Hypothesis testing, Business Statistics and Analytics, business statistics and analytics aktu notes
Summary
TLDRThis video script provides a detailed explanation of hypothesis testing in statistics. It introduces the concept of making assumptions about population parameters, like guessing that students who have breakfast perform better in exams than those who don’t. The script explains key concepts such as null and alternative hypotheses, types of errors, and levels of significance. Real-world examples, such as a judge’s initial assumption of innocence, are used to clarify the testing process. The script also covers directional vs. non-directional hypotheses and the steps involved in hypothesis testing, helping viewers understand the process and its applications.
Takeaways
- 😀 Hypothesis means making an educated guess or assumption about a population parameter.
- 😀 Hypothesis testing is a process where we test whether the assumption we made is valid based on sample data.
- 😀 Null hypothesis (H0) is initially assumed to be true, and alternative hypothesis (H1) challenges this assumption.
- 😀 In hypothesis testing, we draw a sample from the population and analyze it to validate or reject the initial hypothesis.
- 😀 A null hypothesis suggests there is no difference or effect in the population parameter being studied.
- 😀 The alternative hypothesis is the opposite of the null hypothesis, suggesting that a difference or effect exists.
- 😀 The level of significance (α) is the probability of rejecting a true null hypothesis, often set to values like 0.05 or 0.01.
- 😀 Type I error occurs when the null hypothesis is incorrectly rejected, while Type II error happens when the null hypothesis is incorrectly accepted.
- 😀 The steps in hypothesis testing include stating the null and alternative hypotheses, determining the level of significance, and selecting the appropriate statistical test.
- 😀 Hypothesis testing involves the use of critical regions, which are ranges of values that lead to rejecting the null hypothesis, and selecting the suitable statistical test (e.g., t-test, z-test).
Q & A
What is a hypothesis in statistics?
-A hypothesis in statistics is an assumption or a guess about the value of a population parameter. It's essentially a proposed explanation that can be tested using statistical methods.
What is hypothesis testing?
-Hypothesis testing is the process of testing whether a hypothesis about a population parameter is valid, based on sample data. It helps determine if an assumption made about a population is correct or not.
How does hypothesis testing work?
-In hypothesis testing, you first make an assumption about the population parameter. Then, using sample data and statistical methods, you test whether this assumption is true or false. The result helps determine if your initial hypothesis was correct.
What is the null hypothesis?
-The null hypothesis (denoted as H0) is an initial assumption that suggests there is no effect or difference between the sample statistic and the population parameter. It's considered true unless evidence proves otherwise.
What is the alternative hypothesis?
-The alternative hypothesis (denoted as H1) is the opposite of the null hypothesis. It suggests that there is a difference or effect, meaning the population parameter does not equal the value stated in the null hypothesis.
How do you differentiate between null and alternative hypotheses?
-The null hypothesis suggests no difference or effect, while the alternative hypothesis suggests a difference or effect. For example, if the null hypothesis says a mean equals 0, the alternative could say the mean is not equal to 0.
What is a directional hypothesis?
-A directional hypothesis specifies a direction of the difference or relationship. For example, it might suggest that one group has a higher or lower mean than another group, unlike a non-directional hypothesis which simply suggests a difference exists.
What is a non-directional hypothesis?
-A non-directional hypothesis suggests that there is a difference between groups but does not specify whether one is greater or smaller. For example, it might claim that the mean of two groups are different without stating which is larger.
What is the level of significance in hypothesis testing?
-The level of significance (denoted as alpha) represents the probability of rejecting the null hypothesis when it is actually true. It's the threshold below which the null hypothesis is rejected, commonly set at 0.05.
What are Type I and Type II errors in hypothesis testing?
-A Type I error occurs when the null hypothesis is rejected even though it is true, while a Type II error happens when the null hypothesis is accepted even though it is false. Type I is denoted by alpha, and Type II by beta.
