Lecture 9.3 _ Parameter estimation: Error in estimation
Summary
TLDRThis lecture explores the concept of estimators in statistics, focusing on how errors in estimation are distributed and how they improve with an increasing number of samples. The speaker discusses the importance of controlling error through tools like the Chebyshev bound, emphasizing that estimators should reduce error variance and improve accuracy as sample sizes grow. The lecture highlights the design principles for estimators, such as ensuring the expected error approaches zero and the variance diminishes. It concludes with a call to design estimators with these principles in mind to achieve more reliable results.
Takeaways
- 😀 Estimators improve with more samples: The performance of an estimator increases as the number of samples (n) increases. More data results in better accuracy and lower error.
- 😀 Chebyshev's bound helps control error probabilities: The Chebyshev bound is a useful tool to estimate how the error probability behaves with increasing sample size.
- 😀 Expected error should approach zero: A well-designed estimator should have its expected error close to zero, ensuring that large errors occur with low probability.
- 😀 Variance of error decreases with more samples: As the sample size increases, the variance of the error should decrease, resulting in a more reliable estimator.
- 😀 Distribution of error becomes narrower with more data: With an increasing sample size, the error distribution should become more concentrated around the true value, reducing spread.
- 😀 The importance of sample size in error reduction: The error in an estimator decreases in magnitude as more independent, identically distributed samples are used.
- 😀 Estimators have random error distributions: Every estimator has an associated error, which is random and has a certain distribution, typically centered around zero.
- 😀 Probability of large errors can be controlled: By focusing on tools like Chebyshev’s inequality, it is possible to bound the probability that the error exceeds a certain threshold.
- 😀 Good design principles focus on error characteristics: The expected value and variance of the error should both be minimized in the design of good estimators.
- 😀 Larger sample sizes lead to more confidence: With enough samples, estimators can become very reliable, with the probability of large errors approaching zero as sample size increases.
Q & A
What is the primary focus of this lecture?
-The primary focus of the lecture is on the behavior of estimators, particularly how their accuracy improves with an increasing number of samples, and how tools like Chebyshev's inequality help in controlling estimation errors.
How does the number of samples affect the performance of an estimator?
-As the number of samples increases, the performance of the estimator improves. With more data, the estimator’s error becomes more predictable, and the estimate becomes more accurate.
What is the significance of Chebyshev's inequality in this context?
-Chebyshev's inequality is used to bound the probability of large errors in an estimator. It ensures that, as the number of samples increases, the probability of the error exceeding a certain threshold decreases, thus improving the reliability of the estimator.
What happens to the probability of large errors as the sample size grows?
-As the sample size increases, the probability that the error exceeds a certain threshold decreases. This is crucial for ensuring that estimators become more accurate with more data.
Why is it important to have the expected error close to zero?
-Having the expected error close to zero means that the estimator is unbiased, leading to a more reliable estimate. This reduces the likelihood of significant deviations from the true value of the parameter being estimated.
What role does variance play in the design of an estimator?
-Variance measures how spread out the errors are. A good estimator should have low variance, which indicates that the error is consistently small across different samples. Ideally, variance should decrease as the number of samples increases.
What does the term 'concentration of error' mean in the context of estimators?
-Concentration of error refers to the tendency of the error to become more tightly clustered around the true value as the sample size increases, meaning the estimator becomes more accurate and reliable with more data.
What are the key principles for designing a good estimator?
-Key principles include ensuring the expected value of the error is close to zero, minimizing the variance of the error, and making sure the probability of large errors decreases as the number of samples increases.
How can the design of an estimator be improved based on the findings from this lecture?
-The design of an estimator can be improved by focusing on reducing both the expected error and its variance. Additionally, using concentration inequalities like Chebyshev’s inequality can help ensure that errors remain small as the sample size increases.
What is the practical implication of using tools like Chebyshev's inequality in estimator design?
-The practical implication is that by applying Chebyshev's inequality or similar concentration tools, you can quantify and control the probability of large estimation errors. This gives you a more predictable and reliable estimator as the sample size grows.
