How the Child's Mind Informs AI Research - Alison Gopnik at BrainMind
Summary
TLDRThe speaker, a philosopher turned neuroscientist, discusses the profound question of how we understand the world despite receiving limited sensory input. They highlight children's remarkable ability to learn and make sense of the world with minimal data, contrasting it with current AI's reliance on vast datasets. The talk explores the potential for AI to mimic children's model-building, curiosity, and social learning, suggesting these could lead to more efficient and adaptable machine learning.
Takeaways
- 🎓 The speaker's eclectic research interests stem from a foundational problem: understanding how we gain knowledge about the world despite receiving only a narrow stream of sensory input.
- 👶 Studying children is crucial because they actively learn about the world around them, which can provide insights into how humans acquire knowledge.
- 🧠 The speaker's work in developmental psychology has challenged previous assumptions about children's understanding of other people's minds, revealing that even infants are capable of such understanding.
- 🤖 Current AI systems are compared to children with overbearing parents, as they are heavily directed and lack the autonomy to explore and learn from their environment.
- 🧪 Experiments have shown that children as young as 18 months can infer intentions and help others, indicating a complex understanding of social dynamics.
- 🌟 Studying how children learn could help design AI systems that are more capable of generalizing knowledge and learning from limited data.
- 🧠 The speaker highlights three areas where children excel over current AI: model building, curiosity and exploration, and social learning.
- 🤖 AI systems could potentially be designed to be more curious, intrinsically motivated, and capable of learning from social interactions.
- 🔍 There's ongoing research into reinforcement learning where AI systems are rewarded not only for success but also for finding surprising or unexpected outcomes.
- 🌐 The trade-off between exploitation (using current knowledge effectively) and exploration (searching for new knowledge) is a key concept in AI and human cognition.
- 💭 The concept of consciousness is complex and varies greatly, suggesting that it may not be adequately captured by a single definition or theory.
Q & A
What is the foundational problem that the speaker has been concerned with throughout their career?
-The foundational problem the speaker is concerned with is understanding how we know as much as we do about the world around us, given the limited sensory input we receive.
Why does the speaker believe studying children is a good way to answer questions about knowledge acquisition?
-The speaker believes studying children is beneficial because they are actively acquiring knowledge about the world, similar to how AI systems are designed to learn from data.
What did the speaker and other developmental psychologists discover about children's understanding of other people's minds?
-They discovered that even young babies are trying to figure out what's happening in other people's minds, contrary to previous beliefs that children are egocentric.
How did Felix Warneken's experiment with 18-month-olds demonstrate an understanding of others' intentions?
-The experiment showed that 18-month-olds would give a dropped pencil to an adult, but not if the adult threw it, indicating they inferred the adult's intentions and were actively trying to fulfill a perceived desire.
What are the three things that children do, which current AI systems are not good at, according to the speaker?
-The three things are: model building, being curious and exploratory, and learning socially from other people.
What is the significance of the work by colleagues at Berkeley mentioned by the speaker?
-The work involves designing AI systems that are reinforced when they fail, encouraging them to explore and find surprising things, which leads to more robust learning compared to systems that only follow rewards.
What is the 'explorer exploit trade-off' mentioned in the script?
-It refers to the intrinsic balance between being efficient at solving a problem and exploring the space of possibilities for potential solutions.
How does the speaker view the question of consciousness?
-The speaker suggests that the question of consciousness might be a 'bad question', implying that it may be too complex or ill-defined to have a single answer, and that consciousness could be experienced in many different forms.
What does the speaker imply about the consciousness of babies compared to adult philosophers?
-The speaker implies that babies' consciousness is very different from that of adult philosophers, suggesting that the latter's self-reflective consciousness might be an alteration from a more baseline state.
What does the speaker mean when they say consciousness could be a 'bad question'?
-The speaker implies that the concept of consciousness is so complex and multifaceted that it may not be possible to define it in a way that encompasses all its forms and experiences.
Outlines
🔍 The Roots of Knowledge and Learning
The speaker, a philosopher turned neuroscientist, discusses their foundational interest in understanding how humans acquire knowledge about the world. They emphasize the narrow sensory input we receive and the vast knowledge we possess, questioning how this is possible. The speaker's focus is on children, who they see as the epitome of learning, as they actively interpret sensory data to understand the world. This leads to the idea of creating computers that can learn in a similar way, which is highly relevant in the age of AI. The speaker also touches on the importance of studying children's understanding of others' minds, which has been a significant area of research since the 70s and 80s, revealing that even infants are capable of understanding others' mental states.