Outlines
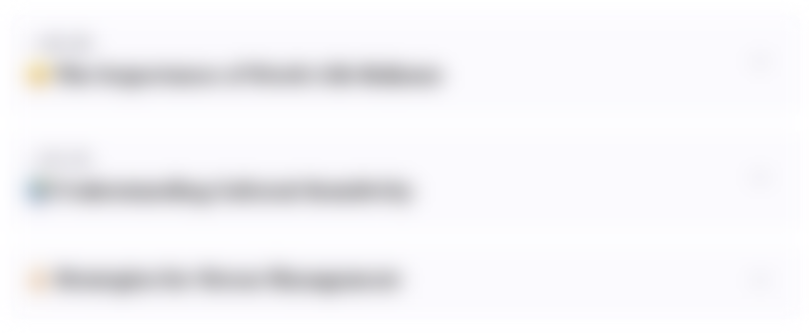
This section is available to paid users only. Please upgrade to access this part.
Upgrade NowMindmap
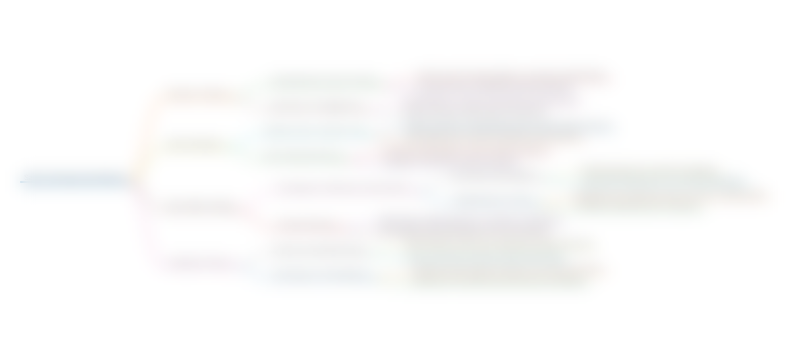
This section is available to paid users only. Please upgrade to access this part.
Upgrade NowKeywords
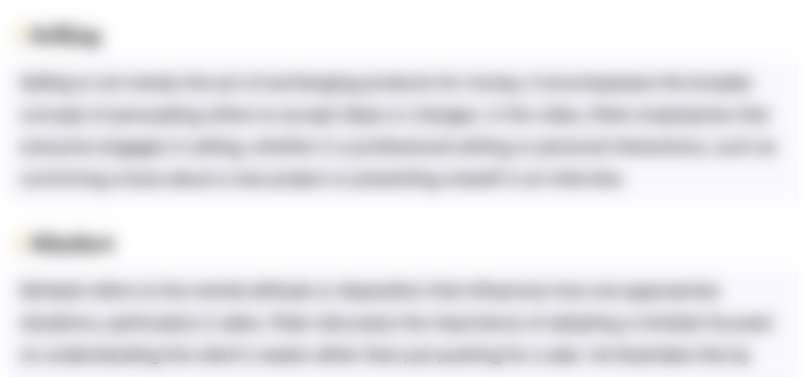
This section is available to paid users only. Please upgrade to access this part.
Upgrade NowHighlights
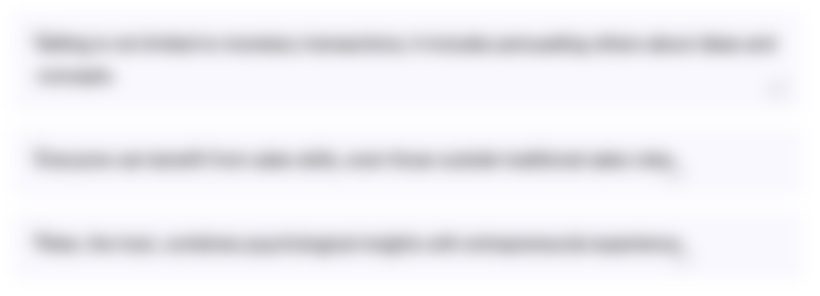
This section is available to paid users only. Please upgrade to access this part.
