Decision Tree Solved | Id3 Algorithm (concept and numerical) | Machine Learning (2019)
Summary
TLDRIn this video, viewers learn about decision trees and the ID3 algorithm through a practical example of predicting whether tennis will be played based on various attributes like outlook, temperature, humidity, and wind. The presenter explains key concepts such as entropy and information gain, detailing the step-by-step process of calculating these metrics to build an effective decision tree. By identifying the best attributes for splitting the data, the video illustrates how to structure the tree, ultimately leading to a final model that predicts outcomes based on conditions. Viewers are encouraged to follow along for a hands-on understanding.
Takeaways
- 😀 A decision tree is a supervised learning algorithm that uses nodes to represent features, links to represent decision rules, and leaf nodes to indicate outcomes.
- 📊 The ID3 algorithm focuses on using entropy and information gain to form decision trees effectively.
- ⚖️ Entropy quantifies the uncertainty in a dataset, with higher values indicating mixed classes and lower values indicating purity.
- 🔍 Information gain measures the effectiveness of an attribute in classifying the training data, calculated as the difference in entropy before and after the split.
- 🌳 The first step in creating a decision tree is to calculate the overall entropy of the dataset to understand its initial uncertainty.
- 📈 For each attribute, entropy must be calculated for its various values to determine which attribute contributes most to reducing uncertainty.
- 🎯 The attribute with the highest information gain becomes the root node of the decision tree.
- 📉 After selecting a root node, branches are created based on the possible values of that attribute, leading to further splits as necessary.
- 💡 The process of calculating entropy, information gain, and creating nodes is repeated for each branch until all examples are classified into pure leaf nodes.
- 📚 The final decision tree provides a visual representation of decisions, with paths leading to outcomes based on the attribute values.
Q & A
What is a decision tree?
-A decision tree is a model that uses a tree-like structure to make decisions. Each node represents a feature or attribute, each link represents a decision rule, and each leaf node represents an outcome.
What does the ID3 algorithm do?
-The ID3 algorithm is used to create decision trees by selecting the best attributes to split the data based on the concepts of entropy and information gain.
How is the root node of a decision tree selected?
-The root node is selected based on the attribute that best classifies the training data, determined by calculating the highest information gain.
What are entropy and information gain in the context of decision trees?
-Entropy measures the uncertainty in a dataset, while information gain is the reduction in entropy after the dataset is split on an attribute. It quantifies how well an attribute separates the data.
How is entropy calculated?
-Entropy is calculated using the formula: - (P / (P + N)) * log2(P / (P + N)) - (N / (P + N)) * log2(N / (P + N)), where P is the number of positive examples and N is the number of negative examples.
What steps are involved in using the ID3 algorithm to form a decision tree?
-The steps include calculating the entropy for the entire dataset, calculating the entropy for each attribute, computing information gain for each attribute, and selecting the attribute with the highest gain as the root node. This process is repeated recursively until leaf nodes are reached.
What was the initial dataset used in the video for decision tree formation?
-The initial dataset involved predicting whether tennis would be played based on five attributes: outlook, temperature, humidity, wind, and the class attribute 'play tennis'.
What are the possible values for the 'outlook' attribute in the tennis dataset?
-The possible values for the 'outlook' attribute are sunny, rainy, and overcast.
What does a leaf node in a decision tree represent?
-A leaf node in a decision tree represents an outcome or final decision, indicating that no further splits are needed.
Why is it important to split the dataset recursively?
-Recursive splitting allows the decision tree to refine predictions based on the remaining data, ensuring that the tree can make accurate predictions by taking into account various attributes and their relationships.
Outlines
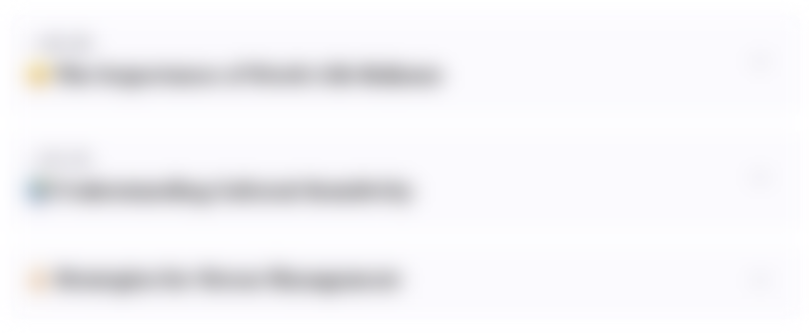
Esta sección está disponible solo para usuarios con suscripción. Por favor, mejora tu plan para acceder a esta parte.
