Regression and Matching | Causal Inference in Data Science Part 1
Summary
TLDRIn this video, the host interviews Yuan, a cognitive scientist, about causal inference in data science. They discuss why data scientists should learn causal inference, its importance beyond A/B testing, and how it can be applied to solve real-world problems. The conversation focuses on two key methods: regression and matching. They explore the challenges of using observational data, the concept of confounders, and the pitfalls of regression. The video also introduces propensity score matching as a solution to the limitations of traditional matching methods, with practical examples to illustrate its application.
Takeaways
- 🔍 Causal inference is crucial in data science for understanding 'why' behind observed effects, not just 'what'.
- 📈 Traditional A/B testing has limitations, such as in social media and marketplaces where interference between treatment and control groups can skew results.
- 🧐 Observational data can be used for causal inference but is prone to selection bias if not properly accounted for.
- 🤔 Understanding confounding variables is key; these are factors that affect both the treatment and the outcome, potentially leading to incorrect conclusions if not controlled.
- ⚖️ Regression analysis can be used to control for confounders by including them in a model to isolate the effect of the treatment on the outcome.
- ❗ Pitfalls of regression include overlooking important variables or incorrectly controlling for mediators and colliders, which can lead to spurious correlations.
- 🔄 Matching methods, such as propensity score matching, can handle various functional forms of confounder influences and are an alternative to regression.
- 📊 Propensity score matching involves predicting the probability of treatment and matching users based on this score to compare outcomes like engagement or conversion rates.
- 🛠 Careful model selection and algorithm design are essential for effective propensity score matching to ensure valid causal inferences.
- 📚 Further methods like difference in difference and synthetic control will be discussed in upcoming videos, promising stronger causal claims through different approaches.
Q & A
What is causal inference and why is it important in data science?
-Causal inference is a method used to determine the cause-and-effect relationship between variables. It's important in data science because it allows us to make predictions and decisions that are based on understanding why something happens, rather than just observing that it does.
Why might A/B testing not be effective in certain scenarios?
-A/B testing might not be effective when there is interference between the treatment and control groups, such as in social media platforms or marketplaces where users share a common pool of resources, leading to changes in supply and demand that can affect the outcome.
What is the role of confounders in causal inference?
-Confounders are variables that affect both the treatment and the outcome, potentially leading to biased conclusions if not accounted for. They need to be controlled for to ensure that any observed effects can be attributed to the treatment rather than other factors.
How does selection bias impact causal inference from observational data?
-Selection bias occurs when the groups being compared (e.g., exposed vs. unexposed to a treatment) differ systematically in ways other than the treatment itself. This can lead to incorrect conclusions about the effect of the treatment, as the differences observed might be due to these other factors rather than the treatment.
What is the regression method in causal inference and how does it work?
-The regression method involves fitting a statistical model that includes the treatment variable and the confounders. It aims to estimate the effect of the treatment on the outcome while holding the confounders constant, thus isolating the treatment's impact.
What are the potential pitfalls when using regression for causal inference?
-Pitfalls include not controlling for all relevant confounders or controlling for the wrong variables. Additionally, assuming the wrong form of relationships (e.g., linear when they are not) or controlling for mediators or colliders can lead to incorrect conclusions.
What is matching in causal inference, and how does it differ from regression?
-Matching involves finding pairs of treated and untreated units that are similar on key characteristics, to compare their outcomes and attribute differences to the treatment. Unlike regression, which statistically controls for confounders, matching creates comparable groups based on observed characteristics.
How does propensity score matching work and why is it useful?
-Propensity score matching involves predicting the probability of receiving the treatment based on observed characteristics and then matching individuals based on these scores. It's useful because it can control for a multitude of confounding factors by reducing them to a single score, simplifying the matching process.
What are the challenges associated with propensity score matching?
-Challenges include the need for an accurate model to predict propensity scores and the requirement for an efficient algorithm to find good matches. If either of these is not done well, the resulting matched groups may not be comparable, leading to invalid conclusions.
Can you provide an example use case for propensity score matching?
