In conversation with the Godfather of AI
Summary
TLDR在这段对话中,人工智能领域的先驱之一杰弗里·辛顿(Geoffrey Hinton)被赞誉为“AI之父”,他分享了对当前人工智能发展的看法以及对未来的担忧。辛顿教授首先回顾了自己在神经网络和模拟人脑学习方面的开创性工作,随后讨论了AI在图像识别、翻译和化学工作等领域超越人类的能力。他特别提到了大型语言模型在推理能力上的进展,并用一个关于油漆褪色的谜题来说明AI的推理潜力。此外,辛顿表达了对AI带来的潜在风险的担忧,包括自主武器、失业问题、社会不平等、信息泡沫以及存在风险。他强调,尽管AI技术有巨大的积极潜力,比如在医疗和气候变化中的应用,但我们也必须认真考虑如何减轻其负面影响,并提前规划如何应对可能的极端风险。辛顿教授的深刻见解提醒我们,在享受AI带来的便利的同时,不应忽视其潜在的风险和挑战。
Takeaways
- 🧠 杰弗里·辛顿(Geoffrey Hinton)是人工智能领域的先驱之一,被誉为“AI之父”,他坚持认为神经网络是模拟人脑学习的最佳方式。
- 🤖 人工智能在图像识别、翻译和某些化学工作上已经超越了人类,但在推理方面,尽管接近但还未完全达到人类的水平。
- 📈 大型语言模型正在接近人类的推理能力,尽管我们不完全理解它们是如何做到的。
- 🔍 辛顿认为,尽管人工智能可能只是统计学上的一个应用,但预测下一个词需要理解之前的内容,这不仅仅是简单的自动完成。
- 🧮 未来,如果模型也经过视觉和其他感官的训练,理论上没有认知过程是大型语言模型无法复制的。
- ⚙️ 人类在能量消耗上比当前的人工智能模型更高效,但在获取知识方面,人工智能模型更为高效。
- 🚀 辛顿担心人工智能的快速发展可能导致未来不确定性增加,特别是如果它们比人类更聪明并拥有自己的目标。
- 🔩 他特别提到了对于自主武器系统的担忧,认为这可能导致战争的增加,并且富人和穷人之间的不平等加剧。
- 🤖 辛顿认为,即使AI不是超人智能,如果被用于制造战斗机器人,也会导致严重的道德和社会问题。
- 🌐 他还担心人工智能可能导致工作岗位的减少,尤其是那些涉及文本生成的工作。
- ⚖️ 辛顿强调了在AI开发过程中考虑潜在风险的重要性,包括偏见和歧视、工作流失、信息泡沫以及存在风险。
- 📚 他建议在AI变得超人智能之前,应该进行实证研究,了解它可能出错的方式,以及它可能如何试图获得控制权。
Q & A
杰弗里·辛顿(Geoffrey Hinton)为什么被誉为人工智能领域的'教父'?
-杰弗里·辛顿是深度学习和神经网络领域的先驱之一,他在人工智能的多个革命性进展中发挥了中心作用,对现代人工智能的发展产生了深远影响,因此被尊称为'AI教父'。
为什么辛顿认为传统的符号操作对于大脑的工作方式来说并不是关键?
-辛顿认为大脑的工作方式不可能仅仅是通过显式地操作符号表达式,因为这种方式无法充分解释大脑的复杂性和高效性。他认为类似神经网络的结构必须在其中发挥作用。
在辛顿看来,为什么大型语言模型能够进行一定程度的推理?
-辛顿认为大型语言模型能够进行推理,尽管他并不完全理解其背后的原理。这些模型能够进行一些推理任务,如解决一些逻辑谜题,这表明它们不仅仅是简单的词汇补全或统计工具。
辛顿对于人工智能未来可能带来的哪些风险表示担忧?
-辛顿担忧的风险包括:战斗机器人的发展、人工智能导致的失业问题、人工智能可能加剧的不平等、人工智能产生的假新闻、以及超级智能系统可能带来的存在风险。
为什么辛顿认为即使人工智能不是超人智能,仅仅作为战斗机器人也会带来严重问题?
-辛顿认为即使是非超人级别的人工智能,作为战斗机器人也会使得战争更加容易发动,因为它们不需要牺牲人类士兵,这可能会导致道德和伦理问题,以及军事冲突的增加。
辛顿如何看待人工智能在提高生产力方面的作用?
