Lecture 9.2 _ Parameter estimation: Introduction to parameter estimation
Summary
TLDRThis lecture delves into the concept of parameter estimation in statistics, exploring the process of estimating unknown parameters from independent and identically distributed (iid) samples. It introduces several examples, including Bernoulli trials, radioactive particle emissions modeled by Poisson distribution, and noise in electronic circuits modeled by Gaussian distributions. The lecturer emphasizes the importance of understanding the underlying distributions, recognizing the role of estimators, and how they relate to the parameter of interest. Key concepts such as valid and good estimators are discussed, setting the stage for deeper exploration in subsequent lectures.
Takeaways
- 😀 Parameter estimation is a key statistical procedure used to find unknown parameters in a model using data samples.
- 😀 IID (independent and identically distributed) samples provide valuable information about the underlying distribution, helping to estimate missing parameters.
- 😀 The Bernoulli p-trials example is frequently used to introduce ideas related to parameter estimation, focusing on estimating the probability of success (p) from a set of binary outcomes.
- 😀 In a typical Bernoulli trial, you repeat an experiment several times, and the task is to estimate the unknown probability p from observed successes and failures.
- 😀 Real-world examples like radioactive particle emission and noise in electronic circuits illustrate the application of parameter estimation in different contexts.
- 😀 A Poisson distribution can model events like radioactive particle emissions, where the goal is to estimate the unknown rate parameter lambda from observed data.
- 😀 In the case of noise in electronic circuits, Gaussian distribution models random fluctuations, and parameters such as mean (mu) and variance (sigma squared) need to be estimated.
- 😀 Estimators are functions that take in sample data and output an estimate for an unknown parameter, often represented as a 'hat' (e.g., θ̂).
- 😀 The key distinction between a parameter (like θ) and an estimator (like θ̂) is that the parameter is fixed, whereas the estimator is a random variable with its own distribution.
- 😀 Good estimators aim to concentrate values around the true parameter and minimize deviation, but their performance depends on their design and the sample data.
- 😀 The design of estimators and their evaluation based on their distribution is a central topic in parameter estimation, and their quality can be assessed using statistical metrics.
Q & A
What is the primary focus of this lecture?
-The primary focus of the lecture is on parameter estimation, a statistical procedure used to estimate unknown parameters of a distribution based on independent and identically distributed (iid) samples.
How does parameter estimation fit into a larger statistical problem?
-Parameter estimation is a smaller procedure within a larger statistical problem. It involves estimating a missing parameter in a model using available data samples, often after the problem has been broken down into manageable pieces.
What is a typical example used to illustrate parameter estimation?
-A typical example used to illustrate parameter estimation is the Bernoulli p-trials, where the goal is to estimate the probability of success (p) in a series of independent and identical Bernoulli trials based on sample data.
What are Bernoulli p-trials, and how are they used in parameter estimation?
-Bernoulli p-trials are experiments with two possible outcomes: success (with probability p) or failure (with probability 1 - p). In parameter estimation, the goal is to estimate the unknown probability p using a sample of iid trials.
How does parameter estimation apply to radioactive substances and particle emissions?
-In the case of radioactive substances, such as uranium or plutonium, the number of alpha particles emitted in a given time period can be modeled using a Poisson distribution. The unknown parameter lambda of the Poisson distribution needs to be estimated based on samples of particle emissions.
What is the role of iid samples in parameter estimation?
-iid (independent and identically distributed) samples are crucial in parameter estimation as they provide reliable data that can be used to estimate unknown parameters of the underlying distribution. Each sample is assumed to be drawn from the same distribution with the same parameters.
How does the example of noise in electronic circuits relate to parameter estimation?
-In electronic circuits, noise causes random fluctuations in measured voltage or current, which can be modeled using a Gaussian (normal) distribution. Parameter estimation in this case involves estimating the mean (mu) and variance (sigma squared) of the Gaussian distribution based on iid measurements of the circuit's noise.
What distinguishes the estimator from the parameter it estimates?
-The parameter (denoted as theta) is a constant, unknown value representing the true property of the distribution, while the estimator (denoted as theta hat) is a function of the sample data and is a random variable itself. The estimator aims to provide an estimate of the parameter.
What is the significance of the hat symbol (θ̂) in statistics?
-The hat symbol (θ̂) is used to denote an estimator of the parameter θ. It indicates that the value is an estimate derived from the sample data, rather than the true, unknown value of the parameter.
What is the difference between a valid estimator and a good estimator?
-A valid estimator is any function that takes the sample data and produces a real number as an estimate for the parameter. A good estimator, however, is one that provides estimates that are statistically close to the true parameter value with high probability, and it should have desirable properties like low bias and low variance.