-A use case could be a data scientist at HelloFresh wanting to determine if users who click on ads are more likely to purchase due to the ad. Propensity score matching could be used to control for users' interests in cooking and compare the conversion rates of clickers and non-clickers.
What other causal inference methods will be discussed in future videos?
-Future videos will cover methods such as difference in differences and synthetic control, which are additional techniques for making causal inferences from observational data.
Outlines
🧐 Introduction to Causal Inference in Data Science
The video begins with a warm welcome and an introduction to the topic of causal inference in data science. The host explains that causal inference is crucial when A/B testing is not feasible and invites a cognitive scientist, Yuan, to share insights. Yuan discusses the importance of causal inference, emphasizing the need to understand 'why' behind product failures to prevent recurrence. The conversation highlights the limitations of A/B testing in certain scenarios, such as social media platforms and marketplaces, where interference between treatment and control groups can skew results. The host and Yuan agree on the necessity of alternative techniques for causal inference when traditional methods fall short.
🔍 Understanding Selection Bias and Confounders
Yuan explains the concept of selection bias and confounders, using the example of Facebook's new AI system for detecting harmful content. The discussion revolves around the challenge of measuring the impact of harmful content on user engagement due to personalized news feeds. Yuan clarifies that confounders are variables that affect both the treatment and the outcome, and their influence must be controlled to make accurate causal inferences. The conversation also touches on the difference between causal effects and mere observations, highlighting the importance of controlling for confounders to draw valid conclusions from observational data.
📊 Regression Method for Causal Inference
The conversation shifts to the regression method, a statistical approach to control for confounders. Yuan elaborates on how regression works by including treatment variables and confounders in a model to extract the partial slope of the treatment, which indicates the effect of the treatment on the outcome while holding other variables constant. The discussion points out potential pitfalls in using regression, such as not controlling for all relevant variables or controlling for the wrong ones. The importance of understanding the functional form of connections and the causal structure of variables is emphasized, with directed acyclic graphs (DAGs) introduced as a tool to represent these relationships.
🤝 Matching Method for Causal Inference
Yuan introduces the matching method as an alternative to regression, particularly useful for dealing with non-linear influences from confounders. The method involves finding untreated units that closely match treated units based on certain characteristics. The discussion addresses the challenges of matching, such as the curse of dimensionality and the difficulty of finding exact matches, especially with smaller datasets. To overcome these challenges, propensity score matching is introduced, which uses a model to predict the probability of treatment and then matches users based on this propensity score, simplifying the matching process and allowing for more efficient comparison of outcomes.
🛠️ Practical Use Cases and Summary of Causal Inference Methods
The final part of the conversation provides a practical use case for propensity score matching, illustrating how it can be used to evaluate the effectiveness of an ad campaign by HelloFresh. The example demonstrates how matching can help control for selection bias and provide a clearer picture of the ad's impact on user behavior. The host and Yuan summarize the key points of the video, reiterating the importance of controlling for confounders and the limitations of statistical control. They also hint at future discussions on other causal inference methods like difference in difference and synthetic control, promising more insights in upcoming videos.
Mindmap
Keywords
💡Causal Inference
💡A/B Testing
💡Confounders
💡Selection Bias
💡Regression
💡Matching
💡Propensity Score Matching
💡Counterfactuals
💡Natural Experiments
💡Harmful Content
Highlights
Causal inference is crucial in data science for understanding 'why' behind observed effects, not just 'what'.
Causal inference becomes essential when A/B testing is not feasible due to interference between treatment and control groups.
Yuan, a cognitive scientist, shares insights from data science interviews at DoorDash, Quora, and Meta.
Two primary causal inference methods discussed are regression and matching.
Regression is used to control for confounding variables and isolate the effect of a treatment.
Matching methods are an alternative when dealing with non-linear relationships or complex data sets.
Selection bias can lead to invalid conclusions if not properly accounted for in observational data.
Confounders are variables that affect both the treatment and outcome, requiring careful control.
Propensity score matching is introduced as a technique to deal with high-dimensional data and find comparable groups.
The importance of understanding the causal structure of variables is emphasized to avoid common pitfalls in regression analysis.
