NVIDIA Reveals STUNNING Breakthroughs: Blackwell, Intelligence Factory, Foundation Agents [SUPERCUT]
Summary
TLDRThe transcript discusses the significant growth of the AI industry, particularly in large language models post the invention of the Transformer model. It highlights the computational demands of training such models, with the latest OpenAI model requiring 1.8 trillion parameters and several trillion tokens. The introduction of Blackwell, a new GPU platform, is announced, which promises to reduce the energy and cost associated with training next-generation AI models. The transcript also touches on the importance of inference in AI and the potential of Nvidia's technologies in training humanoid robots through the Groot model and Isaac lab.
Takeaways
- 🚀 The AI industry has seen tremendous growth due to the scaling of large language models, doubling in size approximately every six months.
- 🧠 Doubling the model size requires a proportional increase in training token count, leading to a significant computational scale.
- 🌐 State-of-the-art models like OpenAI's GPT require training on several trillion tokens, resulting in massive floating-point operations.
- 🔄 Training such models would take millennia with a petaflop GPU, highlighting the need for more advanced hardware.
- 📈 The development of multimodality models is the next step, incorporating text, images, graphs, and charts to provide a more grounded understanding of the world.
- 🤖 Synthetic data generation and reinforcement learning will play crucial roles in training future AI models.
- 🔢 The Blackwell GPU platform represents a significant leap in computational capabilities, offering a memory-coherent system for efficient AI training.
- 🌐 Blackwell's design allows for two dies to function as one chip, with 10 terabytes per second of data transfer between them.
- 💡 Blackwell's introduction aims to reduce the cost and energy consumption associated with training the next generation of AI models.
- 🏭 The future of data centers is envisioned as AI Factories, focused on generating intelligence rather than electricity.
- 🤖 Nvidia's Project Groot is an example of an AI model designed for humanoid robots, capable of learning from human demonstrations and executing tasks with human-like movements.
Q & A
How did the invention of the Transformer model impact the scaling of language models?
-The invention of the Transformer model allowed for the scaling of large language models at an incredible rate, effectively doubling every six months. This scaling is due to the ability to increase the model size and parameter count, which in turn requires a proportional increase in training token count.
What is the computational scale required to train the state-of-the-art OpenAI model?
-The state-of-the-art OpenAI model, with approximately 1.8 trillion parameters, requires several trillion tokens to train. The combination of the parameter count and training token count results in a computation scale that demands high performance computing resources.
What is the significance of doubling the size of a model in terms of computational requirements?
-Doubling the size of a model means that you need twice as much information to fill it. Consequently, every time the parameter count is doubled, the training token count must also be appropriately increased to support the computational scale needed for training.
How does the development of larger models affect the need for more data and computational resources?
-As models grow larger, they require more data for training and more powerful computational resources to handle the increased parameter count and token count. This leads to a continuous demand for bigger GPUs and higher energy efficiency to train the next generation of AI models.
What is the role of multimodality data in training AI models?
-Multimodality data, which includes text, images, graphs, and charts, is used to train AI models to provide them with a more comprehensive understanding of the world. This approach helps models develop common sense and grounded knowledge in physics, similar to how humans learn from watching TV and experiencing the world around them.
How does synthetic data generation contribute to the learning process of AI models?
-Synthetic data generation allows AI models to use simulated data for learning, similar to how humans use imagination to predict outcomes. This technique enhances the model's ability to learn and adapt to various scenarios without the need for extensive real-world data.
What is the significance of the Blackwell GPU platform in the context of AI model training?
-The Blackwell GPU platform represents a significant advancement in AI model training by offering a highly efficient and energy-saving solution. It is designed to handle the computational demands of training large language models and can reduce the number of GPUs needed, as well as the energy consumption, compared to previous generations of GPUs.
How does the Blackwell system differ from traditional GPU designs?
-The Blackwell system is a platform that includes a chip with two dies connected in such a way that they function as one, with no memory locality or cache issues. It offers 10 terabytes per second of data transfer between the two sides, making it a highly integrated and coherent system for AI computations.
What is the expected training time for a 1.8 trillion parameter GPT model with the Blackwell system?
-Using the Blackwell system, the training time for a 1.8 trillion parameter GPT model is expected to be the same as with Hopper, approximately 90 days, but with a significant reduction in the number of GPUs required (from 8,000 to 2,000) and a decrease in energy consumption from 15 megawatts to only four megawatts.
How does the Blackwell system enhance inference capabilities for large language models?