Outlines
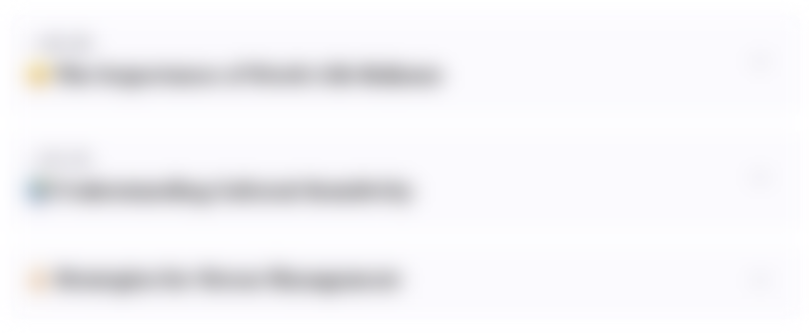
This section is available to paid users only. Please upgrade to access this part.
Upgrade NowMindmap
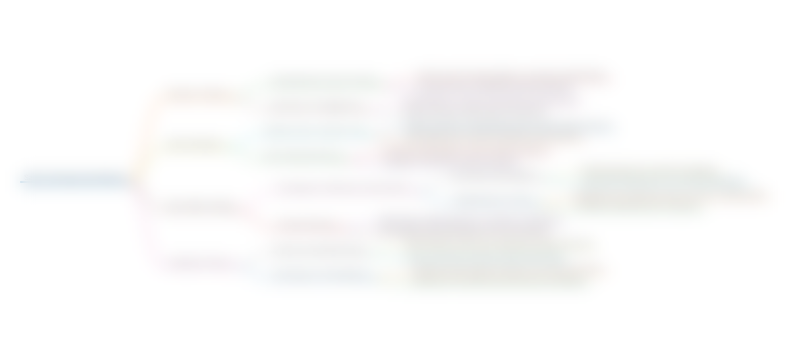
This section is available to paid users only. Please upgrade to access this part.
Upgrade NowKeywords
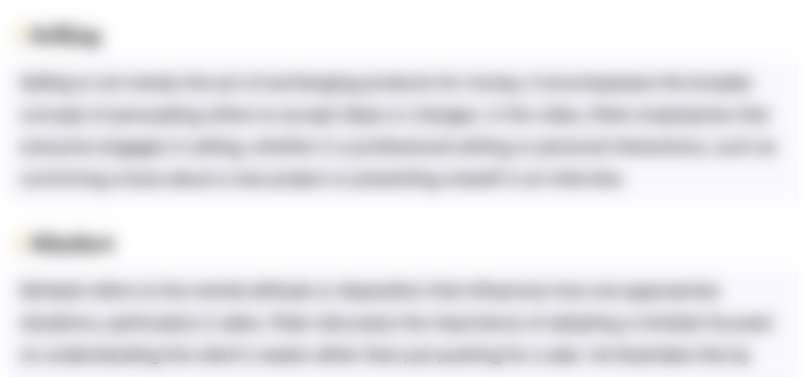
This section is available to paid users only. Please upgrade to access this part.
Upgrade NowHighlights
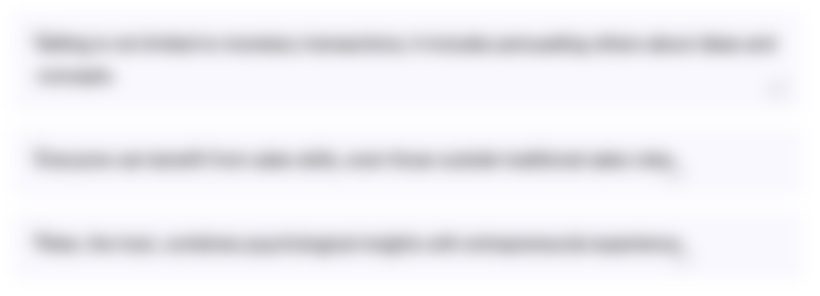
This section is available to paid users only. Please upgrade to access this part.
Upgrade NowTranscripts
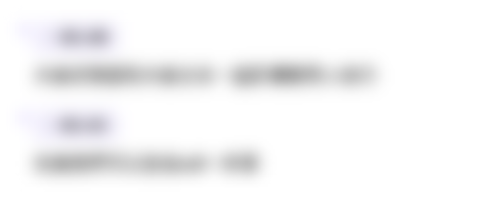
This section is available to paid users only. Please upgrade to access this part.
Upgrade Now5.0 / 5 (0 votes)