Advice for AI beginners | Dario Amodei and Lex Fridman
Summary
TLDRIn this insightful discussion, the speaker offers advice for aspiring AI researchers, emphasizing the importance of hands-on experimentation with AI models. Rather than focusing solely on academic theory, the speaker encourages practical engagement with the technology to gain a deeper understanding. They highlight the potential of under-explored fields such as mechanistic interpretability and long-horizon learning, urging researchers to take risks and explore unconventional ideas. The speaker stresses the importance of breaking away from popular trends and embracing areas that could lead to future breakthroughs, making a lasting impact on the AI field.
Takeaways
- 😀 Start by experimenting with AI models to gain direct experience rather than just reading research papers.
- 😀 Hands-on work with models is crucial to understand their potential, limitations, and new use cases.
- 😀 Focus on underexplored areas in AI, such as mechanistic interpretability, which present opportunities for impactful contributions.
- 😀 Rather than following the crowd, dive into fields with fewer researchers, where your work can stand out.
- 😀 The AI field has many fertile areas that are still largely untapped, offering plenty of low-hanging fruit for new research.
- 😀 Long-horizon learning tasks and multi-agent systems are still in the early stages and offer much room for development.
- 😀 Evaluations of AI models, especially in dynamic real-world environments, are still underdeveloped and need further exploration.
- 😀 Don’t be afraid to pursue ideas that aren't currently popular—many future advancements will emerge from these non-mainstream areas.
- 😀 Breaking through the barrier of fear in choosing unconventional topics can lead to breakthroughs and innovation in AI.
- 😀 The next big advancements in AI will come from areas that are not immediately obvious, but hold long-term potential.
Q & A
What is the primary advice given for people interested in AI?
-The primary advice is to start experimenting with AI models directly. Instead of just reading papers, hands-on experience with models and APIs is crucial to gaining valuable insights and understanding of AI.
Why does the speaker emphasize the importance of hands-on experience with AI models?
-The speaker emphasizes hands-on experience because AI models are still new, and no one fully understands them. Gaining practical experience by working directly with these models provides valuable, experiential knowledge that cannot be obtained through reading alone.
What is meant by 'mechanistic interpretability' in AI?
-Mechanistic interpretability refers to the study of how AI models make decisions and the inner workings of their algorithms. It's a growing area of research that helps explain and understand model behavior, and it’s still relatively underexplored compared to other areas like model architectures.
What is suggested as a promising research area that is less crowded than others?
-The speaker suggests mechanistic interpretability and long-horizon learning as promising areas for research. While model architecture research is highly popular, these areas still have room for exploration and innovation.
What is the significance of long-horizon learning in AI research?
-Long-horizon learning focuses on tasks or goals that span over extended periods, which remains an underdeveloped area. It is crucial for improving AI's ability to handle complex tasks that require long-term planning and decision-making.
What does the speaker mean by 'multi-agent systems' in AI?
-Multi-agent systems involve the interaction of multiple AI agents within an environment. The field is still in its early stages, and there are many opportunities for development in areas like coordination, collaboration, and competition between AI agents.
Why is it important to think about the future of AI, not just its present?
-Thinking about where AI is headed allows researchers to identify emerging fields and long-term challenges that may not be immediately obvious but hold great potential. Focusing on the future helps guide innovative research that can shape the direction of the field.
What is the barrier that prevents many people from exploring new areas in AI?
-The barrier is often the fear of pursuing something that isn’t currently popular or widely accepted. Many researchers stick to familiar topics because they are seen as 'safe,' but breaking through this fear can lead to impactful contributions in less-explored areas.
How can aspiring AI researchers identify exciting opportunities in the field?
-Aspiring researchers can identify exciting opportunities by looking beyond the current trends and focusing on areas that are still underexplored but have the potential for significant impact in the future. These include areas like mechanistic interpretability, multi-agent systems, and long-horizon learning.
What does the speaker suggest about following popular trends in AI research?
-The speaker suggests that while following popular trends can be valuable, it’s often more rewarding to pursue less crowded areas where there is more room for innovation and less competition. Researchers should not be afraid to challenge the status quo and take risks in exploring new directions.
