Hypothesis Testing (Binomial) 2 • Null and Alternative Hypotheses • Stats1 Ex7A • 🤖
Summary
TLDRThis video script delves into hypothesis testing, explaining how to formulate null and alternative hypotheses using real-world examples. The first example focuses on an election candidate's support, testing whether the candidate's claim of 40% support is accurate. The second example explores student lateness, where a school tests if the percentage of late students exceeds the national average of 5%. Through these cases, the script highlights the importance of selecting the right test statistic, formulating appropriate hypotheses, and determining when to reject the null hypothesis based on significance levels. It's an insightful guide to understanding the fundamentals of hypothesis testing in statistics.
Takeaways
- 😀 The null hypothesis (H₀) is what we assume to be true, and the alternative hypothesis (H₁) is what we test for based on observed data.
- 😀 A test statistic is used to summarize the data from the sample, helping to decide whether to reject the null hypothesis.
- 😀 For binomial tests, the test statistic is the count of successes in the sample (e.g., number of supporters or number of late students).
- 😀 In hypothesis testing, a significance level (e.g., 5% or 10%) helps determine the threshold for rejecting the null hypothesis.
- 😀 If the probability of the observed test statistic (based on the null hypothesis) is lower than the significance level, we reject H₀ and accept H₁.
- 😀 In the election case, the researcher suspects the candidate is overestimating her support, which leads to a one-tailed hypothesis (p < 0.4).
- 😀 In the school lateness example, the hypothesis test is one-tailed (p > 0.05), as the teacher suspects that the lateness rate is higher than the national average.
- 😀 The condition for rejecting the null hypothesis is when the probability of the observed data is extremely low (below the chosen significance level).
- 😀 The number of late students observed (6 out of 40) in the school lateness case leads to a rejection of the null hypothesis, indicating a possible attendance problem.
- 😀 To calculate the probability of observing 6 or more late students, use the complementary probability (1 - P(x ≤ 5)) to find the likelihood of more extreme results.
- 😀 Hypothesis testing provides a structured way to evaluate whether the data supports the assumption (null hypothesis) or suggests a different scenario (alternative hypothesis).
Q & A
What is the main goal of hypothesis testing in the examples provided?
-The main goal of hypothesis testing in the examples is to determine whether the observed data (e.g., the number of people supporting a candidate or the number of students arriving late) significantly contradicts the claims made by the null hypothesis, suggesting the alternative hypothesis might be true.
How is the test statistic defined in both examples?
-In both examples, the test statistic is the number of successes observed in the sample. In the first example, it’s the number of people supporting the candidate, and in the second, it’s the number of students who are late.
What are the null and alternative hypotheses in the election candidate example?
-In the election candidate example, the null hypothesis (H0) is that the candidate has 40% support, i.e., p = 0.4. The alternative hypothesis (H1) is that the candidate's actual support is less than 40%, i.e., p < 0.4.
How does the researcher determine when to reject the null hypothesis in the election example?
-The null hypothesis is rejected if the probability of observing 3 or fewer supporters (out of 20 surveyed) is less than 5%, indicating that the observed support is too low to be consistent with the candidate's claim of 40% support.
What is the significance level used in the school attendance example, and how does it affect the hypothesis test?
-The significance level used in the school attendance example is 10%. This means that if the probability of observing 6 or more late students (out of 40) is less than 10%, the null hypothesis is rejected, indicating a potential attendance problem at the school.
Why does the researcher in the school attendance example look at '6 or more' late students?
-The researcher looks at '6 or more' late students because, based on the observed data, if 6 or more students are late, the probability is low enough to reject the null hypothesis and conclude that the school has a higher rate of lateness than the national average of 5%.
What is the role of the null hypothesis in both examples?
-The null hypothesis in both examples represents the assumption that there is no significant difference or effect from the expected value: 40% support in the election case and 5% lateness in the school case. It serves as a baseline against which the observed data is compared.
What would it mean to reject the null hypothesis in these scenarios?
-Rejecting the null hypothesis means that the observed data is unlikely to occur if the null hypothesis were true, leading to the acceptance of the alternative hypothesis. In the election example, it would mean the candidate has less than 40% support, and in the school example, it would indicate a higher than expected lateness rate.