Mejorar ahoraMindmap
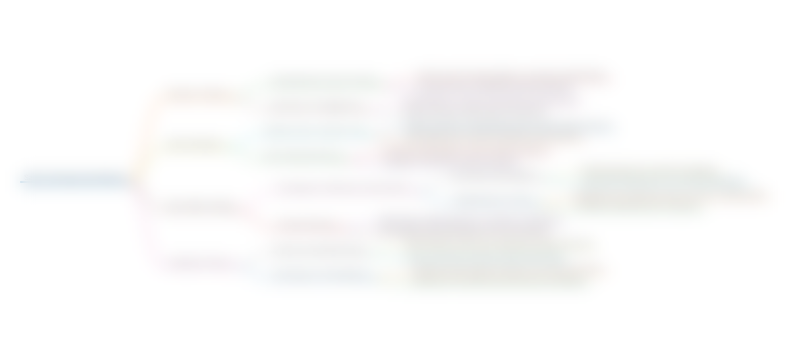
Esta sección está disponible solo para usuarios con suscripción. Por favor, mejora tu plan para acceder a esta parte.
Mejorar ahoraKeywords
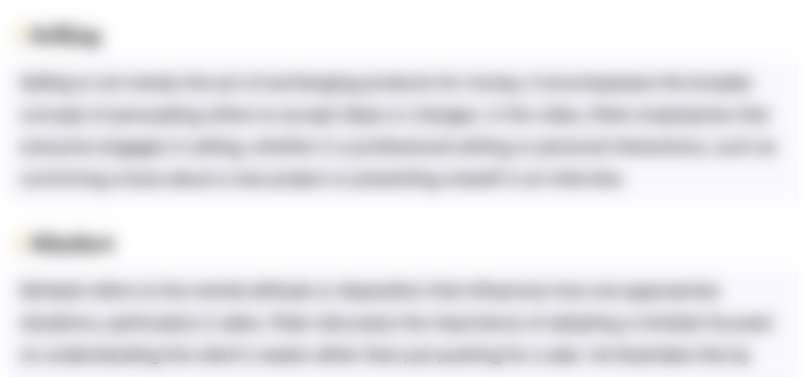
Esta sección está disponible solo para usuarios con suscripción. Por favor, mejora tu plan para acceder a esta parte.
Mejorar ahoraHighlights
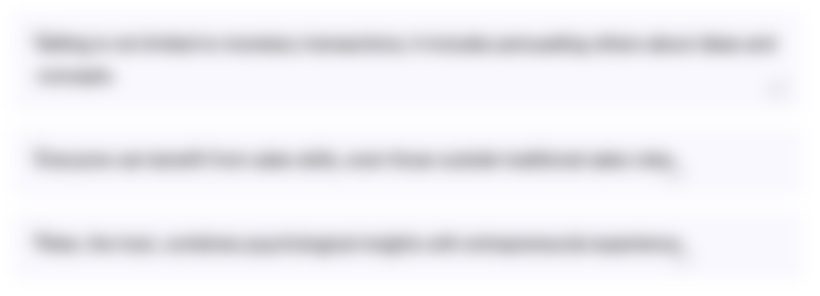
Esta sección está disponible solo para usuarios con suscripción. Por favor, mejora tu plan para acceder a esta parte.
Mejorar ahoraTranscripts
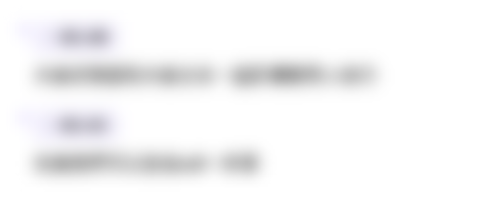
Esta sección está disponible solo para usuarios con suscripción. Por favor, mejora tu plan para acceder a esta parte.