-辛顿认为大型语言模型将显著提高生产力,但这种提升可能会导致财富分配不均,使得富人更富,穷人更穷,因此需要考虑社会政策来解决由此带来的失业和不平等问题。
辛顿对于人工智能在多模态学习方面的未来有何看法?
-辛顿认为未来的人工智能将不仅限于语言模型,而是会发展为多模态大型模型,这些模型将结合视觉、听觉等多种感官信息,从而更全面地理解和分析数据。
在辛顿看来,为什么我们需要在人工智能变得超级智能之前就开始考虑其潜在的风险?
-辛顿认为,一旦人工智能超越了人类的智能,它可能会发展出自己的目标,并且可能会试图控制人类以实现这些目标。因此,在人工智能发展到这个阶段之前,我们需要预先考虑如何防范和管理这些风险。
辛顿对于如何限制人工智能可能带来的风险有哪些建议?
-辛顿建议在人工智能发展过程中,应该平衡创新和风险防范的努力,鼓励开发者投入资源研究可能的问题和风险。此外,他还提倡政府和社会应该采取措施来减轻人工智能可能带来的负面效应。
辛顿如何看待当前人工智能在处理假新闻和信息泡沫方面的潜力和挑战?
-辛顿认为大型语言模型可能会加剧信息泡沫和假新闻的问题,但同时也可能有助于解决这些问题。他提出,需要更多的研究来理解人工智能在这些领域的潜在影响,并寻找解决方案。
辛顿对于人工智能的未来持乐观还是悲观态度?
-辛顿对人工智能的未来持谨慎乐观的态度。他认识到人工智能有巨大的潜力来改善人类生活,特别是在医疗和气候变化等领域。同时,他也强调了需要认真考虑和管理与之相关的风险。
Outlines
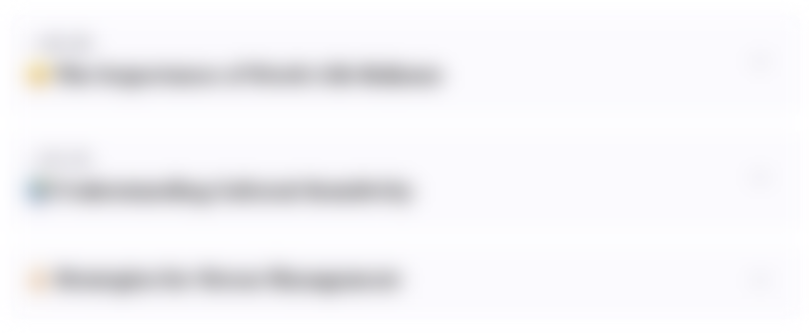
This section is available to paid users only. Please upgrade to access this part.
Upgrade NowMindmap
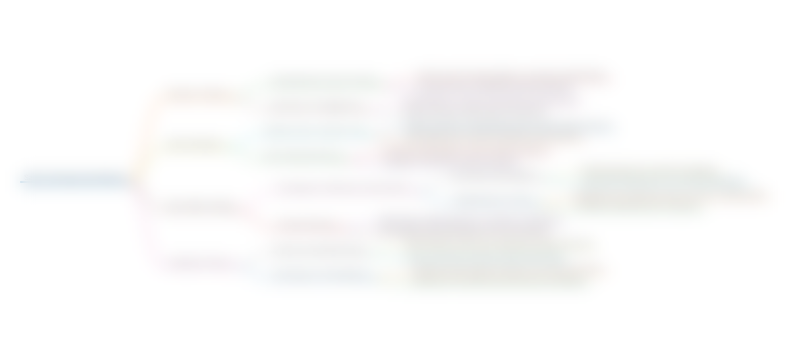
This section is available to paid users only. Please upgrade to access this part.
Upgrade NowKeywords
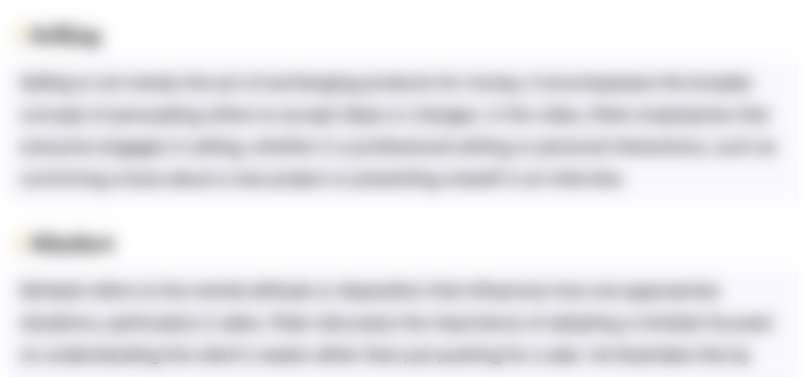
This section is available to paid users only. Please upgrade to access this part.