-The Blackwell system is designed for trillion parameter generative AI and offers an inference capability that is 30 times greater than Hopper for large language models. This is due to its advanced features like the FP4 tensor core, the new Transformer engine, and the Envy link switch, which allows for faster communication between GPUs.
What is the role of the Jetson Thor robotics chips in the future of AI-powered robotics?
-The Jetson Thor robotics chips are designed to power the next generation of AI-powered robots, enabling them to learn from human demonstrations and emulate human movements. These chips, along with technologies like Isaac Lab and Osmo, provide the building blocks for advanced AI-driven robotics that can assist with everyday tasks.
Outlines
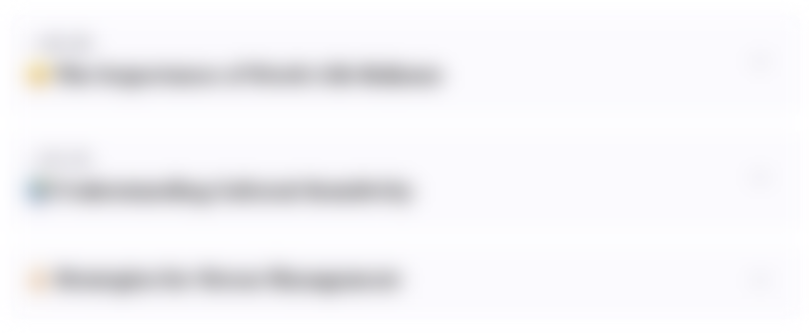
هذا القسم متوفر فقط للمشتركين. يرجى الترقية للوصول إلى هذه الميزة.
قم بالترقية الآنMindmap
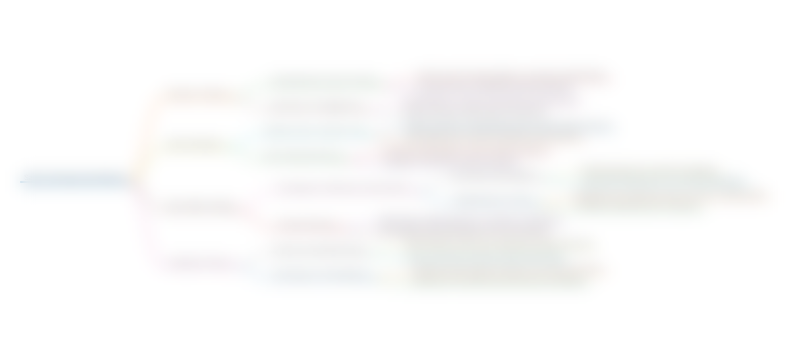
هذا القسم متوفر فقط للمشتركين. يرجى الترقية للوصول إلى هذه الميزة.
قم بالترقية الآنKeywords
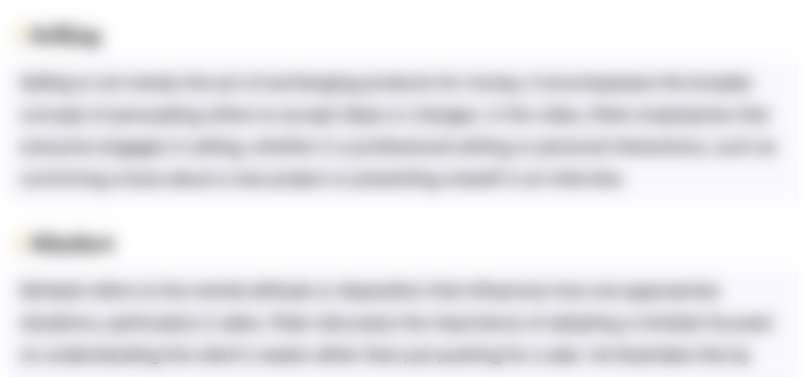
هذا القسم متوفر فقط للمشتركين. يرجى الترقية للوصول إلى هذه الميزة.
قم بالترقية الآنHighlights
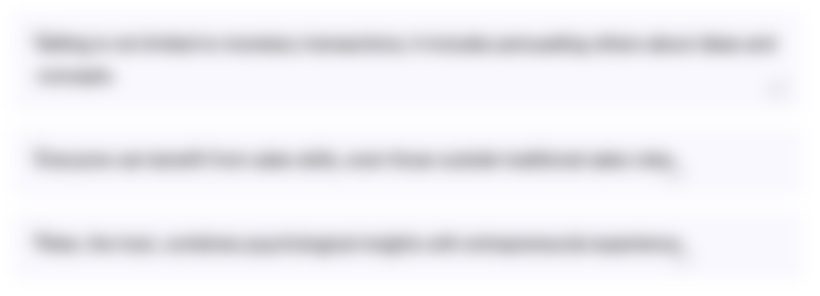
هذا القسم متوفر فقط للمشتركين. يرجى الترقية للوصول إلى هذه الميزة.
