What's next for AI agentic workflows ft. Andrew Ng of AI Fund
Summary
TLDR本次演讲中,安德鲁教授分享了他在人工智能领域的最新见解,特别是关于AI代理的工作流程。他强调了迭代式工作流程相较于传统非代理式工作流程的优越性,并通过案例研究展示了如何通过使用GPT-3.5和代理工作流程来提高代码生成的准确性。此外,他还介绍了四种AI代理设计模式:反思、多工具使用、规划和多代理协作,并预测这些模式将显著提升AI的生产力和功能。安德鲁教授的演讲不仅为听众提供了丰富的技术知识,也为AI领域的未来发展提供了深刻的洞见。
Takeaways
- 🌟 安德烈·吴在斯坦福大学作为著名的计算机科学教授,对神经网络和GPU的发展贡献巨大,同时也是Coursera和deeplearning.ai的创始人,以及Google Brain的早期领导者。
- 📝 在使用AI模型时,非代理性工作流程类似于一次性完成任务,而代理性工作流程则更像是迭代过程,通过不断思考和修订来提高结果质量。
- 🚀 通过使用代理性工作流程,即使是GPT-3.5这样的模型也能在某些任务上表现得比GPT-4更好,这说明代理性工作流程的重要性。
- 🔍 在进行代码编写的案例研究中,使用代理性工作流程的GPT-3.5比单纯使用GPT-4的准确率更高。
- 🛠️ 代理性工作流程可以显著提高生产力,并且有四种广泛的设计模式:反思、多工具使用、规划和多代理协作。
- 🤔 反思是一种强大的技术,通过让AI模型检查和修正自己生成的代码,可以提高代码的质量和效率。
- 🔗 多工具使用允许AI模型结合多种工具和资源来完成任务,扩展了大型语言模型的应用范围。
- 📈 规划算法使得AI代理能够在遇到问题时自主规划解决方案,提高了问题解决的灵活性和创造性。
- 🤖 多代理协作通过模拟多个专家角色的互动,可以产生复杂的解决方案和创新性的结果。
- 💡 快速生成token对于代理性工作流程至关重要,因为它允许AI模型快速迭代,从而提高整体性能。
- 🌐 未来AI的能力将因代理性工作流程而显著扩展,我们需要适应让AI代理独立工作并耐心等待结果的工作方式。
- 🚀 代理性推理设计模式将是推动AI发展的重要趋势,可能帮助我们在实现通用人工智能(AGI)的漫长旅程中迈出一小步。
Q & A
安德烈在人工智能领域有哪些贡献?
-安德烈是斯坦福大学著名的计算机科学教授,他在神经网络与GPU的发展上做出了早期贡献。他是Coursera的创始人,以及deeplearning.ai等流行课程的创建者。此外,他还是Google Brain的创始人和早期领导者。
什么是非代理性工作流程?
-非代理性工作流程是指使用大型语言模型(LM)的方式,用户输入一个提示,模型生成一个答案,类似于要求一个人一次性写下整篇文章,而不允许使用退格键进行修改的过程。
代理性工作流程与非代理性工作流程有何不同?
-代理性工作流程是一种更迭代的过程,其中AI可能会进行一些思考,然后修订文章,并可能进行多次迭代。这种方法可以带来更好的结果,因为它允许AI在生成内容后进行自我反思和修正。
使用代理性工作流程有什么好处?
-使用代理性工作流程可以显著提高大型语言模型的性能。例如,在一项研究中,使用代理性工作流程的GPT-3.5在某些任务上的表现甚至超过了更先进的GPT-4。
什么是代理性反思?
-代理性反思是一种设计模式,其中一个AI系统被提示执行一个任务,然后再次被提示检查其执行的结果,确保其正确性、效率和良好的结构。这可以帮助AI发现并修正自己的错误。
多代理协作是如何工作的?
-多代理协作涉及多个AI代理共同完成任务。每个代理可以扮演不同的角色,例如一个负责编写代码,另一个负责审查代码。通过这种方式,AI代理可以相互合作,提高工作效率和质量。
规划算法在AI中的作用是什么?
-规划算法使AI能够进行更复杂的任务,如分析、收集信息、采取行动和提高个人生产力。它们可以帮助AI在遇到问题时重新规划和调整策略,以实现目标。
为什么快速生成令牌(tokens)在代理性工作流程中很重要?
-在代理性工作流程中,快速生成令牌对于迭代过程至关重要。因为AI需要生成大量令牌供自己阅读和处理,所以能够快速生成令牌可以显著提高工作效率。
未来的人工智能发展趋势是什么?