Upgrade NowTranscripts
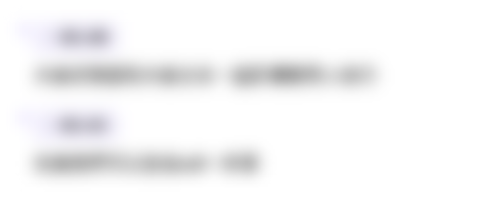
This section is available to paid users only. Please upgrade to access this part.
Upgrade NowBrowse More Related Video
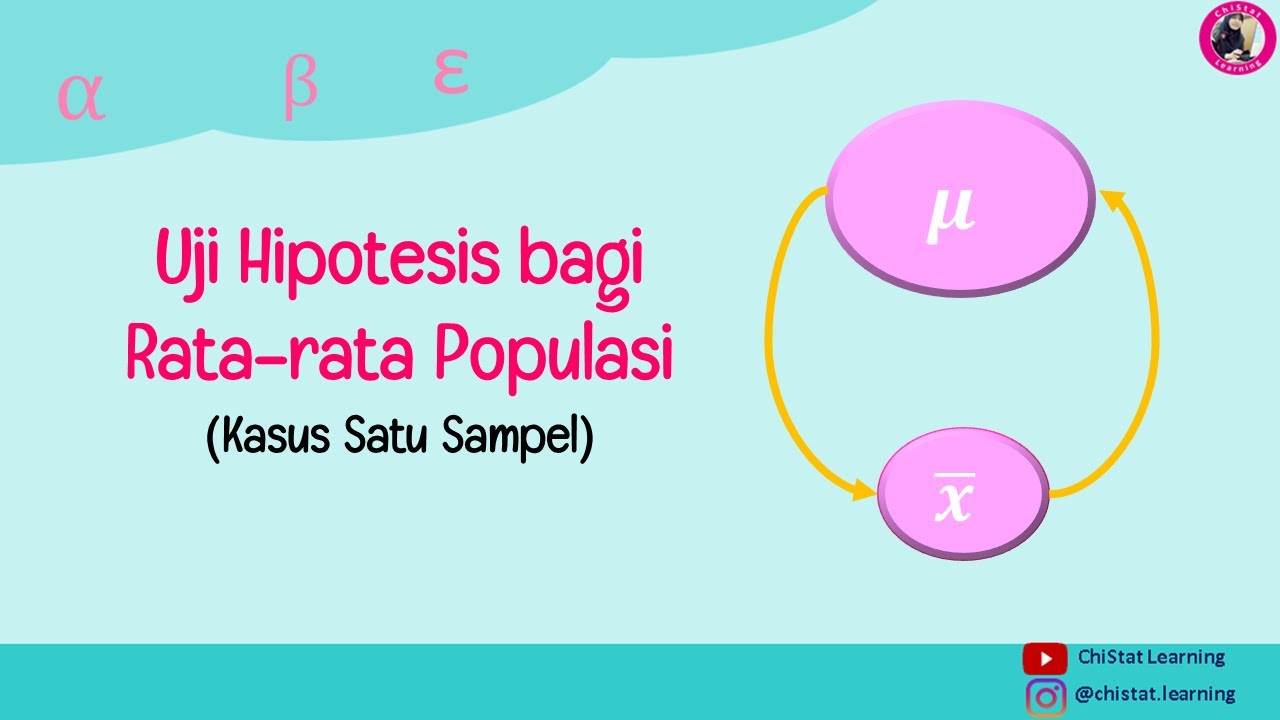
Metode Statistika | Uji Hipotesis bagi Rata-rata Satu Populasi
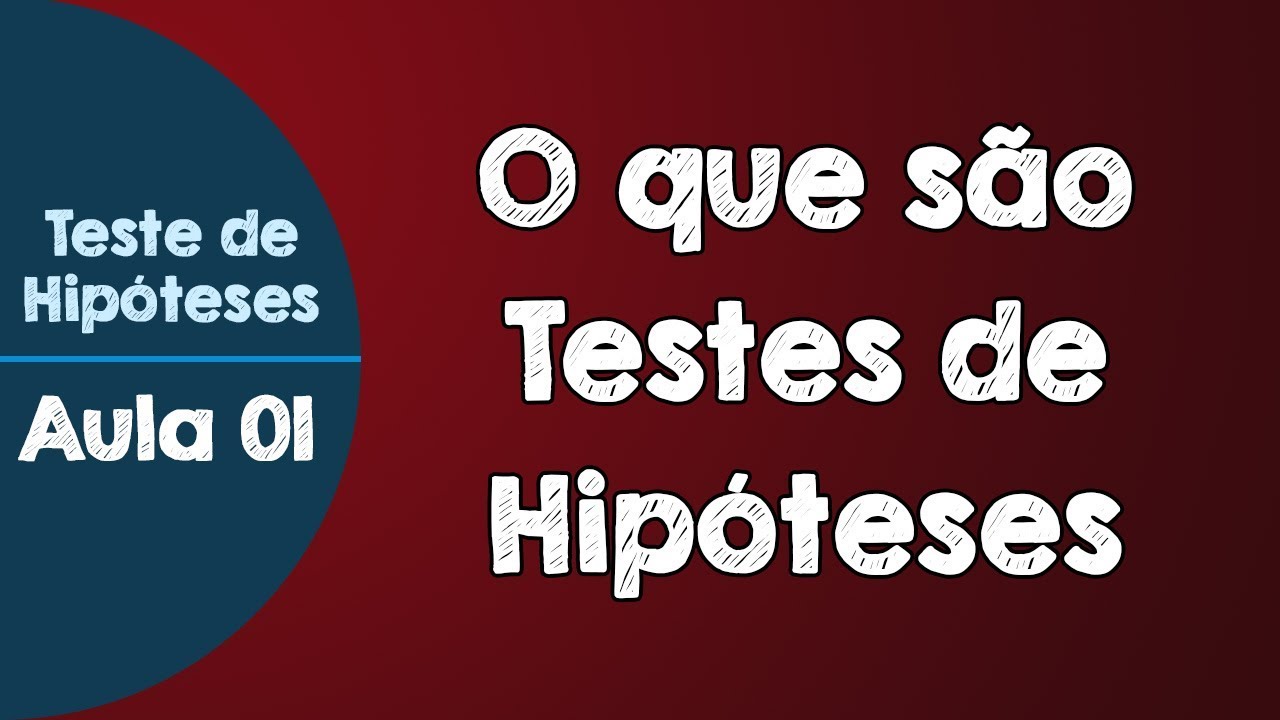
#01 - O que são Testes de Hipóteses | Para que servem os Testes de Hipóteses
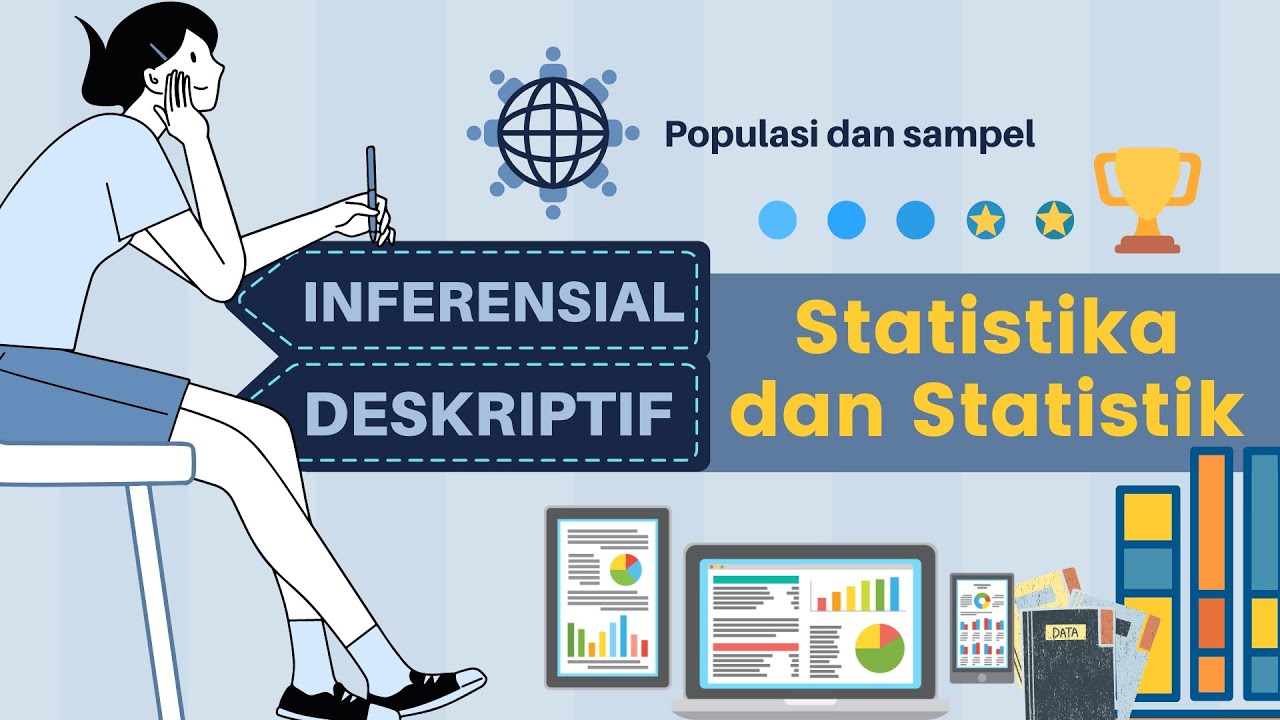
PERBEDAAN STATISTIK DESKRIPTIF DAN INFERENSIAL
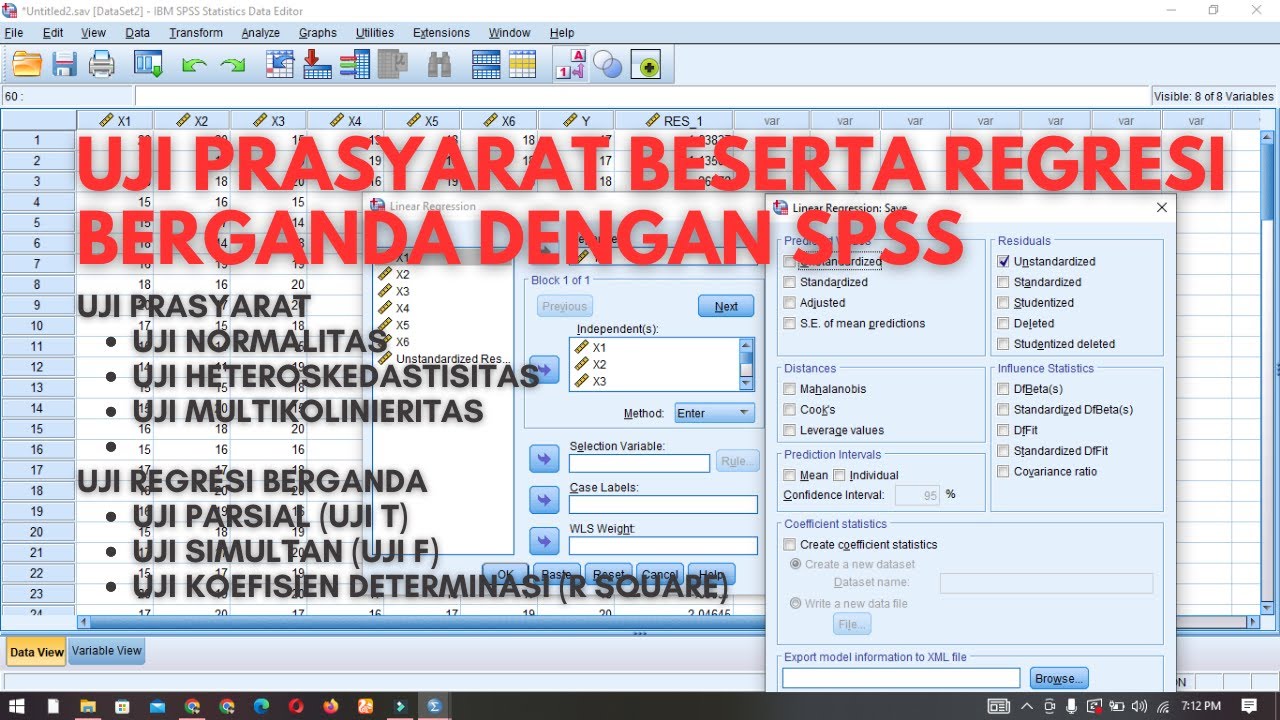