قم بالترقية الآنTranscripts
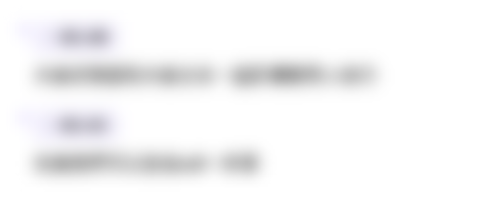
هذا القسم متوفر فقط للمشتركين. يرجى الترقية للوصول إلى هذه الميزة.
قم بالترقية الآنتصفح المزيد من مقاطع الفيديو ذات الصلة

Nvidia's Breakthrough AI Chip Defies Physics
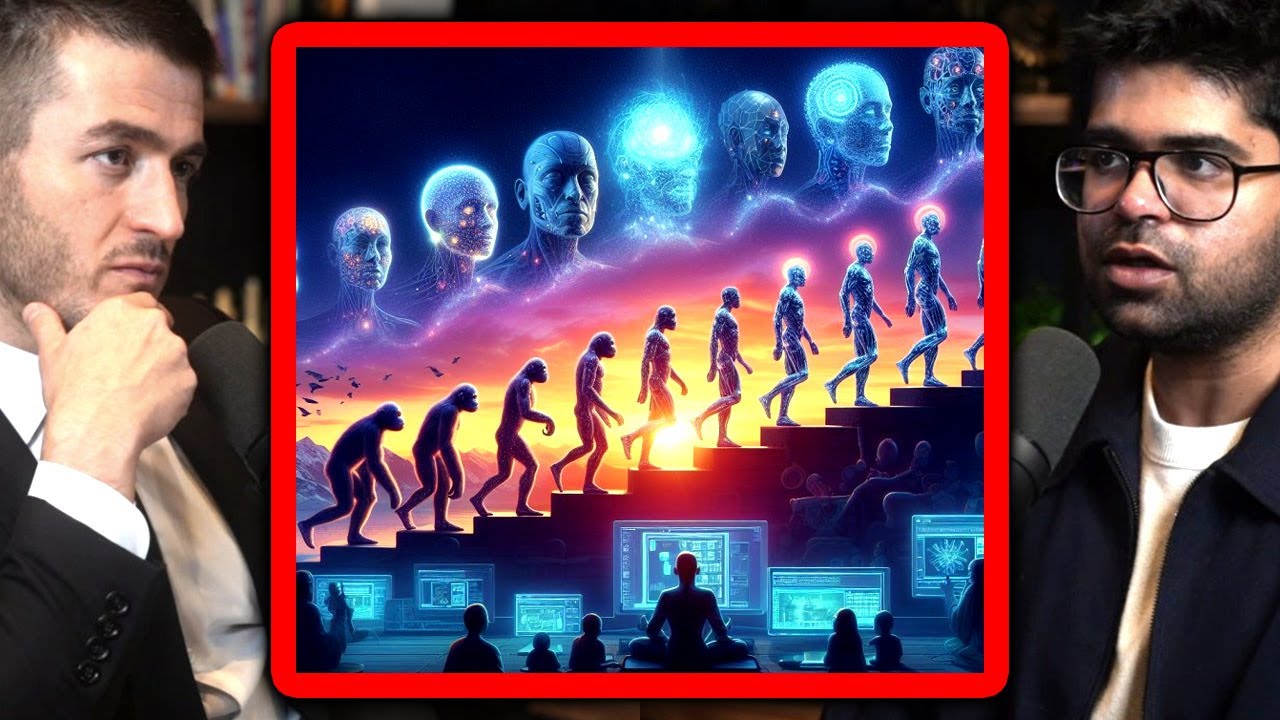
Recent breakthroughs in AI: A brief overview | Aravind Srinivas and Lex Fridman
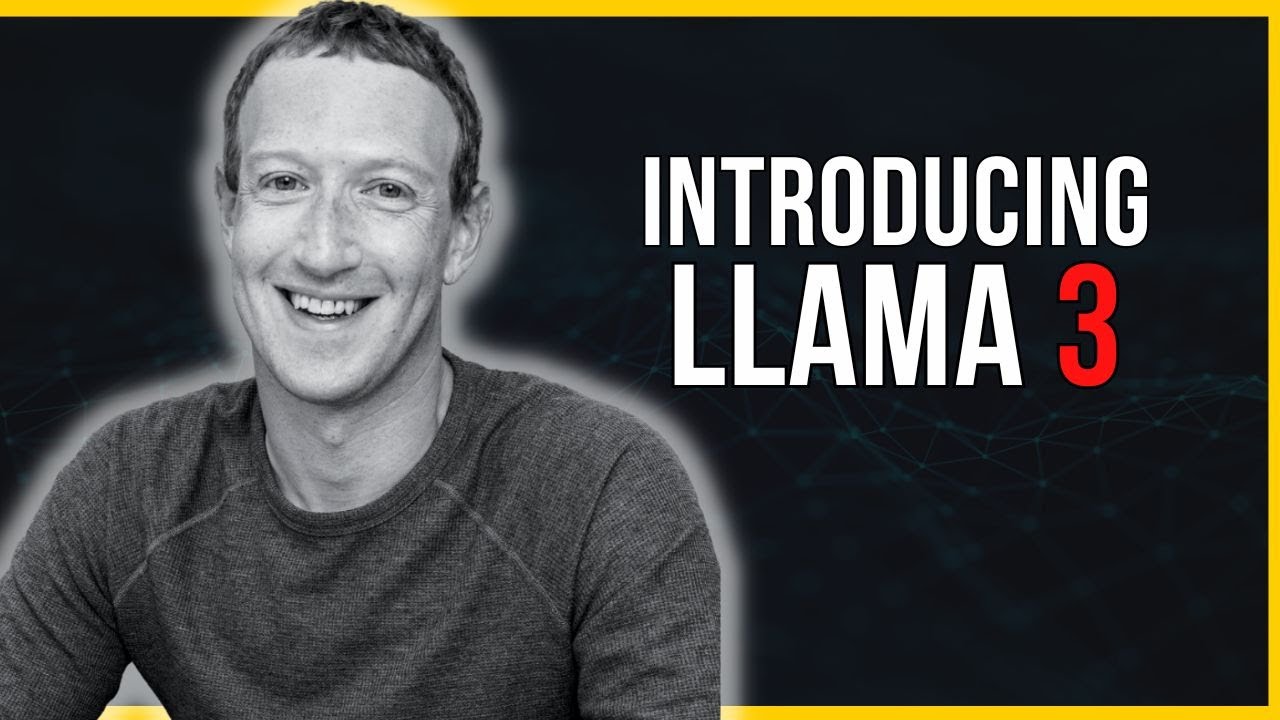
LLAMA 3 Released - All You Need to Know
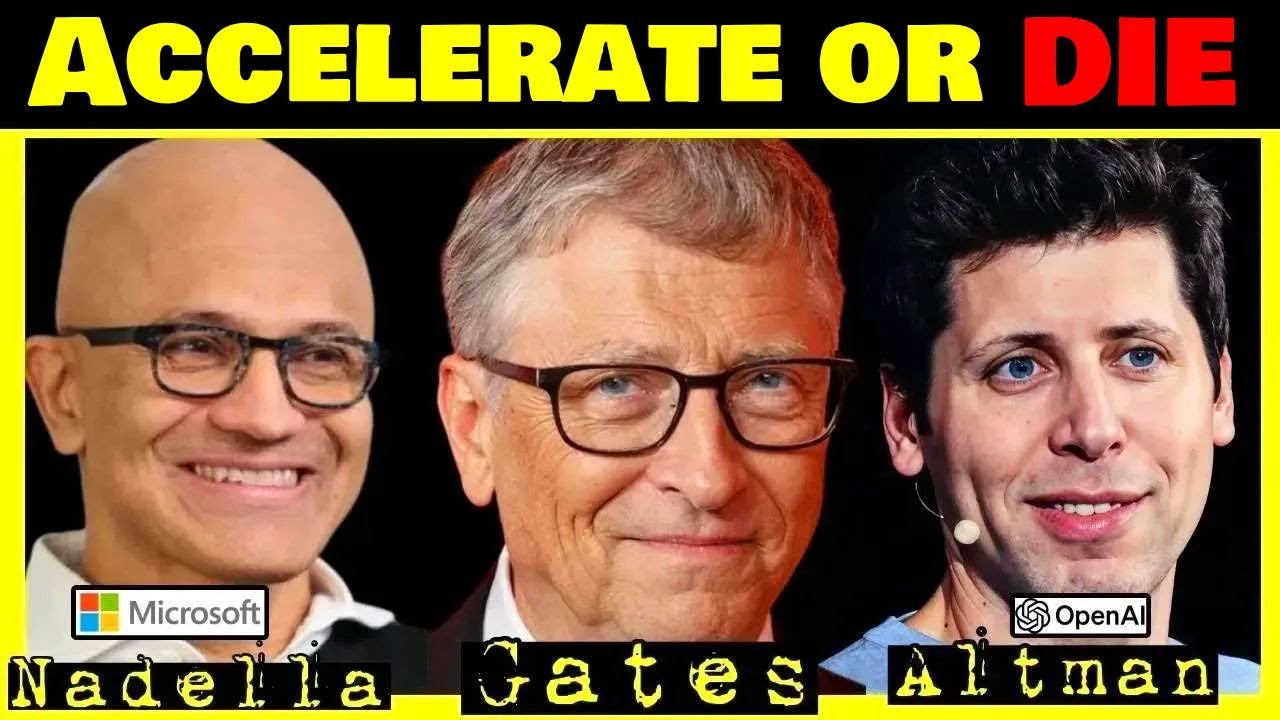
