Experimentation with Generative AI
Summary
TLDRIn this insightful panel discussion, industry leaders explore the intersection of experimentation and generative AI, emphasizing the importance of operationalizing AI to mitigate risks and optimize capabilities. They delve into the role of experimentation in AI development, the challenges of measuring ROI, and the future of automated experimentation. The conversation highlights the need for a culture that embraces data-driven decision-making and the potential of AI to transform organizational learning and innovation.
Takeaways
- 📚 The integration of experimentation and AI is crucial for effective operationalization of AI within organizations.
- 🔍 Experimentation provides a means for companies to gain control, mitigate risk, and gain insights through measurement and data analysis.
- 🚀 The rise of generative AI has increased the importance of experimentation, as it allows for rapid iteration with reduced risk.
- 💡 Experimentation in AI helps in understanding the impact of intelligent applications, ensuring they deliver value without negative consequences.
- 👥 A culture of experimentation is vital for organizations to innovate and make data-driven decisions effectively.
- 🛠️ Feature flags and experimentation platforms enable companies to test and roll out features safely, capturing data to inform decision-making.
- 🤖 Generative AI can be used to create multiple variants of features or content, which can then be tested through experimentation.
- 📈 Measuring the right Key Performance Indicators (KPIs) is essential to understand the true impact of AI and generative AI applications.
- 🔧 The adoption of AI within organizations requires building trust among employees and ensuring responsible AI practices.
- 🌐 Experimentation can be overwhelming for some organizations; thus, starting with simple measurement and gradually adopting a more experimental mindset can be beneficial.
- 🔬 Netflix and other tech giants leverage experimentation to learn rapidly and make decisions based on aggregated insights from numerous tests.
Q & A
What is the role of experimentation in the development of generative AI technology?
-Experimentation plays a crucial role in the development of generative AI technology by providing control, risk mitigation, and insights that help companies make better decisions and optimize their capabilities. It allows for the testing of new features and ideas in a controlled environment to understand their impact before full-scale implementation.
Why is it important to measure the impact of AI and generative AI applications?
-Measuring the impact of AI and generative AI applications is important to ensure that they are delivering the expected outcomes and value without negative impacts on the business. It helps in understanding whether the applications are improving efficiency, customer service, or other KPIs and if they are introducing any unintended consequences.
How can companies effectively operationalize AI and data practices within their organization?
-Companies can operationalize AI and data practices by integrating them into their core business processes, establishing a data-driven culture, and using tools and platforms that support experimentation and measurement. This involves having the right people, processes, and technologies in place to continuously test, learn, and optimize AI applications.
What is the significance of feature flags in the context of experimentation and AI?
-Feature flags are significant in the context of experimentation and AI as they allow companies to release software features behind a flag, which can be turned on or off easily. This enables controlled testing of new features and facilitates A/B testing, reducing the risk associated with rolling out new features to all users.
How does Netflix approach experimentation in its AI models?
-Netflix approaches experimentation in its AI models by testing different model variants on its members to see which ones resonate best with them. It evaluates the models based on their ability to help customers self-serve in help centers, find content, or achieve other desired outcomes. Experimentation is a key part of Netflix's AI development process.
What are some challenges companies face when trying to show the ROI of their investments in AI and generative AI?
-Some challenges companies face when trying to show the ROI of their investments in AI and generative AI include the difficulty in capturing the intangible benefits of AI, the need to measure both short-term and long-term impacts, and the complexity of attributing specific outcomes to AI interventions. Companies need to define clear KPIs and use robust measurement methods to demonstrate ROI.
How can companies ensure responsible AI practices during experimentation?
-Companies can ensure responsible AI practices during experimentation by setting clear guidelines and metrics for ethical AI use, monitoring for unintended consequences, and incorporating feedback mechanisms. They should also consider the impact on stakeholders and ensure transparency in how AI decisions are made.
What is the role of differential privacy in AI experimentation?
-Differential privacy plays a role in AI experimentation by providing mathematical guarantees on individual privacy, ensuring that individual data cannot be identified from a dataset. This is particularly important when dealing with sensitive data or when there are regulatory requirements around data sharing.
How can generative AI be used to increase the throughput of experiments?
-Generative AI can be used to increase the throughput of experiments by automating the generation of new variants or treatments for testing, reducing the cost and time associated with creating these variants. This allows for a higher volume of experiments to be conducted, enabling more rapid optimization and innovation.
What are some strategies for organizations to adopt a culture of experimentation?
-Strategies for organizations to adopt a culture of experimentation include leadership support and commitment, empowering teams to use data for decision-making, investing in the necessary tools and platforms, and fostering a mindset of continuous learning and improvement. It's also important to start with simple experiments and gradually increase complexity as confidence grows.
How can companies balance the need for experimentation with the risk of overwhelming their employees or users?
