MINI-LESSON 4: CLT, The Central Limit Theorem, a nontechnical presentation.
Summary
TLDRThis video lesson delves into the Central Limit Theorem (CLT), a fundamental concept in statistics that allows for the application of statistical methods even without a deep understanding of underlying distributions. The presenter explains that regardless of the initial distribution, as the sample size grows, the sum or average of observations converges to a Gaussian distribution. The video provides examples using uniform and Bernoulli distributions to illustrate how quickly these distributions approach a normal distribution with increased samples. It also touches on the slower convergence for fat-tailed distributions, such as the Pareto, concluding with a teaser for the next lesson on correlations.
Takeaways
- 📚 The Central Limit Theorem (CLT) is fundamental to statistics, allowing for the use of statistical tables without needing to understand the underlying distribution's properties.
- 🎲 CLT states that the sum (or average) of a large number of observations from any distribution with a finite variance will approach a Gaussian (normal) distribution as the sample size increases.
- 🔄 There are two versions of CLT: one for distributions with finite variance and another for those without, leading to a 'stable distribution' which is less commonly studied.
- 📉 The Law of Large Numbers is related to CLT, indicating that the average of a sample becomes more stable than the individual components.
- 🔍 CLT simplifies sampling theory by allowing the use of Gaussian properties, even if the original distribution is not Gaussian.
- 📊 The script provides examples of how different initial distributions, such as uniform or Bernoulli, converge to a Gaussian shape when summed over many observations.
- 📈 The convergence to a Gaussian distribution occurs more quickly with distributions that are not 'fat-tailed', as opposed to those that are.
- 📊 The script demonstrates the convergence process through case studies, including a random walk with coin tosses and a Pareto distribution, which has finite variance and thus converges to a Gaussian.
- 📉 Fat-tailed distributions, which are part of the power-law class, converge to a Gaussian very slowly, if at all, and are more analytically complex.
- 👨🏫 The lesson aims to explain CLT in under 10 minutes, emphasizing its importance in statistical methods and its role in allowing even those without deep statistical knowledge to apply statistical techniques.
- 👋 The presenter ends the lesson by thanking the audience for attending and announces the topic for the next session, which will be about correlations.
Q & A
What is the Central Limit Theorem (CLT)?
-The Central Limit Theorem is a statistical theory that states that the distribution of sample means approximates a Gaussian distribution as the sample size gets larger, regardless of the original distribution of the population, provided that the original distribution has a finite variance.
Why is the CLT important for statistics?
-The CLT is crucial because it allows statisticians to make inferences about populations based on sample data, even when the underlying population distribution is unknown or not normally distributed. It simplifies the process of statistical analysis by enabling the use of Gaussian distribution properties.
What does the CLT say about the sum of observations from any distribution?
-According to the CLT, if you have a set of observations (x1, x2, ..., xn) drawn from any distribution with a finite variance, the sum of these observations, as the number of observations (n) becomes large, will tend to form a Gaussian distribution.
What is the difference between the first and second version of the CLT mentioned in the script?
-The first version of the CLT applies to distributions with finite variance, where the sum of observations converges to a Gaussian distribution. The second version, which is not commonly studied, applies to distributions without finite variance and suggests that the sum of observations converges to a different distribution called the stable distribution, often associated with power laws and fat tails.
How does the CLT relate to the Law of Large Numbers?
-The Law of Large Numbers states that as the sample size increases, the sample mean will get closer to the actual population mean. The CLT builds on this concept by stating that not only does the sample mean become more stable, but its distribution also converges to a Gaussian distribution.
What is the significance of the Gaussian distribution in the context of the CLT?
-The Gaussian distribution is significant because it is a well-understood distribution with known properties. The CLT states that the sum or average of observations from any distribution will tend to form a Gaussian distribution as the sample size increases, which allows for the application of these properties in statistical analysis.
Can you provide an example of how the CLT is demonstrated with a uniform distribution?
-In the script, a uniform distribution between 0 and 1 is used as an example. By drawing a large number of observations from this distribution and summing them, the resulting distribution of sums begins to resemble a Gaussian distribution, demonstrating the CLT in action.
What is a random walk and how does it relate to the CLT?
-A random walk is a sequence of random steps, often used to model certain types of stochastic processes. In the context of the CLT, a random walk with binary outcomes (e.g., coin tosses resulting in +1 or -1) can be summed to show how the distribution of these sums approaches a Gaussian distribution as the number of steps increases.
What is the Pareto distribution and how does it relate to the CLT?
-The Pareto distribution is a power-law distribution that is used to describe the distribution of wealth in a society, among other phenomena. It has finite variance and, according to the CLT, the sum of observations from a Pareto distribution will converge to a Gaussian distribution as the number of observations increases.
Why do distributions with fat tails converge to a Gaussian distribution slowly?
-Distributions with fat tails, such as Pareto distributions, have a higher probability of producing extreme values. This characteristic slows down the convergence to a Gaussian distribution because the influence of these extreme values takes longer to be averaged out as the sample size increases.
