Trust Nothing - Introducing EMO: AI Making Anyone Say Anything
Summary
TLDR元のスクリプトの日本語翻訳を要約した魅力的な内容。簡潔で正確な概要を提供し、ユーザーの興味を引きつけます。
Takeaways
- 😮 オンラインで見るものが本当かどうかをもはや信じられなくなる時代が来ている。
- 🤖「emo」は、写真とオーディオから人が歌っているように見せる技術を実現している。
- 🎨 この技術は、リアルな顔の表情や頭の動きを合成し、非常にリアルなビデオを生成する。
- 🔍 AI生成の女性やモナリザなど、様々な基本画像にこの技術を適用できる。
- 📈 音声入力と顔の動きを結びつけることの困難さを克服している。
- 🚀 「Grock」は、言語処理ユニット(LPU)を使用して、AIとの高速な対話を可能にする。
- 💡 NvidiaのCEOは、将来的にはプログラミングではなく問題解決能力がより重要になると主張している。
- 📚 AIの進化により、自然言語がコンピューターとのコミュニケーションの主流になりつつある。
- 🌐 「emo」プロジェクトは、オーディオとビデオの融合モデルを利用している。
- 📖 この技術は、顔の表情や動きをリアルに再現するために、250時間以上の映像と1億5000万枚以上の画像からなる広範なデータセットを使用して訓練されている。
Q & A
AlibabaグループのEmoとは何ですか?
-Emoは、画像とオーディオ(音声や歌)をアップロードして、その人物が話しているか歌っているように見せることができる技術です。
Emoが生成する動画の特徴は何ですか?
-Emoによって生成される動画は、単に口元の動きだけでなく、顔の表情や頭の傾きの変化も含まれ、リアルな表現が可能です。
Emoの動画生成プロセスにおける難しさは何ですか?
-オーディオから顔の表情へのマッピングの曖昧さがあり、これを解決することが技術的な課題です。
Grockとは何ですか?
-Grockは、言語処理ユニット(LPU)を搭載した、大規模言語モデルと生成AIのための新しいアーキテクチャです。
Grockの特徴は何ですか?
-Grockは、他のどのシステムよりも速い、500トークン以上/秒の推論速度を誇ります。
プログラミングが「死ぬ」とはどういう意味ですか?
-プログラミングが「死ぬ」とは、将来的にはプログラミングのスキルよりも問題解決能力や大言語モデルを使いこなす能力が重要になるという意味です。
NVIDIAのCEO、Jensen Huangはプログラミング教育についてどのような見解を持っていますか?
-彼は、将来的には誰もが自然言語を使ってプログラミングできるようになるべきであり、特定のドメインの問題解決がより重要になると主張しています。
Emoプロジェクトと大言語モデルの関係は何ですか?
-Emoプロジェクトは、AI技術の進化とともに、リアルなビデオ生成が容易になっている一例であり、大言語モデルは自然言語でのコミュニケーション能力を向上させます。
Emoによる動画生成の制約は何ですか?
-生成過程での安定性の問題や、顔以外の体の部分の不自然な動きが挙げられます。
Emoの訓練データはどのようなものですか?
-250時間以上の映像と1億5000万枚以上の画像からなる、多言語かつ多様なコンテンツを含む広範なデータセットです。
Outlines
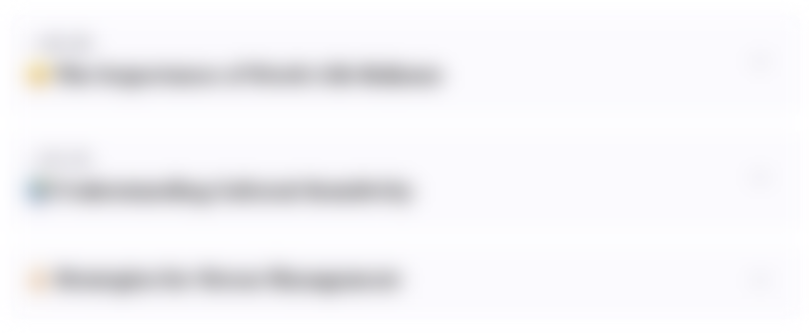
This section is available to paid users only. Please upgrade to access this part.
