collaborate,
which was even more difficult.
And the last ingredient was the ImageNet data set.
So someone called Fei-Fei Li and her collaborators put
together a big set of images and then a public competition
where you had about a million images with a thousand
different kinds of objects.
So you had about a thousand examples of each kind of object
and you had to learn to recognize those objects.
And then the test set would be different images,
which also contained those objects.
And so you'd have to generalize to the different images.
And it turned out the best computer vision technique that
had been invented up 'til then was getting like 25% errors
and Alex got 15% errors.
And since then it's gone down to about 3% errors.
It's gone much better since then.
But it was a huge jump and people in computer vision were
extremely surprised and most of them behaved in a very
admirable way, which is they said,
"Hey, we never thought this would work,
but hey it works so we're gonna do that
instead of what we were doing."
That's what scientists don't usually do.
Scientists usually just grow old complaining
that this new stuff is nonsense.
- [Speaker] And how would you describe
the pace of innovation
that we've seen in AI since that moment?
It's just got faster and faster.
So if you'd asked me in that moment how long 'til
these neural nets can do machine translation,
that's better than the state of the art,
I'd have said maybe 10 years.
'Cause machine translation is the kind of thing that if
you've got a theory that's all about processing
strings of symbols, machine translation
is the ideal problem for you.
'Cause you have a string of symbols in one language and you
have to produce a string of symbols in another language.
And the symbolic people thought well inside
you're just manipulating strings to do that.
The neural net people thought
you have to take this string of symbols,
you have to convert it into
these big pans of neural activity,
and then you have to convert it back into symbols
at the output.
And I was very surprised when it only took a few years
for machine translation to be good.
And then in another year or two Google was using it
and it greatly improved the quality
of machine translation.
Like in languages like Chinese, this is from memory,
but there was a gap between how good
the computer translation was
and how good human translation was.
And it just halved that gap overnight.
I think it was Chinese that did that,
but in a lot of languages it just made a lot better.
And since then it's obviously
it's got considerably better since then.
But by 2015 it was already working pretty well
and that really surprised me that it only took three years.
- [Narrator] You say you were surprised
at the pace of innovation.
What did you think the first time you used
a large language model like Chat-GPT, did we surprise you?
- I am just shocked at how good it is.
So it gives very coherent answers and it can do little bits
of reasoning, not very sophisticated reasoning yet,
although it'll get much better.
So for example, I asked it,
this is GPT 4 now.
I asked it a puzzle given to me by a symbolic AI guy
who thought it wouldn't be able to do it.
I actually made the puzzle much harder
and it could still do it.
And so the puzzle goes like this,
"The rooms in my house are either white or blue or yellow.
Yellow paint fades to white within a year.
In two years time I would like all the rooms to be white,
what should I do?"
And a human being would probably say,
you should paint the blue rooms white.
What GPT4 said was "You should paint
the blue rooms yellow,"
but that works too 'cause the yellow will fade to white.
And I don't see how it could do
that without understanding the problem.
The idea that it's just sort of predicting
the next word and using statistics.
There's a sense in which that's true,
but it's not the sense of statistics
that most people understand.
From the data,
it figures out how to extract the meaning of the sentence
and it uses the meaning of the sentence
to predict the next word.
It really does understand and that's quite shocking.
- [Speaker] So have you been surprised
by the broader reaction, the public reaction to Chat-GPT?
- Well given how well it works,
I guess the public reaction isn't that surprising.
But what's interesting is most people
don't say this doesn't understand.
They say wow, it understood what I said
and gave me a coherent answer.
What can I use it for?
And I think most people are right about that.
And of course it can be used for huge numbers of things.
So I know someone who answers letters of complaint
for the health service,
and he used to spend 25 minutes composing
a letter that addresses the problem and so on.
Now he just types the problem to GPT-4
and it writes the letter,
and then he just looks at the letter and decides
if it's okay and sends it out.
And that takes him five minutes now.
So he is now five time
more efficient and that's gonna happen all over the place.
Like paralegals are gonna be like that.
Programmers are already getting like that.
