2024年开年AI大牛世界论坛关于AI的三大访谈之一 李飞飞、吴恩达对谈:这一次,AI冬天不会到来2024 A Dialogue between Li Fei-Fei and Andrew Ng
Summary
TLDR本次访谈邀请了斯坦福大学教授、被誉为人工智能之母的李飞飞和AI基金的总经理合伙人、Google Brain的创始领导安德鲁·吴,共同探讨了人工智能的现状与未来。两位专家分享了他们对AI技术发展的看法,包括AI在不同领域的应用、AI伦理问题、以及AI对社会和经济的深远影响。他们还讨论了AI技术的最新突破,以及如何平衡技术创新与社会责任。
Takeaways
- 🤖 AI的未来不会被媒体炒作所左右,商业基础比以往任何时候都更加坚实。
- 🚀 尽管存在对AI寒冬的担忧,但AI作为一个通用技术,其商业应用前景仍然非常广阔。
- 🌟 2024年AI可能的重大突破包括视频、时间序列、生物学和化学领域的进展。
- 🖼️ 计算机视觉和图像处理技术即将取得令人兴奋的进展,可能会与大型语言模型相媲美。
- 🧠 公共部门的AI发展将得到更多资源,非营利组织在AI领域的突破也将更加显著。
- 🤔 AI在特定任务上的应用将更加广泛,特别是在数据丰富且模式可重复的领域。
- 🛠️ 企业应关注AI在特定业务和行业中的具体应用,这些应用可能带来独特的竞争优势。
- 📉 对于AI的准确性问题,需要根据行业和风险水平来评估AI的应用范围和限制。
- 📰 关于生成性AI和知识产权的诉讼,特别是纽约时报与OpenAI的案件,反映了创作者经济与AI技术之间的紧张关系。
- 🌐 开源LLM(大型语言模型)与闭源LLM之间的竞争将继续,但模型的发展方向和数据使用将有所不同。
- 💡 AI技术的发展需要新的突破,例如子二次方架构或液态神经网络,以超越现有的Transformer模型。
Q & A
Rajiv Chand 在本次会议上担任什么角色?
-Rajiv Chand 在本次会议上担任主持人(moderator)。
Fe Lee 教授在斯坦福大学的职位是什么?
-Fe Lee 教授是斯坦福大学的教授,同时也是斯坦福人类中心人工智能研究所的联合主任。
Andrew Ng 在本次会议上的角色是什么?
-Andrew Ng 是 AI fund 的常务总经理合伙人,也是 Google Brain 的创始领导。
Fe Lee 教授被广泛认可为什么?
-Fe Lee 教授被广泛认可为人工智能的“祖母”。
Fe Lee 教授和 Andrew Ng 是何时首次相识的?
-Fe Lee 教授和 Andrew Ng 大约在 2007 年的某个会议或研讨会上首次相识。
Fe Lee 教授的哪本书被推荐给了观众?
-Fe Lee 教授的书《The World I See》被推荐给了观众。
Andrew Ng 预测 2024 年人工智能将会有哪些突破?
-Andrew Ng 预测 2024 年人工智能将在视频、时间序列、生物学和化学领域有重大突破。
Fe Lee 教授对未来人工智能的预测是什么?
-Fe Lee 教授预测未来人工智能将在像素空间(pixel space)有令人兴奋的技术进步,并且公共部门的人工智能将得到更好的资源支持。
在讨论中,对于 AI 代理的未来,Fe Lee 教授和 Andrew Ng 有什么不同的观点?
-Fe Lee 教授倾向于使用“辅助代理”(assistive agents)这个术语,而 Andrew Ng 则提到了“自主代理”(autonomous agents)。两者都认为 AI 的未来将更多地与人类协作,而不是完全自动化。
对于人工智能的商业基础,两位嘉宾持何种观点?
