Linear Regression, Clearly Explained!!!
Summary
TLDRThis video, part of the 'StatQuest' series, offers a detailed explanation of linear regression, a powerful statistical method used to fit a line to data and predict outcomes. The video covers the key concepts of linear regression, including least squares, R-squared, and the calculation of p-values, which help assess the strength and significance of the model. Through engaging examples, the video illustrates how these concepts work together to quantify relationships in data, making complex statistical ideas accessible and understandable.
Takeaways
- 📊 **Linear Regression Basics**: The video introduces linear regression, explaining that the primary steps involve using least squares to fit a line to data, calculating R-squared, and then determining a p-value for R-squared.
- 📉 **Fitting a Line**: Linear regression uses the least squares method to find the best-fitting line for the data, minimizing the sum of squared residuals, which are the differences between observed and predicted values.
- 🔍 **Understanding R-squared**: R-squared is a statistical measure that represents the proportion of variance for a dependent variable that's explained by an independent variable or variables in a regression model.
- 🐭 **Example with Mice**: The video uses a dataset of mice, where the aim is to predict mouse size based on weight, showcasing how linear regression can be applied to real-world data.
- ⚖️ **Sum of Squares**: The sum of squares around the mean is compared to the sum of squares around the fitted line, which helps calculate R-squared by showing how much variance is explained by the model.
- 🔄 **Multiple Parameters**: When multiple predictors are used (e.g., mouse weight and tail length), linear regression fits a plane rather than a line, showing that more complex relationships can be modeled.
- 📈 **Adjusted R-squared**: The concept of adjusted R-squared is introduced, which accounts for the number of predictors in the model, providing a more accurate measure when multiple variables are involved.
- 🤔 **Limitations of R-squared**: R-squared alone doesn't indicate whether a model is statistically significant, especially with small datasets or when fitting models to random data points.
- 🔬 **Calculating p-values**: The video explains the process of calculating p-values using the F-statistic, which compares the variance explained by the model to the variance not explained, helping determine statistical significance.
- 🧪 **Practical Application**: Linear regression is highlighted as a powerful tool for quantifying relationships in data, but it requires both a high R-squared value and a low p-value to confirm the reliability and significance of results.
Q & A
What is the main topic of the video script?
-The main topic of the video script is linear regression, specifically general linear models, also known as multiple regression.
What are the three most important concepts behind linear regression mentioned in the script?
-The three most important concepts behind linear regression mentioned in the script are: fitting a line to the data using least squares, calculating the R-squared value, and calculating a p-value for R-squared.
What is the purpose of using least squares in linear regression?
-The purpose of using least squares in linear regression is to find the best-fitting line for the data by minimizing the sum of the squares of the vertical distances (residuals) between the observed data points and the fitted line.
What does the R-squared value represent in the context of linear regression?
-The R-squared value represents the proportion of the variance in the dependent variable that is predictable from the independent variable(s). It indicates the strength of the relationship between the variables.
How is the R-squared value calculated in the script's example with mouse size and weight?
-The R-squared value is calculated by taking the difference between the variation around the mean and the variation around the fit, then dividing that by the variation around the mean. In the script's example, the R-squared value is 0.6, indicating that 60% of the variation in mouse size can be explained by mouse weight.
What is the significance of calculating a p-value for R-squared in linear regression?
-Calculating a p-value for R-squared is important to determine if the observed R-squared value is statistically significant, which helps to assess whether the relationship between the variables is likely due to chance or a true association.
What does the term 'residual' mean in the context of linear regression?
-In the context of linear regression, a 'residual' refers to the difference between the observed value and the value predicted by the regression line for a given data point.
How does the script explain the concept of degrees of freedom in the context of calculating the p-value for R-squared?
-The script explains that degrees of freedom are used to turn the sums of squares into variances in the context of calculating the p-value for R-squared. They are related to the number of parameters in the fit line and the mean line, and they are used to adjust the sums of squares to account for the number of data points and parameters.
What is the role of the F-distribution in calculating the p-value for R-squared?
-The F-distribution is used to approximate the histogram of F-scores that would be obtained if many random datasets were generated and analyzed in the same way. The p-value is then determined by comparing the F-score from the original dataset to this distribution to see how extreme it is relative to the expected distribution of F-scores.