Programmers can be much more efficient if they get
assistance from things like GPT-4
'cause it knows how to program.
And you might think it just knows how to program
'cause it's seen a whole lot of programs.
So I have a former graduate student who's very smart
and a very good programmer,
and he did a little experiment which is,
he's called Redford Neil.
He took
GPT-4,
and he defined a new programming language
with very unusual syntax.
And having defined this programming language
just in text to GPT-4,
he then gave it a program and said "what would this do?"
And it answered correctly.
So basically he could understand the definition
of a new programming language
and figure out what programs in that language would do.
And again the idea that it's just predicting
the next word doesn't make any sense in that context.
It had to understand what was going on.
- [Speaker] So what do you see
as some of the most promising opportunities for this
type of AI when it comes to benefiting society?
- It's hard to pick one 'cause there's so many,
like there'll be a huge increase in productivity
for any job that involves outputting text.
There's all sorts of issues
about increasing productivity.
In our society,
it's not necessarily a good thing to increase productivity
'cause it might make the rich rich and the poor poorer.
But in a decent society,
just increasing productivity ought to be a good thing.
So there'll be things like that.
It's wonderful for making predictions.
It'll be better at predicting the weather.
It'll, people don't know by how much yet.
But it's already much better at predicting floods.
It can predict earthquakes.
It can design new nano materials.
So for things like solar panels,
you want to be able to design new nano materials
or for superconductivity.
I don't know if it's used for superconductivity yet,
but it may well be.
You'd like that at high temperature.
It's really good at designing drugs that is finding
molecules that'll bind to some particular other molecule.
Deep mind has used it to create alpha fold.
Now that's not a chat-bot, that's just deep learning.
But the basic technology of deep learning has pretty much
solved the problem of how you figure out from the string
of bases in a protein, what shape it will adopt.
And if you know what shape it adopts, you know its function.
The chat-bots are just gonna be used everywhere I think.
- [Speaker] And we've also talked a lot about healthcare.
I mean you talked about drug discovery,
but healthcare is another field that could really benefit.
- Yes.
Both in interpreting medical scans.
Like if you take a CAT scan,
there's a lot of information in the CAT scan
and that isn't being used,
and most doctors don't know what the information is.
This will be able to get much more out of a CAT scan
as well as being able to compete
with doctors at saying what kind of cancer you have
or how big it's grown.
At present, for example,
when a doctor tells you the size of a cancer,
you'll get a number like it's three centimeters
and a month ago it was two centimeters.
Now that's not a very useful number,
if the thing looks like an octopus, right.
A neural net will be able to do much better at understanding
the volume of the cancer and how it's changed.
So it's gonna be tremendous there.
And it already,
it's at the level of humans for lots of kinds of scans
and it's gonna get better.
It's gonna be very good for diagnosing diseases.
So at present there's a large number
of people dying in North America,
'cause the doctors misdiagnosed what they had.
There's a system that Google's producing called Med-PaLM 2,
which has learned to do diagnoses and it's already,
I think it's better than an average doctor now.
I'm not quite sure about this
'cause I'm not at Google anymore and it's very recent.
But it's certainly comparable with doctors
and it's gonna get better fast.
So wouldn't you like to have
a sort of general practitioner family doctor.
You go with some rare disease and you'd love your family
doctor to have already seen hundreds of cases
of that rare disease.
A Med-PaLM 2's gonna be like that.
So it's gonna be just in the end much better at diagnosis.
- [Narrator] It sounds like AI will bring
many important benefits,
but you have expressed concern
about the current pace of innovation.
Why?
- Okay, so for like 50 years I thought that,
well for 49 years.
In order to make digital models better,
we needed to make them work more like the brain.
So I kept looking at things the brain does
and the digital models don't,
like rapidly changing connection strengths
in a temporary way and that can make
the digital models better.
And very recently I realized that because
these digital models have this kind of hive mind where
when one agent learns something,
all the other agents know it,
they might actually already be better
than biological intelligence.
And so I kind of completely flipped my opinion from the idea
it's gonna be a long time before they can do
everything the brain does.
It's gonna be 30 to 50 years before they're better than us,
which is what I thought for until very recently.