🤖 Learning from Babies: Principles for AI
The speaker compares the learning abilities of children to current AI systems, noting that children can learn from very limited and messy data, forming generalizable principles that apply in various situations. This is in contrast to AI systems that require vast amounts of curated data. The speaker identifies three key areas where children excel and current AI struggles: model building, curiosity and exploration, and social learning. They suggest that to improve AI, we should consider incorporating these aspects, such as building AI systems that are intrinsically motivated, can learn from others, and can explain things rather than just predict outcomes. The speaker also mentions the work of colleagues who are designing machine learning algorithms that are reinforced when they fail, encouraging exploration and leading to more robust learning.
Mindmap
Keywords
💡Neuroscience
💡Philosophy
💡Eclectic
💡Developmental Psychology
💡Cognition
💡Perception
💡AI Spring
💡Machine Learning
💡Curiosity
💡Social Learning
💡Consciousness
Highlights
Philosophy background influences neuroscience research
Interest in how we know about the world around us
Children's learning as a model for understanding cognition
Children actively figure out how the world works
Influence of developmental psychology on understanding children's knowledge
Children's understanding of other people's minds
Experiments on children's motivations and actions
Children's ability to infer intentions and help others
Studying children's learning to improve AI design
Current AI compared to children with helicopter parents
Children's learning principles are more generalizable
Three things children do that current AIs do not: model building, curiosity, social learning
AI's lack of intrinsic motivation and exploration
Children's sophisticated social learning and imitation
Designing AIs that are curious and intrinsically motivated
Reinforcement learning and the explorer-exploit trade-off
AI systems designed to be surprised and explore
Philosophical view on the question of consciousness
Consciousness as a spectrum with different states
Transcripts
i think of all the neuroscience
researchers i know you have one of the
most wide-ranging
and eclectic set of interests and
influences what influences
pre-neuroscience
do you think made you such have you have
such eclectic research interests well i
began my career and still am an
affiliate in the philosophy department
and one of the wonderful things about
being a philosopher is that you get to
think about all sorts of things but i do
think that even though on the surface
the interests that i have look
wide-ranging
there's a basic foundational problem
that's always been for my whole career
the problem that i'm concerned about and
that problem is how is it that we know
as much as we do about the world around
us
so if you look at the world we get a
narrow little stream of photons at the
back of our eyes and yet somehow we end
up knowing about a world full of people