Mistakes in regression analysis, such as controlling for mediators or colliders, can lead to spurious correlations.
The limitations of regression in handling non-linear relationships and the benefits of matching methods are discussed.
Practical examples, such as measuring the impact of harmful content on user engagement, are used to illustrate causal inference methods.
The video concludes with a teaser for upcoming videos on other causal inference methods like difference in difference and synthetic control.
Yuan's blog post on the applications of causal inference in data science is recommended for further reading.
The video emphasizes the importance of causal inference in making data-driven decisions in various industries.
Transcripts
hey guys welcome back to the daily
interview pro channel in test video and
the next few videos we'll be talking
about causal inference in data science
causal inference has many applications
in data science and it becomes the go-to
method when ev testing is not working
under certain conditions
to give you guys an intuitive way to
understand the topic i have invited my
good friend yuan to share their
knowledge in this field
yuen is a cognitive scientist who
recently went through a few data science
interviews and has successfully landed
offers from door dash quora and meta
in this video we'll focus on two
commonly used causal inference methods
regression and matching we will go over
how to apply each method to solve actual
problems faced by tech comics be sure to
watch the entire video so you get not
only the fundamental knowledge and also
the applications let's get started
hey yun thank you for joining me today
i'm so excited about today's topic
causal inference and before we dive into
specific methods um i think it's helpful
that we start with the motivation of
learning causal inference
so
why should data scientists learn about
causal inference
yeah great question i can think of two
good reasons
um the first is that
which i heard from
a data scientist that lived sean taylor
that data scientists should do more than
just say
what killed a product we need to be able
to know why in order to prevent it from
happening again so if you're working for
netflix and notice that five percent of
the users churned uh last month
you need to use some causal inference to
know the reason so that
you won't have this
high level of churn rate in the future
and another is that um
so a b testing is usually the goat
standard for finding out causal
relationships but it does not always
work though
so can you give us some examples that
advertising is not working
yeah i actually learned a lot of
examples from your past videos so i
think people should really check those
out
so one typical example is social media
or communication software
so it's really weird that
if treatment
users have a new feature like reactions
and then they interact with control
users
um who don't have that feature
and another typical example is in
marketplaces like uber dash or airbnb so
users in each given market share the
same pool of
drivers dashers and the listings
so
the treatment can affect the control
users by changing the supply and demand
in the market
because of uh such interference between
the treatment and the control groups
it's hard to make causal claims uh about
the treatment effect
um for those reasons we need alternative
techniques to make causal inference
yeah makes sense so if advertising can
be tricky under certain conditions right
or for those business models can we use
uh observational data to draw
conclusions you know data that are not
generated from a b tests
yeah
you can do that but then you may suffer
from selection bias that may lead to
invalid conclusions let me tell you a
recent example that i saw in the news