-两位嘉宾都认为人工智能的商业基础比以往任何时候都要强大,AI 正在成为推动下一轮数字革命或工业革命的真正转型驱动力。
Outlines
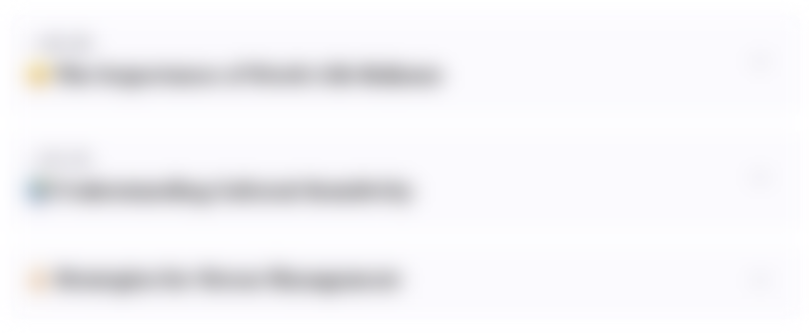
This section is available to paid users only. Please upgrade to access this part.
Upgrade NowMindmap
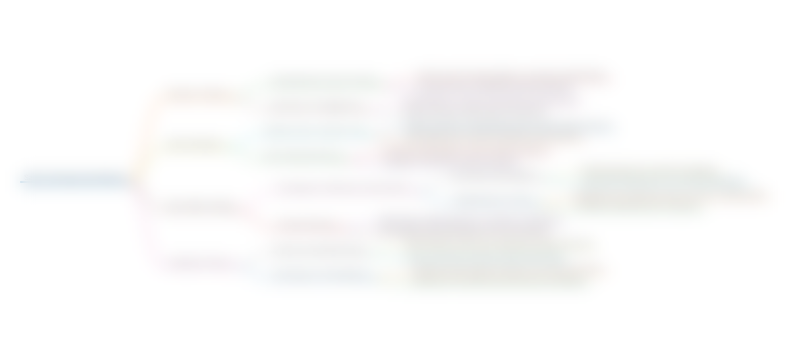
This section is available to paid users only. Please upgrade to access this part.
Upgrade NowKeywords
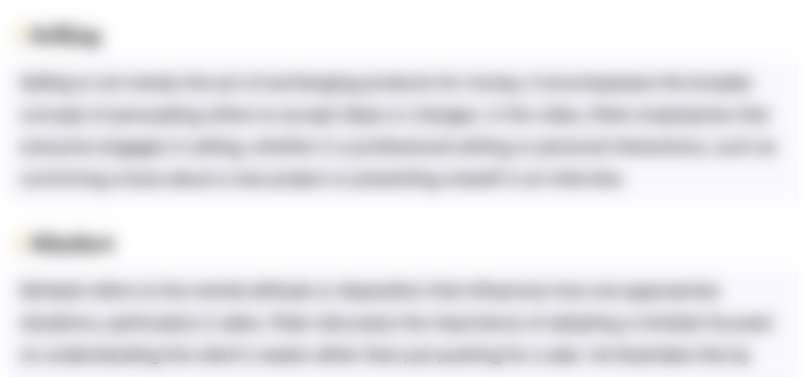
This section is available to paid users only. Please upgrade to access this part.
Upgrade NowHighlights
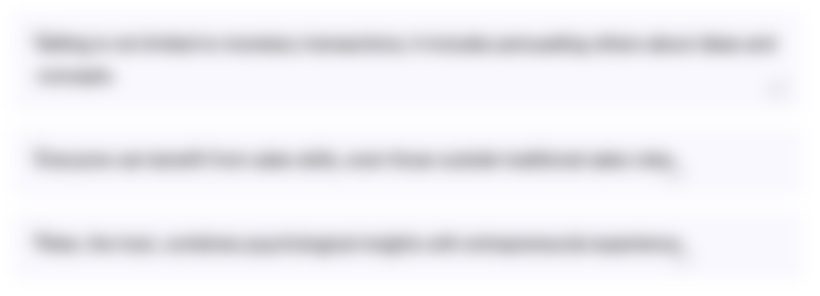
This section is available to paid users only. Please upgrade to access this part.
Upgrade NowTranscripts
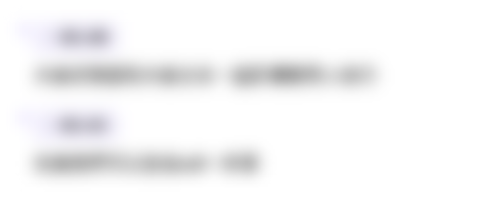
This section is available to paid users only. Please upgrade to access this part.