Outlines
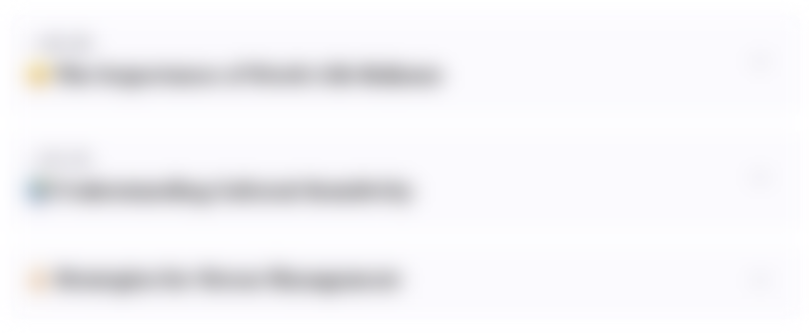
Esta sección está disponible solo para usuarios con suscripción. Por favor, mejora tu plan para acceder a esta parte.
Mejorar ahoraMindmap
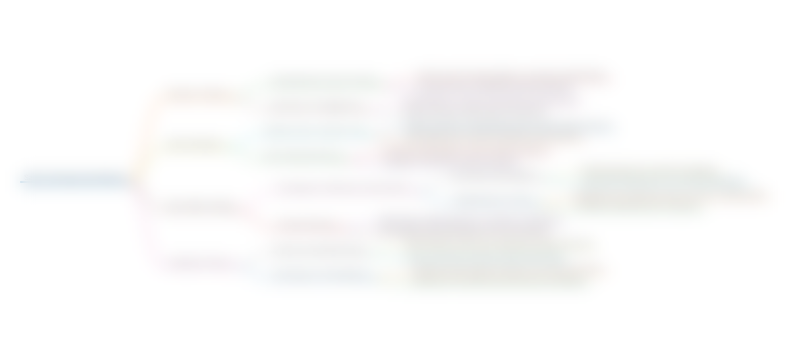
Esta sección está disponible solo para usuarios con suscripción. Por favor, mejora tu plan para acceder a esta parte.
Mejorar ahoraKeywords
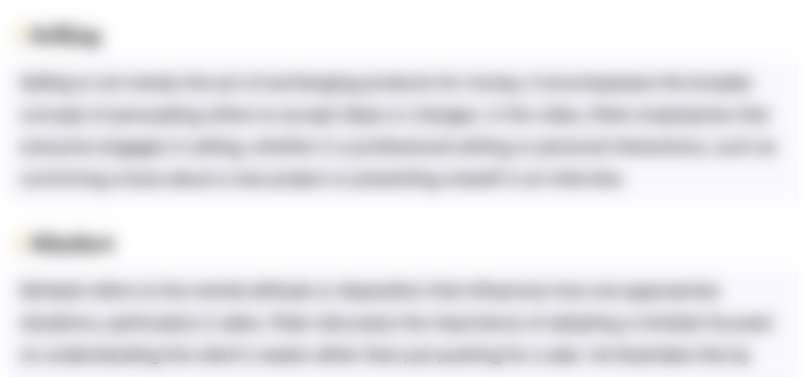
Esta sección está disponible solo para usuarios con suscripción. Por favor, mejora tu plan para acceder a esta parte.
Mejorar ahoraHighlights
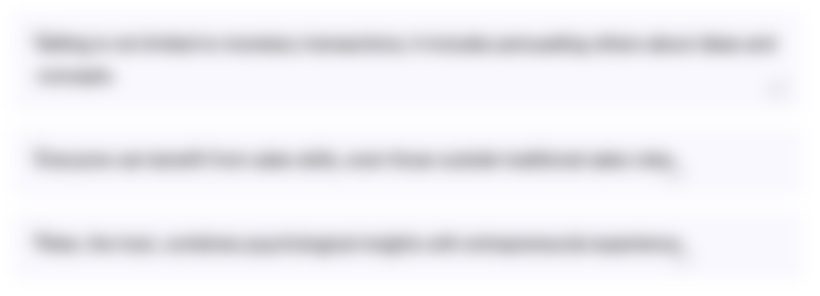
Esta sección está disponible solo para usuarios con suscripción. Por favor, mejora tu plan para acceder a esta parte.
Mejorar ahoraTranscripts
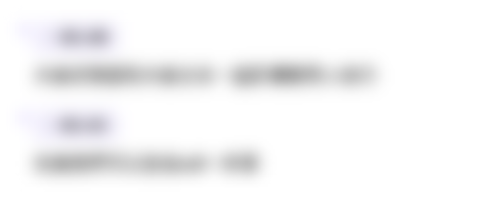
Esta sección está disponible solo para usuarios con suscripción. Por favor, mejora tu plan para acceder a esta parte.
