สร้าง 3D Model จากรูปภาพ
Summary
TLDRThe video explores the fascinating intersection of photography and 3D modeling using advanced techniques like Neural Radiance Fields (NeRF) and photogrammetry. It discusses how AI and deep learning can transform multiple images into realistic 3D scenes, enabling users to create dynamic environments with just a set of photographs. While NeRF presents innovative rendering capabilities, it still has limitations compared to traditional photogrammetry. The video highlights practical applications in filmmaking and visual effects, along with ongoing research aimed at enhancing quality and efficiency in 3D modeling, inviting viewers to consider the future possibilities of this technology.
Takeaways
- 😀 3D models in animation, films, and games are created by combining multiple images, but this process can be complex and time-consuming.
- 🤖 Neural Radiance Fields (NeRF) utilize AI and deep learning to create realistic 3D scenes from just one set of images.
- 📷 By capturing images from all angles, NeRF can generate a 3D environment that allows for realistic movement and interaction.
- 🧠 The deep learning model used in NeRF can be trained specifically on the images provided, making it versatile.
- 🔍 Photogrammetry is a technique that extracts 3D data from 2D images, relying on methods like Structure from Motion (SfM).
- 📐 SfM identifies key points in images (like edges or colors) and determines the camera's position to create 3D vectors.
- 🛠️ NeRF renders images using deep learning to predict views that have not been captured, enhancing the 3D modeling process.
- 📊 Limitations exist in NeRF, as the quality of the generated models may not match that of traditional photogrammetry.
- 💻 Luma Lab enables users to upload images for processing into 3D scenes, which can then be exported to platforms like Unreal Engine.
- 🎥 The primary applications of these technologies are in film and VFX, but their use in gaming is still evolving.
Q & A
What is the primary topic discussed in the transcript?
-The transcript discusses the process of creating 3D models using images and introduces the concept of Neural Radiance Fields (NeRF) and photogrammetry.
What is photogrammetry, and how does it relate to 3D modeling?
-Photogrammetry is the science of extracting 3D information from 2D images. It involves techniques like Structure from Motion (SfM) to identify key points in images and calculate their 3D positions.
How does Neural Radiance Fields (NeRF) differ from traditional photogrammetry?
-NeRF uses deep learning to render images by determining key points and camera positions, allowing for the generation of realistic 3D scenes from a single set of images, whereas photogrammetry typically requires multiple images to create 3D models.
What is the role of deep learning in NeRF?
-Deep learning in NeRF helps to predict and render angles that have not been captured in the original set of images, enhancing the realism of the generated 3D scenes.
What are some limitations of NeRF mentioned in the transcript?
-NeRF has limitations in terms of quality compared to traditional photogrammetry and is still in the research phase to improve its rendering capabilities.
What is a practical application of NeRF as described in the transcript?
-A practical application of NeRF is in the creation of realistic 3D scenes for film production and visual effects (VFX), although it is not yet optimal for game development.
What does the transcript suggest about the quality of 3D models generated from fewer images?
-The transcript suggests that using fewer images can still yield acceptable quality, but using more images (like video frames) can significantly enhance the resulting 3D model's quality.
What future developments in 3D modeling technology are mentioned?
-The transcript references ongoing research projects, such as Nvidia's neural texture generation, which aim to improve 3D surface quality and the potential for generating 3D models from text prompts instead of images.
What example is given for a product that was discussed in relation to the content?
-The transcript discusses 'Gren Canola,' a product related to an advertisement that showcases how images can be processed and rendered into 3D scenes.
What does the speaker encourage viewers to do at the end of the transcript?
-The speaker encourages viewers to like and subscribe to their content to stay updated on future discussions and topics.