-Companies can balance the need for experimentation with the risk of overwhelming their employees or users by starting with small, low-impact experiments, providing clear communication and training, and gradually increasing the scale and complexity of experiments. It's also important to involve users and employees in the process and gather their feedback to ensure alignment with their needs and expectations.
Outlines
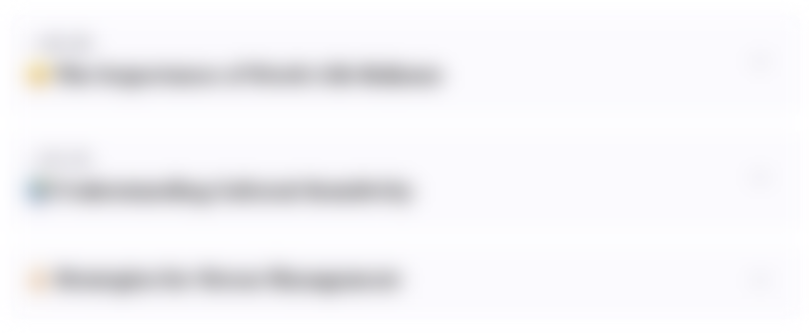
This section is available to paid users only. Please upgrade to access this part.
Upgrade NowMindmap
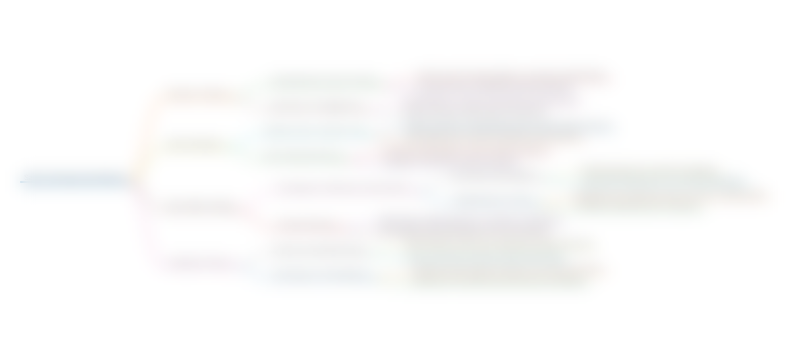
This section is available to paid users only. Please upgrade to access this part.
Upgrade NowKeywords
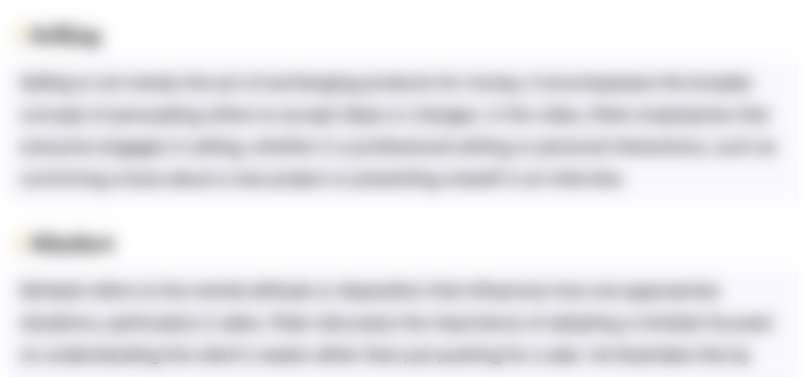
This section is available to paid users only. Please upgrade to access this part.
Upgrade NowHighlights
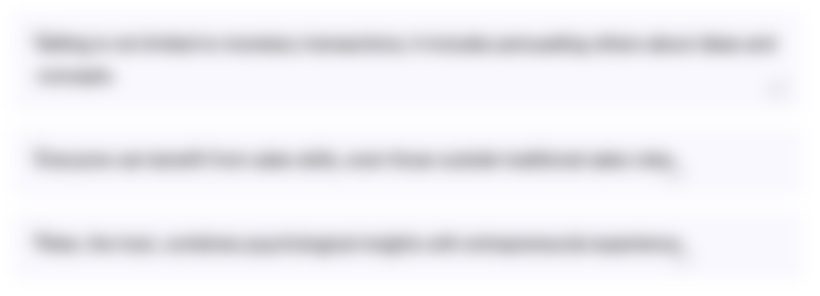
This section is available to paid users only. Please upgrade to access this part.
Upgrade NowTranscripts
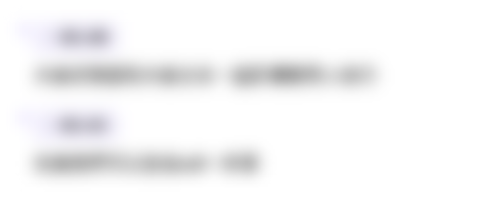
This section is available to paid users only. Please upgrade to access this part.