just um
this month facebook launched a new ai
system that can quickly learn to detect
harmful content
including
hate speech harassment misinformation
you name it
so um
so quickly detecting hardware content is
important for um
facebook or meta users
because
this kind of content may negatively
impact user engagement
so
if you're a data scientist working for
mana how would you go about
measuring the impact of harmful content
on users
yeah very good question i guess uh a
simple way could be we you know identify
those users who were exposed to negative
content right and also those who were
not exposed to negative content and
maybe we could compare them in terms of
the
engagement metrics we care about right
such as time spent uh content
consumption or creation
or
user retention etc
okay that is a good start but here is a
problem though um as we all know
news feed is personalized for each user
based on
so-called ranking signals um like age
the country you live in the education
you receive
and how you interact with past content
recommended to you
so
for
users who are exposed to more harmful
content may differ from users who are
exposed to less
harmful content in terms of those
aspects
and also because of differences in those
aspects
the more exposed users may have
different english engagement levels
compared to the the less exposed users
anyways so it would be wrong for us to
conclude that it is exposure to harmful
content that affected
engagement
to summarize um so variables like um
like these ones that i just mentioned
can affect both the treatment and the
outcome so those variables are called
confounders and they are
the ones that we should watch out for um
so does that make sense
yeah that makes sense so
what is selection bias you have
mentioned earlier
um yeah let me explain this
so um
as mentioned what we can directly
observe from the data is the difference
between exposed users and unexposed
users in terms of their um
engagement metrics um that you talk
about right
but um as we talk about these are not
the same people so they may differ in a
lot more ways than
just whether or not they're exposed
harmful content so ideally
we need to um look at the same users and
imagine like in a parallel universe um
had they not been exposed to harmful
content how their engagement metrics
would have been
so um
what makes the
causal effect different from the
observation is the selection bias so
in this case
users who are exposed to more harmful
content may be more engaged anyways in
that case we have a positive selection
bias
and we may
falsely conclude that
harmful content
increases engagement
but um
it may be the other way around um so um
people exposed to more harmful content
may be less engaged anyways so then in
that case we may reach another false
conclusion that
harmful content hurts engagement
so
because of
the existence of selection bias
it is hard to make valid causal
inference from purely observational data
so both conclusion will be misleading
right uh so given that selection bias
exists how do we make causal inference
then
um so we can statistically control the
confounders that
i just described
by holding them at constant values and
see if the treatment still
affects the outcome or not
and
this can be done in two ways regression
and matching
all right so can you elaborate the
regression method
yeah for sure
so to use regression then we put
treatment variable out that we care
about in this case exposure to harmful
content together with
the confounders that we just mentioned
and then
we fit this model to data
and
they extract the partial slope of the
treatment so basically the slope tells
us
um when all the other variables are held
at constant values
how much um being exposed to harmful
content
affects engagement which is the outcome