Outlines
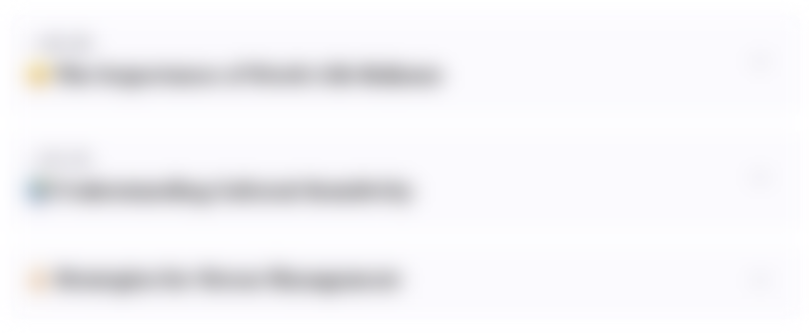
此内容仅限付费用户访问。 请升级后访问。
立即升级Mindmap
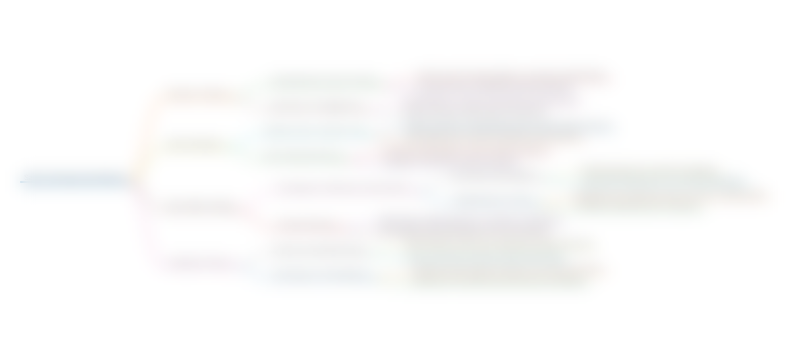
此内容仅限付费用户访问。 请升级后访问。
立即升级Keywords
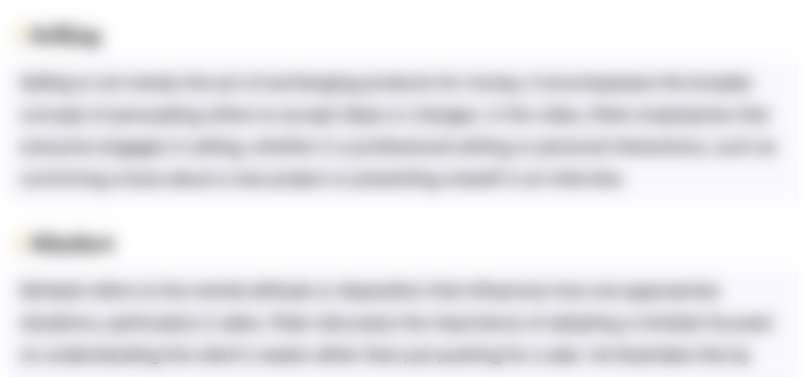
