Machine Learning Revenue Forecasting - Microsoft Case Study
Summary
TLDRIn this presentation, a Microsoft executive discusses the transformative impact of machine learning on revenue forecasting. Drawing from two decades of experience, they highlight the inefficiencies of traditional forecasting methods, which involve extensive human input and biases. By integrating machine learning, Microsoft has significantly improved accuracy, reducing adjustment rates from 3% to 1.5%, while also freeing up resources for more impactful projects. The executive emphasizes the need for time, appropriate technology, and strong buy-in from business leaders to successfully implement machine learning in forecasting, ultimately leading to more reliable financial assessments.
Takeaways
- đ Traditional forecasting at Microsoft involves multiple rounds of analysis and meetings, often taking around six weeks.
- đ The extensive time commitment required for forecasting leads to inefficiencies and delayed accuracy.
- đ€ Integrating machine learning significantly reduces human biases in the forecasting process.
- đ The accuracy of forecasts has improved from a 3% adjustment to approximately 1.5% with the use of machine learning.
- đ„ Human oversight remains crucial, especially in unprecedented situations like the pandemic, to ensure accurate assessments.
- đ Collaboration between finance analysts and data scientists is essential for effectively interpreting financial data.
- âł Implementing machine learning for forecasting is a long-term journey that took Microsoft several years to fully adopt.
- đ» Having the right technology and building trust in its capabilities are vital for successful machine learning implementation.
- đą Securing buy-in from business leaders is critical, as forecasts significantly influence sales cycles and resource allocations.
- đ The overall transformation to machine learning allows finance resources to be redirected to more impactful projects.
Q & A
What experience does the speaker have with Microsoft?
-The speaker has roughly 20 years of experience with Microsoft, working in various positions, including regional and worldwide roles in revenue forecasting.
How is the traditional forecasting process described?
-The traditional forecasting process involves multiple rounds of assessments and meetings, starting weeks before the quarter ends and continuing after the quarter closes, requiring hundreds of hours from analysts.
What are the main issues with the traditional forecasting method?
-The main issues include human bias, a lengthy forecasting cycle, and inefficiencies that consume a significant amount of time and resources.
How has machine learning impacted the forecasting process at Microsoft?
-Machine learning has improved the accuracy of forecasts, reduced the time needed for forecasting, and eliminated much of the human bias previously involved in the process.
What two types of forecasts does Microsoft utilize?
-Microsoft utilizes product forecasts, which focus on customer needs and product sales, and sales forecasts, which incorporate pipeline data, market trends, and revenue details.
Why is human interaction still important in the forecasting process?
-Human interaction remains essential for interpreting data and making informed decisions, especially in situations like the pandemic, where historical data may not fully apply.
What types of data does Microsoft consider in its forecasting?
-Microsoft considers various data types, including historical revenue data, recurring contracts, new business, macroeconomic trends, and new product launches.
What improvements have been observed in forecast accuracy due to machine learning?
-Forecast accuracy has improved from an adjustment of about 3% to around 1.5%, with ongoing improvements expected as the system learns from past forecast rounds.
What are the three key elements for successful implementation of machine learning in forecasting?
-The three key elements are time for implementation, the right technology, and buy-in from business leaders.
How long did it take for Microsoft to convince leaders of the benefits of machine learning in forecasting?
-It took Microsoft several years to convince business leaders and field personnel of the benefits of using machine learning for forecasting.
Outlines
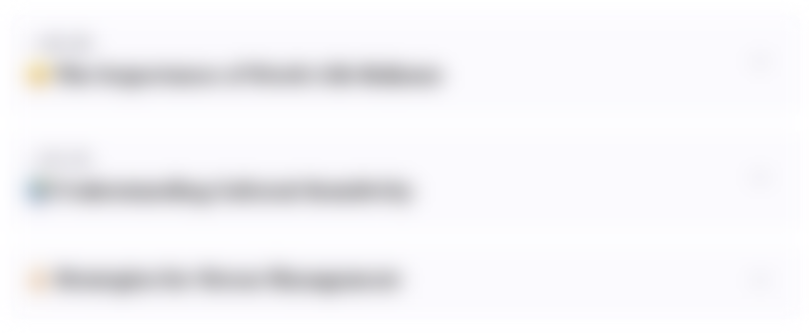
Dieser Bereich ist nur fĂŒr Premium-Benutzer verfĂŒgbar. Bitte fĂŒhren Sie ein Upgrade durch, um auf diesen Abschnitt zuzugreifen.
