Power Analysis, Clearly Explained!!!
Summary
TLDRIn this StatQuest video, Josh Starmer explains the concept of power analysis, emphasizing its importance in avoiding p-hacking. Through an example of testing two drugs, he illustrates how power analysis helps determine the ideal sample size to confidently reject the null hypothesis. The video explains how factors like overlap between distributions and sample size impact power. It also covers how to calculate effect size, and provides a step-by-step guide on how to perform a power analysis. Ultimately, the video highlights the importance of sample size in ensuring accurate conclusions in statistical testing.
Takeaways
- ๐ Power analysis helps determine the sample size needed to ensure high probability of correctly rejecting the null hypothesis.
- ๐ Power is influenced by the overlap between distributions and the sample size of the study.
- ๐ P-hacking occurs when researchers adjust sample sizes after seeing p-values, leading to unreliable results.
- ๐ If the overlap between two distributions is minimal, smaller sample sizes can still provide high power for detecting differences.
- ๐ Larger sample sizes are needed when the distributions overlap more, as this makes it harder to detect differences.
- ๐ Using more than one measurement to estimate population means improves accuracy by reducing the impact of outliers.
- ๐ A single measurement can give a poor estimate of the population mean due to high variability in estimated means.
- ๐ The central limit theorem suggests that results from power analysis apply to any underlying distribution, not just normal distributions.
- ๐ A common threshold for statistical significance (alpha) is 0.05, and a common target for power is 0.8 (80% chance of detecting true effects).
- ๐ To calculate effect size (d), the difference between group means is divided by the pooled standard deviation, giving a measure of how different the groups are.
- ๐ Once you have the effect size, power, and alpha values, you can use an online power calculator to determine the required sample size for your study.
Q & A
What is the purpose of a power analysis in statistical testing?
-A power analysis determines the sample size needed to ensure a high probability of correctly rejecting the null hypothesis when it is false. It helps avoid p-hacking by guiding the experiment's design to gather sufficient data for reliable conclusions.
Why is power analysis important for avoiding p-hacking?
-Power analysis helps avoid p-hacking by ensuring that researchers do not artificially manipulate sample sizes to achieve statistical significance. It provides a clear and scientifically sound method to determine the required sample size upfront.
What are the two main factors that affect statistical power?
-The two main factors that affect statistical power are the overlap between the two distributions being compared and the sample size. Greater overlap requires a larger sample size to achieve sufficient power.
How does sample size affect the confidence in estimated means?
-As sample size increases, the confidence in the estimated means improves because the extreme effects of outliers are diminished. Larger sample sizes lead to estimated means that are closer to the population mean, reducing variability.
What happens when you only collect one measurement to estimate the population mean?
-When only one measurement is collected, the estimated mean can vary widely, making it unreliable. There is a high chance that the estimate will be far from the actual population mean, which reduces confidence in the result.
What is the relationship between overlap of distributions and sample size?
-If the distributions overlap significantly, a larger sample size is needed to detect a meaningful difference between the groups. In contrast, when there is little overlap, smaller sample sizes can still achieve high power.
How does the central limit theorem relate to power analysis?
-The central limit theorem indicates that as sample size increases, the distribution of sample means approaches a normal distribution, even if the underlying data are not normally distributed. This helps improve the reliability of statistical tests and is fundamental to conducting power analysis.
What is an effect size and how is it used in power analysis?
-Effect size is a measure of the difference between two means, divided by the pooled standard deviation. It quantifies the magnitude of the difference and is used in power analysis to estimate the overlap between distributions and determine the required sample size.
What is the typical value of power used in a power analysis, and why?
-A common value for power is 0.8, which corresponds to an 80% probability of correctly rejecting the null hypothesis when it is false. This value provides a reasonable balance between minimizing the risk of Type II errors and ensuring sufficient sample size.
How does increasing the sample size affect the probability of rejecting the null hypothesis?
-Increasing the sample size decreases the variability in the estimated means, which increases the probability of correctly rejecting the null hypothesis. This is because larger sample sizes make it easier to detect true differences between groups.