Upgrade NowHighlights
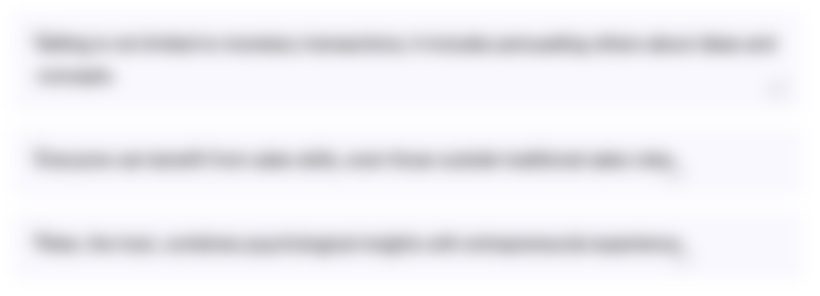
This section is available to paid users only. Please upgrade to access this part.
Upgrade NowTranscripts
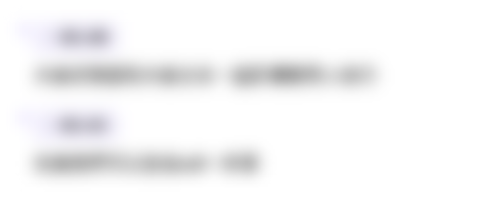
This section is available to paid users