and objects and places and science and
abstract things and the big question for
me has always been how does that happen
how is that possible it seemed to me
that a very good way of answering that
question was to look at children because
they're the ones who are doing that more
than any other creatures that we know of
they're the ones who are actually taking
that data and figuring out how the world
works and then that also leads to the
question of trying to understand what's
going on in their minds
what their motivations what their brains
and minds are like that enables them to
do that so effectively once that's what
you care about you can think about how
could you construct a computer that
could do the same thing and that's
become very relevant because the great
new ai spring has been about computers
that can learn that can take data and
make sense out of it you said that
scientists at the time who were trying
to understand how we come to understand
the world one didn't think that there
was any point in looking at children
they act as many physicists or
biologists and are actually able to come
up with
explanatory theories that they can then
use to make predictions about the world
my work and the work of a bunch of other
developmental psychologists starting in
the 70s and 80s
really basically found new techniques
for asking the question about what it is
the children knew first we discovered
that children understand things about
other people's minds
children were supposed to be so cystic
and egocentric and starting in the late
80s a number of developmental
psychologists started saying is that
really true
what do children understand about what's
going on in other people's minds and we
discovered that even even young babies
are trying to figure out what's
happening in other people's minds so
were there ways that we could ask them
in their language instead of our
language what they know and when we did
that
the sort of things that we would do is
look at what they do look at how they
act to try and help someone else so
the experiment you were talking about
very clever experiment by felix
wernicken who's now at
university of michigan he showed that
if you
took even an 18 month old and you
dropped a pencil on the floor the 18
month olds would come and give it to you
but not if you threw it to the floor
which means that they were both
inferring something about what you
wanted and also
actively trying to get you something
that you want what are some of the other
ways in which studying how
zero to ten year olds learn could help
us design ais better right so
one way that i like to put it now is if
you think look at our current ais
they're a bit like children who have
super hyper telecom helicopter tiger
moms so they have programmers who are
saying here's your score get your score
higher
and the great discovery of machine
learning in the last 10 years has been
you don't actually have to say get your
score higher by doing this you just tell
them get your score higher give them a