Outlines
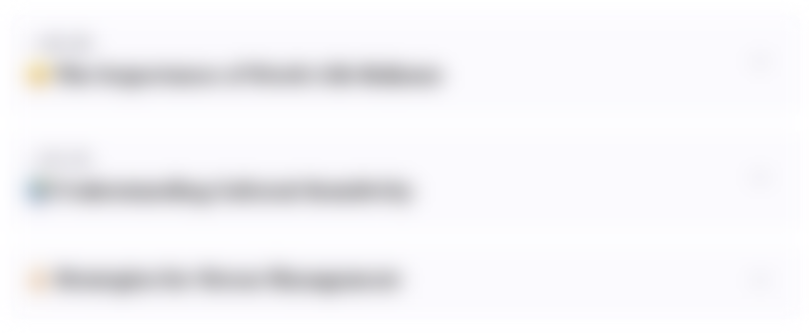
Esta sección está disponible solo para usuarios con suscripción. Por favor, mejora tu plan para acceder a esta parte.
Mejorar ahoraMindmap
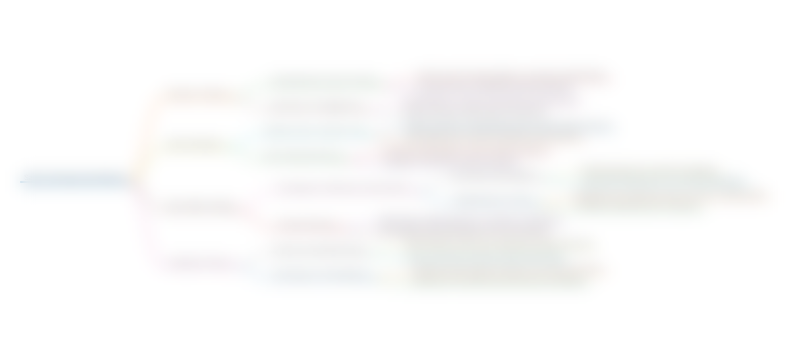
Esta sección está disponible solo para usuarios con suscripción. Por favor, mejora tu plan para acceder a esta parte.
Mejorar ahoraKeywords
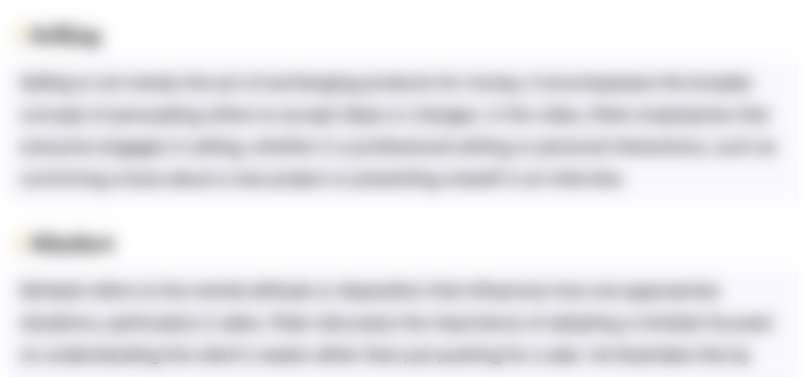
Esta sección está disponible solo para usuarios con suscripción. Por favor, mejora tu plan para acceder a esta parte.
Mejorar ahoraHighlights
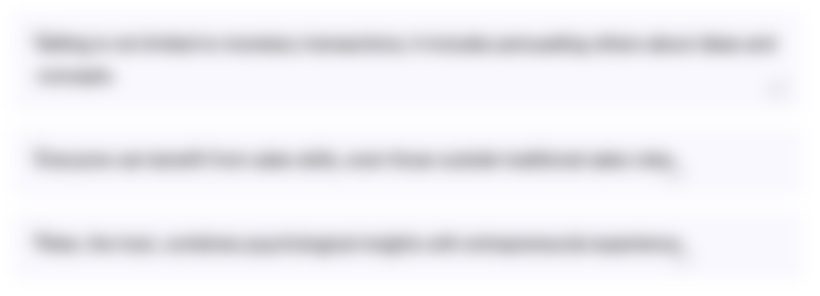
Esta sección está disponible solo para usuarios con suscripción. Por favor, mejora tu plan para acceder a esta parte.
Mejorar ahoraTranscripts
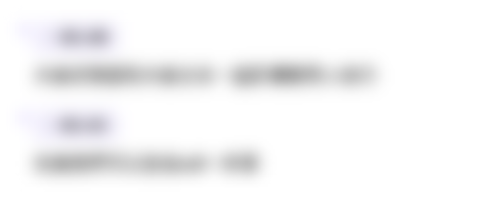
Esta sección está disponible solo para usuarios con suscripción. Por favor, mejora tu plan para acceder a esta parte.
Mejorar ahora5.0 / 5 (0 votes)