此内容仅限付费用户访问。 请升级后访问。
立即升级Highlights
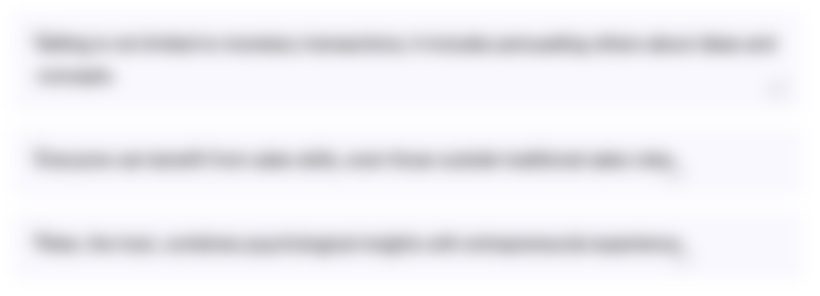
此内容仅限付费用户访问。 请升级后访问。
立即升级Transcripts
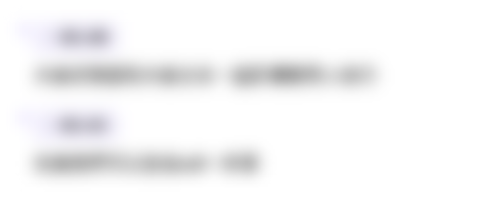
此内容仅限付费用户访问。 请升级后访问。
立即升级浏览更多相关视频
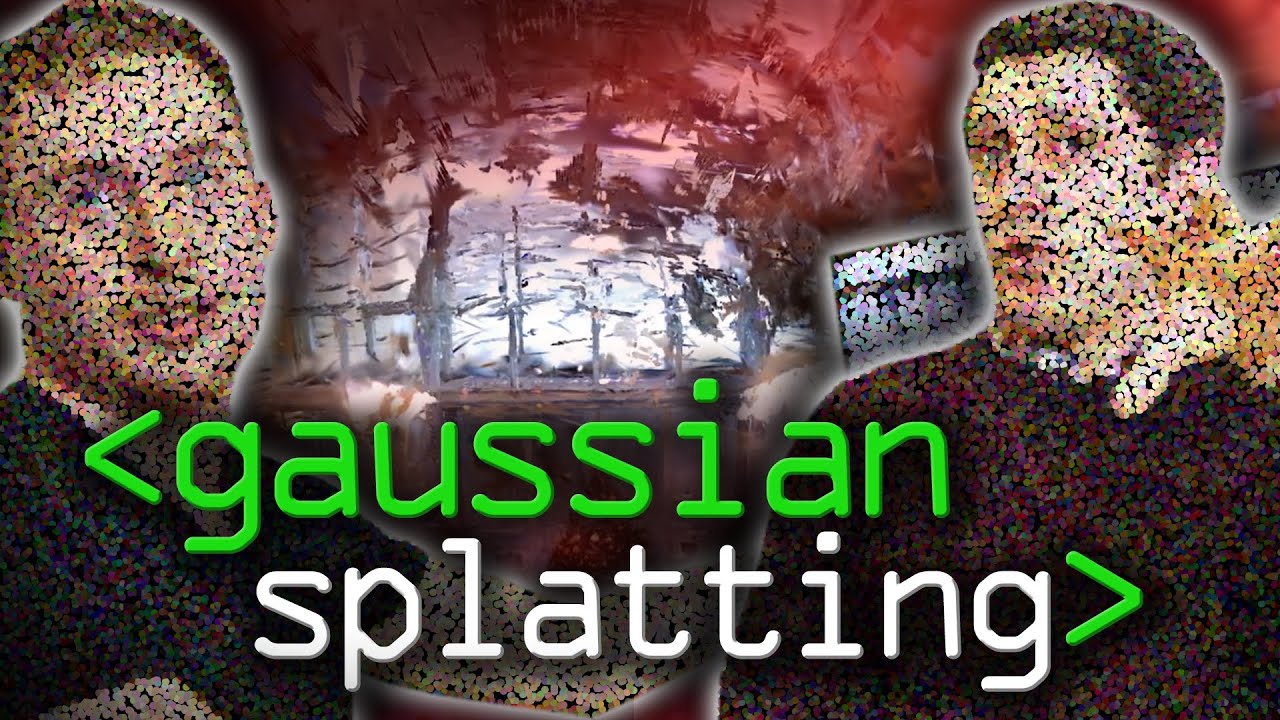