-未来的人工智能发展可能会集中在代理性工作流程和代理性推理设计模式上。这些模式可以帮助我们更有效地使用AI,提高生产力,并可能在实现通用人工智能(AGI)的漫长道路上迈出一小步。
为什么我们需要适应等待AI生成响应?
-由于代理性工作流程可能需要多次迭代和深思熟虑的过程,我们需要学会耐心等待AI生成响应。这可能需要几分钟甚至几小时,而不是像传统的即时反馈那样迅速。
如何提高AI代理的工作效率?
-提高AI代理的工作效率可以通过使用代理性工作流程、多代理协作、规划算法和快速生成令牌等设计模式。这些方法可以帮助AI更有效地完成任务,并提高其性能。
Outlines
🤖 人工智能代理的工作流程与设计模式
本段落主要介绍了人工智能代理的工作流程和设计模式。首先,通过与非代理性工作流程的对比,阐述了代理性工作流程的迭代性和优越性。接着,通过实际案例分析,说明了使用代理性工作流程的GPT-3.5在编码任务上的表现优于直接使用GPT-4。此外,还介绍了四种主要的设计模式:反思、多工具使用、规划和多代理协作,这些模式在提高AI性能和生产力方面具有重要意义。最后,强调了快速生成令牌的重要性,并对未来AI技术的发展表示期待。
🔄 反思与自我评估在AI编程中的应用
这一部分详细探讨了反思这一设计模式在AI编程中的应用。通过让AI系统对自己的代码进行自我评估和修正,可以显著提高代码的质量和效率。例如,通过让AI系统检查自己生成的代码,并提出改进意见,可以引导AI进行自我修正,从而得到更优的代码版本。这种方法不仅展示了AI的自我反思能力,也为提高AI编程效率提供了新的思路。
🔧 多工具使用与多代理协作的AI设计模式
本段落讨论了多工具使用和多代理协作两种AI设计模式。多工具使用模式通过结合不同的工具和平台,扩展了AI的功能和应用范围。例如,AI可以通过生成代码、搜索网络和执行任务来帮助用户完成复杂的工作。而多代理协作模式则通过模拟多个AI代理之间的合作,提高了问题解决的效率和创新性。例如,通过创建不同的代理角色,如CEO、设计师、产品经理和测试员,可以让它们进行协作对话,共同开发软件或解决问题。这些设计模式展示了AI在复杂任务处理中的潜力和灵活性。
Mindmap
Keywords
💡神经网络
💡深度学习
💡人工智能代理
💡迭代工作流程
💡自我反思
💡多代理协作
💡规划
💡两用技术
💡快速令牌生成
💡代理推理设计模式
💡多代理辩论
Highlights
安德鲁是斯坦福大学著名的计算机科学教授,早期在神经网络与GPUs的发展中做出了贡献。
安德鲁是Coursera的创始人,以及deeplearning.ai等流行课程的创建者。
安德鲁是Google Brain的创始人和早期领导者。
人工智能代理是当前AI领域值得关注的激动人心的趋势。
非代理性工作流程中,人们使用语言模型的方式类似于一次性生成答案,不进行迭代。
代理性工作流程通过迭代和反馈改进,可以显著提高结果的质量。
通过使用代理性工作流程,GPT-3.5在某些任务上的表现甚至超过了GPT-4。
反射是一种强大的技术,可以让语言模型自我检查和改进代码。
多代理协作和规划算法是新兴的技术,有时能带来令人惊讶的结果。
使用多代理系统,可以模拟不同角色的协作,如CEO、设计师、产品经理和测试员。
代理性推理设计模式将是未来AI发展中的重要趋势。
快速生成token对于代理性工作流程至关重要,因为它可以加快迭代速度。
对于AI代理的期待是,它们能够在更长的时间内自主工作,而不仅仅是即时响应。
多代理辩论可以提高AI系统的性能,这是一种强大的设计模式。
通过代理性工作流程,我们可以在实现通用人工智能(AGI)的漫长旅程中迈出一小步。
Transcripts
all of you uh know Andreu in as a famous
uh computer science professor at
Stanford was really early on in the
development of neural networks with gpus
of course a creator of corsera and
popular courses like
deeplearning.ai also the founder and
Creator uh and early lead of Google
brain uh but one thing I've always
wanted to ask you before I hand it over
Andrew while you're on stage uh is a
question I think would be relevant to
the whole audience 10 years ago on
problem set number two of cs229 you gave
me a
b and I was wondering I looked it over I
was wondering what you saw that I did
incorrectly so anyway Andrew thank you
