Scale Your Data Ingestion With an Ingestion Framework
Summary
TLDRIn this webinar, Liz McQuish and Sai Shri Ram Swami, data and analytics consultants at Thurgood, discuss scaling data ingestion using an ingestion framework. They highlight challenges around data quality, fragmentation, and security, and propose an ingestion framework to standardize processes, ensuring consistency and efficiency. The webinar explores real-world examples of ingestion frameworks implemented for clients in industries like consumer goods and pharmaceuticals, showing how these frameworks enhance data governance, scalability, and ease of integration with new data sources. Key benefits include improved data quality, reduced development effort, and better security and compliance.
Takeaways
- 😀 Data ingestion frameworks streamline the process of ingesting data into an organization by standardizing and structuring the approach.
- 😀 Without a proper data ingestion framework, challenges like inconsistent data quality, fragmentation, and inefficient pipeline development arise.
- 😀 Standardizing data ingestion processes ensures consistency, reduces development overhead, and promotes the reuse of code.
- 😀 Metadata plays a crucial role in driving data ingestion functionality and can simplify the process of adding new data sources without the need for extensive code changes.
- 😀 A strong focus on data governance ensures that data ingestion adheres to proper security, compliance, and privacy standards, minimizing vulnerabilities.
- 😀 Data lineage tracking is essential for understanding how data flows through the system, identifying any transformations, and ensuring traceability.
- 😀 Data quality assurance tools like validation checks, error handling, and auditing are vital to ensure accurate and reliable data ingestion.
- 😀 Data ingestion frameworks are scalable, allowing for seamless integration of new data sources, markets, and feeds without significant additional effort.
- 😀 Ingestion frameworks reduce the effort of adding new data sources by applying generic rules and allowing customization only where necessary, like retailer-specific transformations.
- 😀 In real-world implementations, like for a consumer goods company and a pharmaceutical company, metadata-driven ingestion frameworks have greatly reduced complexity and development time.
- 😀 A data ingestion framework enables schema evolution, metadata harvesting, failure handling, and dependency management, all of which contribute to more robust, scalable data pipelines.
Q & A
What is the primary focus of the webinar discussed in the script?
-The primary focus of the webinar is scaling data ingestion using an ingestion framework. It covers the challenges faced with data ingestion, how an ingestion framework can address those challenges, and real-world examples of implementations at customers.
What are some of the common data ingestion challenges mentioned in the webinar?
-The common data ingestion challenges include inconsistent data quality, ad-hoc ingestion practices leading to fragmentation, non-standardized development processes increasing maintenance efforts, and the lack of security and compliance measures that can cause vulnerabilities.
How does the ingestion framework help mitigate data ingestion challenges?
-The ingestion framework helps by standardizing processes, reducing development overhead, incorporating metadata to drive functionality, ensuring data governance and compliance, and enabling easy scalability for new data sources or markets.
What are the key benefits of using a data ingestion framework?
-The key benefits include establishing consistency and uniformity in data quality, reducing development and maintenance efforts, ensuring compliance and data governance, facilitating scalability, and allowing new data sources to be onboarded with minimal effort.
What role does metadata play in the ingestion framework?
-Metadata plays a crucial role in driving the functionality of the system. It helps control the data flow, defines how each file is interpreted, and ensures uniformity in data ingestion across different sources. Metadata also simplifies the onboarding of new markets or data sources by updating configuration files instead of changing the code.
What is data lineage, and why is it important in the context of data ingestion?
-Data lineage refers to the tracking of data's journey from its source to its destination, including any transformations it undergoes. It is important because it ensures transparency, accountability, and helps identify issues with data integrity or quality.
Can you explain the concept of a three-layered architecture in a data lake?
-A three-layered architecture in a data lake includes the bronze layer (L0), the silver layer (L1), and the gold layer (L1+). The bronze layer stores raw, untransformed data; the silver layer handles transformations and harmonization; and the gold layer is used for aggregations and preparing data for reporting.
What is the purpose of the L1+ layer in the ingestion framework discussed?
-The L1+ layer is responsible for further transforming and curating data for reporting purposes, including data aggregation and roll-ups. It standardizes data across different sources while allowing for retailer-specific transformations in the L1 layer.