Upgrade NowBrowse More Related Video

AI AND THE GLOBAL SCALE
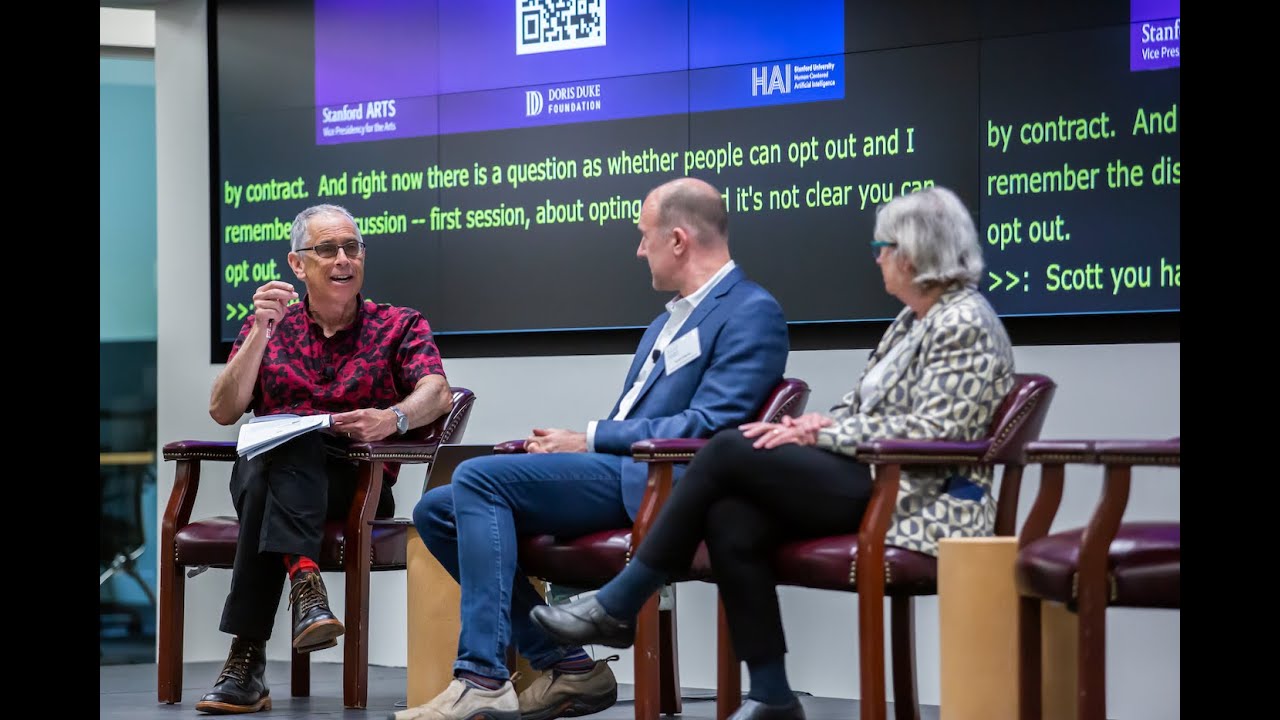
Creativity in the Age of AI: Generative AI Issues in Art Copyright & Open Source

Advice for AI beginners | Dario Amodei and Lex Fridman
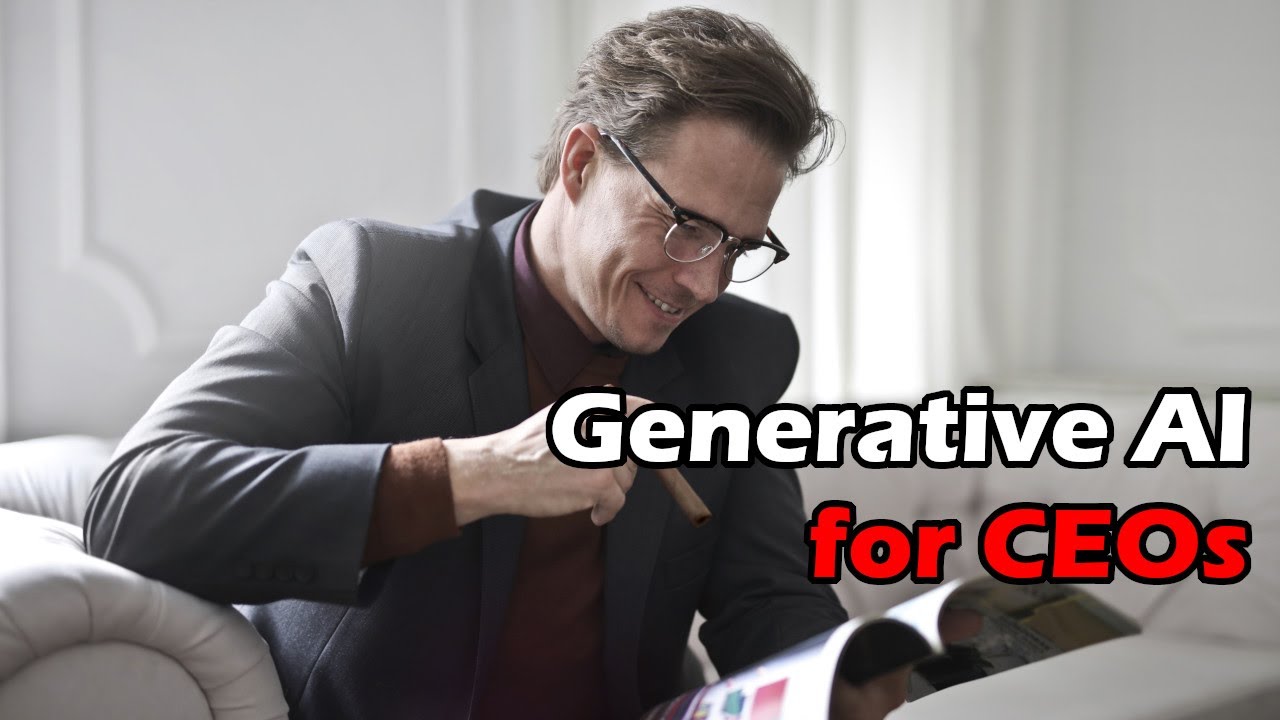