Upgrade durchfĂŒhrenMindmap
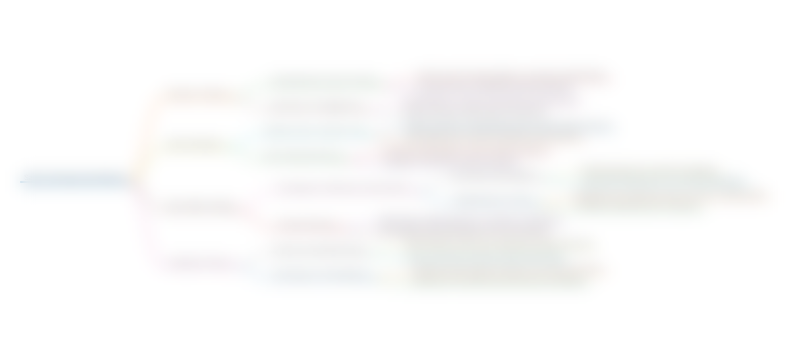
Dieser Bereich ist nur fĂŒr Premium-Benutzer verfĂŒgbar. Bitte fĂŒhren Sie ein Upgrade durch, um auf diesen Abschnitt zuzugreifen.
Upgrade durchfĂŒhrenKeywords
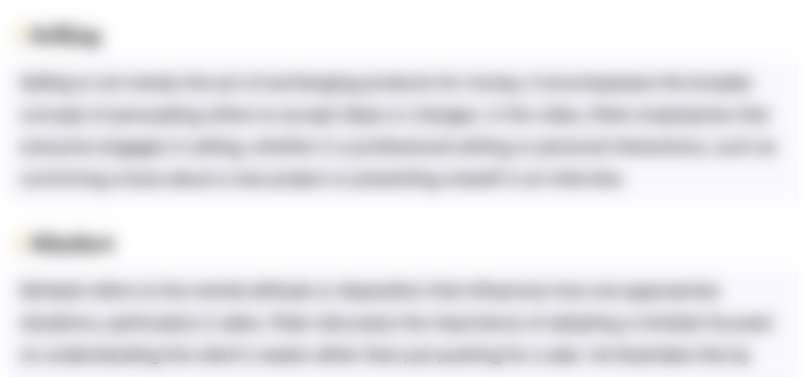
Dieser Bereich ist nur fĂŒr Premium-Benutzer verfĂŒgbar. Bitte fĂŒhren Sie ein Upgrade durch, um auf diesen Abschnitt zuzugreifen.
Upgrade durchfĂŒhrenHighlights
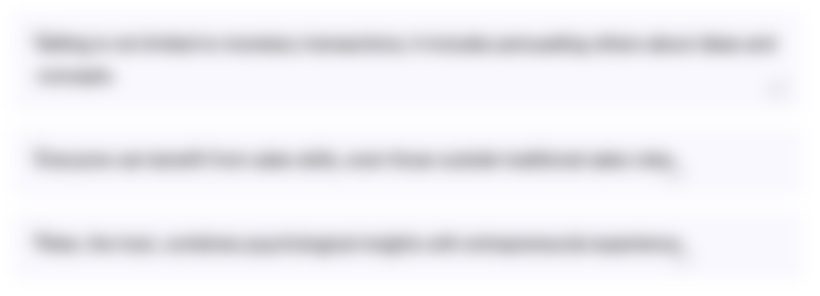
Dieser Bereich ist nur fĂŒr Premium-Benutzer verfĂŒgbar. Bitte fĂŒhren Sie ein Upgrade durch, um auf diesen Abschnitt zuzugreifen.
Upgrade durchfĂŒhrenTranscripts
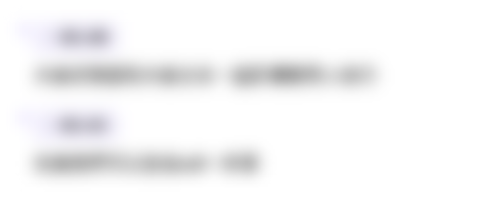
Dieser Bereich ist nur fĂŒr Premium-Benutzer verfĂŒgbar. Bitte fĂŒhren Sie ein Upgrade durch, um auf diesen Abschnitt zuzugreifen.
Upgrade durchfĂŒhrenWeitere Ă€hnliche Videos ansehen
5.0 / 5 (0 votes)