Mejorar ahoraVer Más Videos Relacionados

Machine Learning Tutorial Python - 9 Decision Tree

Learning Decision Tree

Konsep memahami Algoritma C4.5
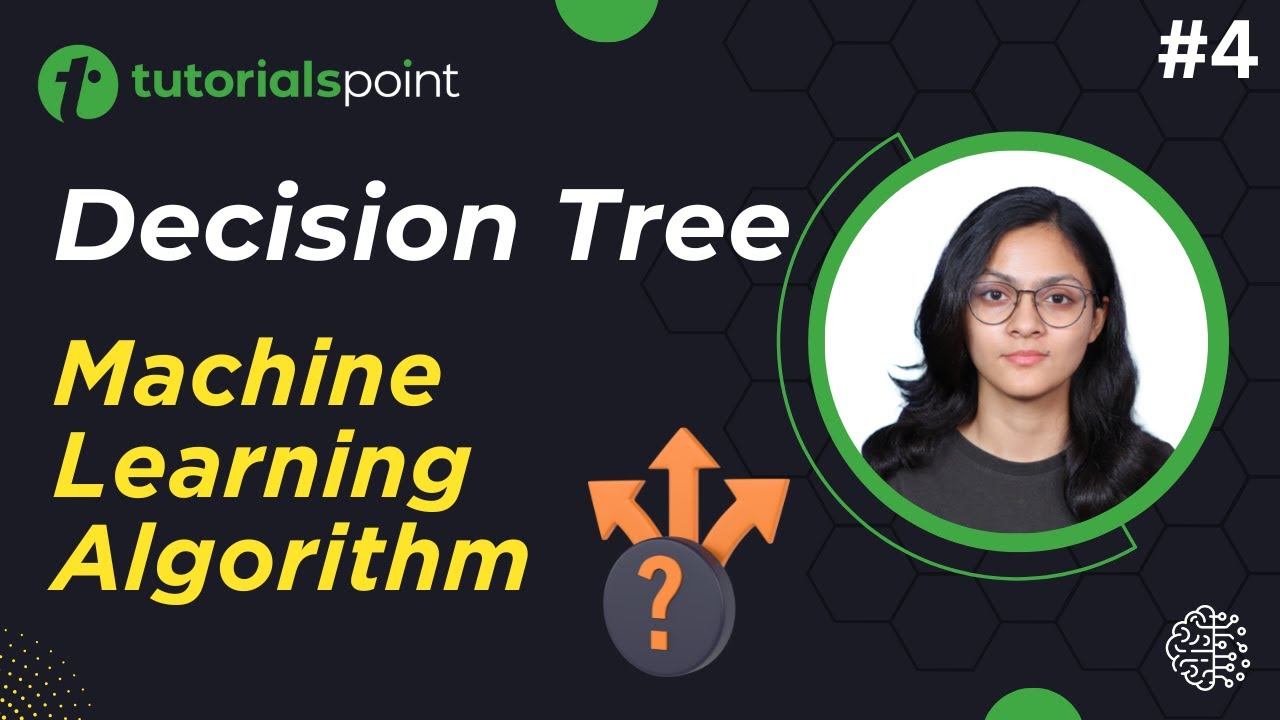
Decision Tree Algorithm | Decision Tree in Machine Learning | Tutorialspoint
