Statistik Cukup pengertian
Summary
TLDRThis video delves into the concept of *sufficient statistics* in statistics, explaining its role in parameter estimation and its application to various distributions like Bernoulli and Poisson. The instructor defines a sufficient statistic as one that retains all essential information about a distribution's parameter, simplifying data without losing crucial details. The video also introduces the idea of *minimum sufficient statistics*, which is the most compact form of a sufficient statistic. Through practical examples, viewers are guided in recognizing and confirming sufficient statistics, making complex statistical concepts more accessible.
Takeaways
- π Statistics are used to make parameter estimates for distributions, and these estimates are based on random samples.
- π A statistic is defined as a function of a random sample, and can be used to estimate a distribution parameter like the mean (XΜ) for a normal distribution.
- π The concept of a 'sufficient statistic' is introduced, which means a statistic provides all the information needed to estimate a parameter, without any additional information from the sample.
- π A sufficient statistic for a distribution is one where the conditional probability of the sample given the statistic does not depend on the parameter being estimated.
- π In the case of a Bernoulli distribution, the sum of the sample (S = X1 + X2 + X3) is a sufficient statistic for the parameter ΞΈ (probability of success).
- π The script explains how to calculate conditional probabilities to demonstrate sufficiency, showing that knowing the sum (S) of a sample reveals all relevant information about the parameter ΞΈ in a Bernoulli distribution.
- π For a distribution with more than one parameter (like the normal distribution), a sufficient statistic for all parameters can still be defined.
- π The concept of 'joint sufficiency' is introduced when there are multiple parameters. This ensures that the statistic provides all the information needed for each parameter involved.
- π To check if a statistic is sufficient, we must show that the conditional probability of the sample, given the statistic, is independent of the parameters being estimated.
- π A 'minimal sufficient statistic' is the smallest statistic that still captures all the information needed to estimate parameters efficiently, sometimes having fewer dimensions than the original sample.
Q & A
What is the primary topic discussed in this video?
-The primary topic of the video is 'sufficient statistics', focusing on the definition, properties, and examples of sufficient statistics in statistics.
What is meant by 'sufficient statistics' in the context of this video?
-Sufficient statistics are defined as statistics that provide enough information to estimate a parameter of a distribution, so that no additional information about the parameter can be obtained from other statistics once the sufficient statistic is known.
Can you explain how 'sufficient statistics' work with the Bernoulli distribution in this video?
-In the case of the Bernoulli distribution, the sufficient statistic is the sum of the outcomes (0s and 1s), which helps estimate the parameter, Theta. The likelihood function is derived from the joint probability of the outcomes, and the sufficient statistic is the sum of these values, which retains all the necessary information about Theta.
What is the mathematical condition for a statistic to be sufficient?
-A statistic is considered sufficient for a parameter if the conditional probability (likelihood) of the data given the statistic does not depend on the parameter. In other words, once the statistic is known, no further information about the parameter can be gained from the data.
How does the video define 'sufficient statistic' for the Bernoulli distribution?
-For the Bernoulli distribution, the sufficient statistic is the sum of the observations (X1, X2, ..., X3) because it encapsulates all the information about the parameter, Theta, without requiring knowledge of the individual observations.
What is the relationship between the likelihood function and sufficient statistics in the video?
-The likelihood function is the key to determining sufficient statistics. If the likelihood function of the data, when conditioned on the sufficient statistic, does not depend on the parameter being estimated, then the statistic is sufficient.
What is the role of the 'conditional probability' in determining if a statistic is sufficient?
-The conditional probability, in the context of sufficient statistics, helps determine whether the statistic contains all the information about the parameter. If the conditional probability of the data given the statistic is independent of the parameter, then the statistic is sufficient.
How does the video demonstrate the concept of sufficient statistics using examples of random variables?
-The video provides examples like the Bernoulli and Poisson distributions, showing how the sufficient statistics (such as the sum of the outcomes) encapsulate all the necessary information about the parameter, and how the likelihood functions are derived for these distributions.
What is a 'minimum sufficient statistic' as mentioned in the video?
-A minimum sufficient statistic is a sufficient statistic that has the smallest dimension, meaning it reduces the sample data to the least amount of information needed to estimate the parameter, while still being sufficient.
How does the video explain the process of determining whether a statistic is sufficient for more than one parameter?
-For cases with more than one parameter, the video outlines the need to show that the conditional probability of the data given the statistic does not depend on the parameters. This ensures the statistic remains sufficient for multi-parameter distributions.