Upgrade NowBrowse More Related Video
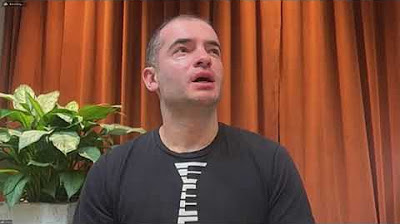
Interview with Dr. Ilya Sutskever, co-founder of OPEN AI - at the Open University studios - English
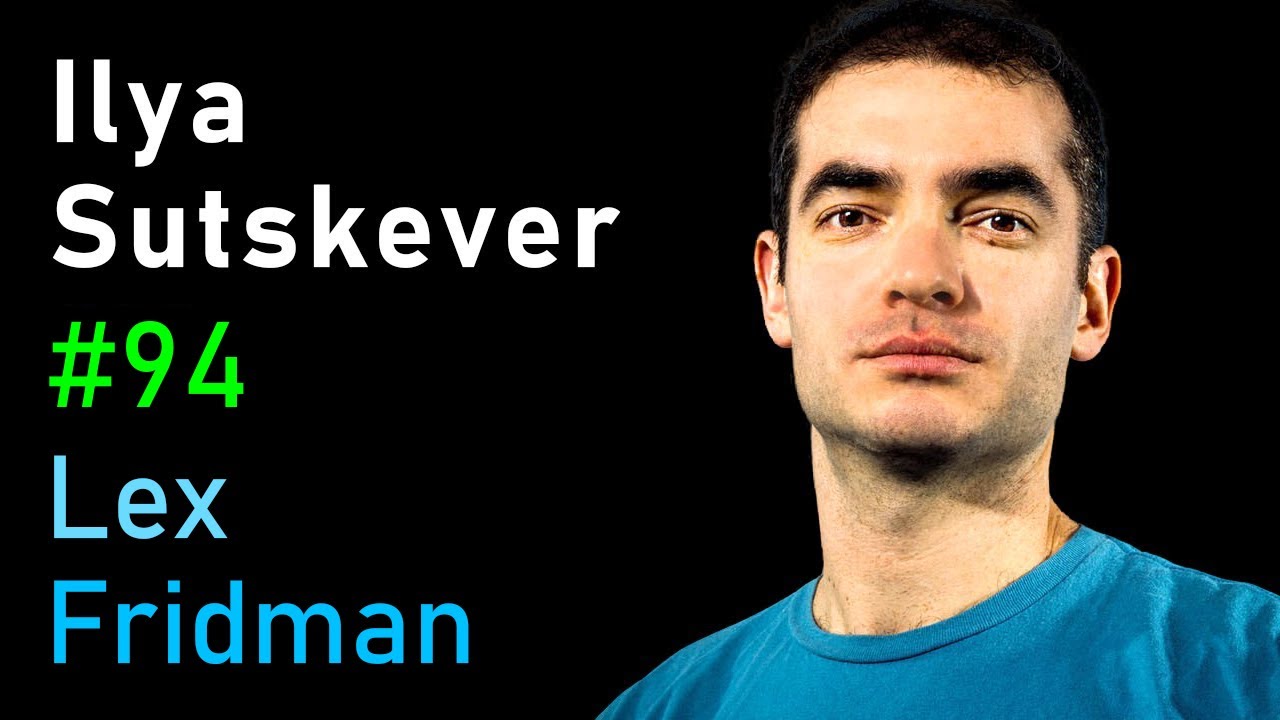