Tutorial Uji Asumsi Klasik (Prasyarat) Beserta Uji Regresi Berganda Dengan SPSS
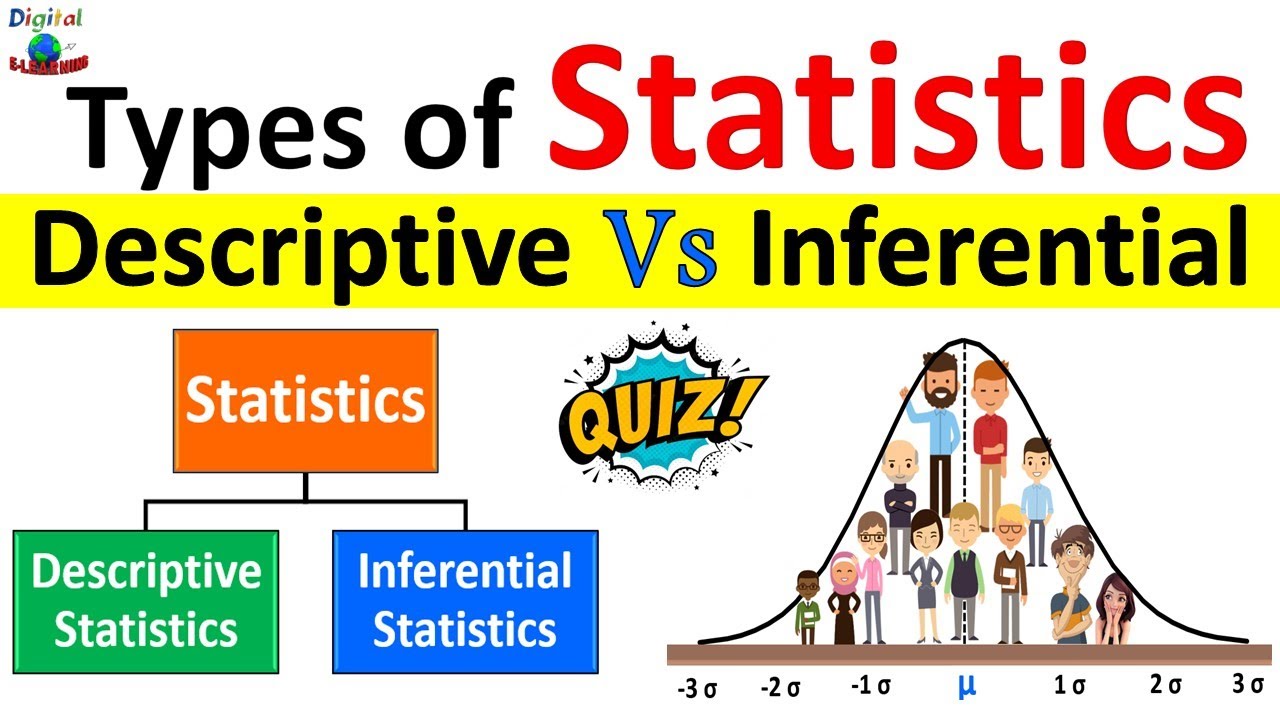
Descriptive Statistics vs Inferential Statistics | Measure of Central