How does the script illustrate the potential issue with adding too many parameters to a regression model?
-The script illustrates the potential issue by pointing out that adding more parameters to a model can lead to a higher R-squared value due to random chance, even if those parameters do not have a true relationship with the dependent variable. This is why adjusted R-squared and p-values are important to ensure the model's validity.
What is the adjusted R-squared and why is it used in regression analysis?
-Adjusted R-squared is a modified version of R-squared that adjusts for the number of predictors in the model. It is used to provide a more accurate measure of the model's goodness of fit, especially when comparing models with different numbers of predictors, as it penalizes the addition of unnecessary predictors.
Outlines
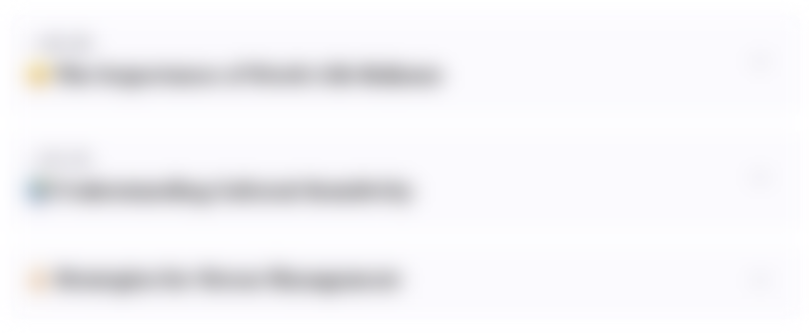
Dieser Bereich ist nur für Premium-Benutzer verfügbar. Bitte führen Sie ein Upgrade durch, um auf diesen Abschnitt zuzugreifen.
Upgrade durchführenMindmap
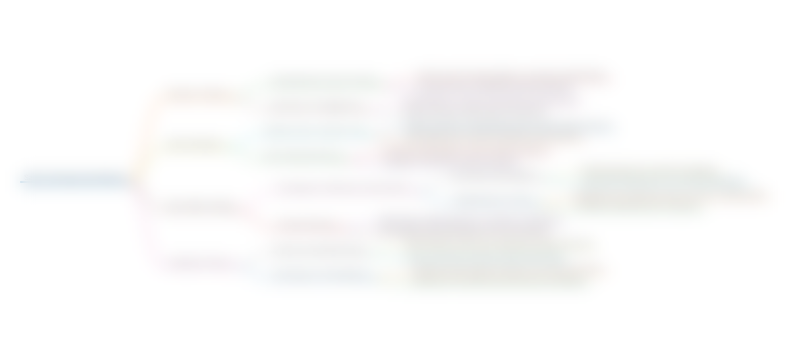
Dieser Bereich ist nur für Premium-Benutzer verfügbar. Bitte führen Sie ein Upgrade durch, um auf diesen Abschnitt zuzugreifen.
Upgrade durchführenKeywords
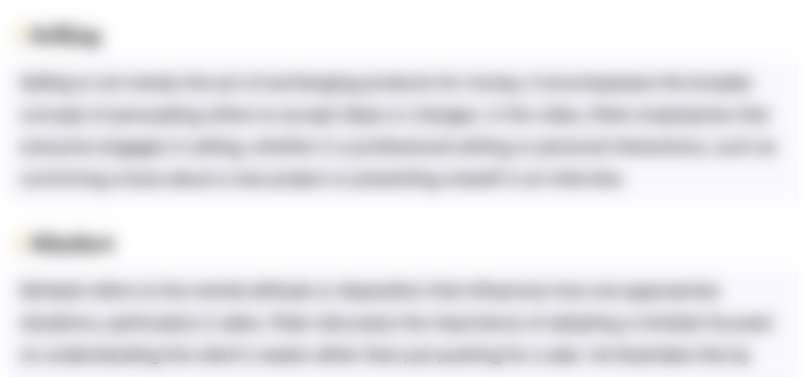
Dieser Bereich ist nur für Premium-Benutzer verfügbar. Bitte führen Sie ein Upgrade durch, um auf diesen Abschnitt zuzugreifen.