that we care about and we can visualize
this using a type of plot
called a partial regression plot
so imagine that the confounders will be
held at constant values right so how do
we find those constant values
the short answer is that it doesn't
matter too much in practice so
with the models we don't
manually
assign constant values to co-founders
your estimator should be able to
take account of their influences
and when we interpret the models it also
doesn't matter
so we can plug in any ver any values for
those
variables that we control for
because
anything cancels out when we subtract
the
equation for the
untreated users from the equation
for the treated users
so
any other
things we need to pay attention to or
any pitfalls when we use regression
yeah there are loads
of those so um which i think boils down
to two things um so one mistake is to
con is not to control for all the things
that we should control for and the other
is controlling for like uh the wrong
variables
so um
as
to avoid the first mistake you need to
know at the main really well to
understand um like what factors are at
the play um in that domain
but then
as for the second one we should pay
attention to
how variables are connected and that has
two aspects
the first aspect is the so-called
functional form of the connections
so a quick example is that if you use
linear regression um to control for your
confounders
then
it can only control for confiners that
have linear relationships with the
outcome if your
co-founders have other types of
relationships
or even unknown relationships with the
outcome then linear regression does not
do the job but then the other um
aspect has to do with the causal
structure of how variables are connected
um and we can use directed acyclic
graphs or dags to represent causal
relationships
um so
it's a
complicated topic but today all you need
to know is that um in a dag
um nodes represent variables and edges
show their connections
so
we can
here is the doc that drew for the
harmful content case
so
here is one type of mistake
so
usually it's usually the case that the
more friends you have
the more um posts that you uh get shared
um
from your friends and then the more
likely it is that you may get exposed to
some kind of harmful content
we can so in this case um the number of
posts shared with you is a mediator
through which the size of your social
network
impacts your exposure to harmful content
so what happens when we control for
mediators for example we can look at
users who
get a ton of shared posts
then
we may like conclude that oh um users
with many friends uh have just um
um like as high a risk of being exposed
to um however content as users with few
friends
so um if we control for mediators then
we lose generating relationships in the
data
and here is another type of mistake that
you can make which is controlling for a
type of variables called colliders
so colliders are basically
common effects of other variables
so let me give you a concrete example
say
you look at if you
look at users who are super engaged late
at night right so
chances are
many of those users have a lot of
friends so they have a lot of people to
talk to like into the night and then
those users may be um may have insomnia
so they cannot fall asleep
so
so in this case uh like the time spent
uh late at night is a collider of the
number of friends you have and whether
or not you have insomnia
if you control if we control for this
collider by only looking at um
users who spend a lot of time on
facebook late at night then we may
conclude that oh popularity have has
something to do with insomnia
but that would be um a spurious
correlation that does not actually exist
so
in reality
it is hard to know what variables are
