MINI-LESSON 6: Fooled by Metrics (Correlation)
Summary
TLDRThe video script delves into the pitfalls of relying on metrics, highlighting two main issues: the randomness of metrics and the tendency to select the most favorable data. It uses the example of Pearson correlation to illustrate how even with independent variables, correlation coefficients can deviate from zero, emphasizing the stochastic nature of such measures. The script further discusses how researchers might manipulate data to find the 'best' correlation, leading to misleading conclusions. It also touches on the problem of dimensionality, where the number of possible correlations grows exponentially with the number of variables, exacerbating the risk of spurious correlations. The speaker critiques certain fields of research for their misuse of statistical methods, advocating for a more cautious interpretation of data.
Takeaways
- 📊 Metrics are random variables: They aren't fixed and can vary widely.
- 🔍 Correlations aren't always reliable: Observing a correlation doesn't guarantee a true relationship.
- 🎲 Metrics can be manipulated: Researchers can pick the highest correlation among many to fit their narrative.
- 📉 Example of randomness: Using random variables can yield different correlations each time, often deviating significantly from zero.
- 📐 Distribution matters: With more data points, the distribution of correlation compresses, lowering standard deviation.
- 🔍 Misleading correlations: Some studies show correlations between unrelated variables, like US spending on science and suicides.
- 📈 Choosing the best correlation: Researchers can exploit multiple correlations to find and report the highest one.
- 🎛️ Importance of large sample sizes: More data points reduce the randomness and improve reliability of correlations.
- 📊 Dimensionality problem: Increasing the number of variables leads to exponentially more correlations, increasing the chance of spurious findings.
- 🧮 Spurious correlations: High numbers of correlations in large datasets can lead to false interpretations if not carefully managed.
Q & A
What are the two main points discussed in the transcript about metrics?
-The two main points discussed are: 1) Metrics are random variables, and 2) Metrics can be gamed because they are random variables. People often take the upper bound of these metrics, leading to misleading conclusions.
Why does the speaker compare metrics to random variables?
-The speaker compares metrics to random variables to highlight that metrics are not fixed values but can vary based on different observations. This variability can lead to different outcomes when metrics like correlation are calculated, even if the underlying variables are independent.
How does the speaker illustrate the randomness of correlation?
-The speaker uses an example with two independent random variables, X and Y, and shows that calculating Pearson correlation multiple times results in different values each time. This illustrates that correlation is a stochastic variable and not a fixed measure.
What does the speaker mean by 'metrics will be gamed'?
-The speaker means that researchers or analysts might selectively choose metrics or correlations that show the most favorable or extreme results, thereby misleadingly representing the data. This is possible because metrics, being random variables, can produce varying results, allowing for cherry-picking.
What is the significance of the 'law of large numbers' in the context of metrics?
-The 'law of large numbers' is mentioned to explain that as the sample size increases, the distribution of the correlation becomes more compressed, leading to a more stable and less variable estimate of the correlation. This helps in reducing the randomness but doesn't eliminate it completely.
What example does the speaker give to show how researchers can misuse correlations?
-The speaker gives examples of absurd correlations, such as the relationship between U.S. spending on science and technology and the number of suicides by hanging, to illustrate how researchers can misuse correlations by selecting extreme or coincidental relationships that appear significant but are actually meaningless.
What is the speaker's criticism of certain fields like psychology and political science?
-The speaker criticizes these fields for relying on metrics and correlations without sufficient scrutiny, leading to findings that are often spurious or meaningless. The speaker argues that these fields produce data that are frequently unreliable because they do not account for the random nature of the metrics they use.
How does the speaker suggest testing the randomness of metrics?
-The speaker suggests using Monte Carlo simulations to replicate random datasets and observe the correlations or slopes that emerge. This method helps demonstrate how entirely random data can still produce seemingly significant correlations, underscoring the importance of skepticism in interpreting such metrics.
What problem does the speaker highlight regarding the number of random variables in a dataset?
-The speaker highlights that as the number of random variables (p) increases, the number of possible correlations (which grows at a rate of p squared) increases significantly. This leads to a higher chance of finding spurious correlations, making it challenging to identify genuine relationships without a large enough sample size (n) to compensate for this complexity.
What advice does the speaker offer for interpreting correlations or slopes in research?
-The speaker advises that correlations and slopes should be interpreted with scrutiny. A small correlation or slope is much closer to zero than it might appear, and researchers should be cautious about drawing strong conclusions from these metrics without considering the potential for randomness and spuriousness.