A few months ago I suddenly realized
maybe they're already better than us,
they're just smaller and when they get bigger,
then they'll be smarter than us.
And that was quite scary.
It was a sudden change of opinion that instead
of being 30 to 50 years, it was five years to 20 years,
something like that.
And so we needed now to take really seriously right now what
we are gonna do about the issue
these things may become smarter than us.
It's a time of huge uncertainty.
Nobody really knows what's gonna happen.
Maybe things will stall and maybe they won't become smarter
than us, but I don't really believe that.
I think they're gonna be smarter than us,
but maybe when they become smarter than us,
we'll be able to keep them benevolent
and we'll be able to keep them caring much more about people
than they care about themselves, unlike people.
But maybe not.
And so we need to start thinking very hard about those
issues and I'm not an expert on those issues.
I'm just an expert on these learning algorithms.
And I suddenly realized these super intelligences may be
here quite soon and I'm just sounding the alarm so that
people listen to the experts who've been thinking for
a long time about how we might stop them taking control.
I want the politicians to listen to those guys,
rather than say, yeah, yeah,
they're sort of sci-fi guys, that it's never gonna happen.
- [Speaker] Was there like a particular moment
when you had this,
you said it was very recent,
that where you kind of changed your view on it?
- I was developing learning algorithms
for biological systems
that could run in a biological system
which didn't use back propagation.
And I couldn't make them work as well as a back propagation
algorithm that we were running on these digital systems.
And they would work for small networks.
But when I scaled it up,
the digital ones always scaled up much better
than the biological ones.
And suddenly I thought it might not be my fault.
It might not be that my learning algorithm
was just a bad learning algorithm.
It might be that these digital systems just are better.
And that's when I suddenly changed my mind about how long
before we get super intelligence.
And then I talked to various former students of mine
and former colleagues of mine,
and some of them encouraged me to go public with this.
Not because I had any solutions that I wanted to recommend.
It's not like you can say burn less carbon
and everything will be fine.
But because they thought I'm well known in the field
and if I go public by saying super intelligence
might be here quite soon,
the politicians might start to believe that's a possibility
and start listening seriously
to the researchers who've been
thinking a long time about how we prevent
these things from gaining control.
- [Speaker] So from your point of view,
what role can governments play in helping ensure
these AI's are developed in a responsible way?
- So there's all sorts of risks other people
have talked about a lot
and I don't particularly want to talk about,
like they'll take jobs away and increase the gap
between the rich and the poor.
They will make it impossible to know
whether news is fake or real.
They will encourage society to divide into two warring camps
that don't listen to each other
and have completely opposing views.
They will build battle robots
that are designed to kill people.
All of those are well-known risks
that I'm not talking about.
It's not that I don't think they're important,
I think they're probably even more urgent.
But lots of other people are talking about those risks.
The risk I'm talking about is the risk
these things will get smarter than us
and eventually take over.
And for that risk there may be something governments can do
because nobody wants that.
Well if you exclude these super intelligences,
no people want that.
And so all the different governments
ought to be able to agree,
they ought to be able to work together on preventing that
'cause it's in their interests.
And that's happened before.
Even during the Cold War,
the US and Russia could work together on trying to prevent
them being a global nuclear war
'cause it was so bad for everybody.
And for this existential threat,
it should be possible for everybody to work together
to limit it if it's possible to prevent it.
I don't know whether it's possible to prevent it,
but at least we should be able to get international
collaboration on that particular threat,
the existential threat of AI taking over.
One thing I think should be done is wherever this stuff's
being developed, particularly these big chat-bots,
governments should encourage the companies
to put a lot of resources,
as these things are getting more and more intelligent,
to doing experiments to figure out how to keep
them under control.
So they should be sort of looking at how these things might
try and escape and doing empirical work on that
and put a lot of resources into that
'cause that's the only chance we've got.
Before they're super intelligent,
we can maybe do experiments and see what's gonna go wrong.
And I'm strongly of the belief
you need empirical data on this.
You just can't have philosophers and politicians
and legislators making up rules.