there that we should control for let
along how they are connected so using
regression blindly can be really
dangerous
yeah that makes sense
um yeah thank you so much for the uh
explanation of the pitfalls uh and you
mentioned another method matching right
so can we talk about um you know given
that we have regression why do we need
to know matching and how do we do it
yeah
so um i kind of answered the why
question like
when i just talked about regression so
um
we'll talk about like how linear
regression can only control for linear
influences from the co-founders but
matching can deal with any types of
functional forms
and
but the whole question is really tricky
so a quick answer is that um when using
matching or basically uh um for each uh
treated unit uh like an exposed user
we find one or several untreated units
in this case like unexposed users
and a match um then based on some
characteristics that we care about like
the ones we mentioned before
and then
um if we um
dare to make this assumption that those
users
are only different in terms of um
whether they get the treatment or not
but they are not different otherwise
then we can um
attribute the difference in their
outcomes in this case engagement
just to
the treatment
not to other things so that is the main
idea
of matching
yeah so the idea sounds reasonable to me
but i guess the question is how do we
actually match um like you mentioned
untreated units and treated units
yeah so that is the hard question so um
the most direct way to do this is that
we can
this is a toy dataset that i created for
the meta example
so we have a
group of exposed users and another group
of unexposed users
and we have like certain characteristics
that we um want to match them um
that we want to match them on right so
then
we can
find um exposed to users who have
exactly the same age
live in the same country
receive the same highest degree
as those unexposed users
so in this case
like user 3 can be
matched to user 9 because they have the
same values
for those variables that we want to
control for and so is
user 6 um
that is matched to user 15. so um this
method can be quite painful first of all
it suffers from the so-called cursive
dimensionality um because we need to go
through the matching process as many
times as there are variables that we
want to control for
and then
unless we have a really big data set um
which i think is no problem for meta but
maybe
but it may be a problem for smaller
companies with smaller data sets it can
be hard to find um
enough exact matches that we can use for
comparison later
so sounds like for each data point we
have we need to find its pair right and
that's why
we will have less data that could be
used to draw conclusions
so is there any method we could use to
address this kind of problem
yeah we can use
propensity score matching so basically
the idea is that instead of matching
users directly based on those
characteristics
we
build a model
that takes those characteristics about
the users to predict
the probability that they might be
exposed to harmful content so this
predicted probability is called a
propensity score
for each user so
instead of going through the matching
process many many times
we just match unexposed users
to exposed users based on a single
propensity score and then
we can
and then after users are matched then we
can compare their engagement levels to
see
if
there's any difference that we can
attribute to the treatment
so
this method
solves the two problems that i just
mentioned but
it it's also very challenging so first
of all we need a good model that can
accurately predict
the propensity to receive the treatment
in this
case the probability of being exposed
harmful content and the second of all we
need a good algorithm that um