Outlines
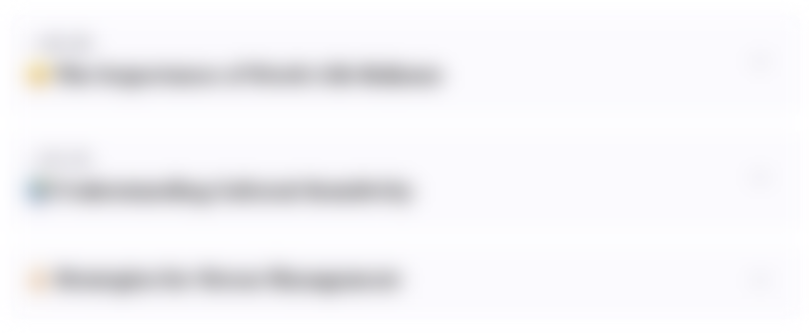
This section is available to paid users only. Please upgrade to access this part.
Upgrade NowMindmap
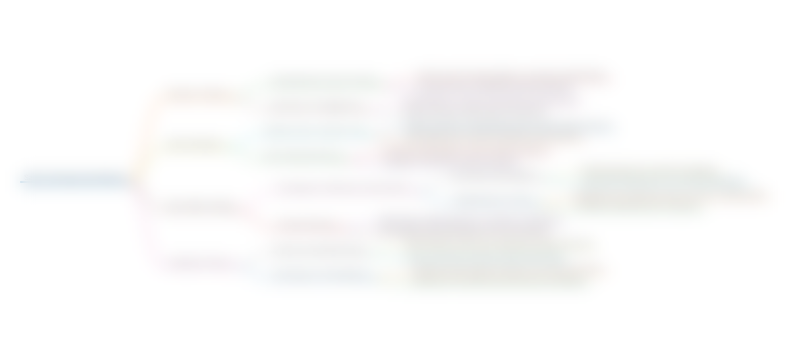
This section is available to paid users only. Please upgrade to access this part.
Upgrade NowKeywords
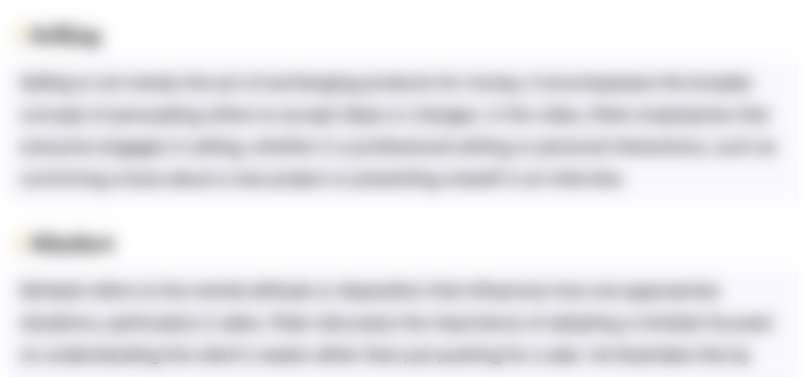
This section is available to paid users only. Please upgrade to access this part.
Upgrade NowHighlights
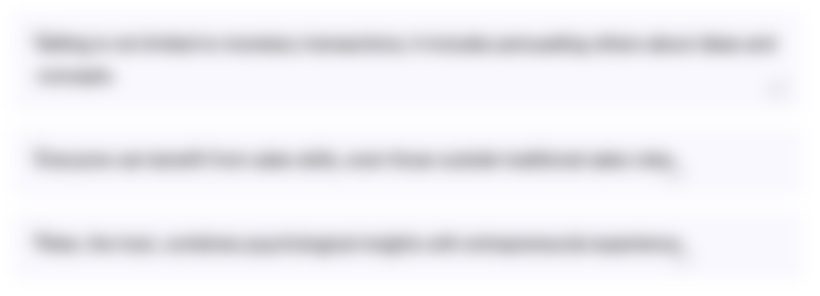
This section is available to paid users only. Please upgrade to access this part.
Upgrade NowTranscripts
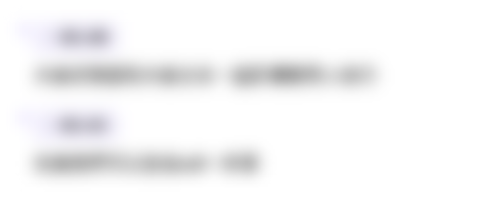
This section is available to paid users only. Please upgrade to access this part.
Upgrade Now5.0 / 5 (0 votes)