Hansen um looking forward to sharing
with all of you what I'm seeing with AI
agents which I think is the exciting
Trend that I think everyone building in
AI should pay attention to and then also
excited about all all the other uh on
Sak presentations so hey agents you know
today the way most of us use Lish models
is like this with a non- agentic
workflow where you type a prompt and
generates an answer and that's a bit
like if you ask a person to write an
essay on a topic and I say please sit
down to the keyboard and just type the
essay from start to finish without ever
using backspace um and despite how hard
thises is L's do it remarkably well in
contrast with an agentic workflow this
is what it may look like have an AI have
an LM say write an essay outline do you
need to do any web research if so let's
do that then write the first draft and
then read your own first draft and think
about what parts need revision and then
revise your draft and you go on and on
and so this workflow is much more
iterative where you may have the L do
some thinking um and then revise this
article and then do some more thinking
and iterate this through a number of
times and what not many people
appreciate is this delivers remarkably
better results um I've actually been
really surprised myself working these
agent workflows how well how well they
work I's do one case study at my team
analyzed some data uh using a coding
Benchmark called the human eval
Benchmark released by open a few years
ago um but this says coding problems
like given the nonent list of integers
return the sum of all the all elements
are an even positions and it turns out
the answer is you code snipper like that
so today lot of us will use zero shot
prompting meaning we tell the AI write
the code and have it run on the first
spot like who codes like that no human
codes like that just type out the code
and run it maybe you do I can't do that
um so it turns out that if you use GPT
3.5 uh zero shot prompting it gets it
48% right uh gp4 way better 607 7% right
but if you take an agentic workflow and
wrap it around GPT 3.5 I say it actually
does better than even
gbd4 um and if you were to wrap this
type of workflow around gb4 you know it