How does the significance level affect the rejection of the null hypothesis?
-The significance level determines the threshold for rejecting the null hypothesis. If the probability of the observed data is lower than the significance level (e.g., 5% or 10%), the null hypothesis is rejected. In both examples, if the calculated probability is below the significance level, the null hypothesis is deemed unlikely, and the alternative hypothesis is considered more plausible.
Why does the researcher use the binomial distribution in these examples?
-The binomial distribution is used because the outcomes are binary (either a person supports the candidate or not, and either a student is late or not). The binomial distribution models the probability of observing a specific number of successes in a fixed number of independent trials, which is appropriate for both examples.
Outlines
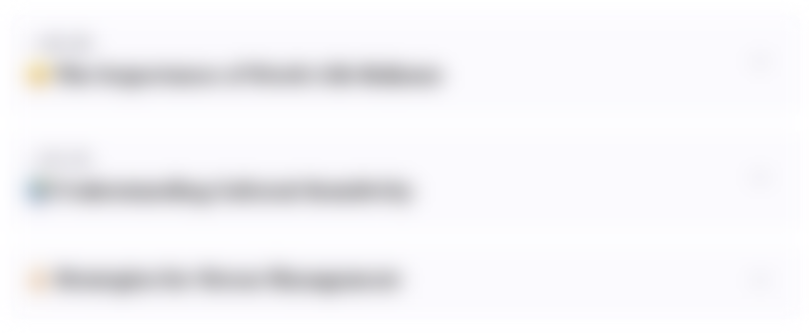
此内容仅限付费用户访问。 请升级后访问。
立即升级Mindmap
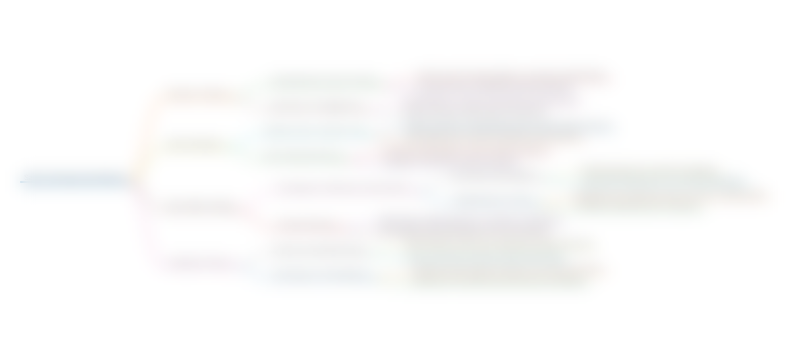
此内容仅限付费用户访问。 请升级后访问。
立即升级Keywords
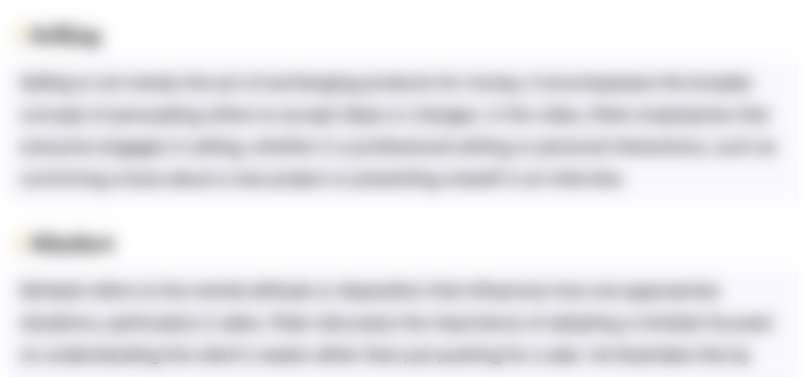
此内容仅限付费用户访问。 请升级后访问。