bunch of statistical data and billions
of examples they can figure out how to
do it themselves in some ways babies are
like the opposite of that so with very
small amounts of data
very messy data not well curated data
they seem to be able to learn
principles that are
much more generalizable that they can
apply in many more different
circumstances so the puzzle is what is
it that they're doing that's letting
them letting them
do that in a way that current ais can't
the three things that children but we
know babies are doing that current ais
are not very good at doing our
model building so actually building this
goes back to the work
i did in the 80s building theories ideas
about how the world works explaining how
the world works not just
predicting um they're curious they're
exploratory so the ais are kind of stuck
inside of their mainframes we can feed
them data but they can't go out and
get data for themselves the third thing
is learning socially so
babies are learning from other people
and they're extremely tuned into what
other people are doing they imitate
other people they listen to what other
people say but they don't just do this
in a kind of simple mindless way they do
it in a very sophisticated way so we're
taking some basic problems like figuring
out how objects work or how people work
and then trying to see could we get an
ai that is curious is intrinsically
motivated could we get an ai that can
learn from other people can imitate them
and imitate intelligently can we get an
ai that explains things that tries to
make up models and it doesn't just uh
doesn't just predict things the
curiosity
do you imagine uh
that a machine learning algorithm could
actually tell its humans
feed me a different kind of corpus of
information
there's beautiful work that my
colleagues at berkeley um poked agarol
and deepak paltech and
uh trevor daryl and anders andre malik
and ayosh efris have done
um of actually designing so one of the
big techniques in current machine
learning is reinforcement learning so
that's what i was saying you know you
have an alpha go it gets a score on an
atari game let's say and then it tries
to it gets reinforced for getting a
higher score and then it tries to figure
out how to get more reinforcement um
the very clever design that they have is
a system that is trying to build a model
of the world like kind of like
predictive coding trying to predict the
world but it also gets reinforced when
it fails
so it's going around essentially trying
to find things that are surprising
trying to find things that don't fit
with the way the world works and it gets
a little as it were shot of you know ai
dopamine when
when it's surprised when things are
weird when things don't work and it
turns out that that kind of a system
first of all does really explore the
space and it does it in a more robust
way than
uh the helicopter
ai that is just you know following the
trail of breadcrumbs of the rewards so
one of the really basic ideas that's in