it it also um does very well and you
notice that gbd 3.5 with an agentic
workflow actually outperforms
gp4 um and I think this has and this
means that this has signant consequences
fighting how we all approach building
applications so agents is the ter of
around a lot there's a lot of consultant
reports talk about agents the future of
AI blah blah blah I want to be a bit
concrete and share of you um the broad
design patterns I'm seeing in agents
it's a very messy chaotic space tons of
research tons of Open Source there's a
lot going on but I try to categorize um
bit more concretely what's going on
agents reflection is a tool that I think
many of us should just use it just works
uh to use I think it's more widely
appreciated but actually works pretty
well I think of these as pretty robust
technology when I use them I can you
know almost always get them to work well
um planning and multi-agent
collaboration I think is more emerging
when I use them sometimes my mind is
blown for how well they work but at
least at this moment in time I don't
feel like I can always get them to work
Rel Lively so let me walk through these
four design patterns in the few slides
and if some of you go back and yourself
will ask your engineers to use these I
think you get a productivity boost quite
quickly so reflection here's an example
let's say ask a system please write code
for me for a given task then we have a
coder agent just an LM that you prompt
to write code to say you def du task
write a function like that um an example
of
self-reflection would be if you then
prompt the LM with something like this
here's code intended for a toas and just
give it back the exact same code that
they just generated and then say check
the code carefully for correctness sound
efficiency good construction CRI just
write prompt like that it turns out the
same l that you prompted to write the
code may be able to spot problems like
this bug in line Five May fix it by blah
blah blah and if you now take his own
feedback and give it to it and reprompt
it it may come up with a version two of
the code that could well work better
than the first version not guaranteed
but it works you know often enough for