立即升级Highlights
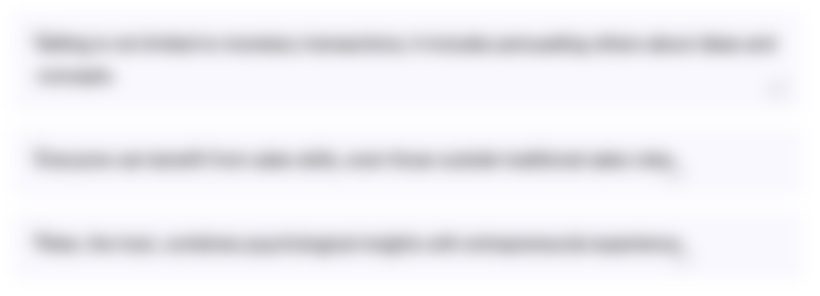
此内容仅限付费用户访问。 请升级后访问。
立即升级Transcripts
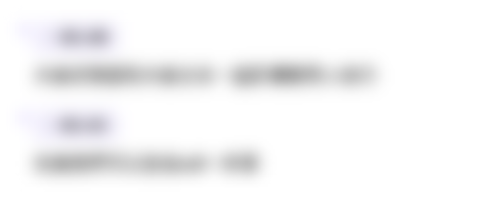
此内容仅限付费用户访问。 请升级后访问。
立即升级浏览更多相关视频
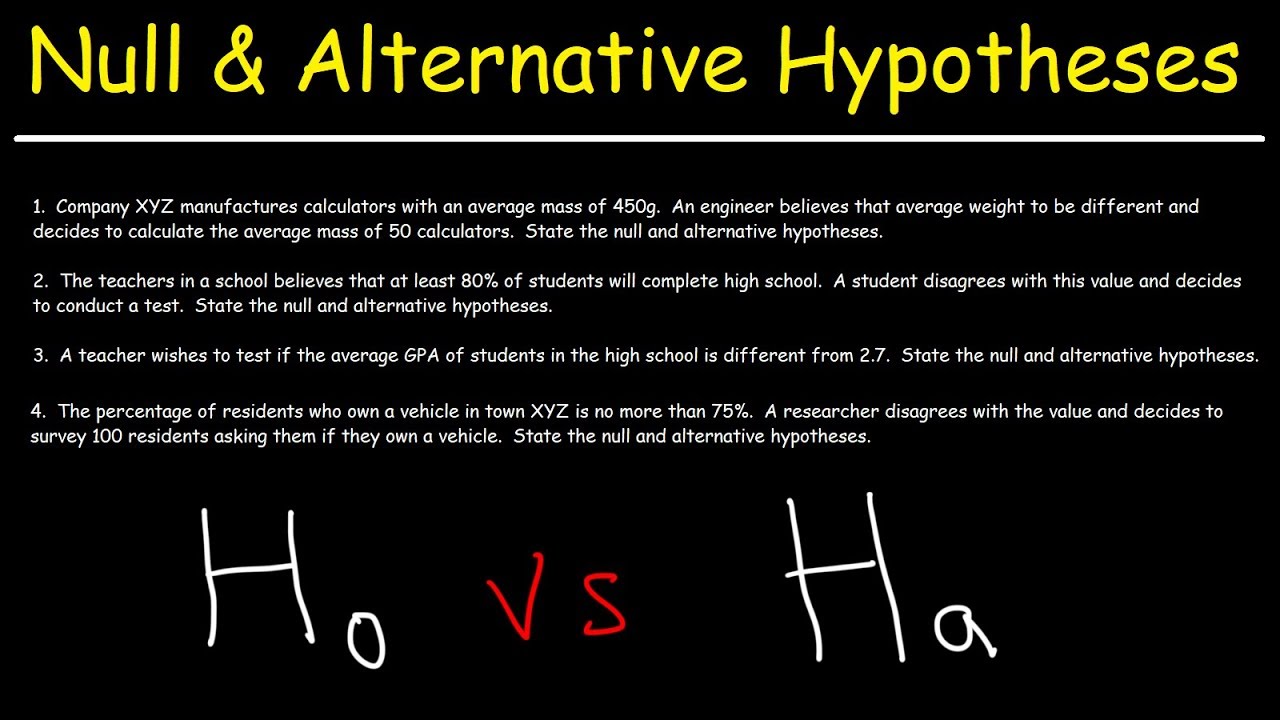
Hypothesis Testing - Null and Alternative Hypotheses
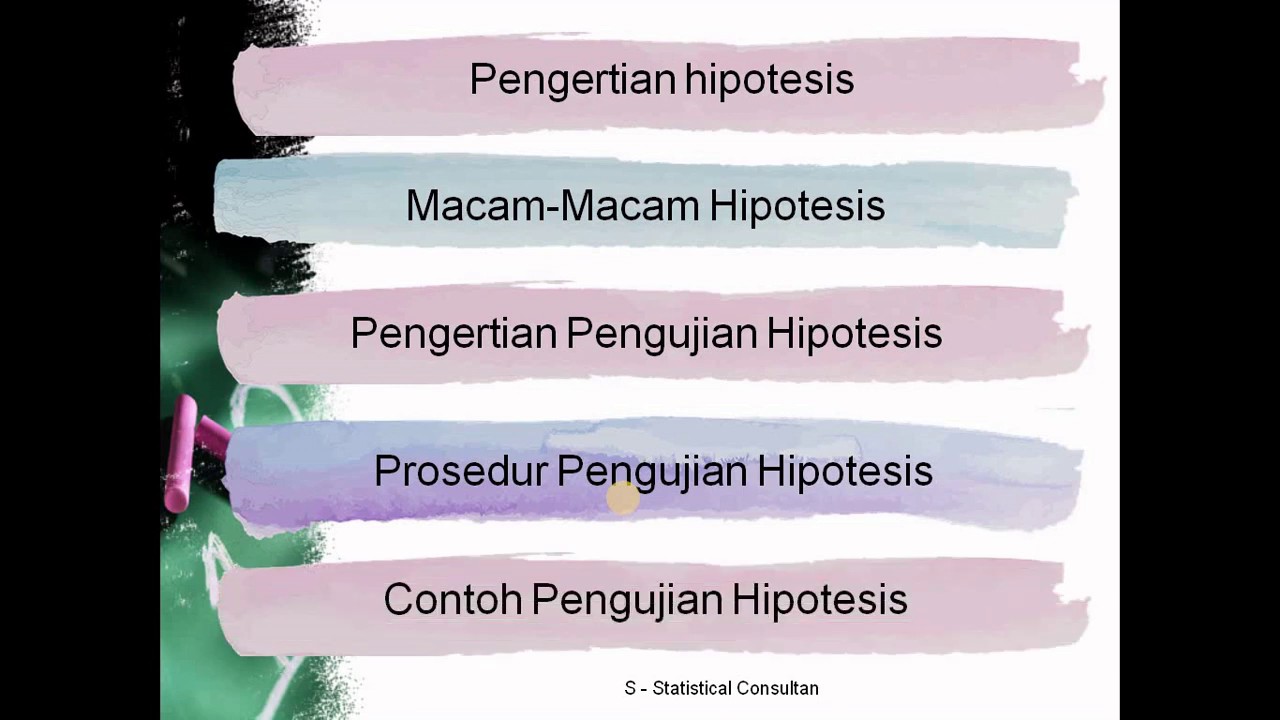
Pengujian Hipotesis (Seri MK Statistika)
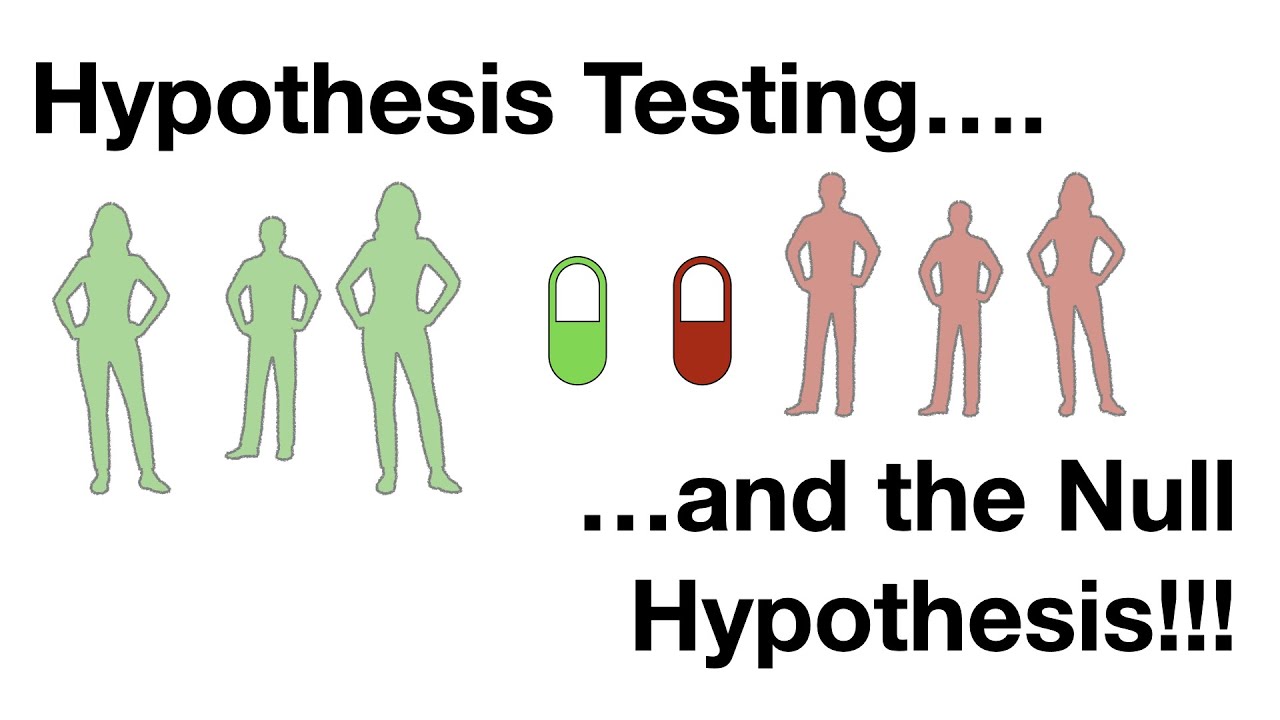
