PLS SEM Second Order menggunakan smartPLS Pada Indikator Refleksif
Summary
TLDRIn this video, Tri Widya Pangestika explores second-order reflective indicators using SmartPLS, detailing the two-tier testing process involving latent variables. The tutorial illustrates how to structure a second-order model with first-order indicators, analyze validity through loading factors, and assess reliability via Cronbach's Alpha. Key concepts like convergent and discriminant validity are discussed, emphasizing statistical significance through p-values and t-statistics. By the end, viewers learn to interpret results effectively, including how various factors reflect overall characteristics, enhancing their understanding of advanced structural equation modeling.
Takeaways
- 📊 The second-order model in SmartPLS involves testing latent variables at two levels: first-order indicators and second-order latent variables.
- 🔍 Reflective indicators are characterized by a relationship from latent variables to their indicators, allowing for assessment of construct validity.
- 📈 A model's effectiveness can be visually represented, showing how first-order latent variables serve as indicators for a higher-order latent variable.
- 📝 Each first-order latent variable can have multiple indicators, and the relationship is established through factor loading analysis.
- 🛠 SmartPLS allows for easy importation of data and visualization of model diagrams, aiding in the clarity of complex relationships.
- ✅ Validity is determined through criteria such as loading factor values, with a threshold of above 0.7 indicating strong indicators.
- 🔒 Reliability of a model is assessed through Cronbach's Alpha and composite reliability, both needing to exceed 0.7 for confidence in the results.
- 📏 Discriminant validity can be checked using the Fornell-Larcker criterion, ensuring that the square root of AVE exceeds the correlations between constructs.
- 📊 The R-squared value indicates the explanatory power of the model, with values greater than 0.7 considered strong.
- 📉 Statistical significance is confirmed when the p-value is less than 0.05 or when the t-statistic exceeds 1.96, validating the relationships between variables.
Q & A
What is the focus of the video presented by Tri Widya Pangestika?
-The video focuses on second-order sample modeling using reflective indicators in SmartPLS.
What does 'second-order' refer to in the context of latent variables?
-Second-order refers to the testing conducted on two levels: first on latent variables with their indicators, and second on the relationships between first-order latent variables and the second-order latent variable.
How many first-order latent variables are illustrated in the video?
-There are five first-order latent variables illustrated in the video.
What criteria are used to evaluate the goodness of fit for the model?
-The model's goodness of fit is evaluated based on convergent validity, discriminant validity, and reliability.
What is the significance of a loading factor greater than 0.7?
-A loading factor greater than 0.7 indicates that the indicators are valid and reflect the underlying latent variables well.
What is the role of Cronbach's Alpha in the analysis?
-Cronbach's Alpha assesses the reliability of the measurement; a value above 0.7 indicates acceptable reliability.
How is discriminant validity assessed in the video?
-Discriminant validity is assessed using the Fornell-Larcker criterion, which compares the square root of average variance extracted (AVE) with correlations between latent variables.
What does a p-value less than 0.05 indicate?
-A p-value less than 0.05 indicates that the relationship between the variables is statistically significant.
What is the significance of the R-squared value in the model?
-The R-squared value indicates the proportion of variance in the dependent variable that can be explained by the independent variables; values above 0.7 suggest a strong model.
Which first-order variable was found to have the highest contribution to the second-order latent variable?
-The variable 'skill variety' had the highest contribution to the second-order latent variable, with a value of 0.963.
Outlines
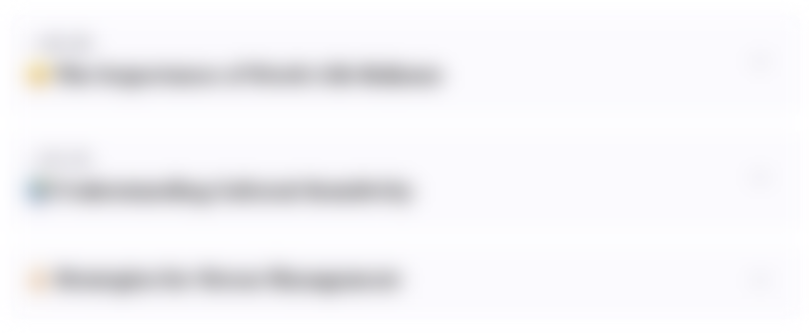
Esta sección está disponible solo para usuarios con suscripción. Por favor, mejora tu plan para acceder a esta parte.