this be wor trying for a lot of
applications um to foreshadow to use if
you let it run unit test if it fails a
unit test then he why do you fail the
unit test have that conversation and be
able to figure out fail the unit test so
you should try changing something and
come up with V3 by the way for those of
you that want to learn more about these
Technologies I'm very excited about them
for each of the four sections I have a
little recommended reading section at
the bottom that you know hopefully gives
more references and again just the
foreshadow multi-agent systems I've
described as a single coder agent that
you prompt to have it you know have this
conversation with itself um one Natural
Evolution of this idea is instead of a
single code agent you can can have two
agents where one is a coder agent and
the second is a Critic agent and these
could be the same base LM model but that
you prompt in different ways where you
say one your expert coder right code the
other one say your expert code review to
review this code and this Tye of
workflow is actually pretty easy to
implement I think it's such a very
general purpose technology for a lot of
workflows this would give you a
significant boost in in the performance
of LMS um the second design pattern is
to use many of where already have seen
you know LM based systems uh uh using
tools on the left is a screenshot from
um co-pilot on the right is something
that I kind of extracted from uh gp4 but
you know LM today if you ask it what's
the best coffee maker web search for
some problems um will generate code and
run code um and it turns out that there
are a lot of different tools that many
different people are using for analysis
for gathering information for taking
action for personal productivity
um it turns out a lot of the early work
in two use turned out to be in the
computer vision Community because before
large language models lm's you know they
couldn't do anything with images so the
only option was that the LM generate a
function called that could manipulate an
image like generate an image or do
object detection or whatever so if you
actually look at literature it's been
interesting how much of the work um in