ai and computation in lots of areas
is something that's called an explorer
exploit trade-off and the phenomenon is
there's an intrinsic trade-off between
what you need to do to be most effective
most efficient
get something done quickly and
effectively and what you need to do to
explore the space of possibilities and
you can kind of you know think see why
that's true right i mean there's big
giant space of possibilities only one of
them is actually or a small set is
actually going to be the one that's
going to work how do you do that how do
you explore possibilities
while you're exploring them you're not
actually effectively solving the problem
but when you're solving the problem
you're not exploring all the other ways
that you could solve the problem so
there's been some really interesting
work trying to see what humans do and
adult humans go back and forth between
different kinds of ways of trying to
solve the problem but it's really
challenging so finally um you came from
philosophy and then went into brain
science what what is consciousness
so i think that's one of those ones
where the answer is that it's a bad
question that philosophers this is an
old philosopher trick right
but i do think that's a that's true in
this case i think what's
who knows what the answer is going to be
but i think it's very unlikely that
there's going to be one answer perhaps
not coincidentally um the people who
have thought about consciousness tend to
focus on the kind of consciousness you
have when you're an adult philosopher
sitting in your armchair thinking about
consciousness which kind of makes sense
but that's really different from the
consciousness that you have when you're
a baby looking around in the world very
different from the kind of i think the
the recent work on psychedelics has
given us a lovely kind of um
demonstration of the fact that you can
have a kind of consciousness that's very
different from that sort of professorial
self-reflective consciousness you could
have a consciousness the characteristic
of which is that you don't feel a
difference between yourself and the
world anymore right when you start
casting your net more widely thinking
about animals thinking about children
thinking about
what people call altered states although
i think actually those maybe the sort of
baseline states from which professorial
consciousness is the kind of alter
alteration uh you get a much more varied
much
less predictable but much richer view of
what's going on
Weitere ähnliche Videos ansehen
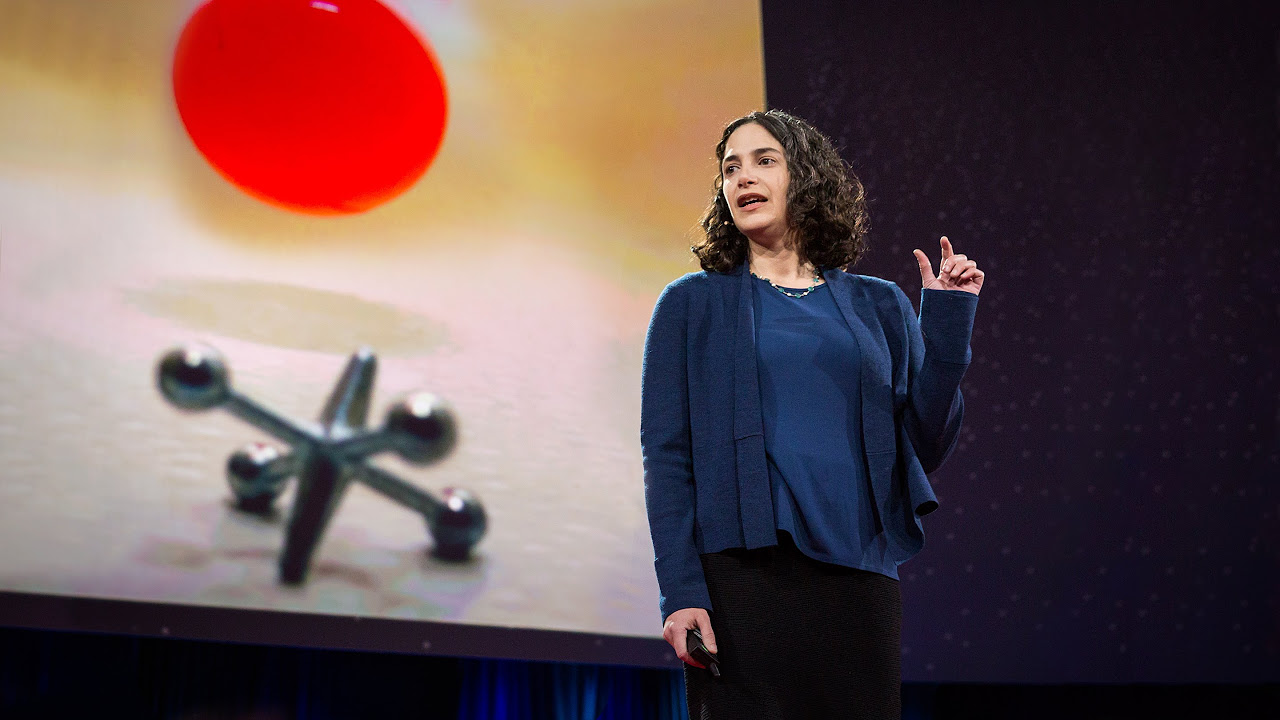
Laura Schulz: The surprisingly logical minds of babies
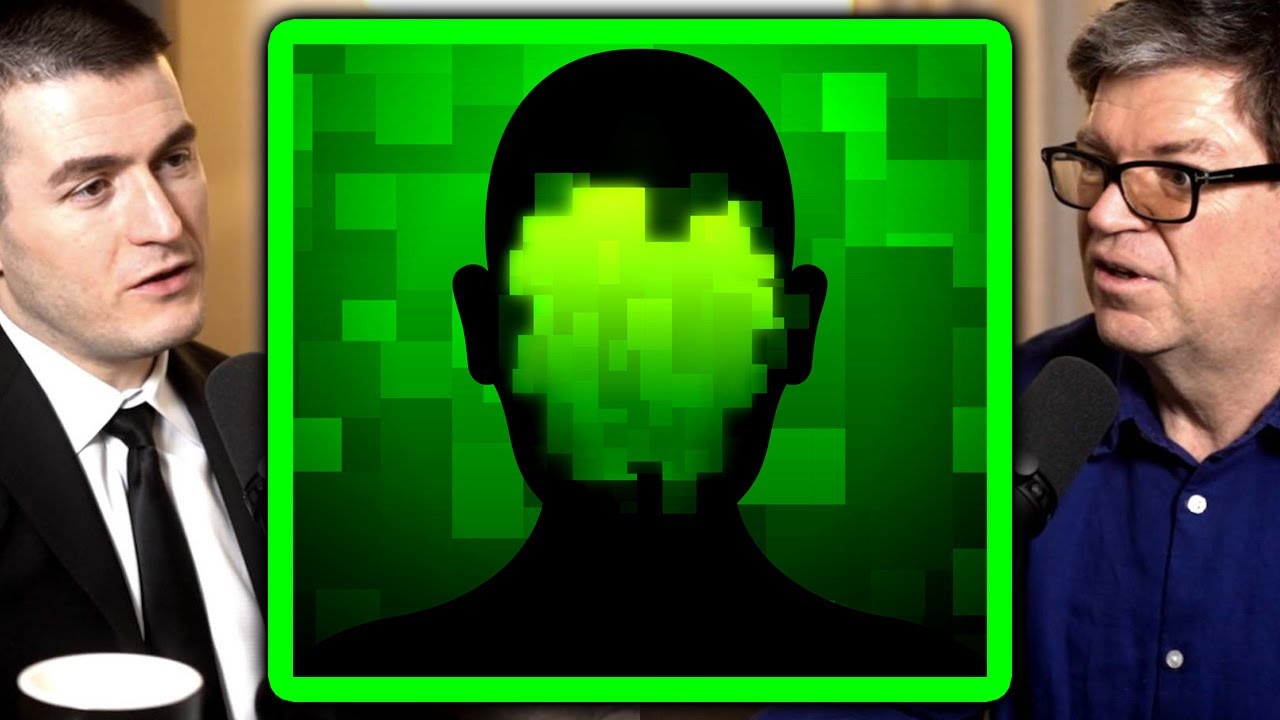
LLMs are not superintelligent | Yann LeCun and Lex Fridman

Genius Machine Learning Advice for 11 Minutes Straight
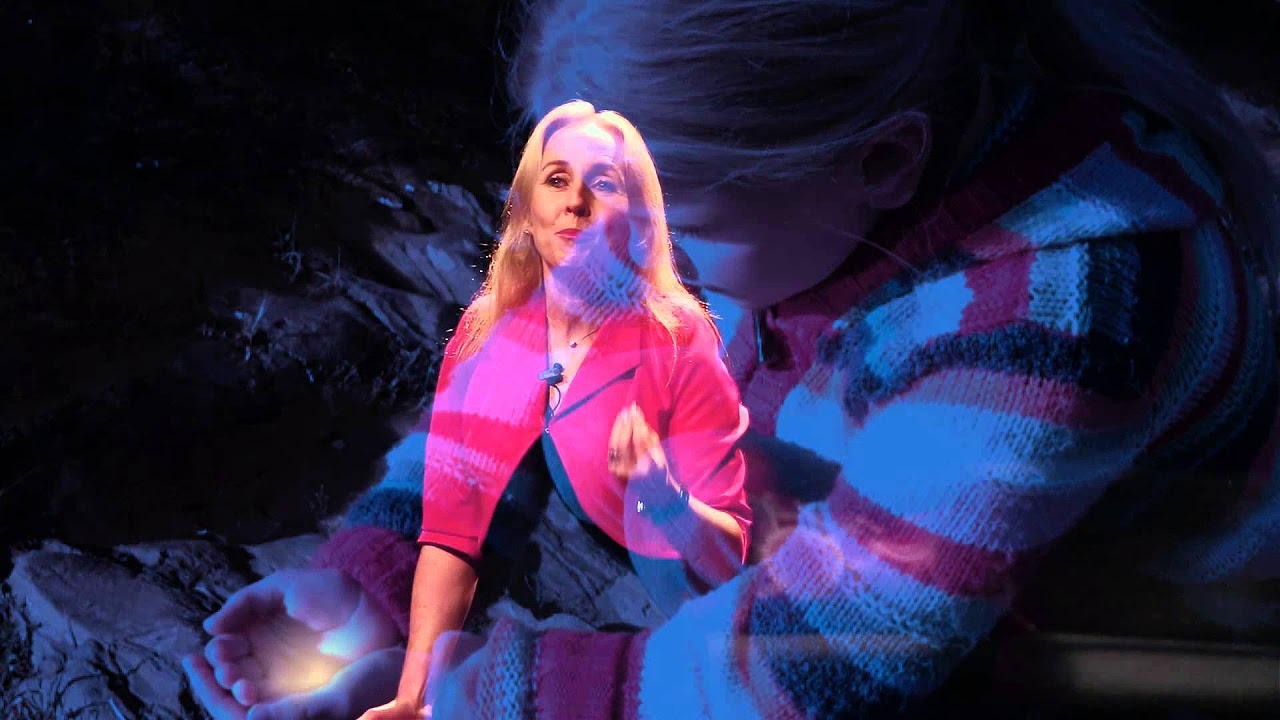
The power of ummmm... | Kath Murdoch | TEDxWestVancouverED

How to Empower Education with Artificial Intelligence | Luca Longo | TEDxDublinInstituteofTechnology

Episode 09: Machine Learning and AI
5.0 / 5 (0 votes)