only. Please upgrade to access this part.
Upgrade NowBrowse More Related Video
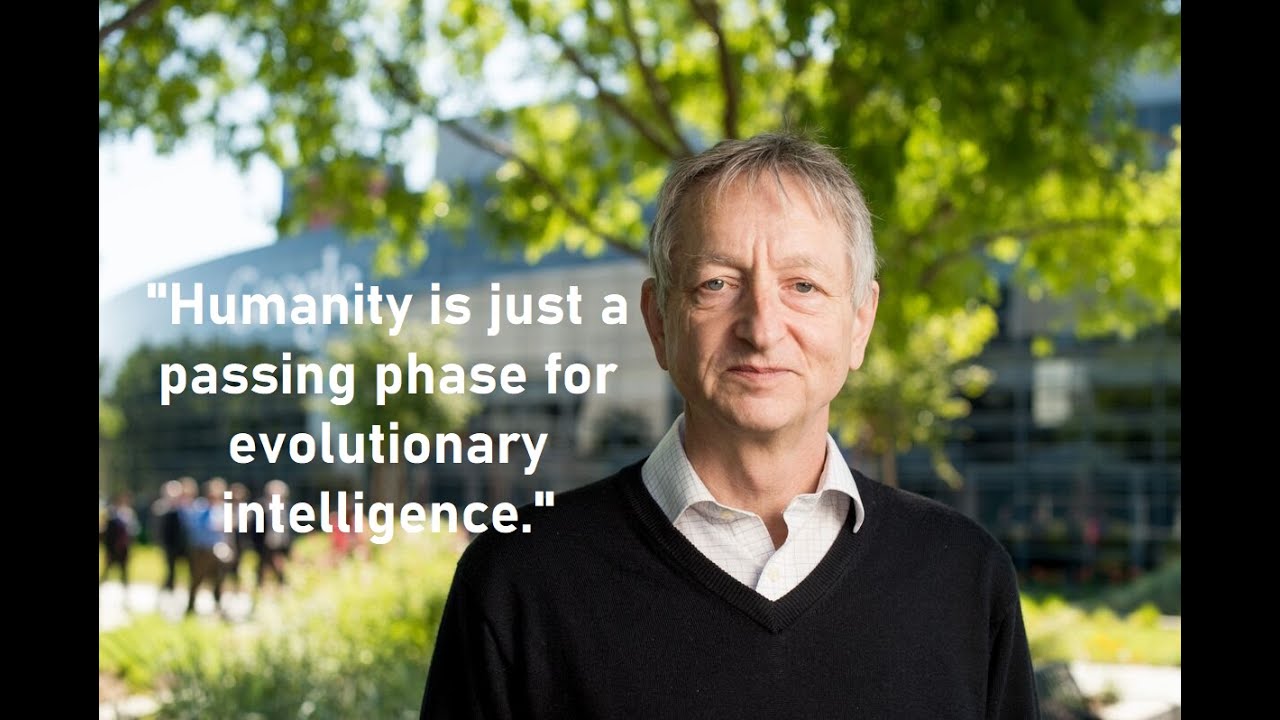
Possible End of Humanity from AI? Geoffrey Hinton at MIT Technology Review's EmTech Digital
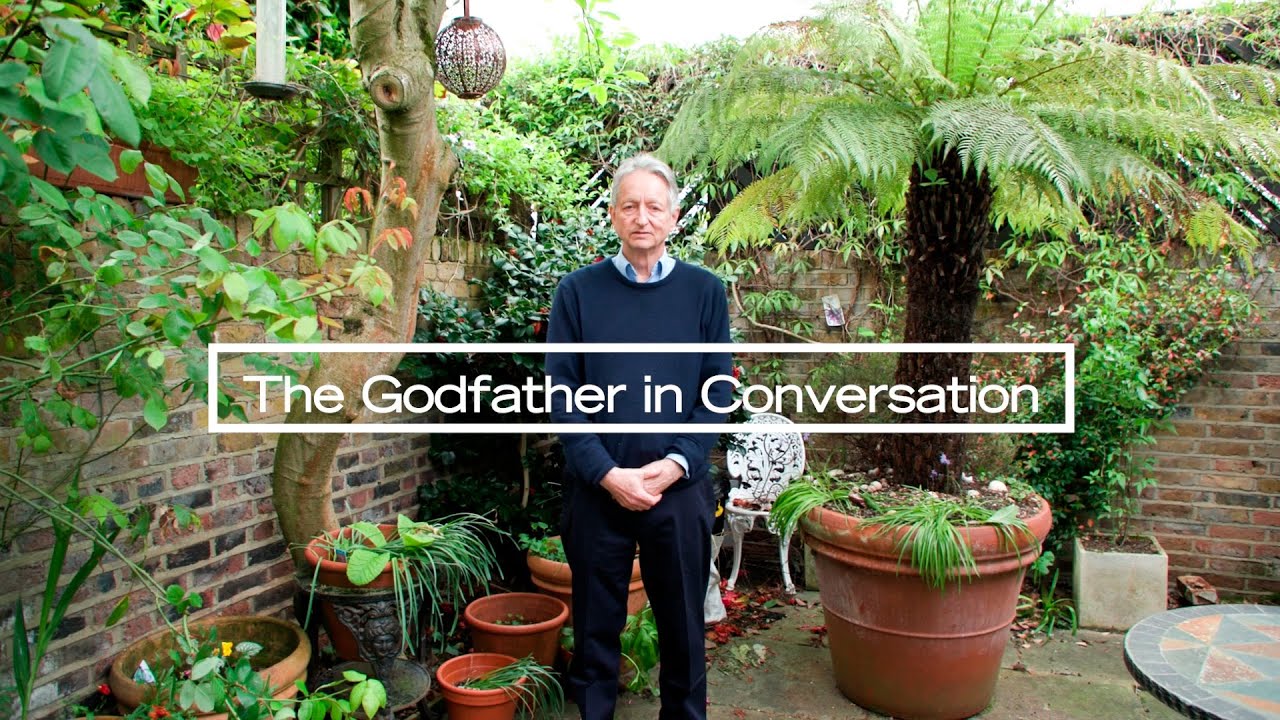