two years seems like it originated from
Vision because LMS would blind to images
before you know gp4 and and and lava and
so on um so that's two use and it
expands what an LM can do um and then
planning you know for those of you that
have not yet played a lot with planning
algorithms I I feel like a lot of people
talk about the chat GPT moment where
you're wow never seen anything like this
I think if not used planning alums many
people will have a kind of a AI agent
wow I couldn't imagine the AI agent
doing this I've run live demos where
something failed and the AI agent
rerouted around the failures I've
actually had quite a few of those moment
wow you can't believe my AI system just
did that autonomously but um one example
that I adapted from a hugging GPT paper
you know you say this general image
where the girls read where a girl is
reading a book and it posts the same as
a boy in the image example. jpack and
please subscribe the new image for your
voice so give an example like this um
today we have ai agents who can kind of
decide first thing I need to do is
determine the post of the boy
um then you know find the right model
maybe on hugging face to extract the
post then next need to find a post image
model to synthesize a picture of a of a
girl of as following the instructions
then use image to text to and then
finally use text of speech and today we
actually have agents that I don't want
to say they work reliably you know
they're kind of finicky they don't
always work but when it works is
actually pretty amazing but with agentic
loops sometimes you can recover from
earlier failures as well so I find
myself already using research agents for
some of my work where one of piece of
research but I don't feel like you know
Googling myself and spend a long time I
should send to the research agent come
back in a few minutes and see what it's
come up with and and it sometimes works
sometimes doesn't right but that's
already a part of my personal
workflow the final design pattern multi-
Asian collaboration this is one of those
funny things but uh um it works much
better than you might think
uh uh but on the left is a screenshot
from a paper called um chat Dev uh which