Mejorar ahoraVer Más Videos Relacionados
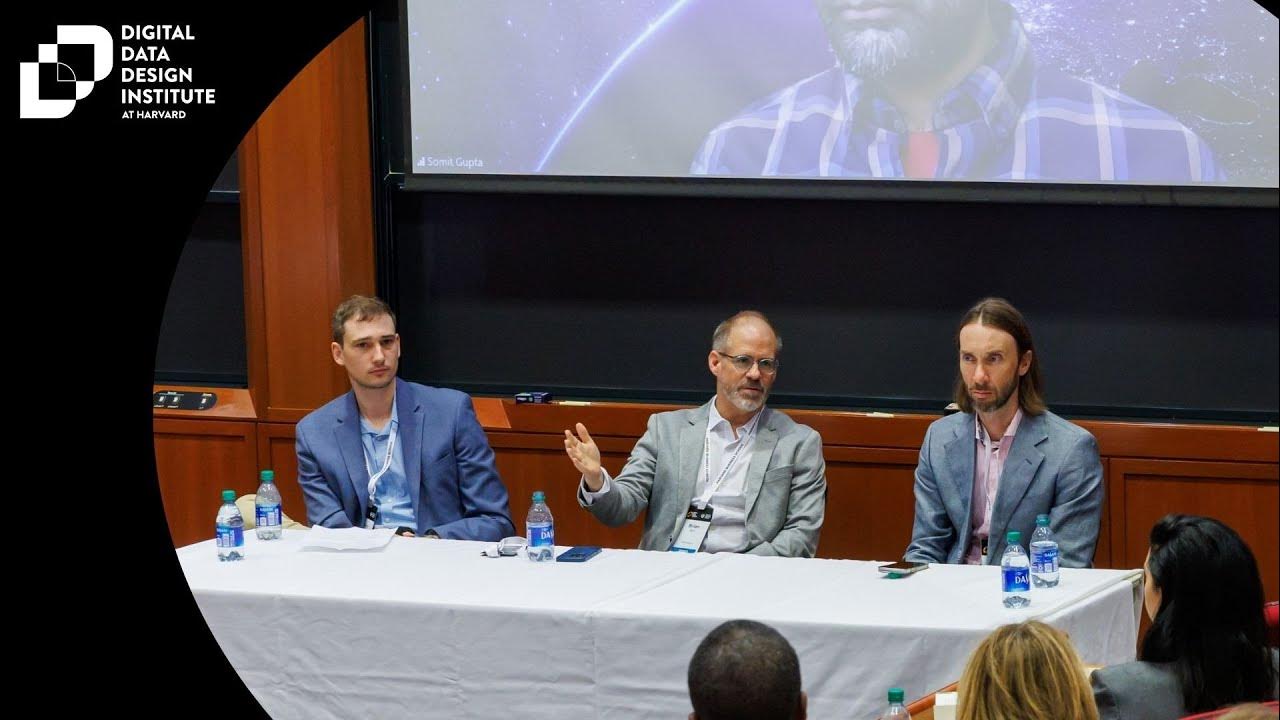
Experimentation with Generative AI
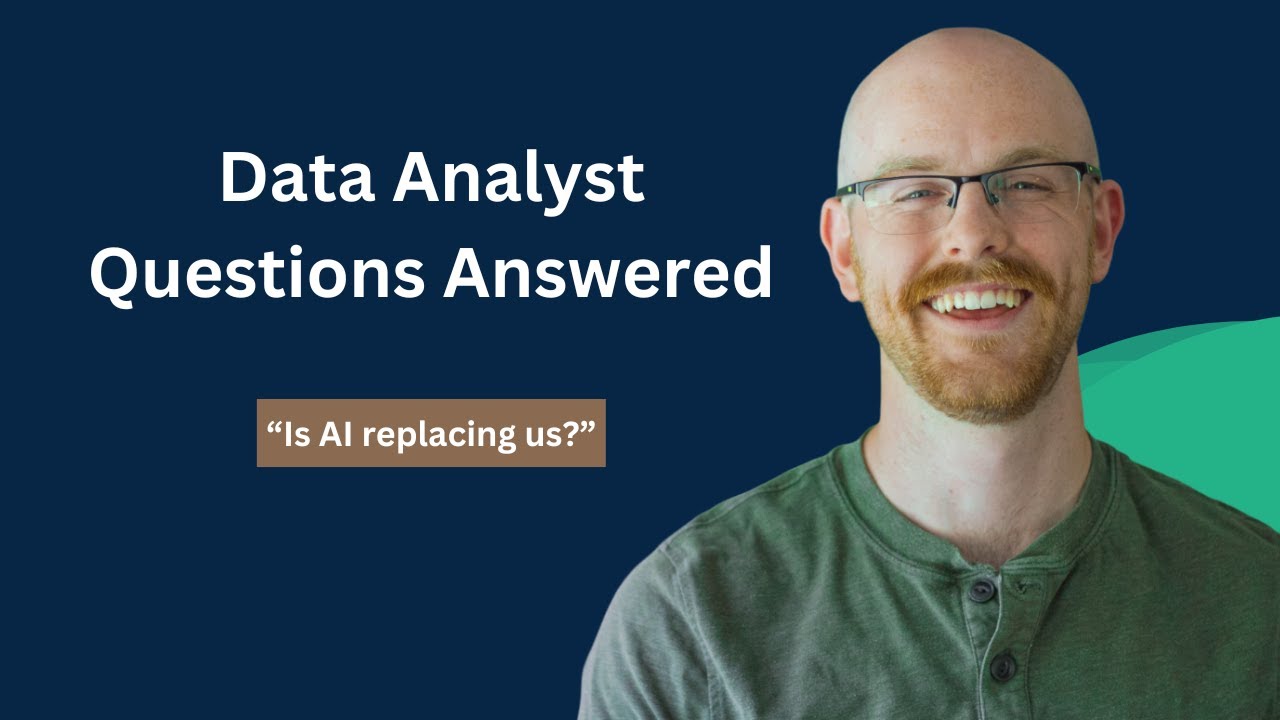
Most Commonly Asked Questions by Aspiring Data Analysts

