The Chain Rule
Summary
TLDRIn this StatQuest video, Josh Starmer explains the chain rule, a fundamental concept in calculus. Starting with a review of derivatives using basic examples, he builds up to more complex scenarios involving exponential and square root functions. Starmer uses relatable analogies, like predicting shoe size based on weight and height, to demonstrate how the chain rule connects different relationships. He concludes with an example from machine learning, showing how the chain rule helps minimize the squared residuals in a loss function. The clear, step-by-step approach simplifies the concept for viewers.
Takeaways
- 📚 The video explains the chain rule, assuming the viewer is familiar with derivatives.
- 📉 A parabola is used to explain how the derivative gives the slope of the tangent, showing how 'awesomeness' changes with respect to liking StatQuest.
- 🧮 The chain rule is illustrated using a simple example of predicting height from weight, and shoe size from height.
- 🔗 The chain rule connects two relationships: height based on weight and shoe size based on height.
- 📐 The derivative of shoe size with respect to weight is found by multiplying the derivative of height with respect to weight and the derivative of shoe size with respect to height.
- 🚶♂️ A more complex example involving hunger and craving for ice cream is presented, showing how to use the chain rule to find how craving changes over time.
- 🔄 The video emphasizes the application of the chain rule even when equations are not in a simple, separate form, using parentheses to clarify relationships.
- 📊 A practical example of applying the chain rule in machine learning is given, focusing on residual sums of squares to find the best fit line for weight and height data.
- 🧩 The chain rule is repeatedly applied by separating equations into simpler components to compute derivatives efficiently.
- 🎯 The video concludes with finding the intercept that minimizes squared residuals to determine the best fit line, demonstrating how the chain rule helps in optimizing functions.
Q & A
What is the chain rule in calculus?
-The chain rule is a fundamental concept in calculus that allows us to compute the derivative of a composite function by multiplying the derivatives of the inner and outer functions.
Why is the chain rule important in the context of the examples provided?
-The chain rule helps connect changes between multiple variables, as demonstrated in the examples with weight, height, and shoe size, as well as hunger and craving for ice cream. It allows us to understand how changes in one variable affect another through an intermediary variable.
How does the chain rule apply to the weight, height, and shoe size example?
-In the example, the chain rule shows how weight indirectly affects shoe size through height. The derivative of shoe size with respect to weight is calculated as the product of the derivative of shoe size with respect to height and the derivative of height with respect to weight.
What is the relationship between the slope and the derivative in the provided examples?
-The slope of a line represents the rate of change between two variables, and this is the same as the derivative in the examples. For instance, the slope of the green line between weight and height is 2, so the derivative of height with respect to weight is also 2.
How does the chain rule simplify complex derivative calculations?
-The chain rule breaks down complex composite functions into simpler parts by differentiating the outer function first and then multiplying it by the derivative of the inner function. This is useful when dealing with nested functions, such as in the ice cream craving and hunger example.
Why is the chain rule especially useful in the example with hunger and craving for ice cream?
-The chain rule simplifies the process of calculating how ice cream cravings change with respect to time since the last snack by considering how hunger changes with time and how cravings change with hunger. Without the chain rule, the calculation would be more complex and less intuitive.
How does the chain rule help in machine learning applications, like calculating the residual sum of squares?
-In machine learning, the chain rule helps compute the derivative of the loss function, such as the residual sum of squares, by breaking down the derivative into simpler parts, making it easier to find the optimal parameters (e.g., the intercept) that minimize the loss.
What is the significance of using parentheses in the chain rule examples?
-Parentheses help isolate the inner function or 'stuff inside' in a composite function, making it easier to apply the chain rule by clearly identifying the inner and outer functions for differentiation.
What role does the power rule play in the chain rule examples?
-The power rule is used in combination with the chain rule to differentiate functions that involve powers, such as the square of a variable. It simplifies finding the derivative of a function raised to a power, which is a common occurrence in the examples.
How does the video explain the process of minimizing the squared residual in machine learning?
-The video explains that minimizing the squared residual involves finding the derivative of the squared residual with respect to the intercept and setting it to zero. The chain rule is used to calculate the derivative by considering the relationship between the residual and the intercept.