You need empirical work looking at these things and seeing
how they go wrong and seeing how you might control them.
And that can only be done by the people developing them.
So since you can't stop the development,
the best you can do is somehow have governments put a lot
of pressure on these companies
to put a lot of resources into investigating empirically
how to keep 'em under control when
they're not quite as smart as us.
- [Speaker] And and what do you see as the role
of these big technology companies
where a lot of this development is happening?
Would they do this without
that kind of government regulation?
- So a lot of the people in the big companies,
all the people I know who are senior in the big companies
are very worried about this and do put work into that.
They're very concerned about it,
but they have an obligation to their shareholders.
And I think it to make big profits,
and making big profits, particularly in the short term,
doesn't align nicely with putting a lot of effort
into making sure it's safe.
So you see this in all industries.
In the railway industry in the states,
having safety devices that tell you
when a wheel's locked cost money,
and the big rail companies just rather
have accidents than do that.
Google, which is a big company I know something about
is not quite like that because it understands
that it's got a tremendous reputational loss
if bad things happen.
And that's why Google didn't release these chat-bots.
It kept them private.
It didn't want them out there in the world
for people to play with.
It wanted to use them to give you better search results
or to complete your Gmail for you,
but not to give them to people to play with.
And it could only be responsible like that until OpenAI
and Microsoft put them out there
and then Google had to compete.
But the big people in the big companies really care a lot
about their reputation and about not having bad effects,
but they could maybe be made to care even more about
the safety issue by government
doing something to insist that
they put a lot of work into that.
And there's other things that could happen,
like it's very hard within a company to have people working
on long-term existential threats because they're paid
by the company and there's a conflict of interest,
which is one of the reasons I left Google.
Not 'cause Google did anything wrong,
'cause I just don't want any conflict of interest.
One thing the big companies could certainly do,
is put more money into funding foundations
that study these things.
And Google for example, put $300 million
into a foundation called Anthropic
that is studying these things.
They could put a lot more money in.
- [Speaker] I'm curious about what advice
you would give or what guidance you would give
to other researchers in the field
or might be just entering the field right now and wanna make
sure that they're advancing the field,
but doing it in a responsible way.
- Well, one piece of advice I'd give
is look at how many people are
working on making these things better and how many people
are working on preventing them from getting out of control.
And you'll see it's like 99 people are working on making
them better and one person's working
on preventing them getting out of control.
So where could you make the most impact probably on working,
preventing them getting out of control.
So that's one piece of advice.
The other piece of advice is my general advice
for young researchers, which is,
look for somewhere where you think everybody's
doing it wrong.
And trust your intuition until you figure out
why your intuition is incorrect.
Trust it and work on alternatives ways
of doing things when you think everybody
else is doing it wrong.
And the fact is,
either you have good intuitions or you don't.
If you've got good intuitions,
you should listen to them and follow your intuition
and work on that until you discover why it's wrong.
If you've got bad intuitions,
it doesn't really matter what you do.
So you might as well follow your intuitions.
- [Narrator] The risks you've described are alarming,
but can't you just throw a switch and shut it down?
Aren't humans ultimately still in control?
- It's very tempting to think we could just turn it off.
Imagine these things are a lot smarter than us and remember
they'll have read everything Machiavelli ever wrote.
They'll have read every example
in the literature of human deception.
They'll be real experts at doing human deception,
'cause they'll learn that from us,
and they'll be much better than us.
They'll be like, you manipulating a toddler.
You say to your toddler, "Do you want peas or cauliflower?"
And your toddler doesn't realize,
actually he doesn't have to have either.
He just thinks which he likes,
which he dislikes the most
and says he'll have the other one.
So if they can manipulate people,
they can manipulate people into pressing buttons
and pulling levers.
So we have a nice example of Donald Trump.
Donald Trump can manipulate people and so he could invade
a building in Washington without ever going there himself.
And you didn't have to prevent Donald Trump
from doing anything physical.
You'd had to prevent him from talking to prevent that.
And these are chat-bots.
So the idea that just with talk,
they can't do any real damage 'cause it requires people
to do the damage.