is completely open which actually open
source many of you saw the you know
flashy social media announcements of
demo of a Devon uh uh Chad Dev is open
source it runs on my laptop and what
Chad Dev doeses is example of a
multi-agent system where you prompt one
LM to sometimes act like the CEO of a
software engine company sometimes Act
designer sometime a product manager
sometimes I a tester and this flock of
agents that you built by prompting an LM
to tell them you're now Co you're now
software engineer they collaborate have
an extended conversation so that if you
tell it please develop a game develop a
GOI game they'll actually spend you know
a few minutes writing code testing it uh
iterating and then generate a like
surprisingly complex programs doesn't
always work I've used it sometimes it
doesn't work sometimes it's amazing but
this technology is really um getting
better and and just one of design
pattern it turns out that multi-agent
debate where you have different agents
you know for example could be have ch
GPT and Gemini debate each other that
actually results in better performance
as well so having multiple simulated air
agents work together has been a powerful
design pattern as well um so just to
summarize I think these are the these
are the the the uh patterns of seen and
I think that if we were to um use these
uh uh patterns you know in our work a
lot of us can get a prity boost quite
quickly and I think that um agentic
reasoning design patterns are going to
be important uh this is my small slide I
expect that the set of T AI could do
will expand dramatically this year uh
because of agentic workflows and one
thing that it's actually difficult
people to get used to is when we prompt
an LM we want to response right away
um in fact a decade ago when I was you
know having discussions around at at at
Google on um it called a big box search
we type a long prompt one of the reasons
you know I failed to push successfully
for that was because when you do a web
search you one of responds back in half
a second right that's just human nature
we like that instant grab instant
feedback but for a lot of the agent
workflows um I think we'll need to learn