How does the ingestion framework reduce development efforts for new data sources?
-The ingestion framework reduces development efforts by allowing new data sources to be integrated with minimal changes. Once the framework is in place, adding new data feeds, markets, or retailers involves simply configuring metadata or updating configuration files rather than redesigning the entire ingestion flow.
What security measures are mentioned in the webinar that are part of the ingestion framework?
-The ingestion framework includes security measures such as data encryption, access control, anonymization of sensitive data, and ensuring compliance with legal requirements to protect data from unauthorized access and mitigate reputational risks.
Outlines
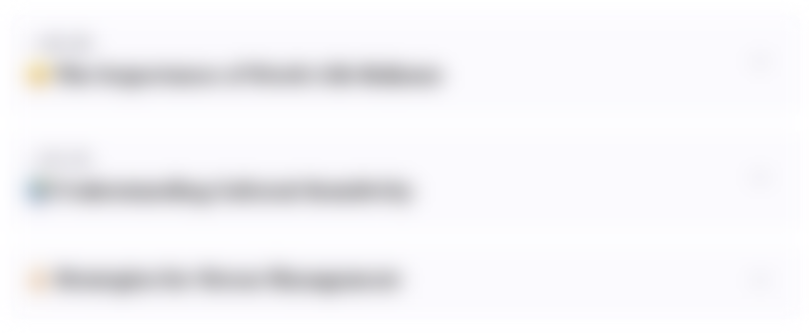
此内容仅限付费用户访问。 请升级后访问。
立即升级Mindmap
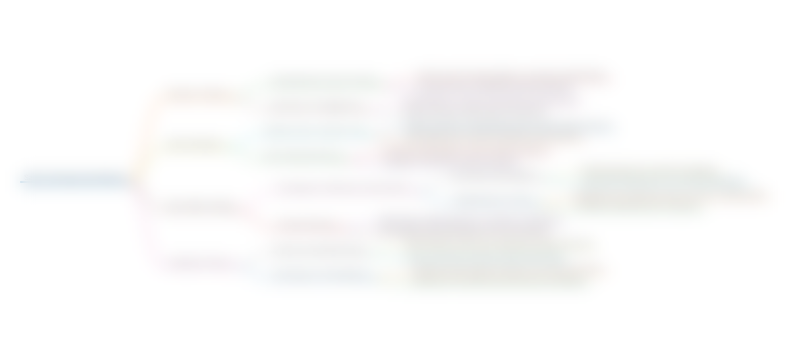
此内容仅限付费用户访问。 请升级后访问。
立即升级Keywords
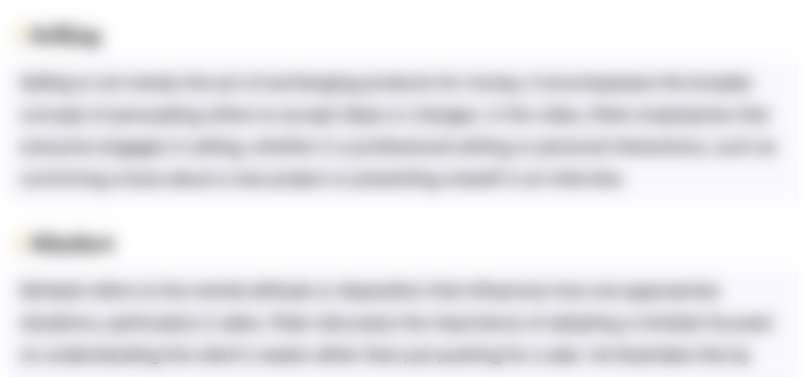
此内容仅限付费用户访问。 请升级后访问。
立即升级Highlights
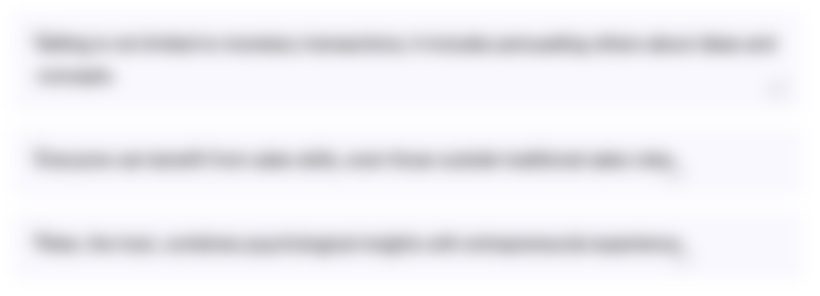
此内容仅限付费用户访问。 请升级后访问。
立即升级Transcripts