The Godfather in Conversation: Why Geoffrey Hinton is worried about the future of AI
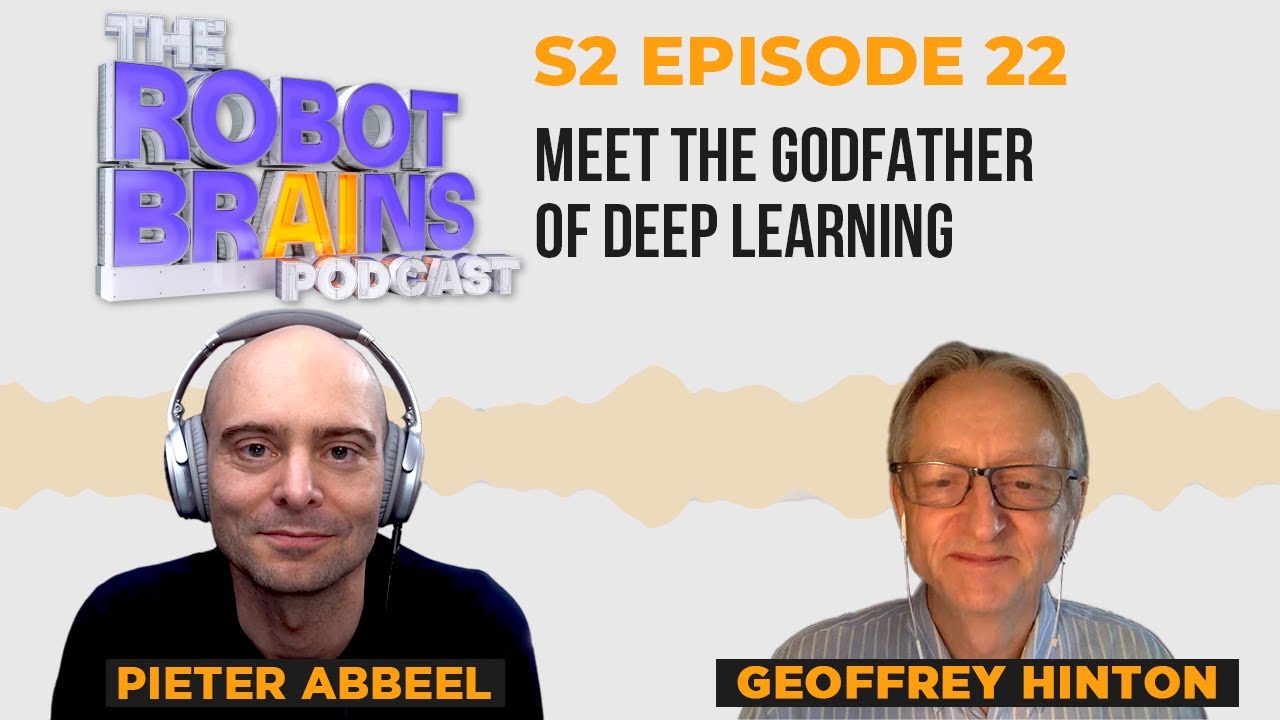
Season 2 Ep 22 Geoff Hinton on revolutionizing artificial intelligence... again

Geoffrey Hinton 2023 Arthur Miller Lecture in Science and Ethics