Advice from a top 1% AI researcher
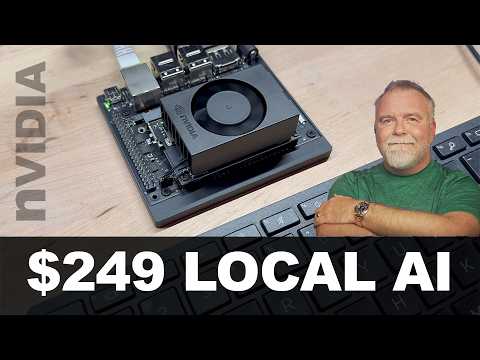
NVIDIA's $249 Secret Weapon for Edge AI - Jetson Orin Nano Super: Driveway Monitor
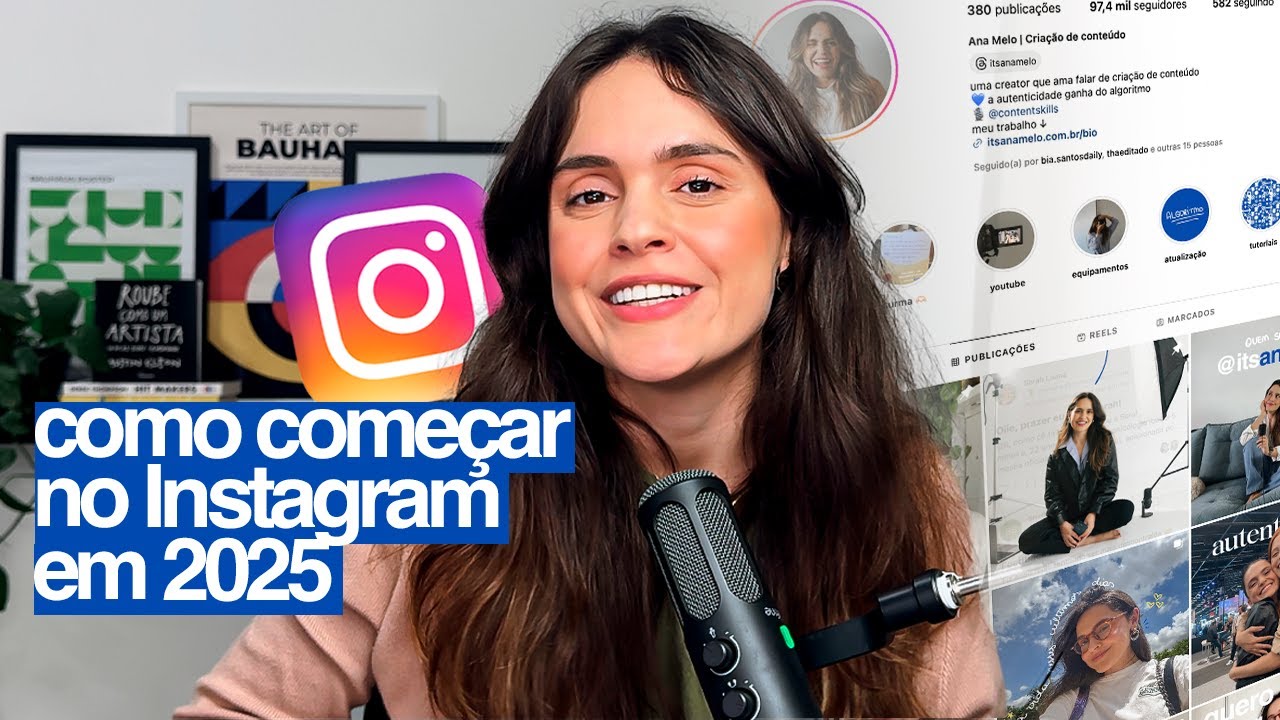