Outlines
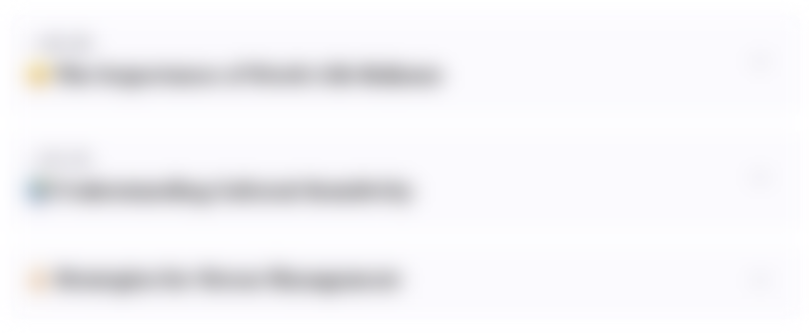
Esta sección está disponible solo para usuarios con suscripción. Por favor, mejora tu plan para acceder a esta parte.
Mejorar ahoraMindmap
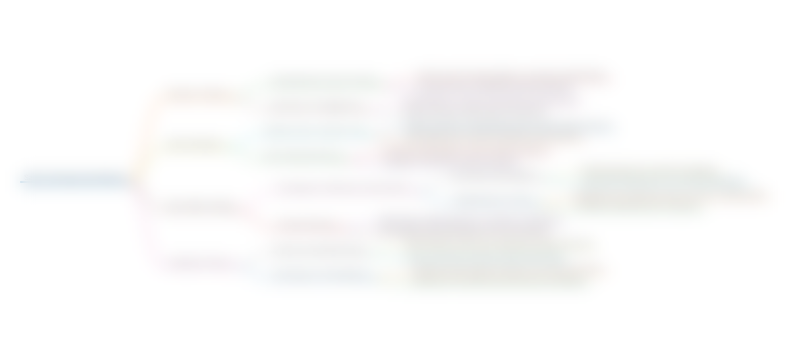
Esta sección está disponible solo para usuarios con suscripción. Por favor, mejora tu plan para acceder a esta parte.
Mejorar ahoraKeywords
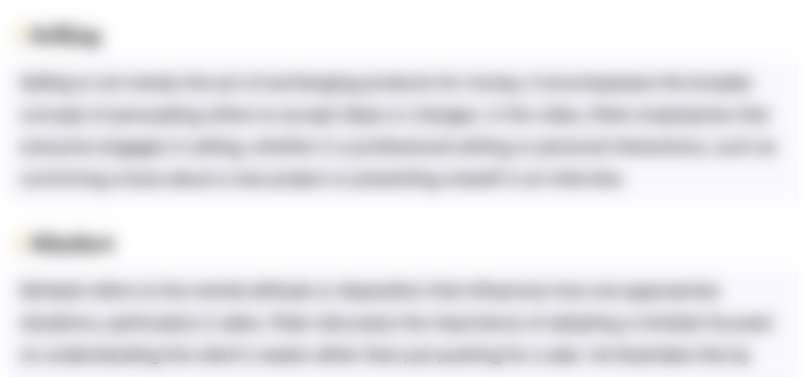
Esta sección está disponible solo para usuarios con suscripción. Por favor, mejora tu plan para acceder a esta parte.
Mejorar ahoraHighlights
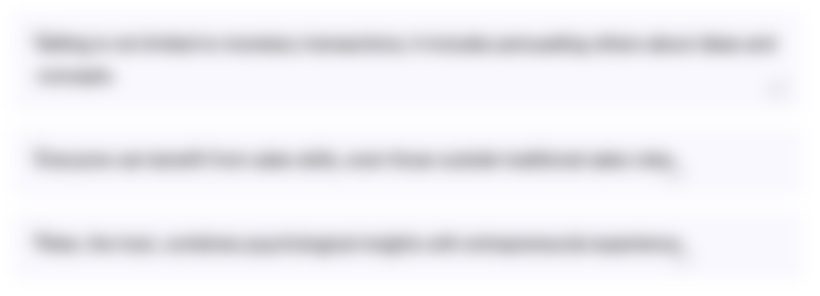
Esta sección está disponible solo para usuarios con suscripción. Por favor, mejora tu plan para acceder a esta parte.
Mejorar ahoraTranscripts
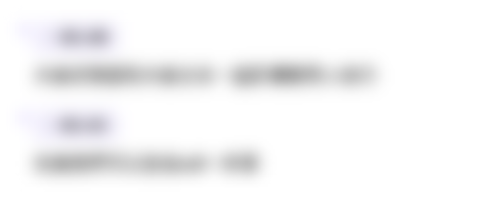
Esta sección está disponible solo para usuarios con suscripción. Por favor, mejora tu plan para acceder a esta parte.
Mejorar ahora5.0 / 5 (0 votes)