Upgrade durchführenHighlights
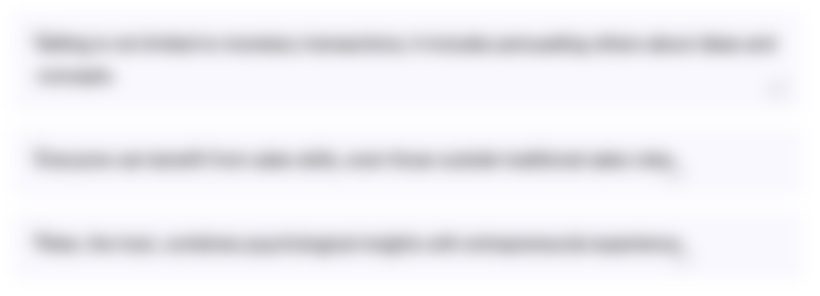
Dieser Bereich ist nur für Premium-Benutzer verfügbar. Bitte führen Sie ein Upgrade durch, um auf diesen Abschnitt zuzugreifen.
Upgrade durchführenTranscripts
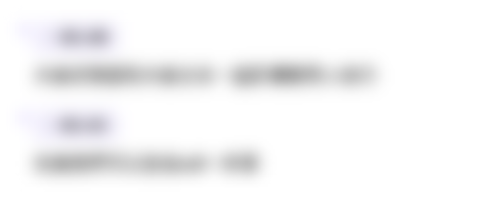
Dieser Bereich ist nur für Premium-Benutzer verfügbar. Bitte