Microsoft Reveals SECRET NEW MODEL | GPT-5 DELAYED | Sam Altman speaks out against "Doomers"
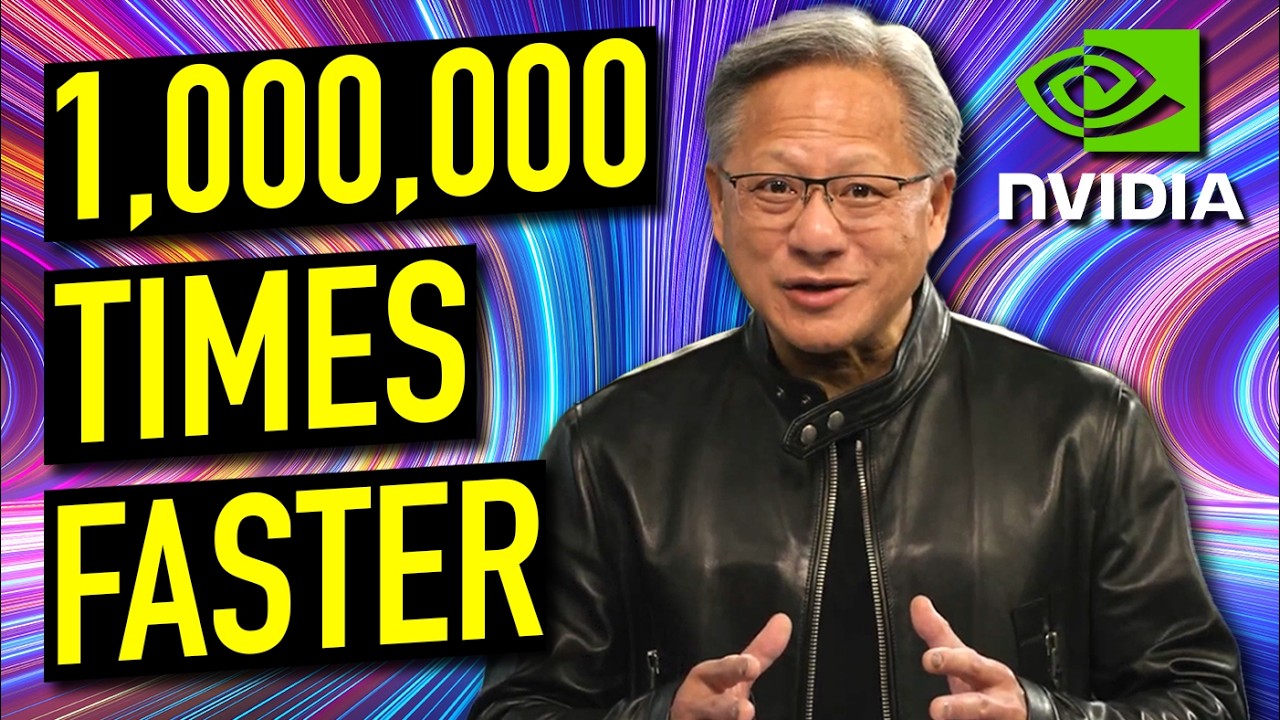
NVIDIA CEO Jensen Huang Leaves Everyone SPEECHLESS (Supercut)

Is DeepSeek stealing from OpenAI | Lex Fridman Podcast
5.0 / 5 (0 votes)