guia completo para COMEÇAR e engajar NO INSTAGRAM em 2025 | Ana Melo
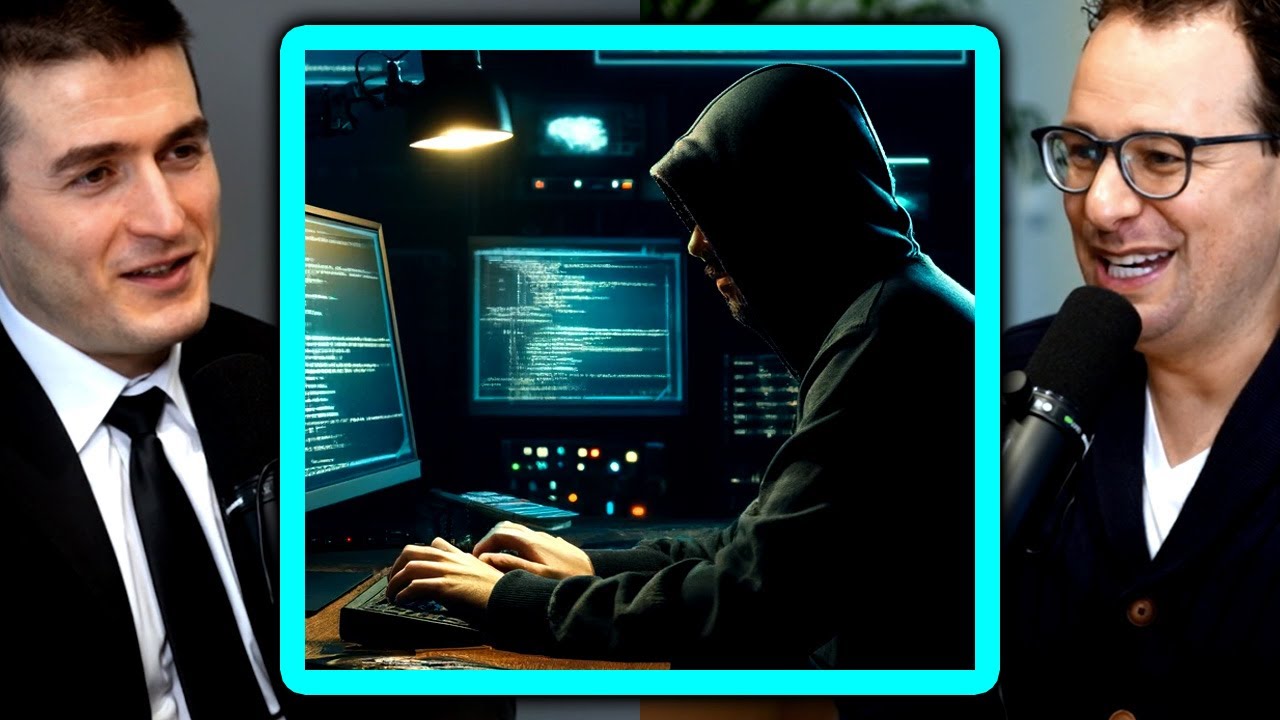
Advice for AI researchers | Dario Amodei and Lex Fridman
5.0 / 5 (0 votes)