Generative AI for CEOs: How to think about AI today, and how to get the most ROI from it

Common business use cases for generative AI
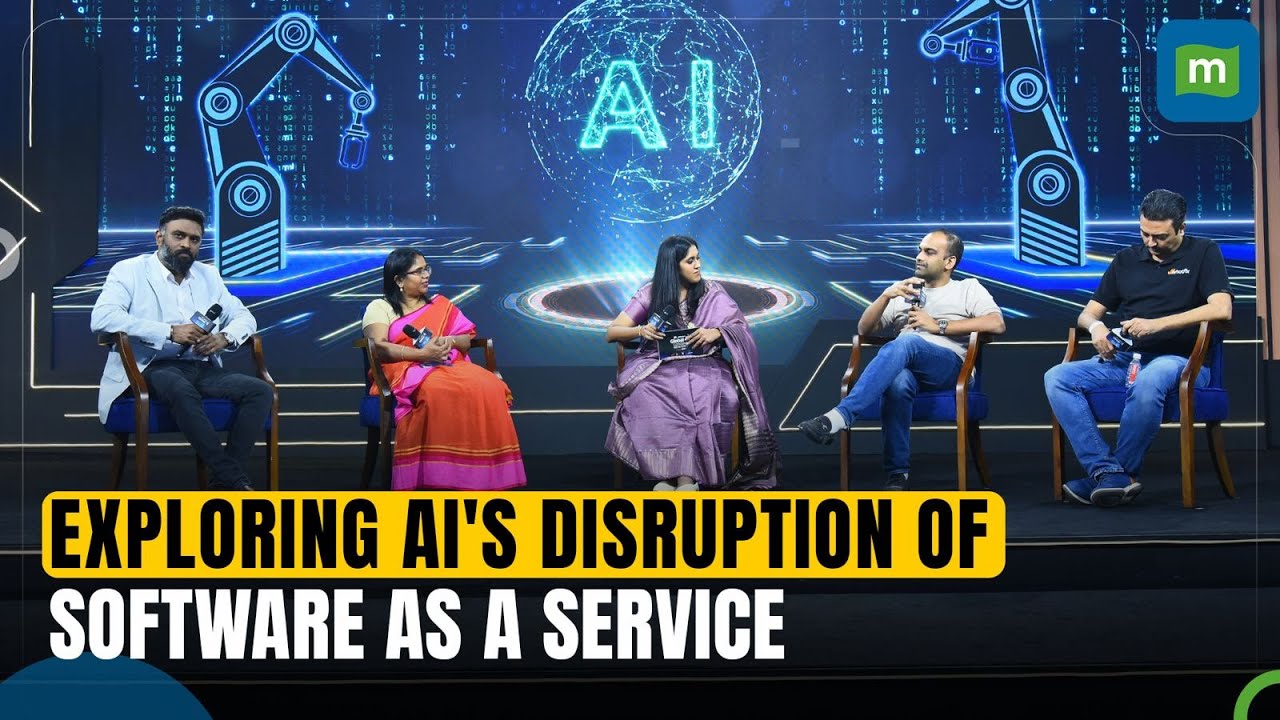
RIP SaaS? Exploring AI's Disruption of Software as a Service | Global AI Conclave
5.0 / 5 (0 votes)