3D Gaussian Splatting! - Computerphile
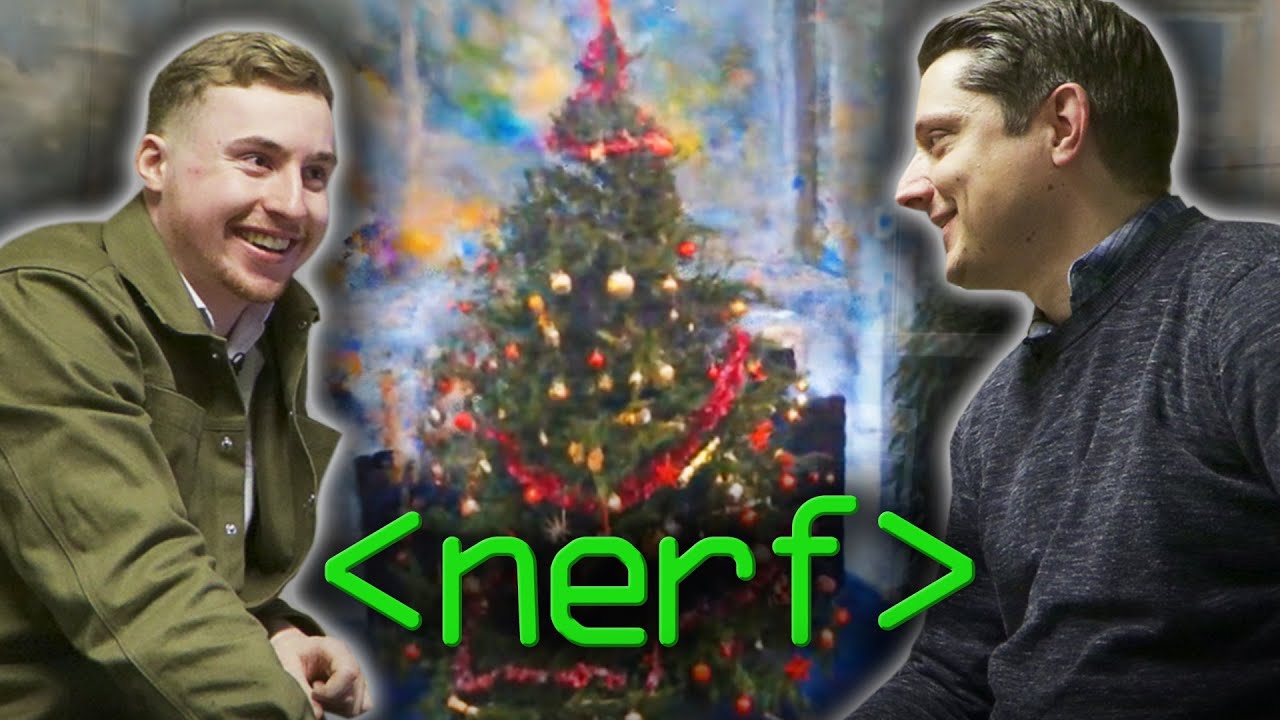
NERFs (No, not that kind) - Computerphile
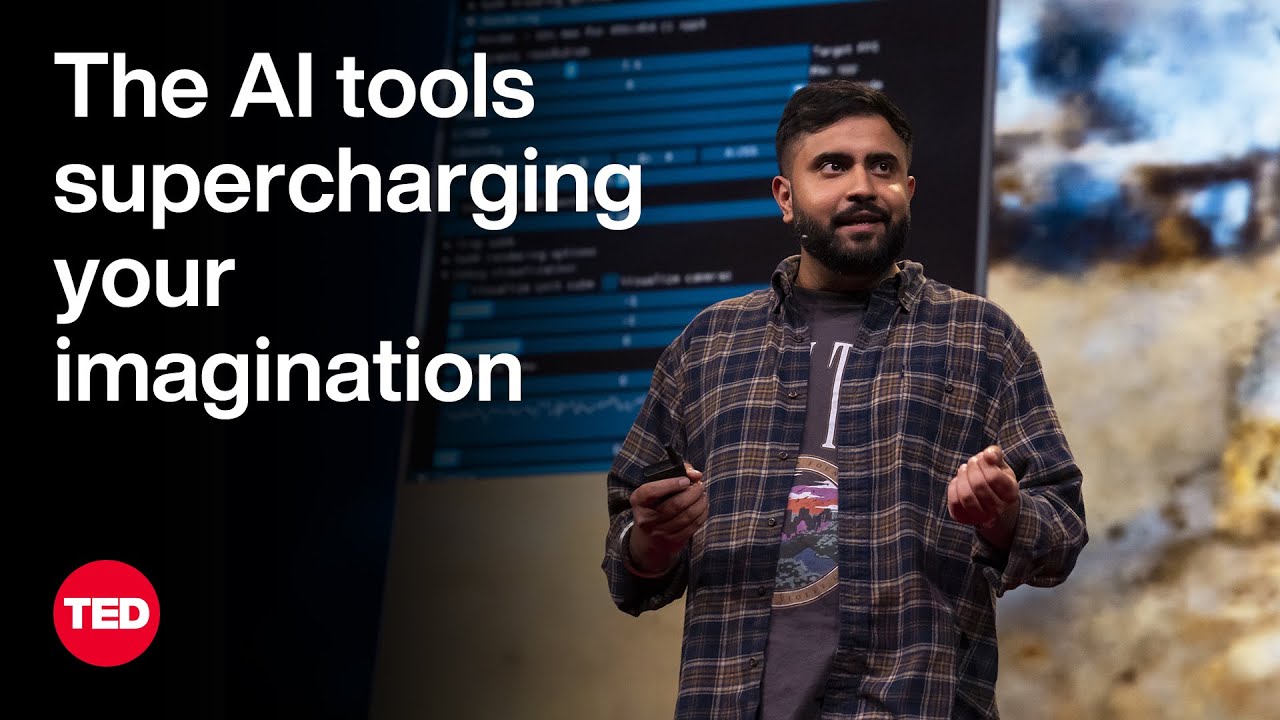
The AI-Powered Tools Supercharging