The most important ideas in modern statistics
Summary
TLDRThis video script discusses eight revolutionary ideas in statistics that have shaped the field from 1970 to 2021. It highlights the importance of counterfactual causal inference, the bootstrap method, simulation-based inference, overparameterization, regularization techniques, multi-level models, and the role of computational power. The script emphasizes the evolution of statistical practice, the significance of robust inference, and the innovative use of plots and visuals for data analysis. It also touches on the concept of adaptive decision analysis and the impact of robust statistics in providing trustworthy analyses despite potential assumption violations.
Takeaways
- 📊 Statistics is an evolving field with influential ideas shaping its trajectory.
- 📈 Andrew Gelman and Aki Vehtari are authorities in Bayesian statistics, known for their work on Bayesian data analysis.
- 🔍 The concept of counterfactual causal inference allows making causal statements from observational data.
- 🔄 The bootstrap method is a versatile algorithm for estimating the sample distribution of a statistic using a single dataset.
- 💻 The rise of computational power has highlighted the importance of computation in statistics, enabling complex simulations and analyses.
- 🔧 Overparameterized models and neural networks offer extreme flexibility in modeling a wide range of phenomena.
- 🔧 Regularization techniques are used to prevent overfitting in flexible models by maintaining a degree of simplicity.
- 📈 Multi-level models, also known as hierarchical or mixed effect models, are used to aggregate information and provide more nuanced analyses.
- 🔄 The Expectation-Maximization (EM) algorithm and the Metropolis algorithm are key statistical algorithms that address complex estimation problems.
- 🔧 Adaptive decision analysis allows for the modification of experiments based on interim data, improving the design and decision-making process.
- 🔍 Robust inference provides trustworthy statistical analyses even when assumptions are violated, offering more confidence in the results.
Q & A
What is the main focus of the video?
-The video discusses eight innovations in statistics that have significantly shaped the field, making it accessible to a general audience.
Who are Andrew Gelman and Aki Vitter, and why are they considered authorities in statistics?
-Andrew Gelman and Aki Vitter are renowned statisticians known for their work in Bayesian statistics. They are considered authorities because they have written extensively on Bayesian data analysis, which is highly respected among practitioners.
What is the difference between experimental data and observational data in statistics?
-Experimental data comes from controlled experiments where researchers can manipulate variables, allowing for causal claims. Observational data, on the other hand, comes from observing real-world scenarios where researchers cannot control who receives a treatment, limiting them to making only correlational claims.
How does counterfactual causal inference help in dealing with observational data?
-Counterfactual causal inference allows statisticians to make adjustments to observational data, getting closer to causal statements by considering what would have happened in an alternate reality where the treatment was not applied.
What is the bootstrap method and why is it significant?
-The bootstrap is an algorithm for estimating the sample distribution of a statistic by resampling with replacement from the original data set. It is significant because it simplifies the process of creating confidence intervals and is applicable to many kinds of statistics, highlighting the importance of computation in statistics.
What is the role of simulations in statistics?
-Simulations allow statisticians to assess experiments and new statistical models without actually conducting them, saving resources and time. They can be used to evaluate the power and type I error of experimental designs, such as clinical trials.
Why is increasing the number of parameters in a statistical model beneficial?
-Increasing the number of parameters in a model provides more flexibility, allowing it to better represent complex real-world scenarios. This can lead to more accurate predictions and a better understanding of the data.
What is regularization in the context of statistical models?
-Regularization is a technique used to prevent overfitting in extremely flexible models by enforcing simplicity. It helps balance the complexity of the model, ensuring it does not just approximate the data but represents a more general phenomenon.
How do multi-level models differ from simpler statistical models?
-Multi-level models, also known as hierarchical or mixed effect models, assume additional structure over the parameters, allowing for the aggregation of data from different levels or groups. This structure is useful for combining information from various sources and can be applied in both frequentist and Bayesian frameworks.
What is the expectation maximization (EM) algorithm and its significance?
-The EM algorithm is a statistical algorithm used for estimation problems, particularly when the model contains latent variables or unobserved data. It allows for the estimation of parameters in complex models that cannot be solved directly.
What is robust inference and its importance in statistics?
-Robust inference provides trustworthy statistical analyses even when assumptions are violated. It ensures that the results of statistical analyses, such as confidence intervals or estimated values, remain reliable even if the underlying assumptions are not perfectly met.