Ilya Sutskever: Deep Learning | Lex Fridman Podcast #94

What we see and what we value: AI with a human perspective—Fei-Fei Li (Stanford University)
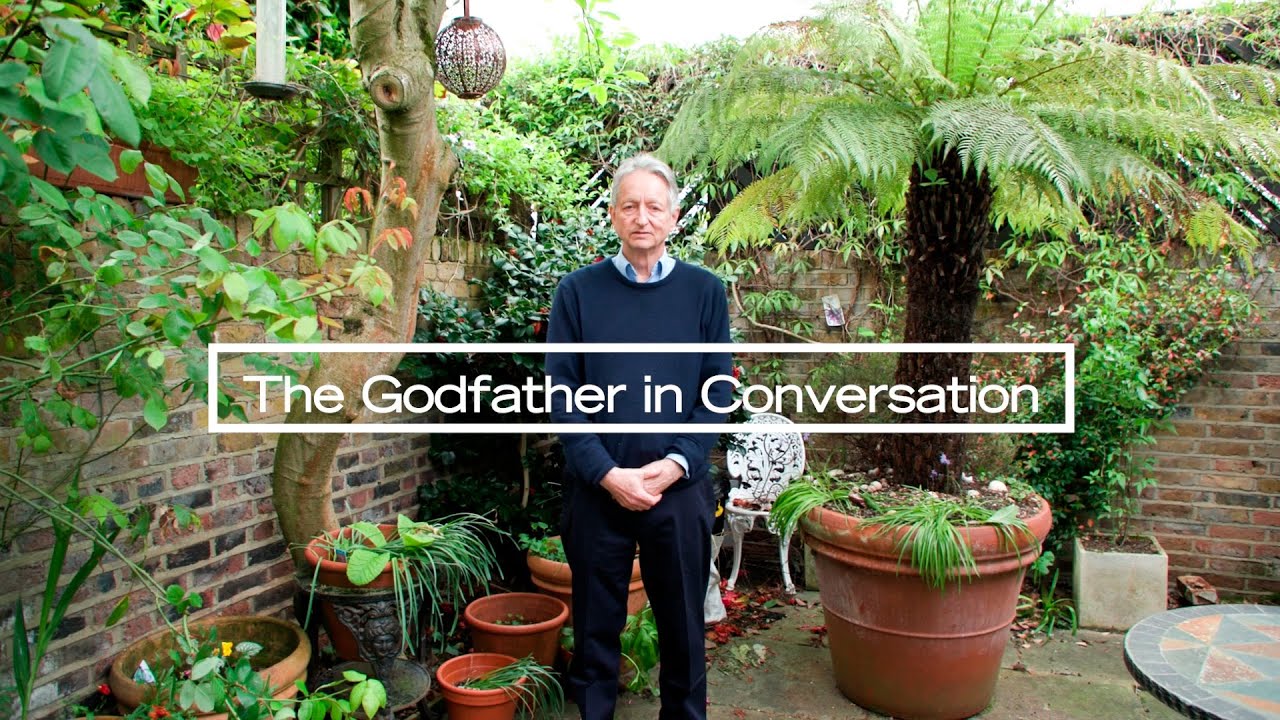
The Godfather in Conversation: Why Geoffrey Hinton is worried about the future of AI