Your Imagination | Bilawal Sidhu | TED
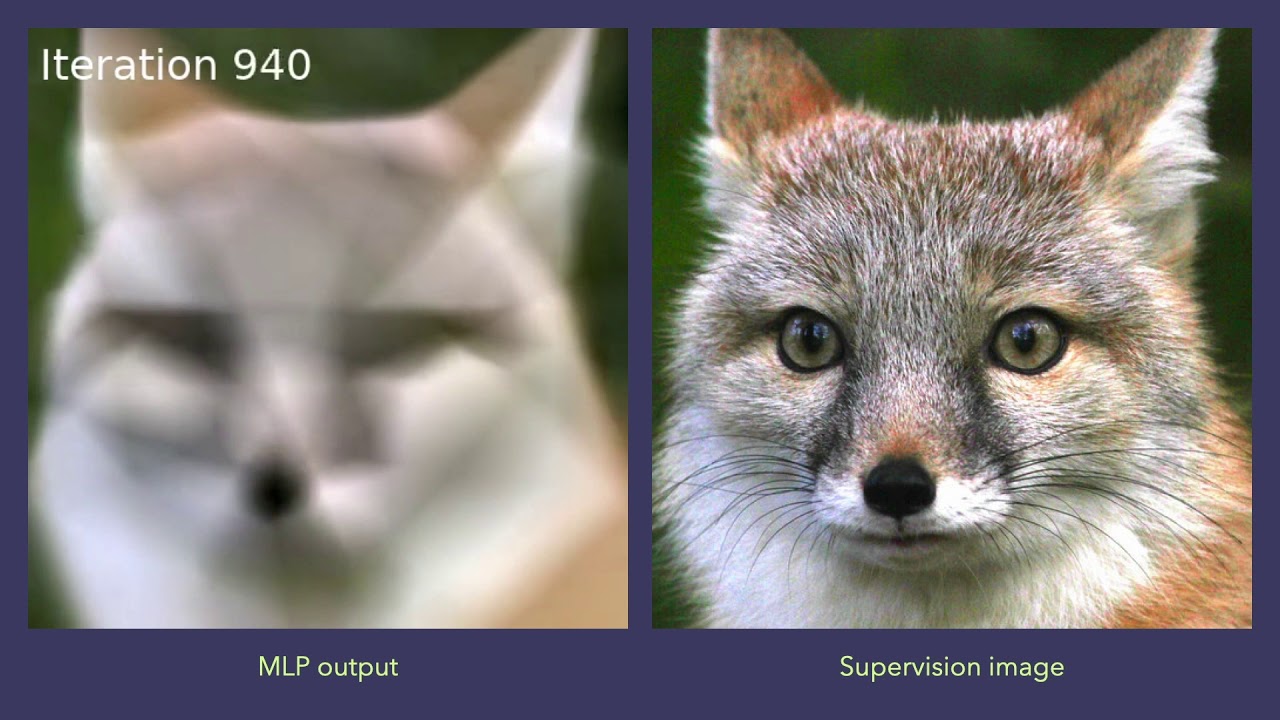
Fourier Features Let Networks Learn High Frequency Functions in Low Dimensional Domains
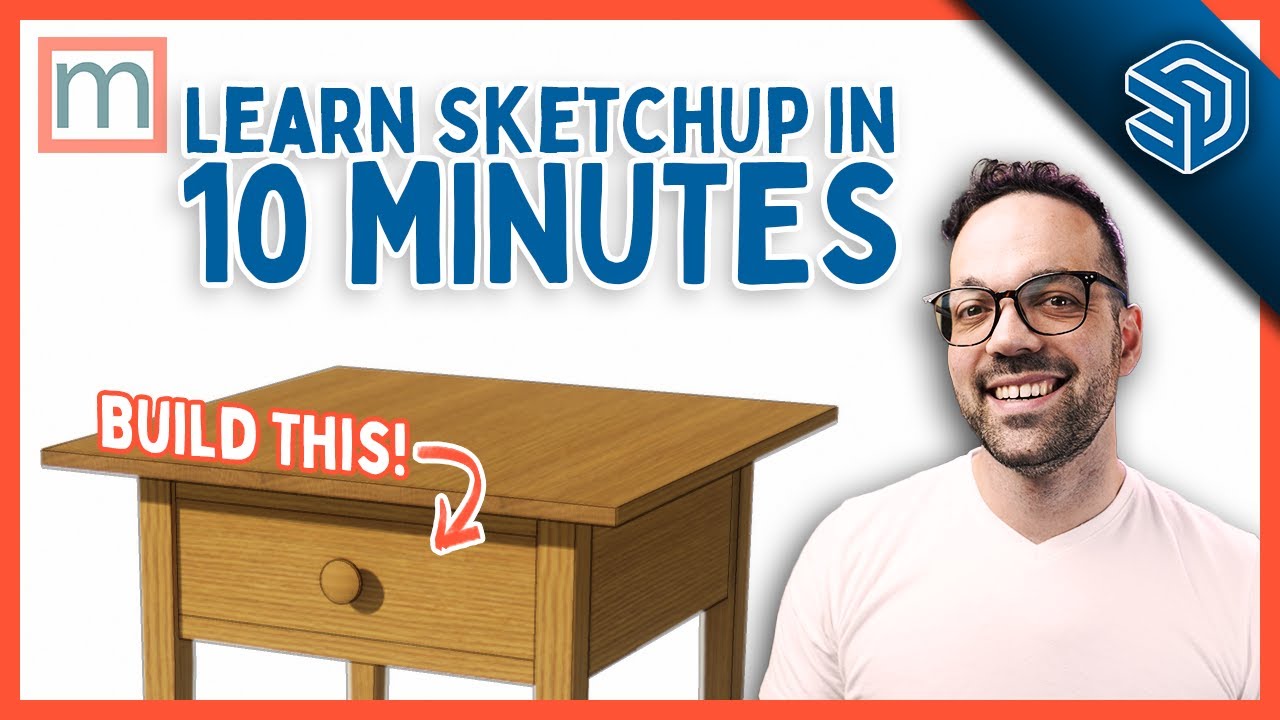