Outlines
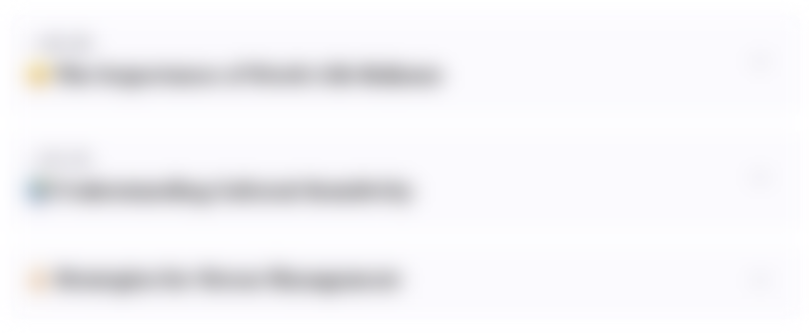
This section is available to paid users only. Please upgrade to access this part.
Upgrade NowMindmap
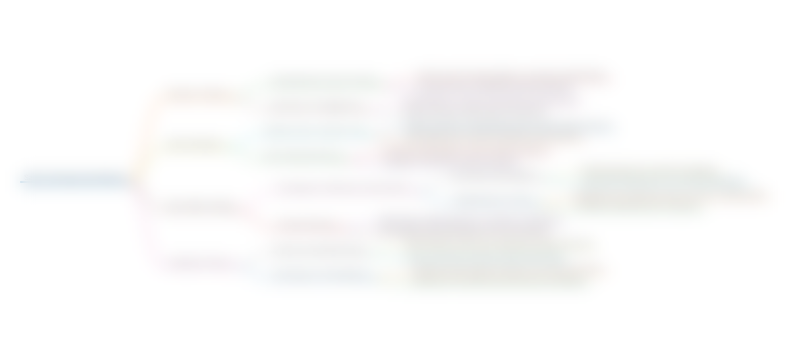
This section is available to paid users only. Please upgrade to access this part.
Upgrade NowKeywords
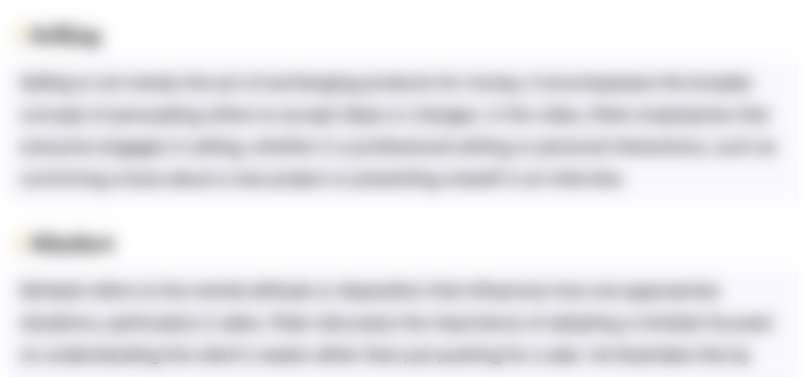
This section is available to paid users only. Please upgrade to access this part.
Upgrade NowHighlights
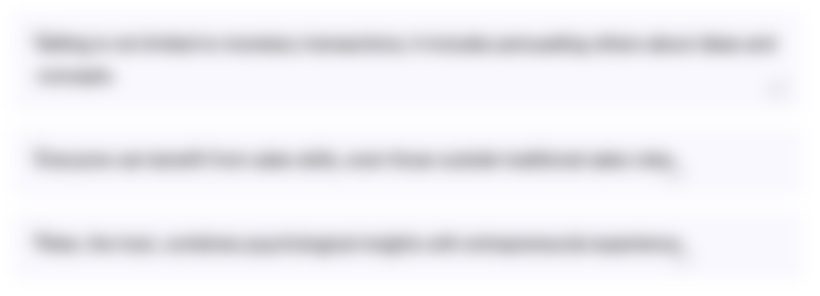
This section is available to paid users only. Please upgrade to access this part.
Upgrade NowTranscripts
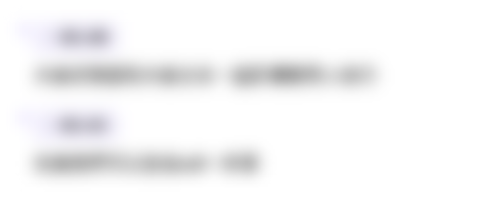
This section is available to paid users only. Please upgrade to access this part.
Upgrade Now5.0 / 5 (0 votes)