Outlines
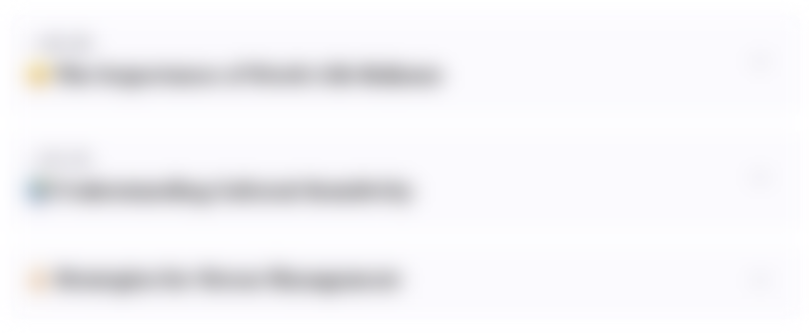
此内容仅限付费用户访问。 请升级后访问。
立即升级Mindmap
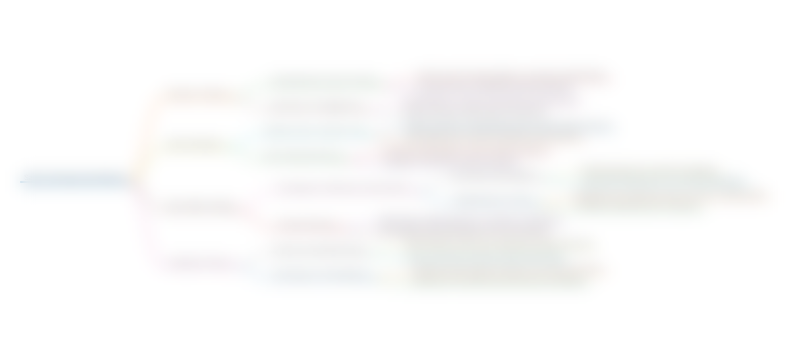
此内容仅限付费用户访问。 请升级后访问。
立即升级Keywords
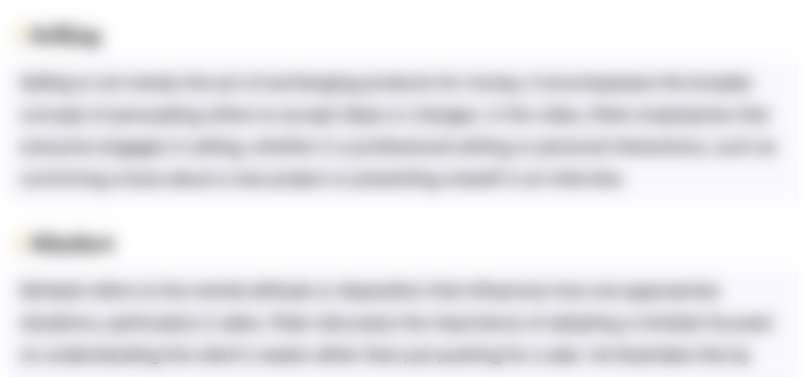
此内容仅限付费用户访问。 请升级后访问。
立即升级Highlights
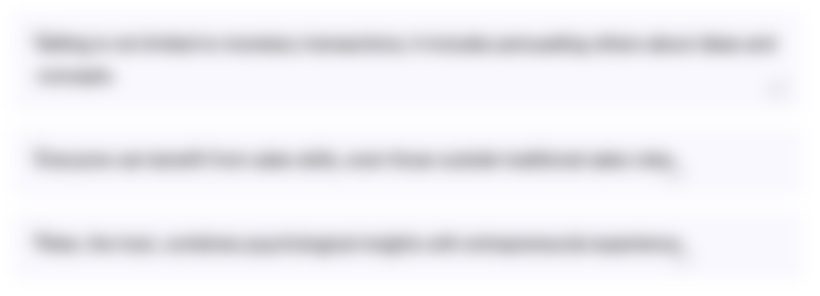
此内容仅限付费用户访问。 请升级后访问。
立即升级Transcripts
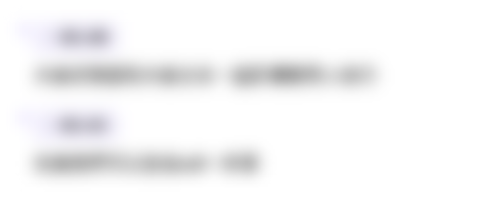
此内容仅限付费用户访问。 请升级后访问。
立即升级5.0 / 5 (0 votes)