SketchUp Tutorial for Beginners - Learn SketchUp in 10 MINUTES | (SketchUp Free 2022)
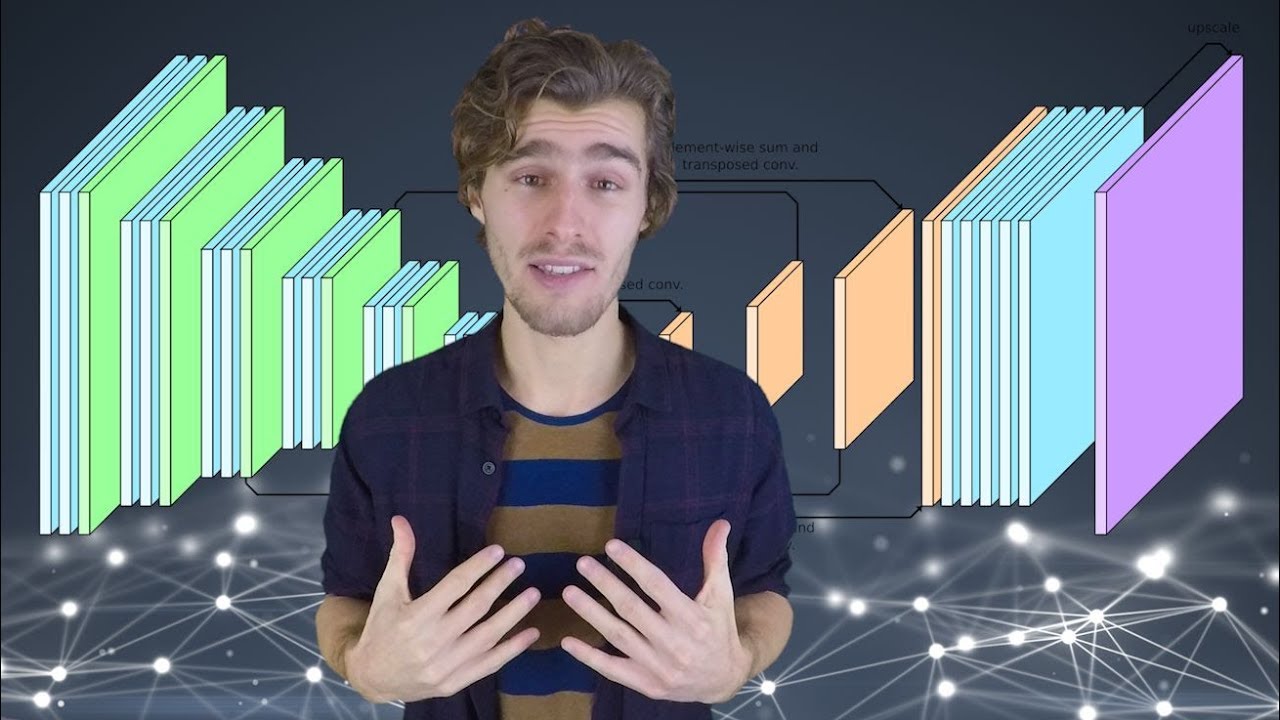
'How neural networks learn' - Part II: Adversarial Examples
5.0 / 5 (0 votes)