Hypothesis Testing and The Null Hypothesis, Clearly Explained!!!

Stats: Hypothesis Testing (P-value Method)
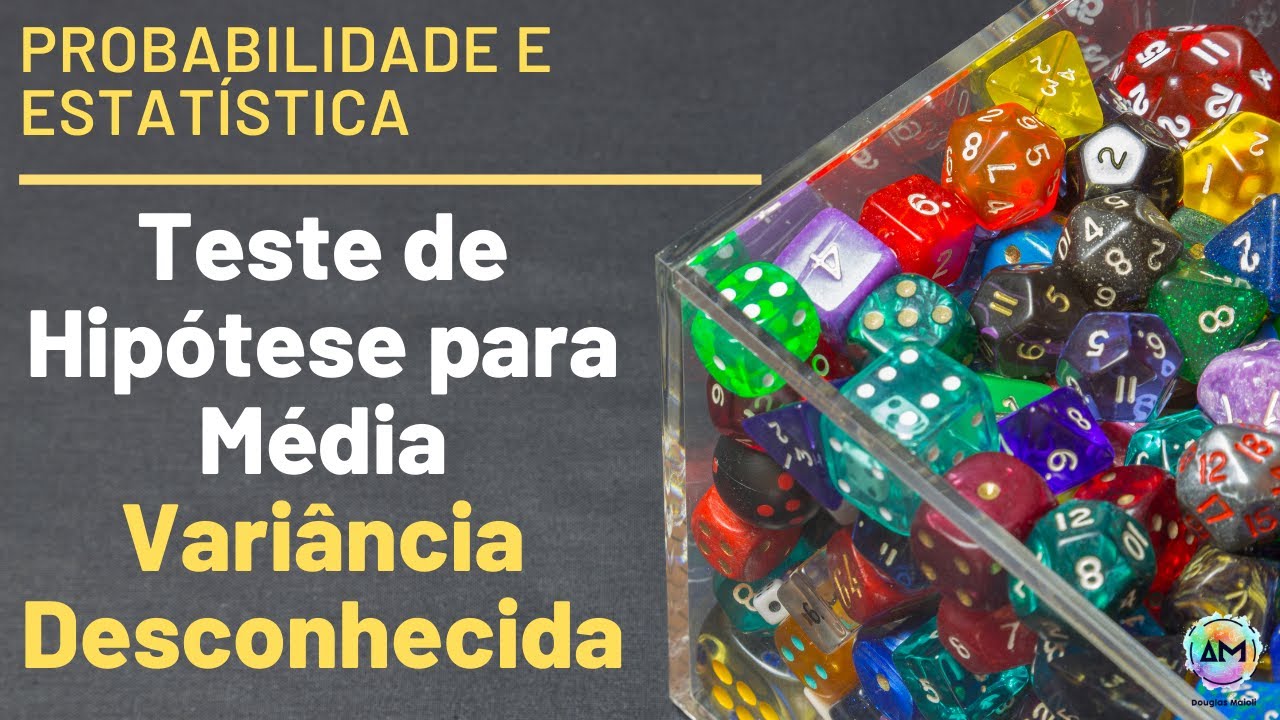
Inferência Aula 32 - Teste de Hipótese para Média com Variância Desconhecida
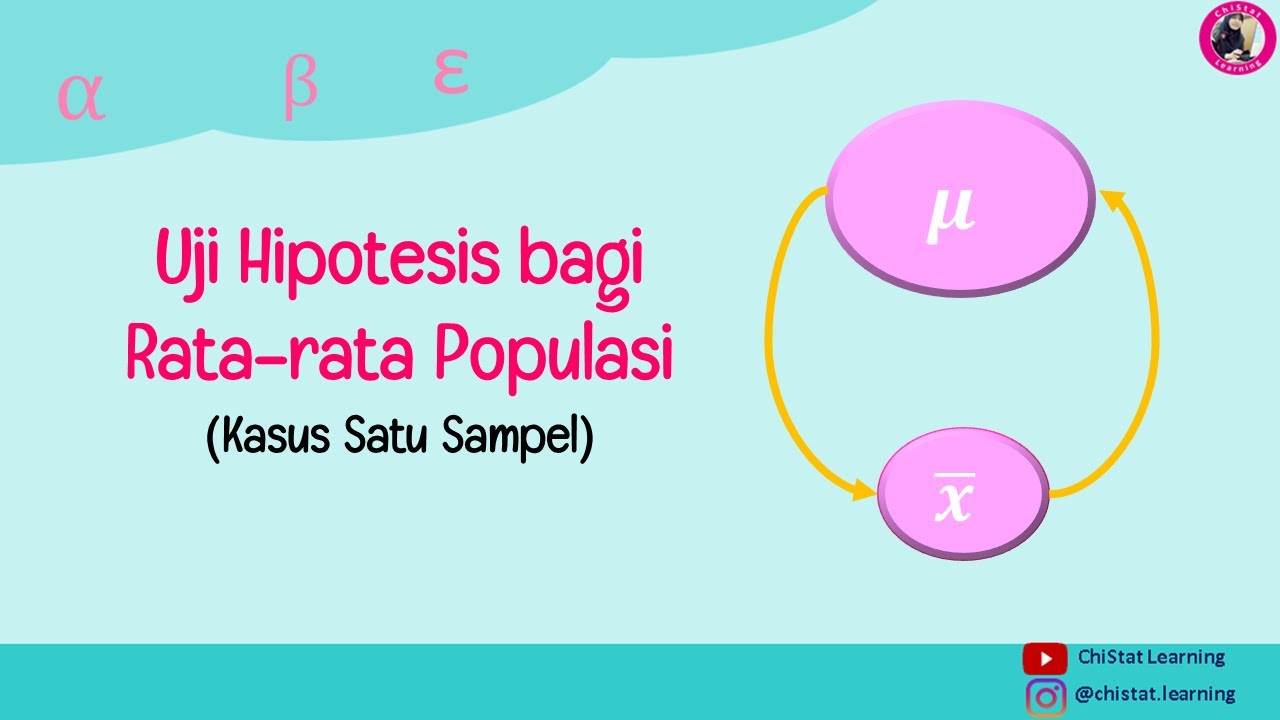
Metode Statistika | Uji Hipotesis bagi Rata-rata Satu Populasi
5.0 / 5 (0 votes)