Outlines
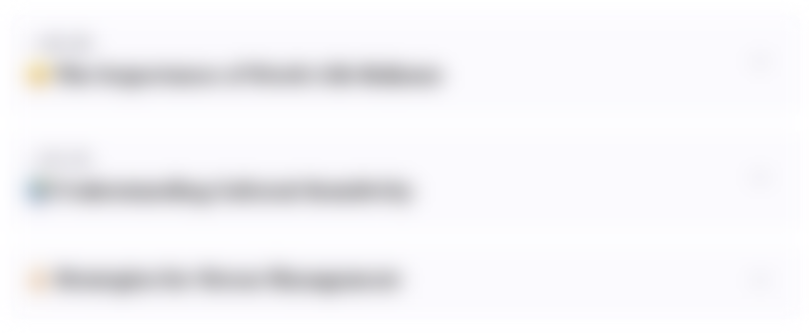
This section is available to paid users only. Please upgrade to access this part.
Upgrade NowMindmap
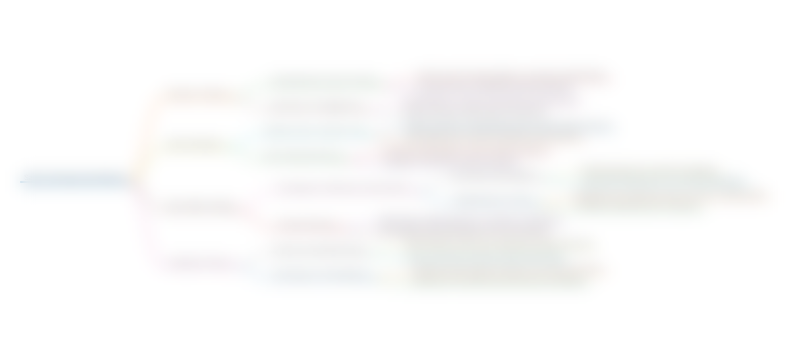
This section is available to paid users only. Please upgrade to access this part.
Upgrade NowKeywords
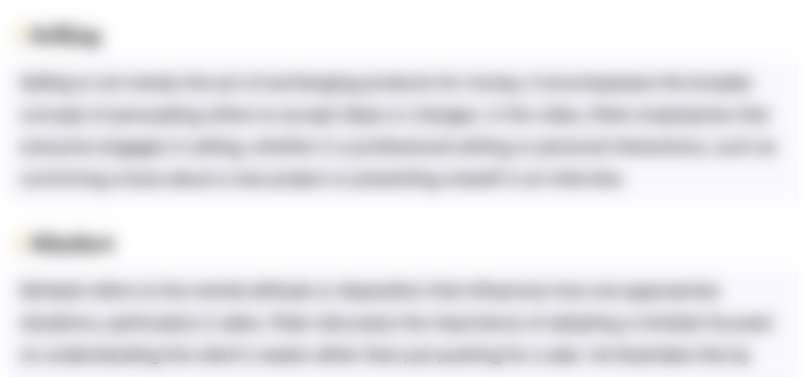
This section is available to paid users only. Please upgrade to access this part.
Upgrade NowHighlights
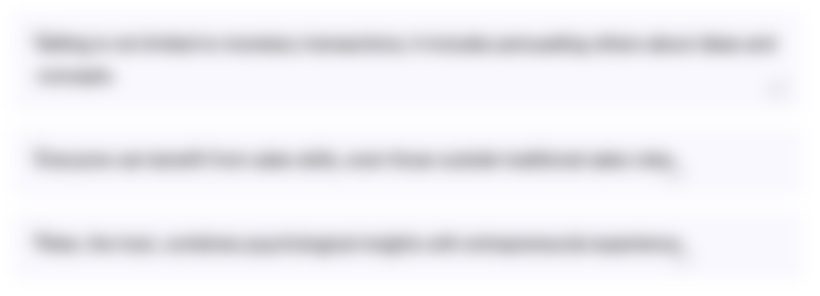
This section is available to paid users only. Please upgrade to access this part.
Upgrade NowTranscripts
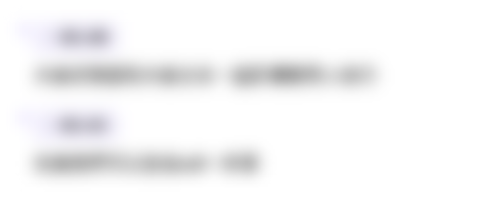
This section is available to paid users only. Please upgrade to access this part.
Upgrade Now5.0 / 5 (0 votes)