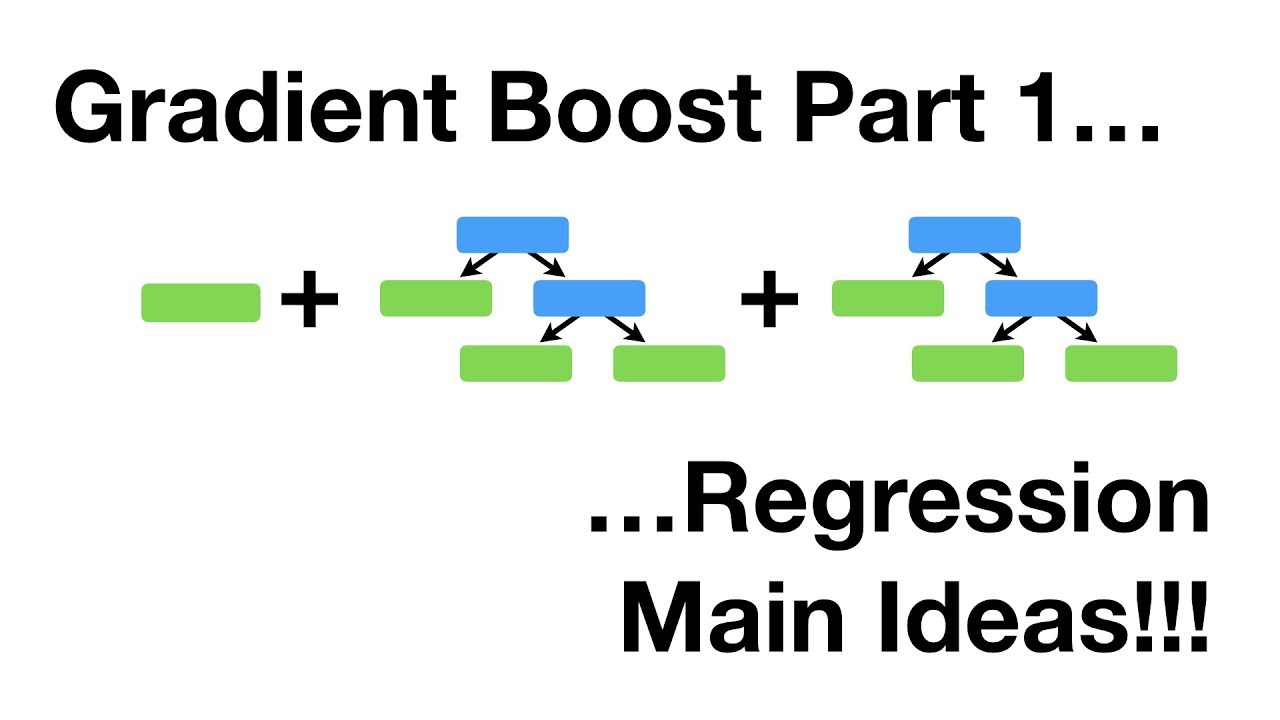
Gradient Boost Part 1 (of 4): Regression Main Ideas
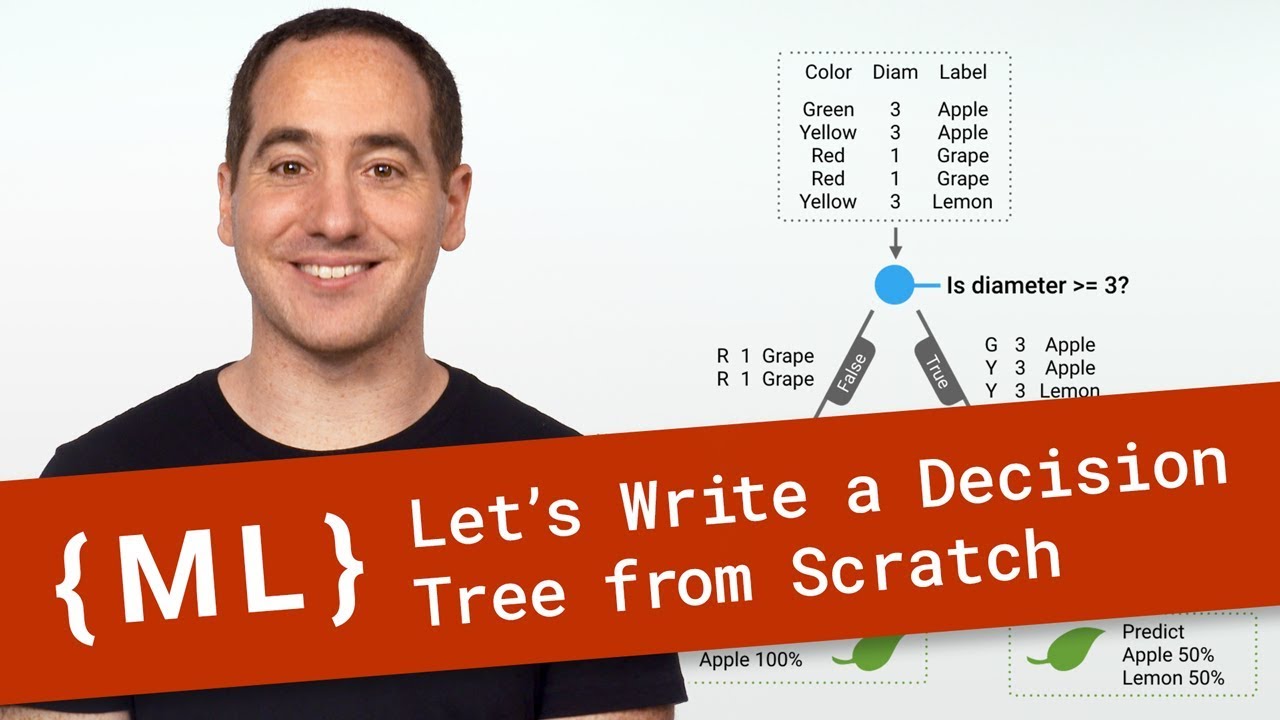
Let’s Write a Decision Tree Classifier from Scratch - Machine Learning Recipes #8
5.0 / 5 (0 votes)