führen Sie ein Upgrade durch, um auf diesen Abschnitt zuzugreifen.
Upgrade durchführenWeitere ähnliche Videos ansehen
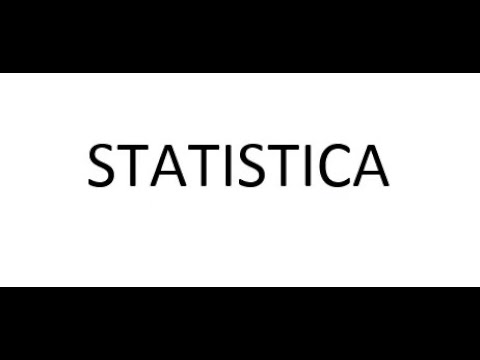
STATISTICA parte 2.3 : correlazione e regressione
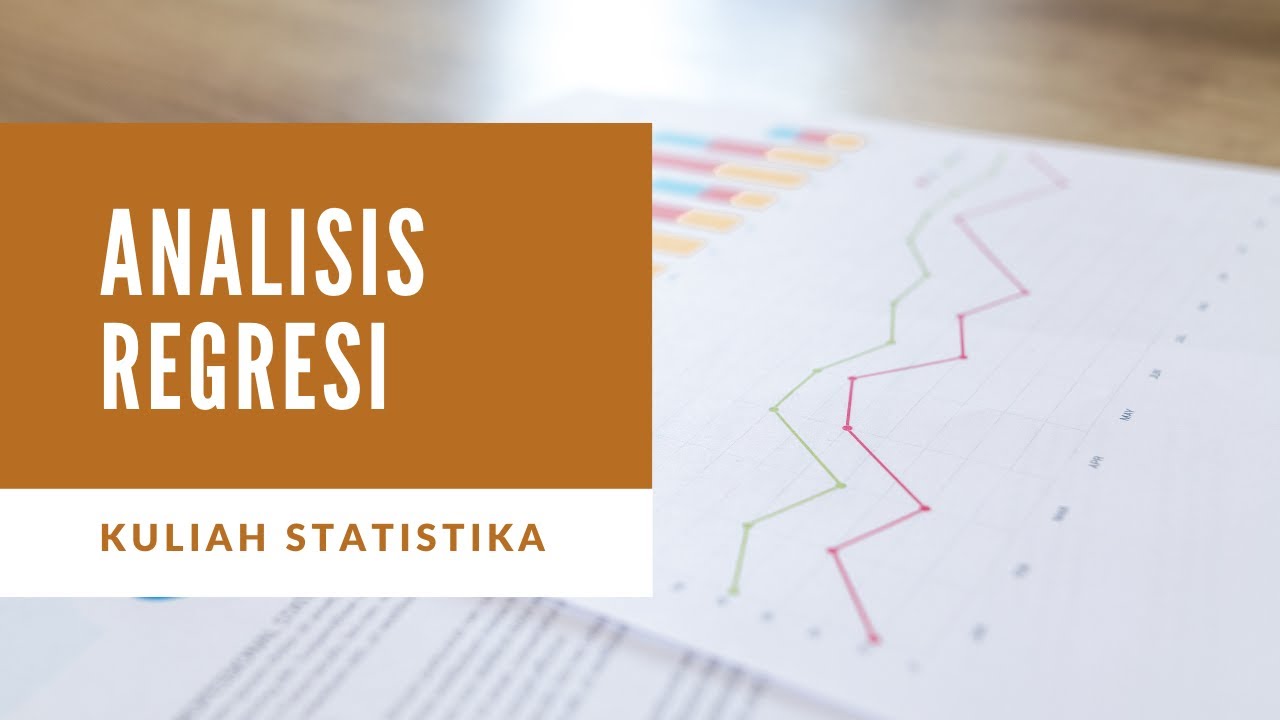
KULIAH STATISTIK - ANALISIS REGRESI
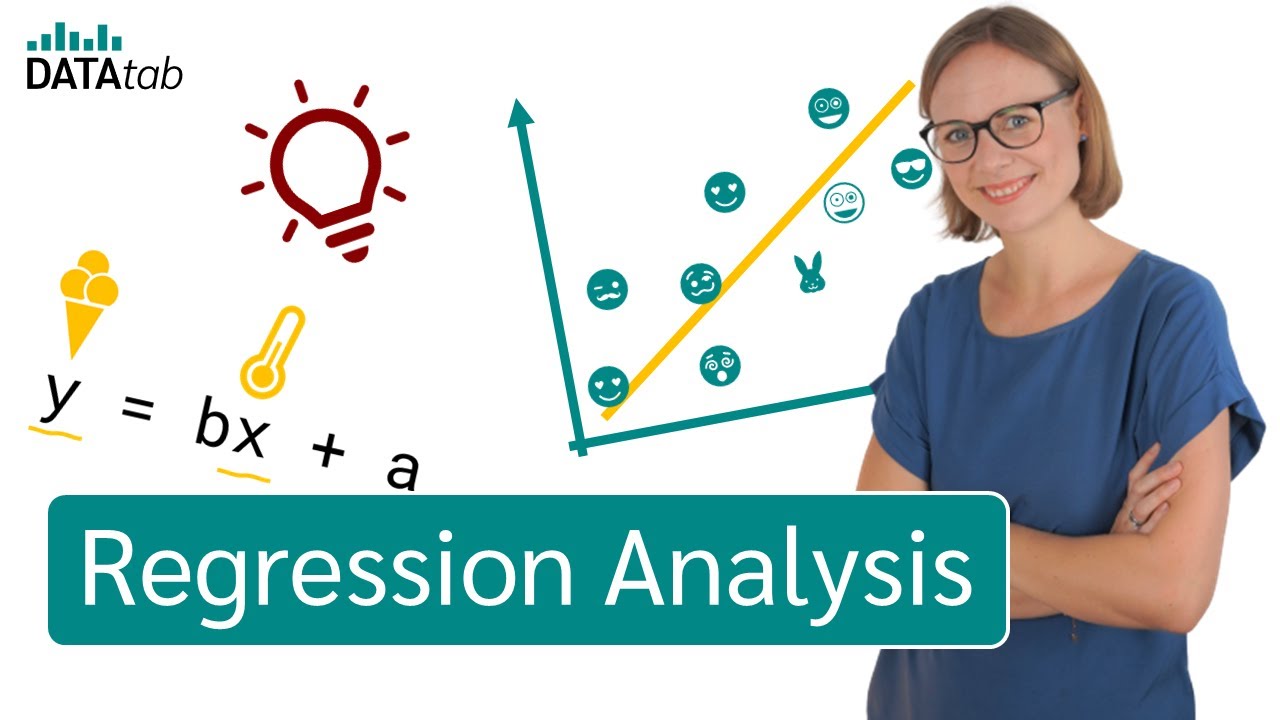