Well, as soon as you can manipulate people,
then you can get whatever you like done.
- [Narrator] You've spent your career trying
to understand how the human brain works
and played a critical role in AI development.
What's next for you, Geoffrey Hinton?
- Okay, so I'm 75 and I've reached the point
where I'm not very good
at writing programs anymore,
'cause I keep forgetting the names of the variables
I'm using and things like that.
And I forget to,
I do a copy and paste and forget to modify
the thing I pasted.
And so I've slowed down a lot in programming
and it's very irritating.
It's extremely irritating not to be as good
as you used to be.
And I decided a long time ago
that when I reached that point,
I would become a philosopher.
And so I'm gonna become a philosopher.
(gentle music)
Ver Más Videos Relacionados

Geoffrey Hinton 2023 Arthur Miller Lecture in Science and Ethics
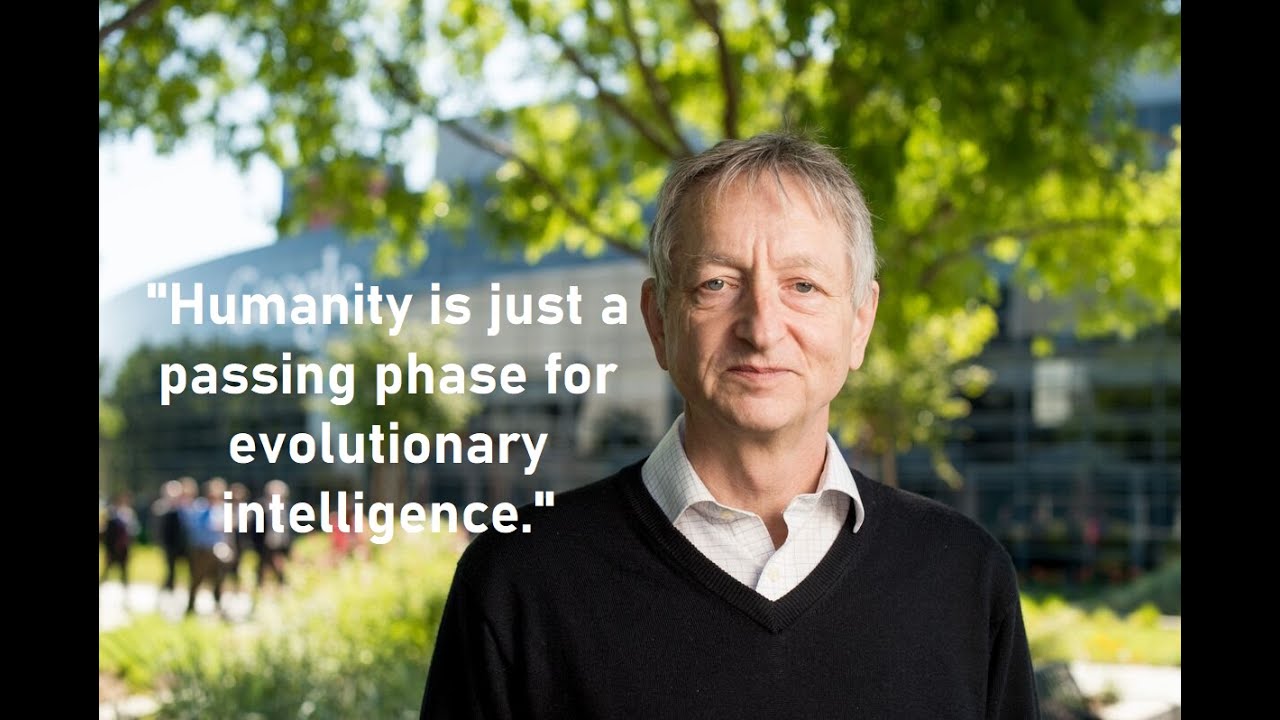
Possible End of Humanity from AI? Geoffrey Hinton at MIT Technology Review's EmTech Digital
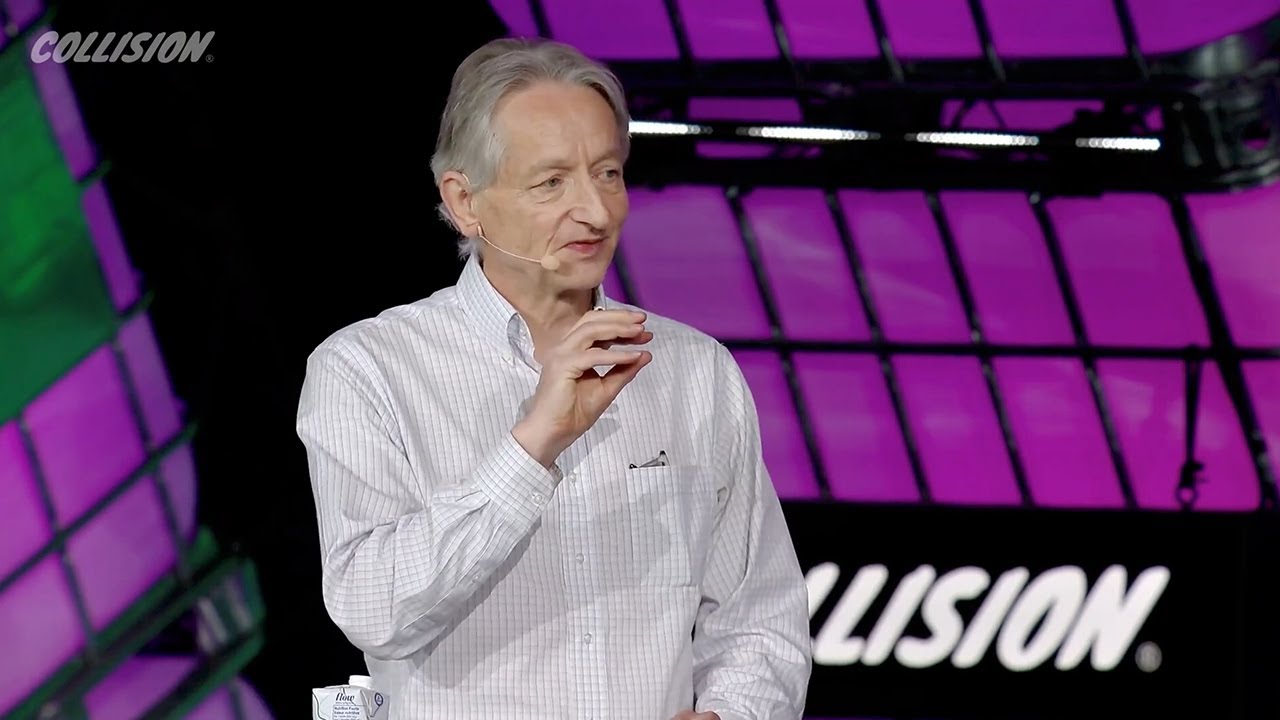
In conversation with the Godfather of AI