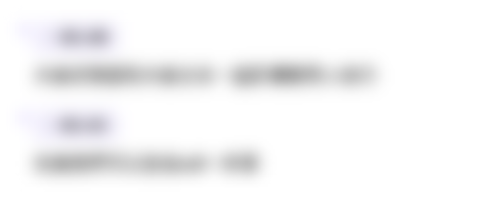
此内容仅限付费用户访问。 请升级后访问。
立即升级浏览更多相关视频
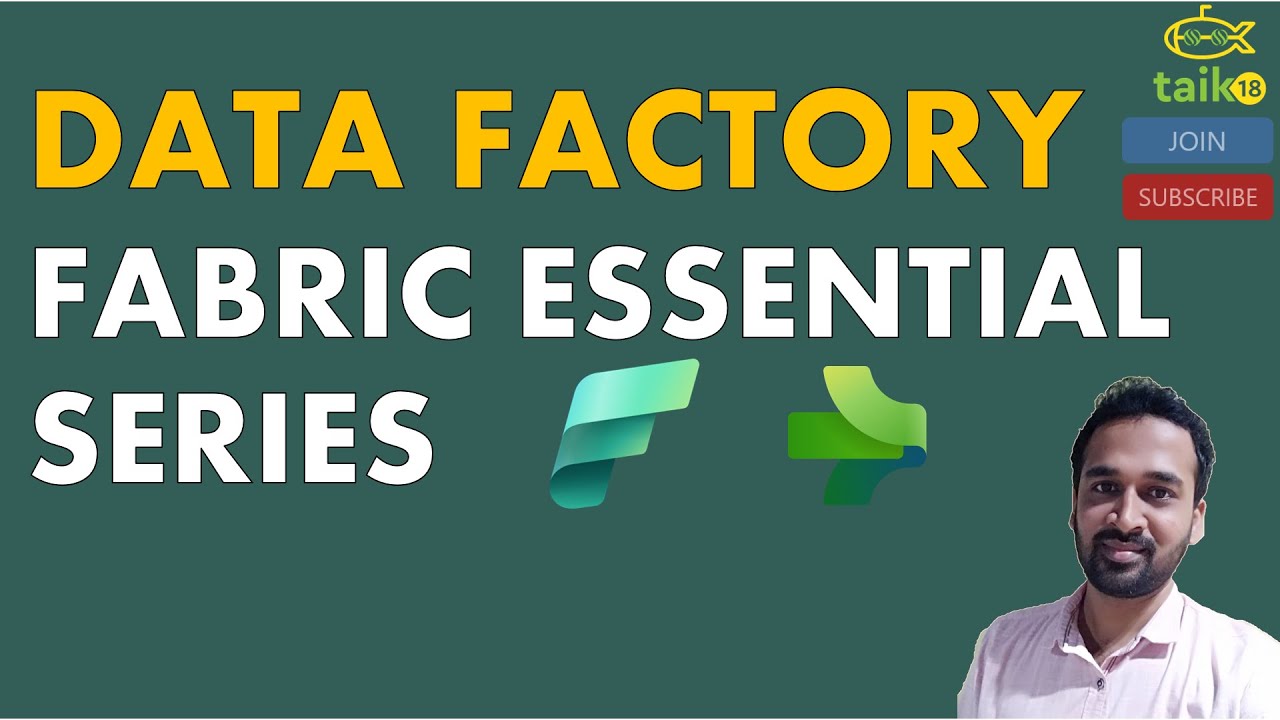
Microsoft Fabric Data Factory compared to Azure Data Factory by Taik18
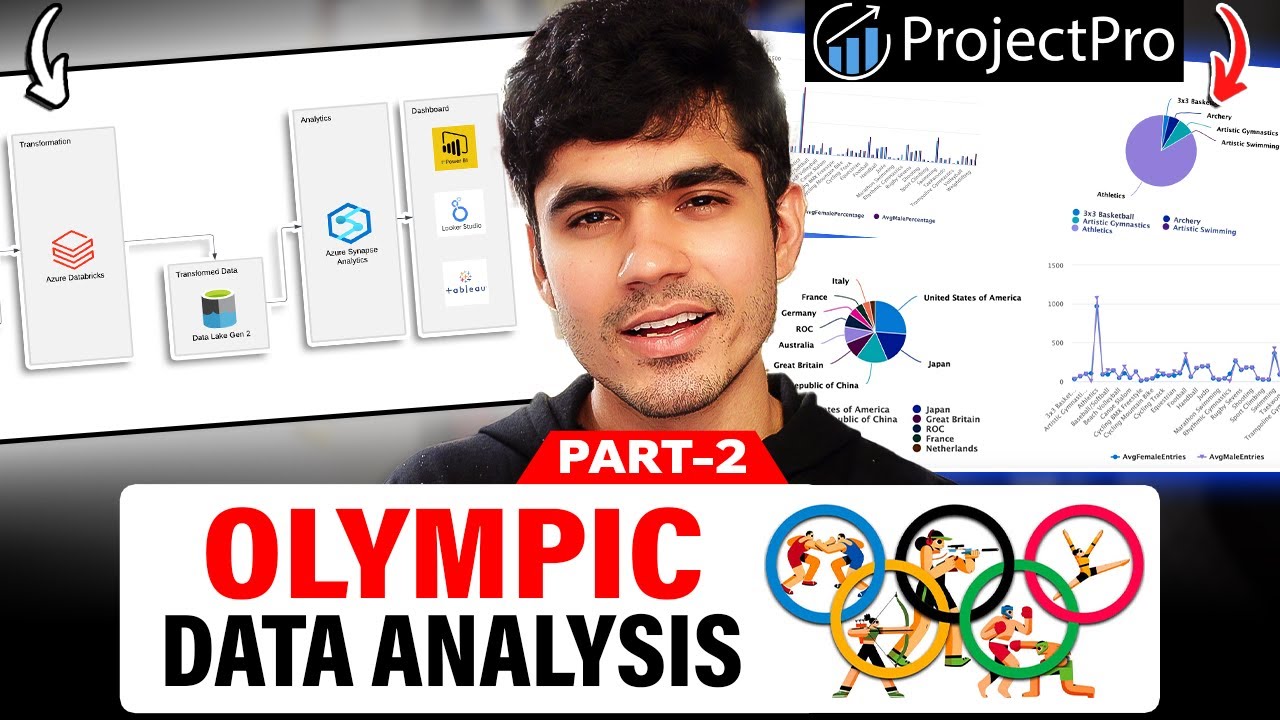
Olympic Data Analytics | Azure End-To-End Data Engineering Project | Part 2
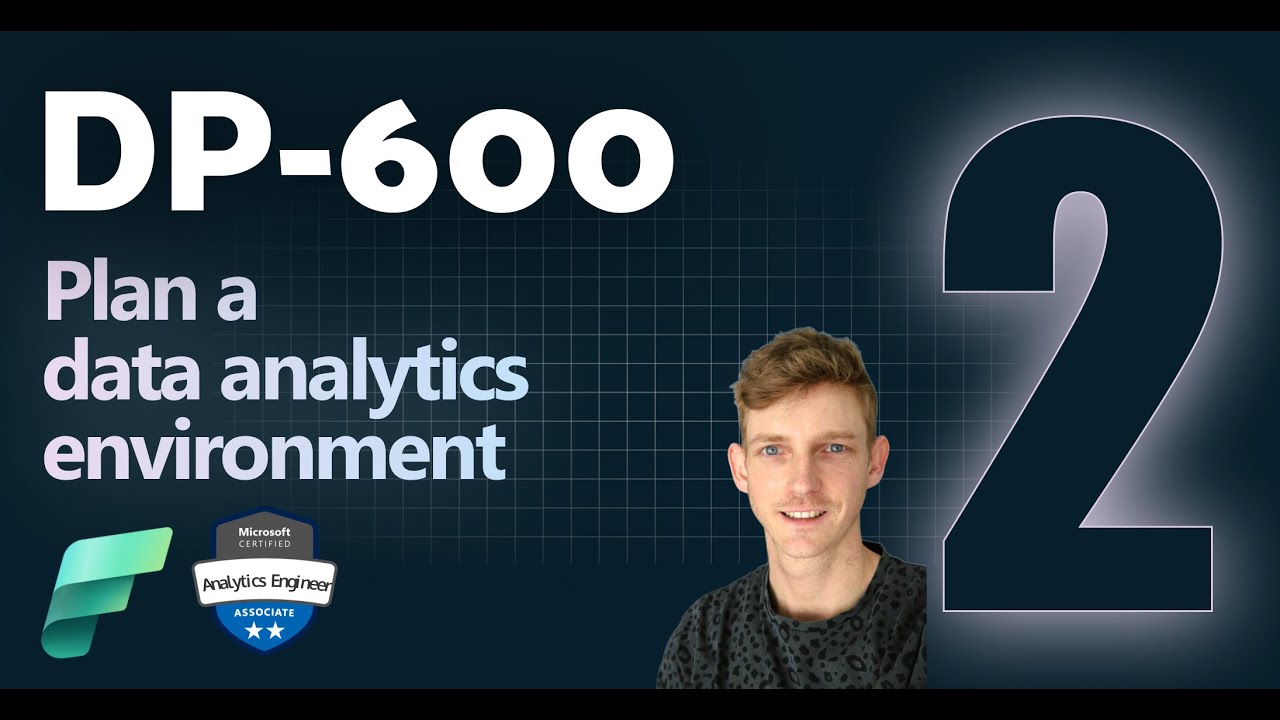