Tendency | Types of Statistics
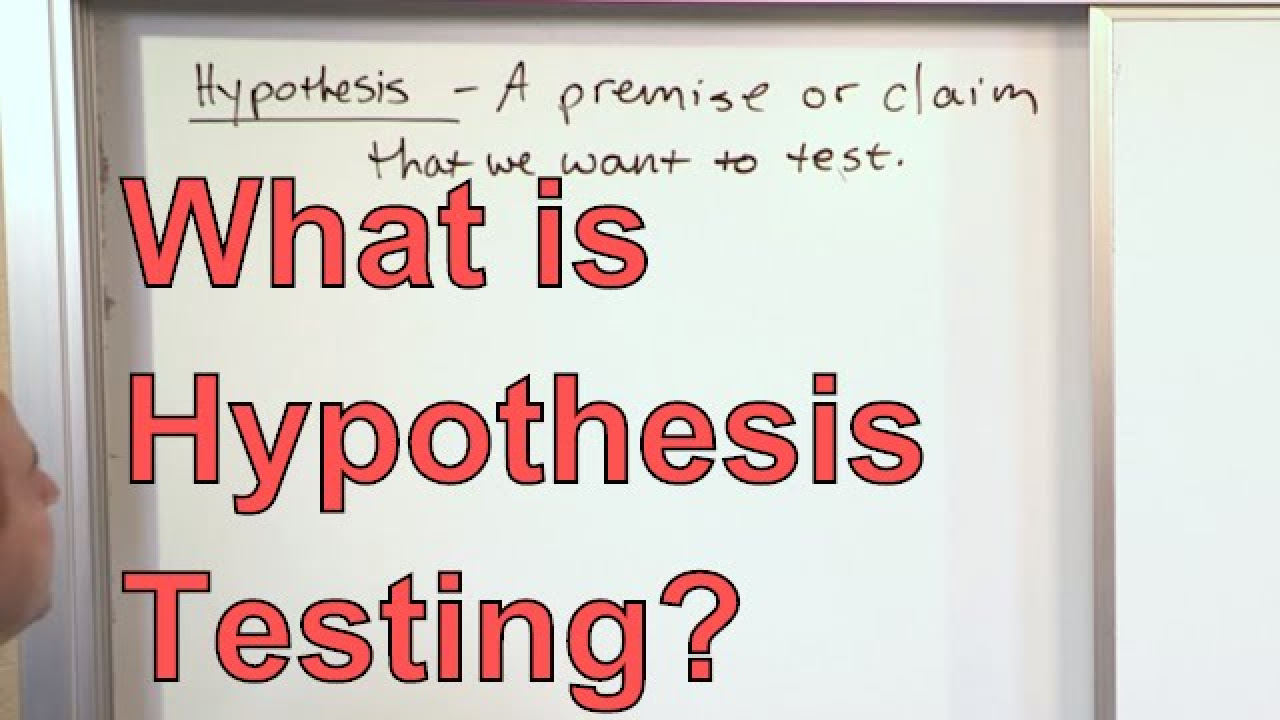
Intro to Hypothesis Testing in Statistics - Hypothesis Testing Statistics Problems & Examples
5.0 / 5 (0 votes)