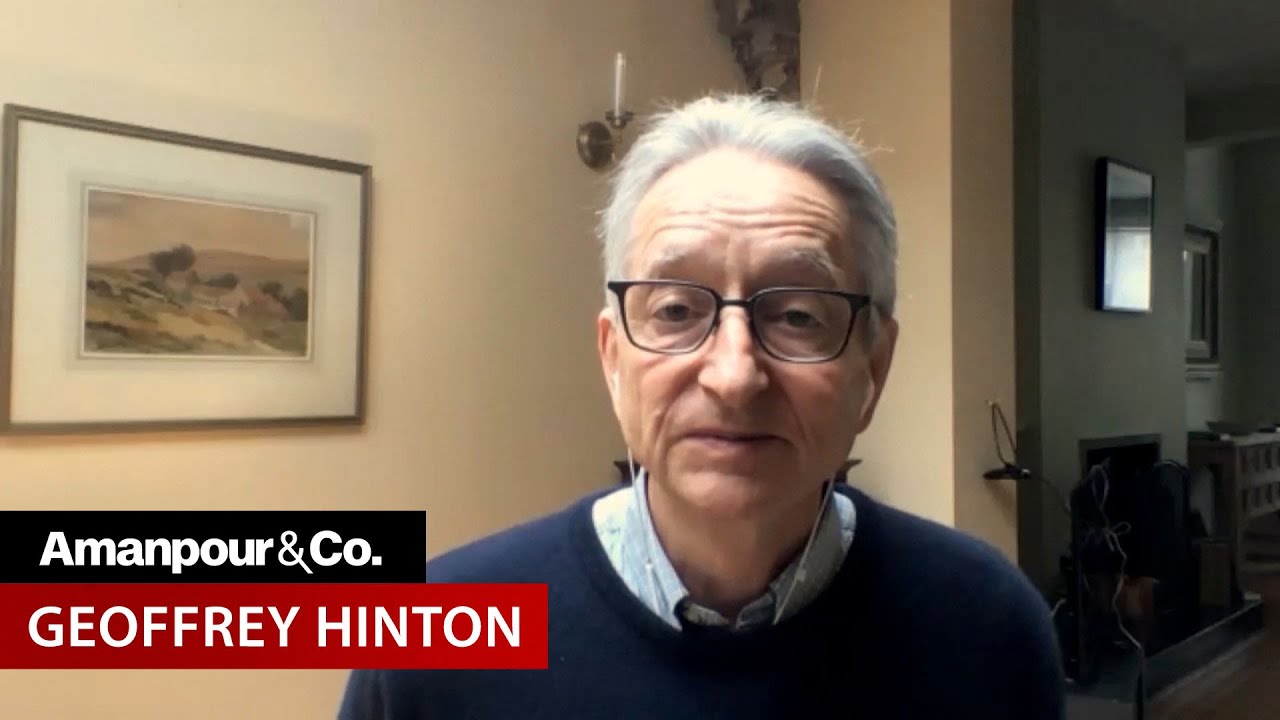
“Godfather of AI” Geoffrey Hinton Warns of the “Existential Threat” of AI | Amanpour and Company
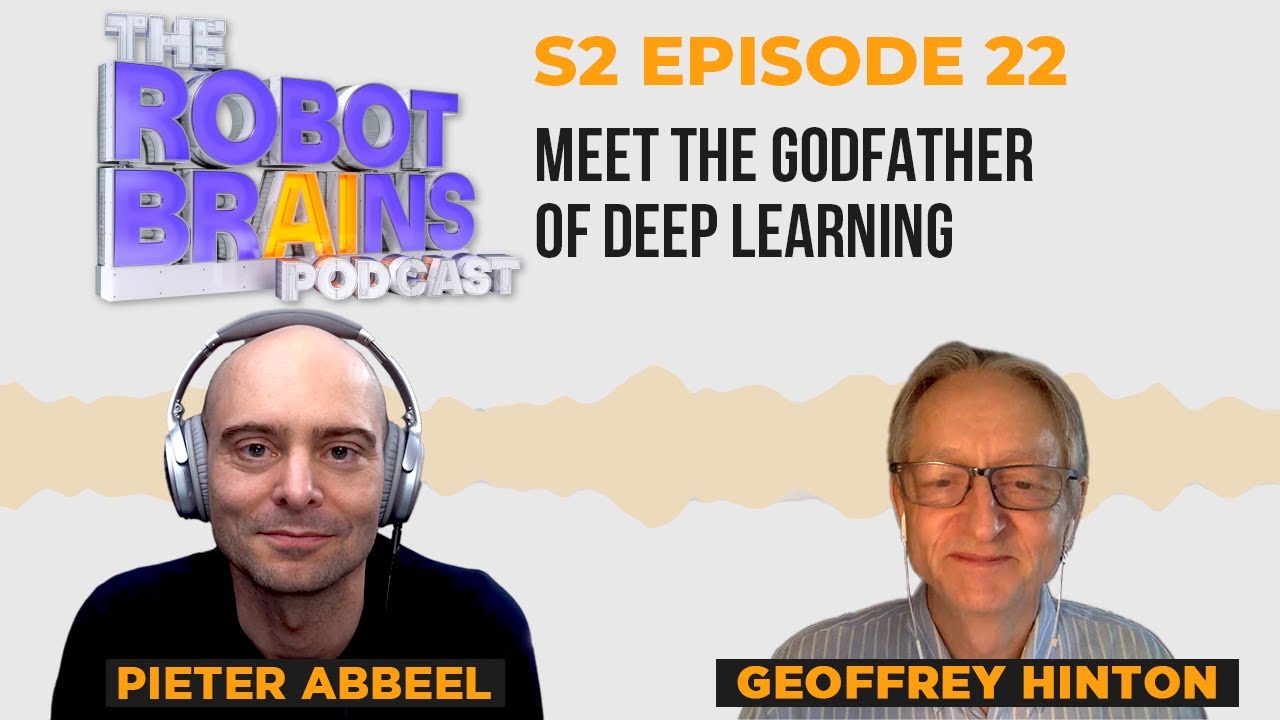
Season 2 Ep 22 Geoff Hinton on revolutionizing artificial intelligence... again
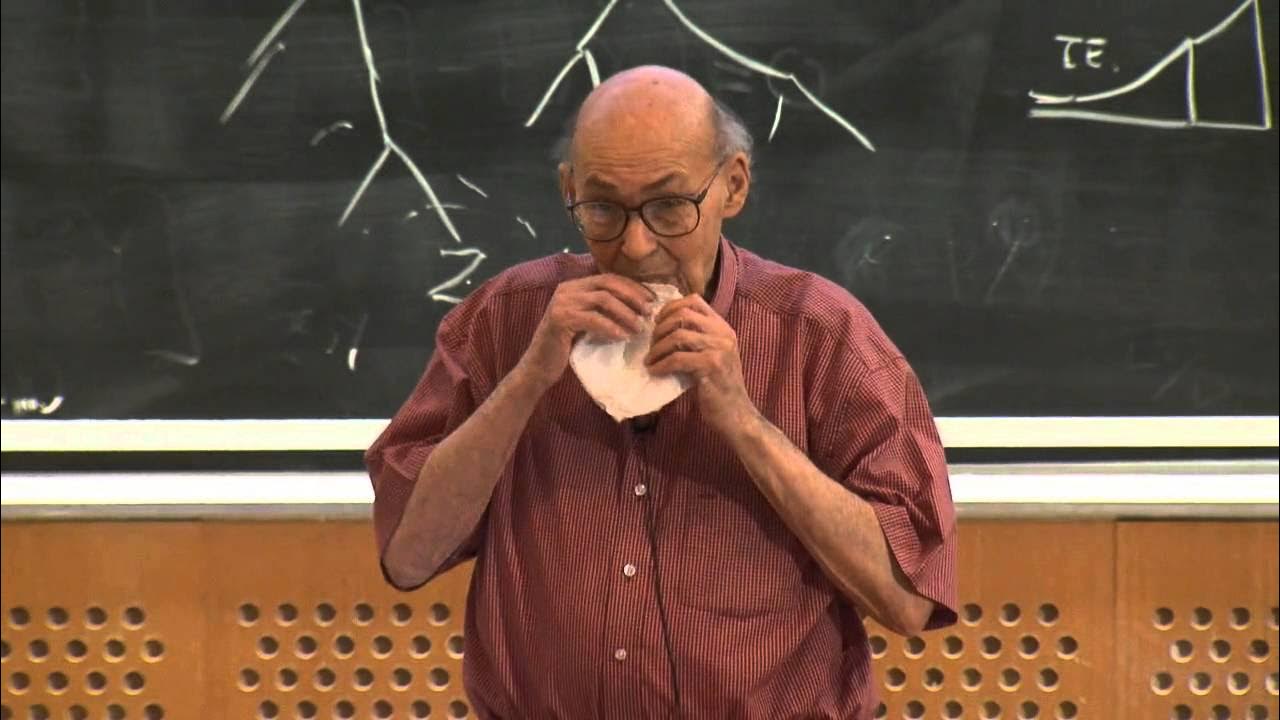
3. Cognitive Architectures
5.0 / 5 (0 votes)