Requirements, admin portal, gateways in Microsoft Fabric | DP-600 EXAM PREP (2 of 12)
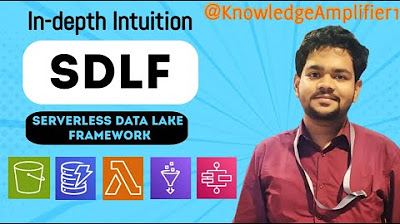
Building a Serverless Data Lake (SDLF) with AWS from scratch
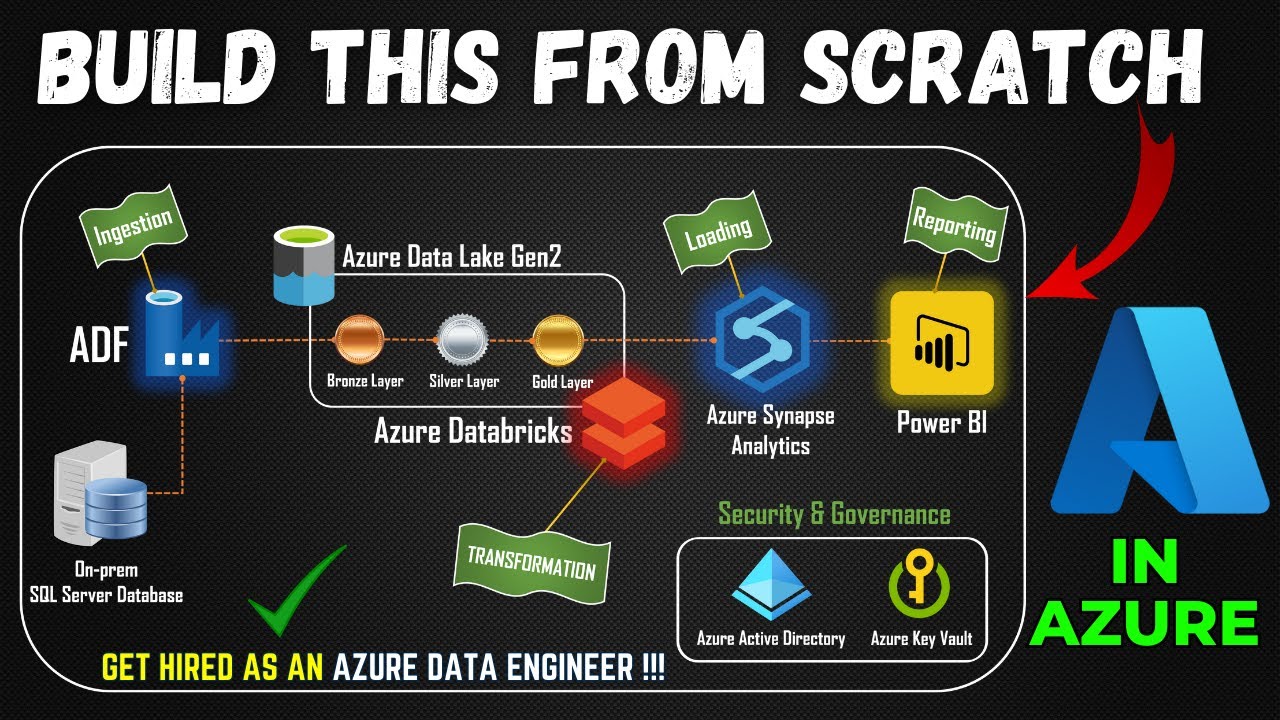
Part 1- End to End Azure Data Engineering Project | Project Overview
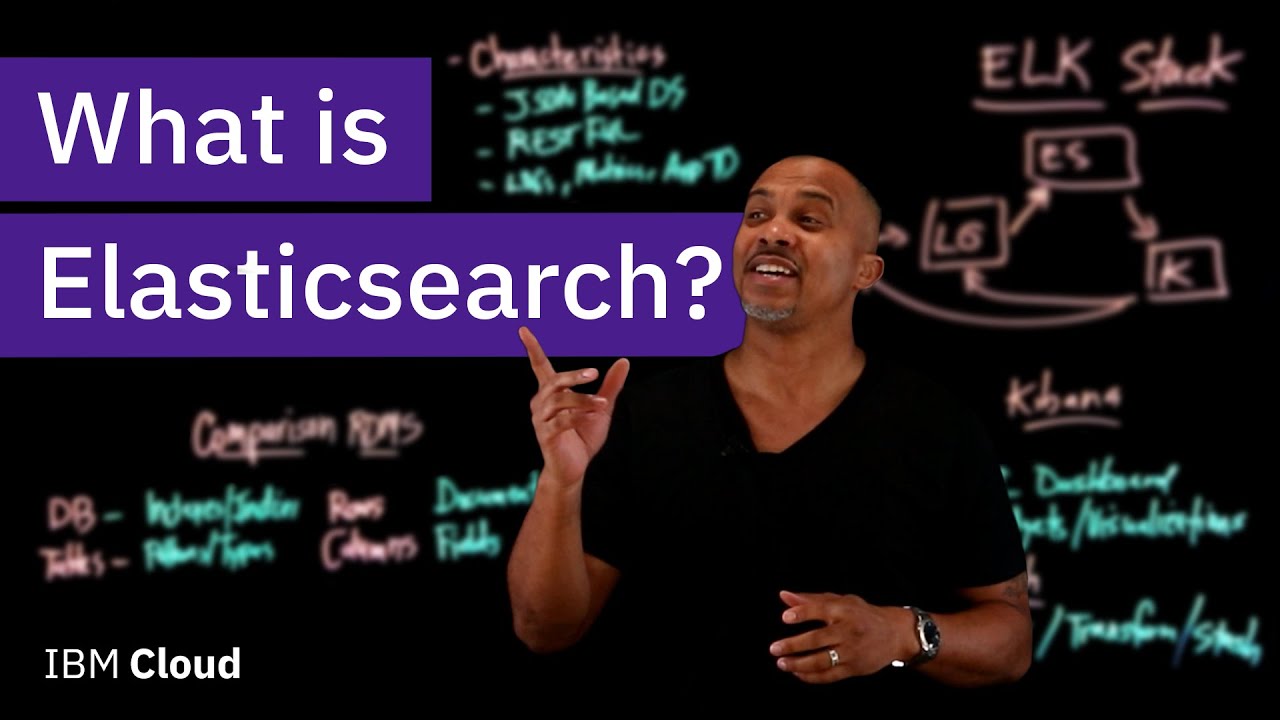
What is Elasticsearch?
5.0 / 5 (0 votes)