79% of Regression Analysis Basics in under 18 Minutes [Simple, Multiple and Logistic Regression]
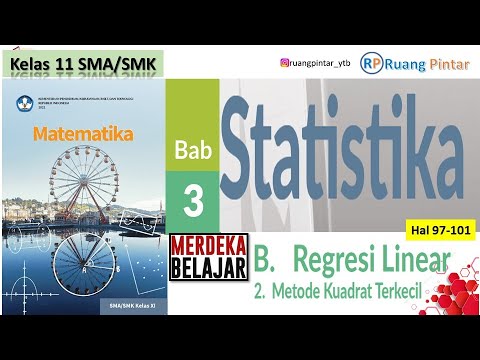
Metode Kuadrat Terkecil Hal 97-101 Bab 3 STATISTIK Kelas 11 SMA Kurikulum Merdeka

Regression Lecture
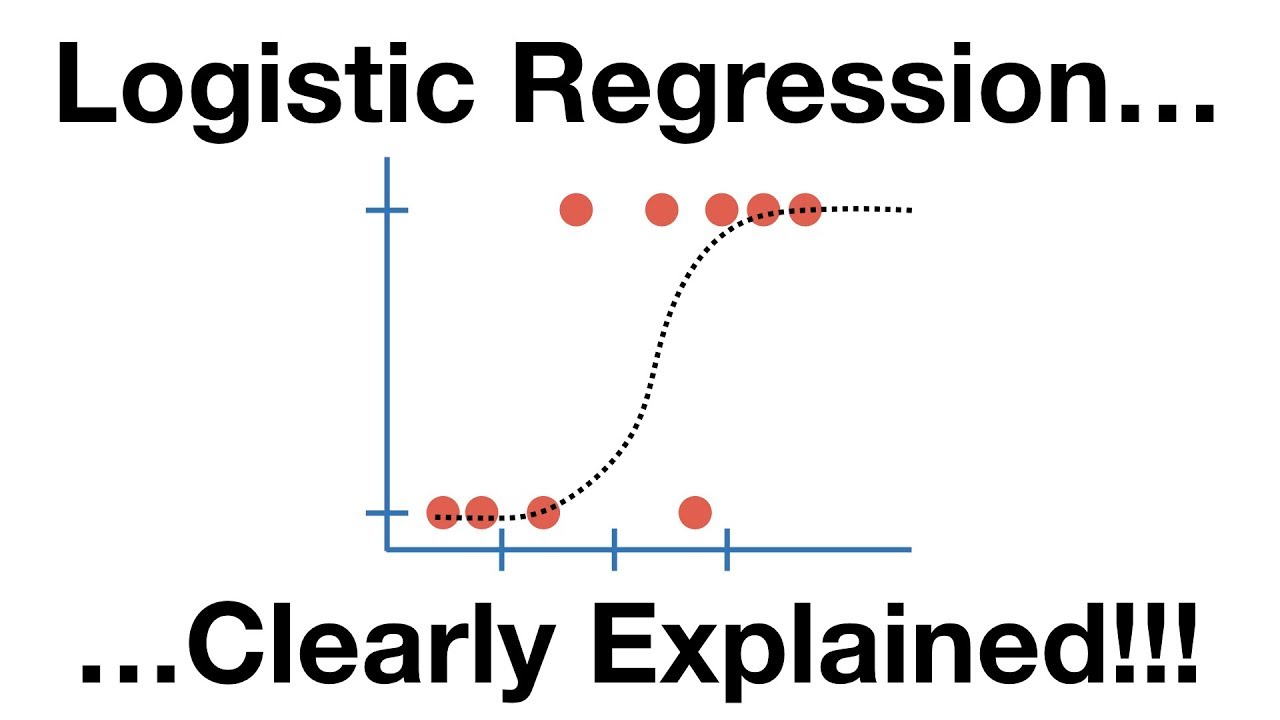
StatQuest: Logistic Regression
5.0 / 5 (0 votes)