that can quickly find um
similar users um in terms of their
propensity scores
so um
um
both are really demanding and if either
goes wrong then um
we cannot find comparable groups that we
can draw valid conclusions based on
yeah thank you for explaining that so
given that both the prediction and the
matching part are pretty challenging um
so what are the use cases of this
particular method can you give us some
examples
so let's say like you're a data
scientist working at like hellofresh and
then you want to see you put out a
um ad campaign like
for the food that you're selling and
then you want to know if
um
people who click on the ads are more
likely to buy food
from you because of this ad or not all
right so um because of the selection
bias that we mentioned it can be um
misleading just to compare uh people who
click on the ad and um who don't and
compare their conversion rates because
people who
um
because just like um
news feed ads are also personalized so
um
users who are shown this ad may have
higher interests in cooking anyways so
they um may be more likely to buy foot
from you
without a width or without clicking on
this ad
so a better way to answer that question
would be using propensity score matching
um so first um we use uh characteristics
of the users to predict
in this case it's uh the prediction is
more complex because we need to predict
how likely it is that a user is shown
this ad and then after being shown this
ad
how likely it is that they're going to
click on it um so then
um after
generating this propensity score for
your users then you can match
the clickers and non-clickers on this
propensity score and compare their
conversion rates
so that's just another example but there
are many many user use cases that you
can imagine so
despite the difficulty it is a it's
still a
very
popular method for causal inference
yeah thank you for the example so
could you summarize what we have learned
the two methods we have learned in this
video and you know to help our audience
to learn them better
yeah sure um so basically we learned
that when the data is purely
observational
it is dangerous to
draw causal conclusions because of the
selection bias that we mentioned so to
solve that problem we can use
regression or matching to control for a
type of variables called co-founders
that can affect both the treatment and
the outcome
so after um confounders are controlled
for then we can be more confident
whether or not the treatment has
a true effect
on the outcome
um
but um
[Music]
but
overall um because of
various problems that we just mentioned
statistical control only allows us to
make a
pretty weak causal influence so um
methods based on counterfactuals and
natural experiments can help us
make stronger causal claims and we will
talk about these methods later
sounds good thank you so much
alright guys thanks for watching if you
want to learn more about applications of
causal influence in data science yuen
has a great blog post about it feel free
to check it out
in the next few videos you and i will
talk about other common use causal
inference methods in data science such
as difference in difference and
synthetic control stay tuned we will see
you soon
浏览更多相关视频
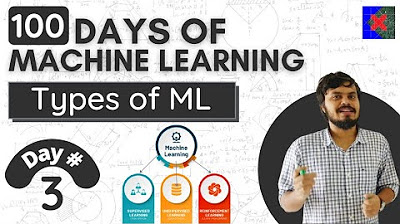
Types of Machine Learning for Beginners | Types of Machine learning in Hindi | Types of ML in Depth
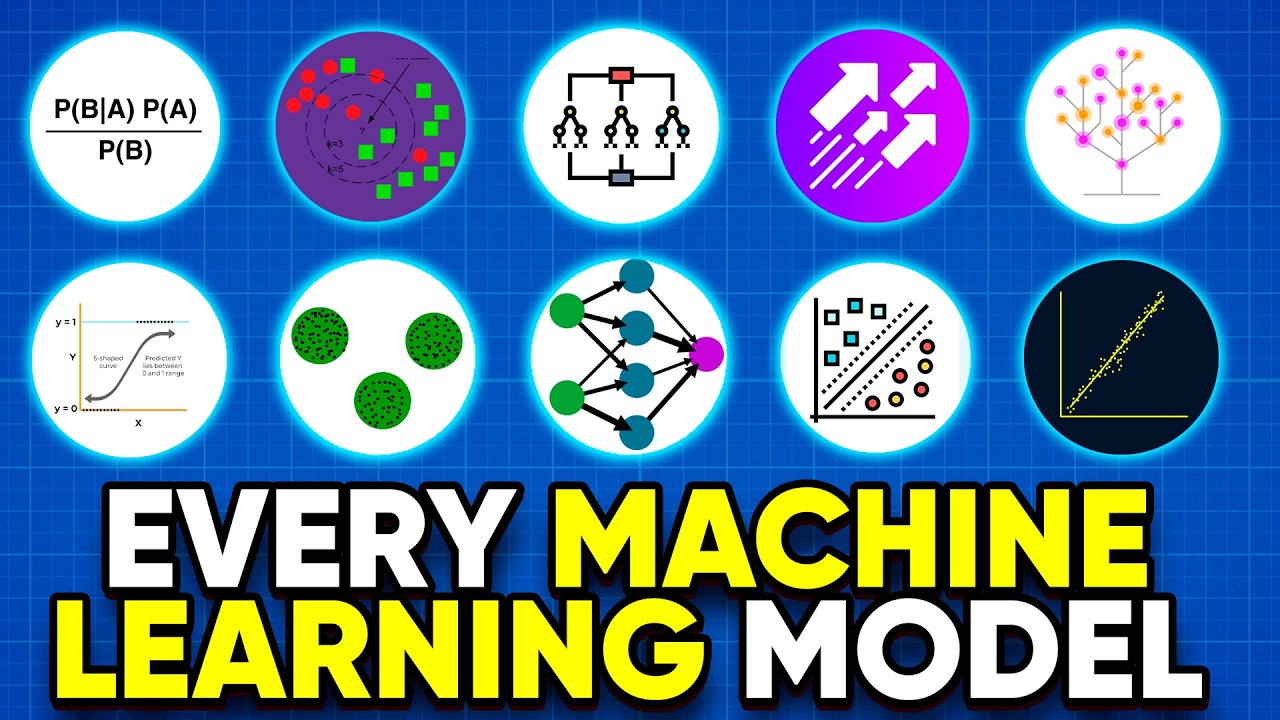
All Machine Learning algorithms explained in 17 min

100+ Statistics Concepts You Should Know

Data Collection Stratergy For Machine Learning Projects With API's- RapidAPI
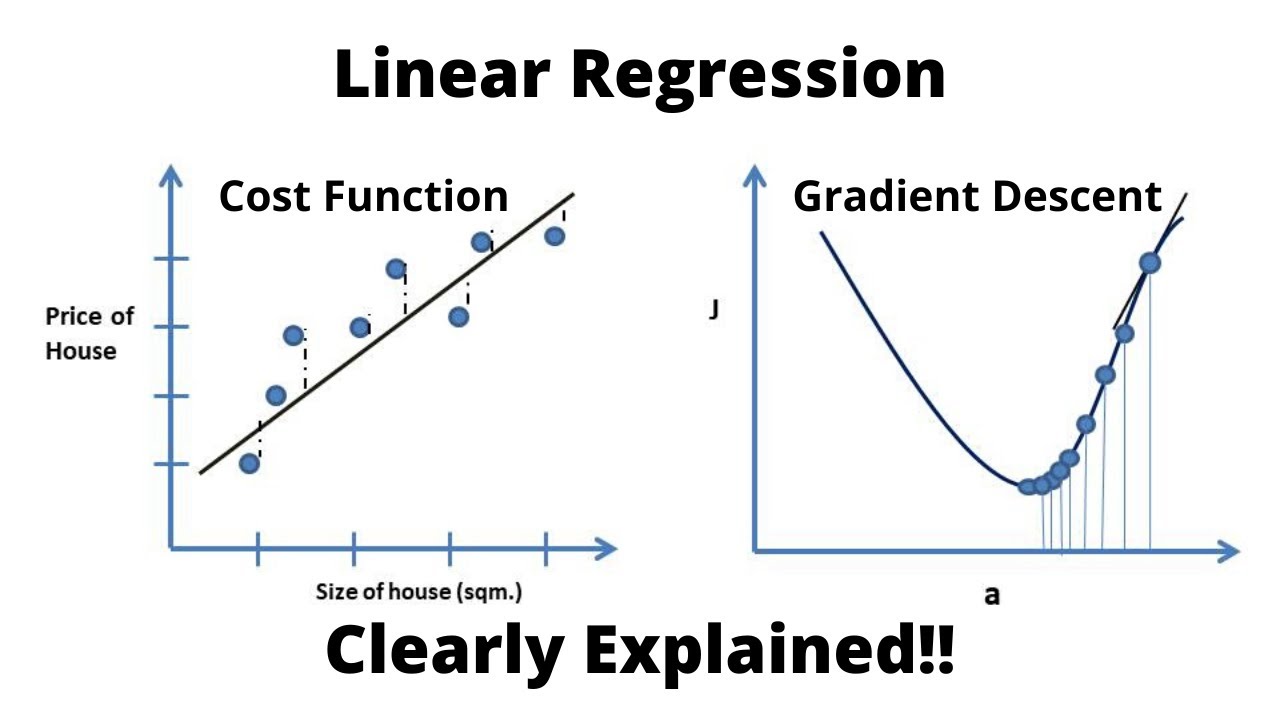
Linear Regression, Cost Function and Gradient Descent Algorithm..Clearly Explained !!

Lec-10: Foreign Key in DBMS | Full Concept with examples | DBMS in Hindi
5.0 / 5 (0 votes)