Upgrade NowMindmap
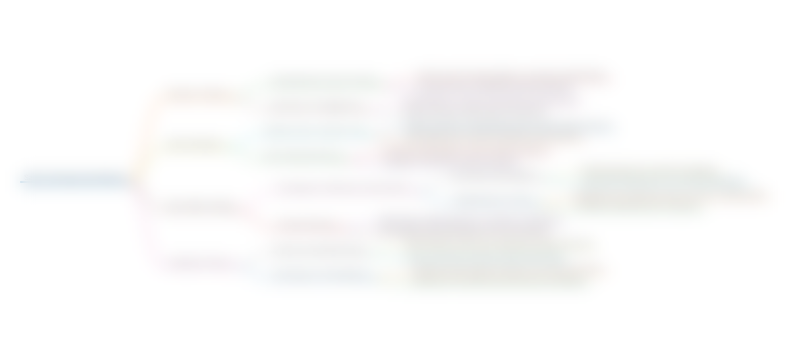
This section is available to paid users only. Please upgrade to access this part.
Upgrade NowKeywords
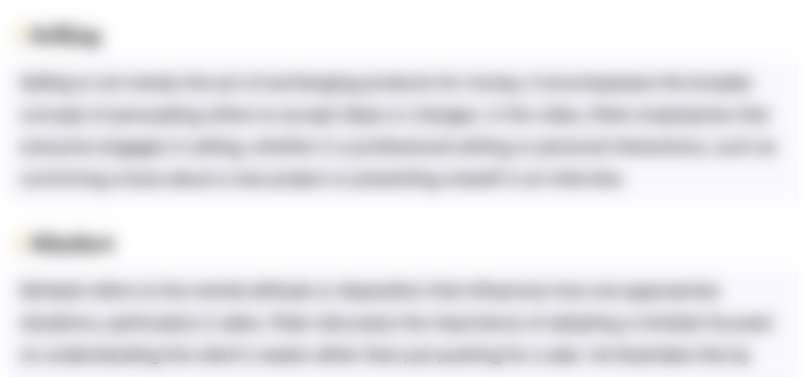
This section is available to paid users only. Please upgrade to access this part.
Upgrade NowHighlights
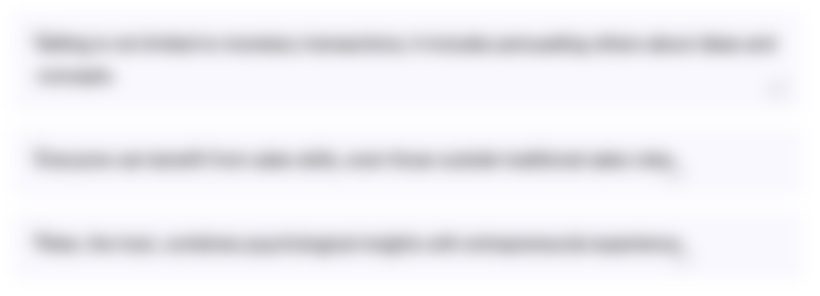
This section is available to paid users only. Please upgrade to access this part.
Upgrade NowTranscripts
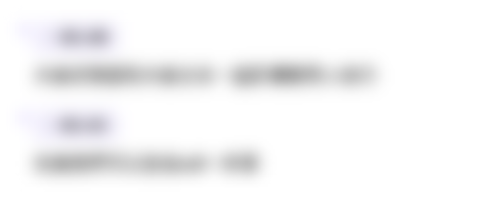
This section is available to paid users only. Please upgrade to access this part.
Upgrade NowBrowse More Related Video
5.0 / 5 (0 votes)