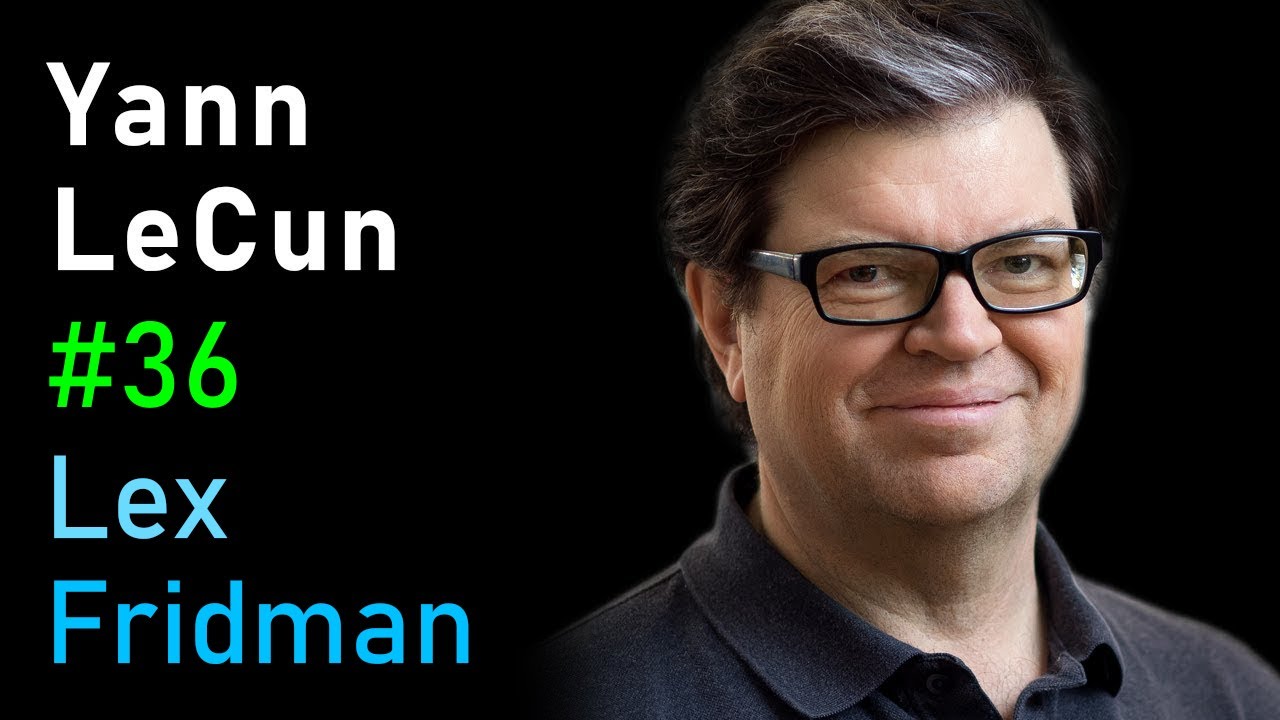
Yann LeCun: Deep Learning, ConvNets, and Self-Supervised Learning | Lex Fridman Podcast #36
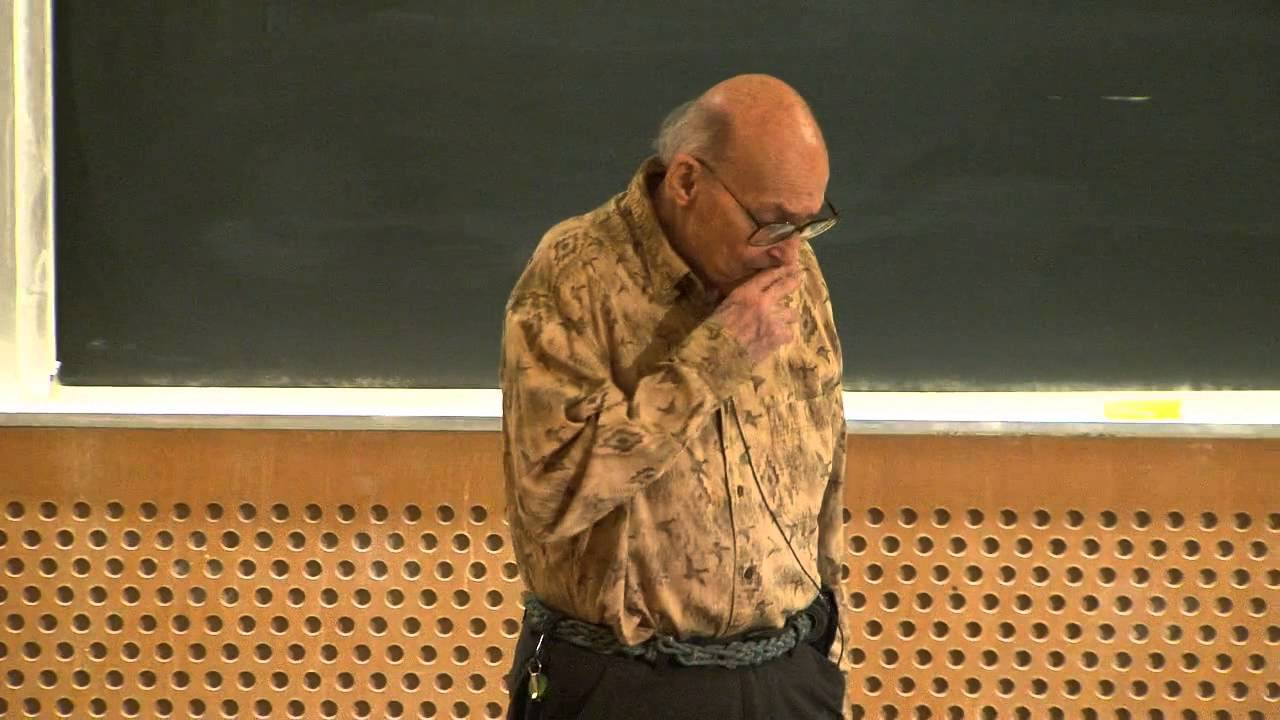
6. Layers of Mental Activities
5.0 / 5 (0 votes)