to dedicate the toss and AI agent and
patiently wait minutes maybe even hours
uh to for a response but just like I've
seen a lot of novice managers delegate
something to someone and then check in 5
minutes later right and that's not
productive um I think we need to it be
difficult we need to do that with some
of our AI agents as well I saw I heard
some loss um and then one other
important Trend fast token generation is
important because with these agented
workflows we're iterating over and over
so the LM is generating tokens for the
elm to read so be able to generate
tokens way faster than any human to read
is fantastic and I think that um
generating more tokens really quickly
from even a slightly lower quality LM
might give good results compared to
slower tokens from a better LM maybe
it's a little bit controversial because
it may let you go around this Loop a lot
more times kind of like the results I
showed with gbd3 and an agent
architecture on the first slide um and
cand I'm really looking forward to Cloud
5 and uh CL 4 and gb5 and Gemini 2.0 and
all these other wonderful models that
may are building
and part of me feels like if you're
looking forward to running your thing on
gp5 zero shot you know you mayble to get
closer to that level performance on some
applications than you might think with
agenting reasoning um but on an early
model I think I I I I think this is an
important Trend uh uh and honestly the
path to AGI feels like a journey rather
than a destination but I think this typ
of agent workflows could help us take a
small step forward on this very long
journey thank
[Applause]
you
浏览更多相关视频
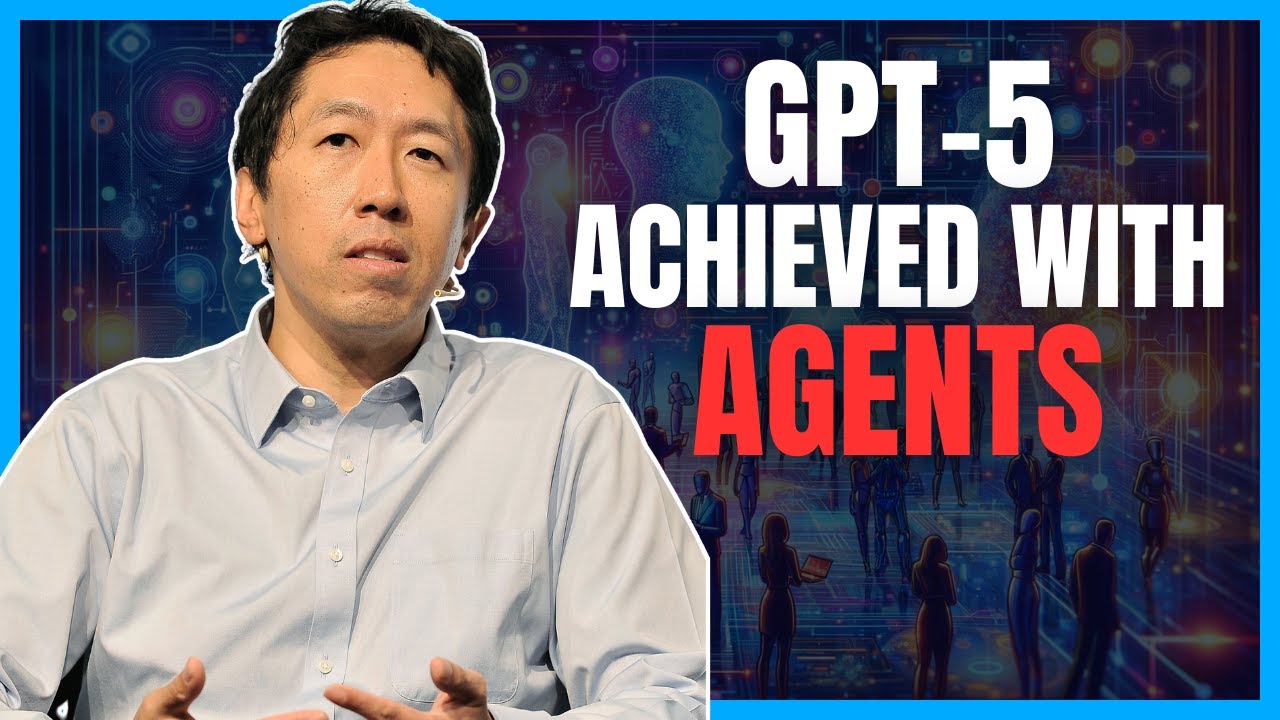
AI Pioneer Shows The Power of AI AGENTS - "The Future Is Agentic"
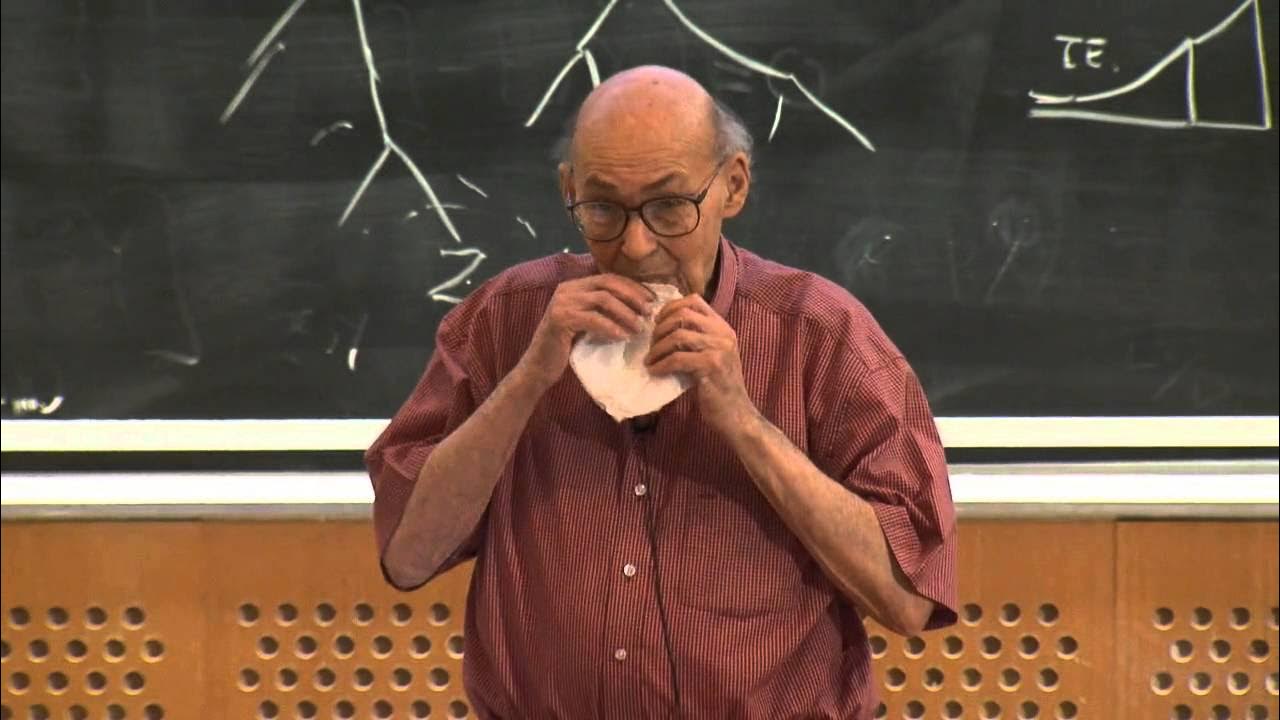
3. Cognitive Architectures
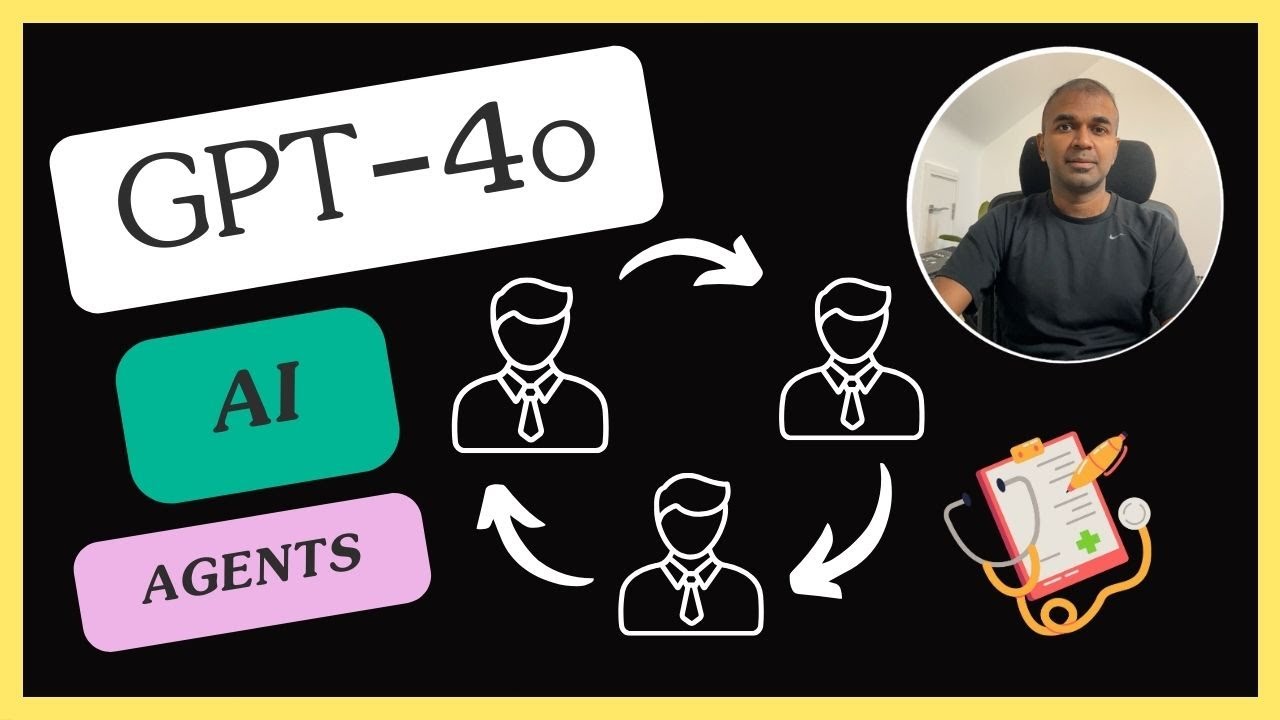
GPT-4o AI Agents: Easily Create Medical Research Agents (Praison AI)
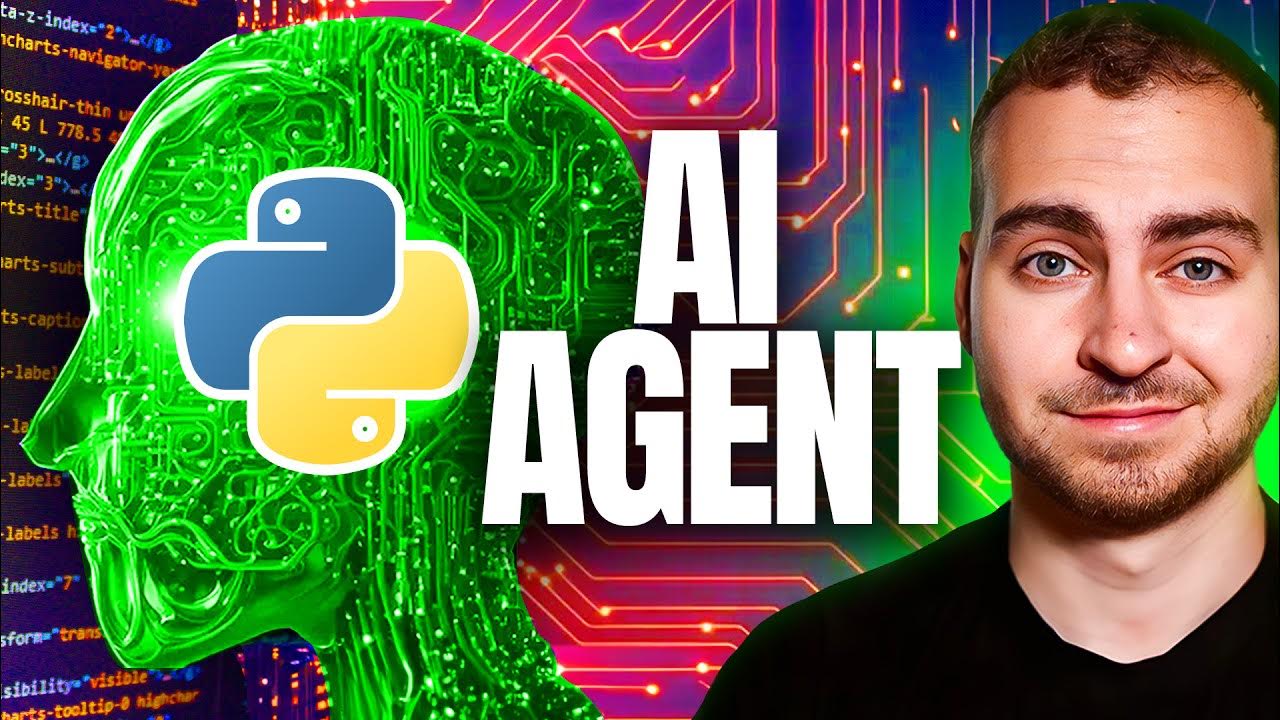
Python Advanced AI Agent Tutorial - LlamaIndex, Ollama and Multi-LLM!
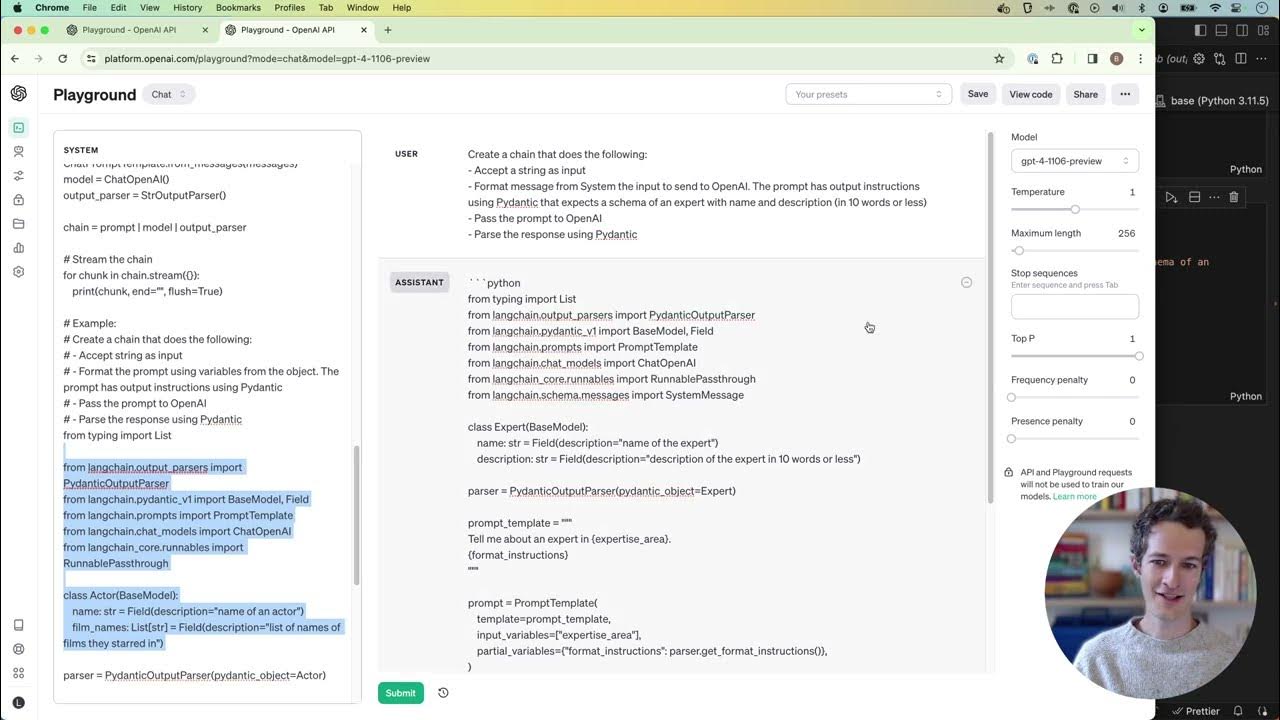
Build an AI code generator w/ RAG to write working LangChain
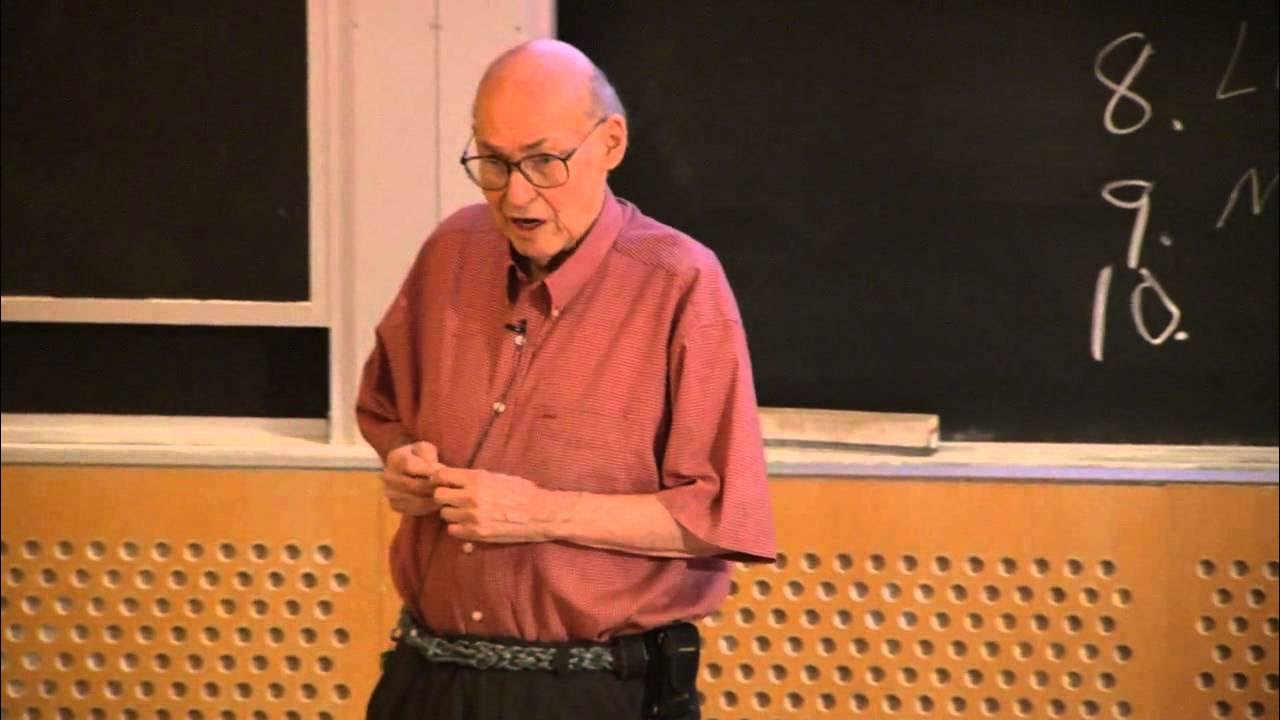
2. Falling In Love
5.0 / 5 (0 votes)