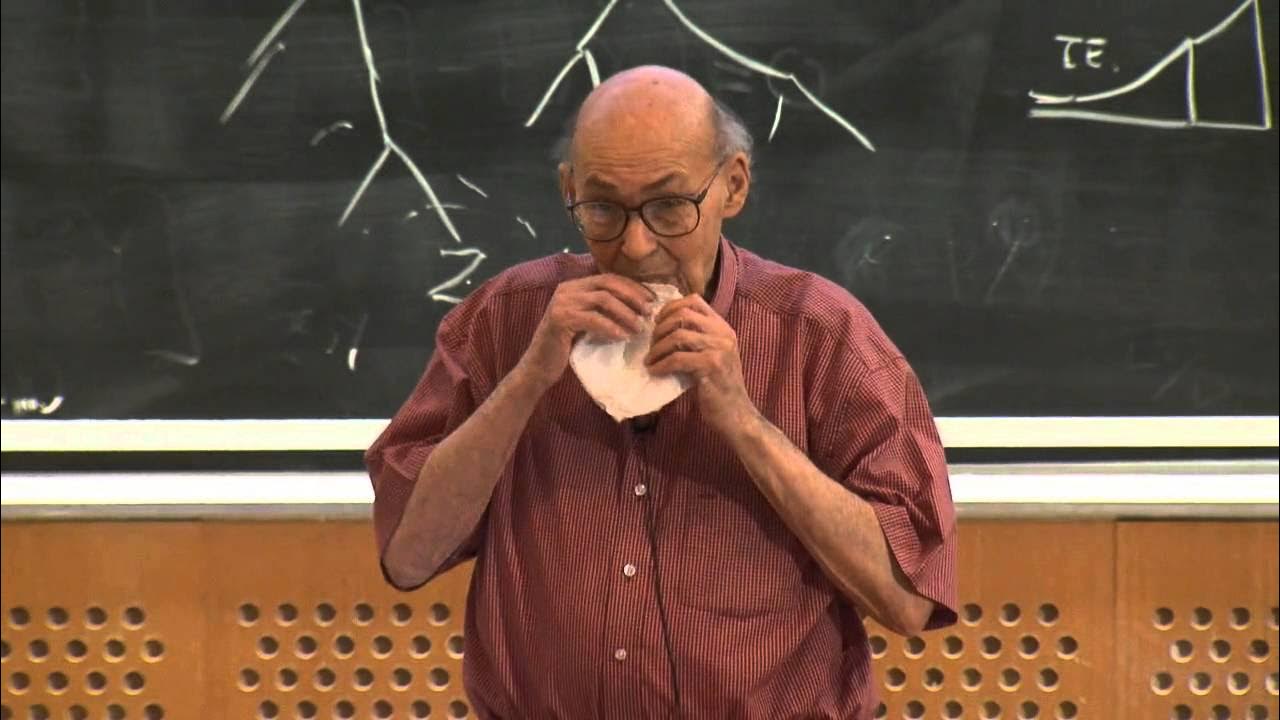
3. Cognitive Architectures
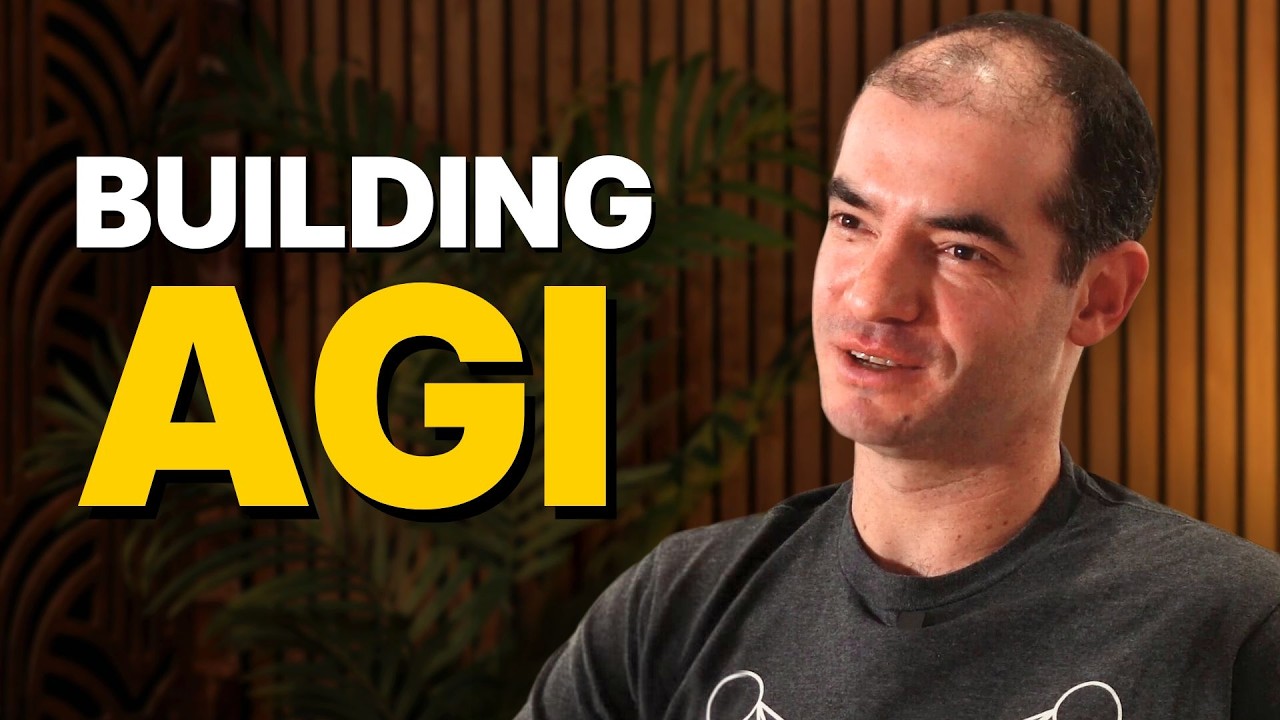
Ilya Sutskever (OpenAI Chief Scientist) - Building AGI, Alignment, Spies, Microsoft, & Enlightenment
5.0 / 5 (0 votes)