Mejorar ahoraMindmap
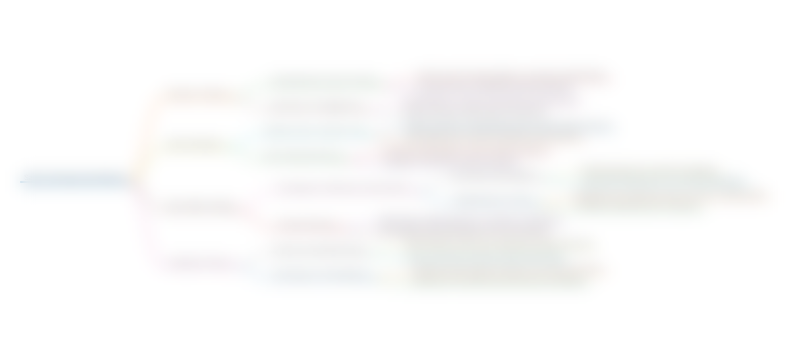
Esta sección está disponible solo para usuarios con suscripción. Por favor, mejora tu plan para acceder a esta parte.
Mejorar ahoraKeywords
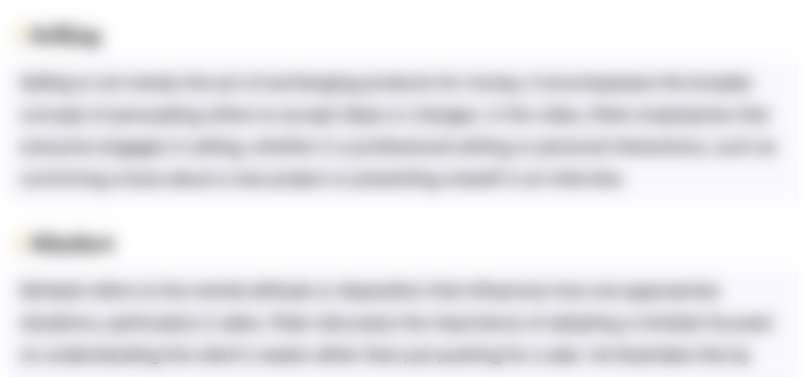
Esta sección está disponible solo para usuarios con suscripción. Por favor, mejora tu plan para acceder a esta parte.
Mejorar ahoraHighlights
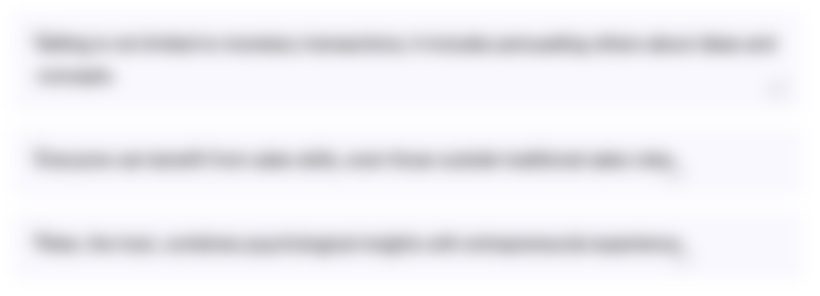
Esta sección está disponible solo para usuarios con suscripción. Por favor, mejora tu plan para acceder a esta parte.
Mejorar ahoraTranscripts
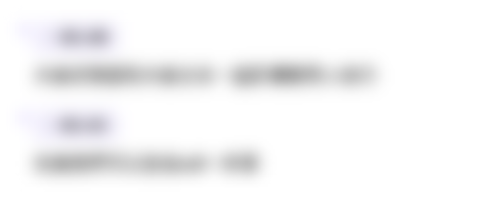
Esta sección está disponible solo para usuarios con suscripción. Por favor, mejora tu plan para acceder a esta parte.
Mejorar ahoraVer Más Videos Relacionados
5.0 / 5 (0 votes)