Outlines
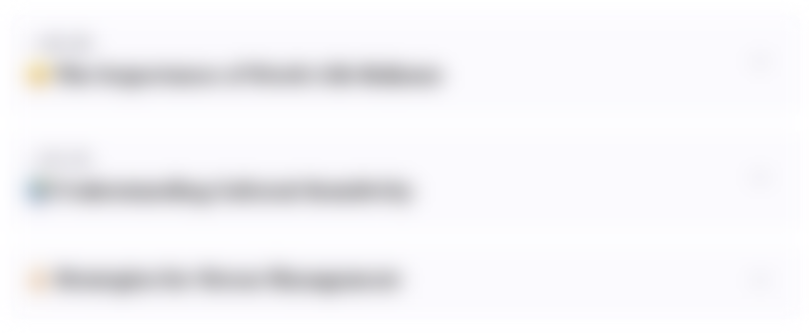
This section is available to paid users only. Please upgrade to access this part.
Upgrade NowMindmap
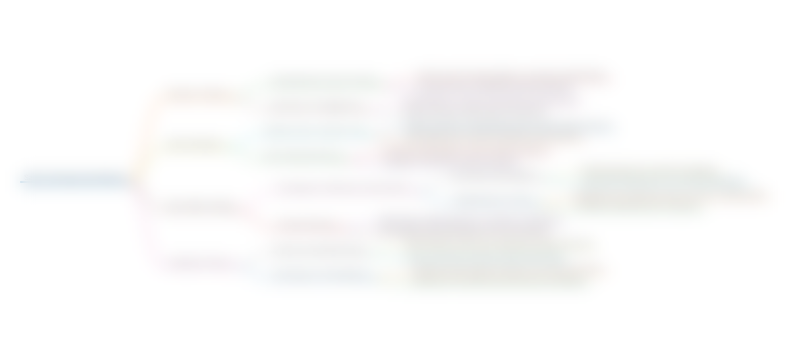
This section is available to paid users only. Please upgrade to access this part.
Upgrade NowKeywords
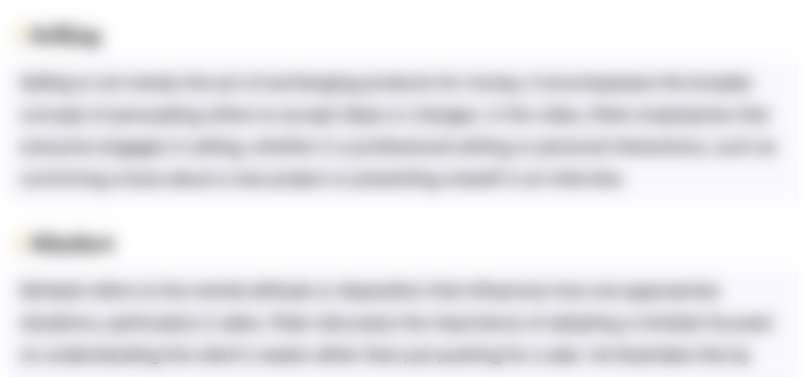
This section is available to paid users only. Please upgrade to access this part.
Upgrade NowHighlights
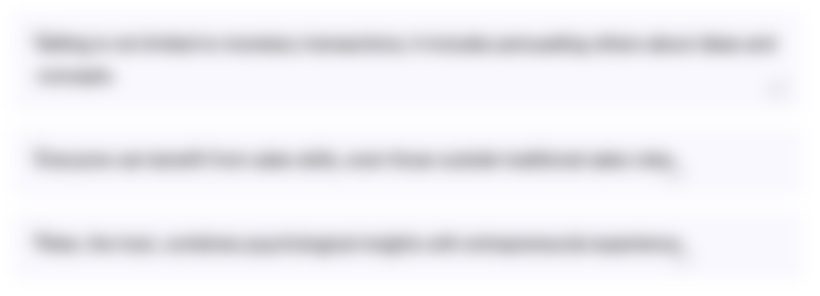
This section is available to paid users only. Please upgrade to access this part.
Upgrade NowTranscripts
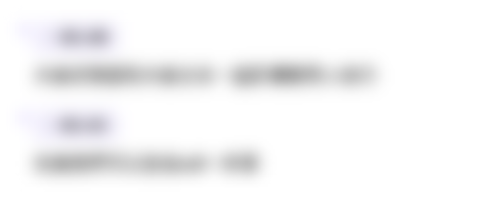
This section is available to paid users only. Please upgrade to access this part.
Upgrade NowBrowse More Related Video

Measures of Variability (Range, Standard Deviation, Variance)
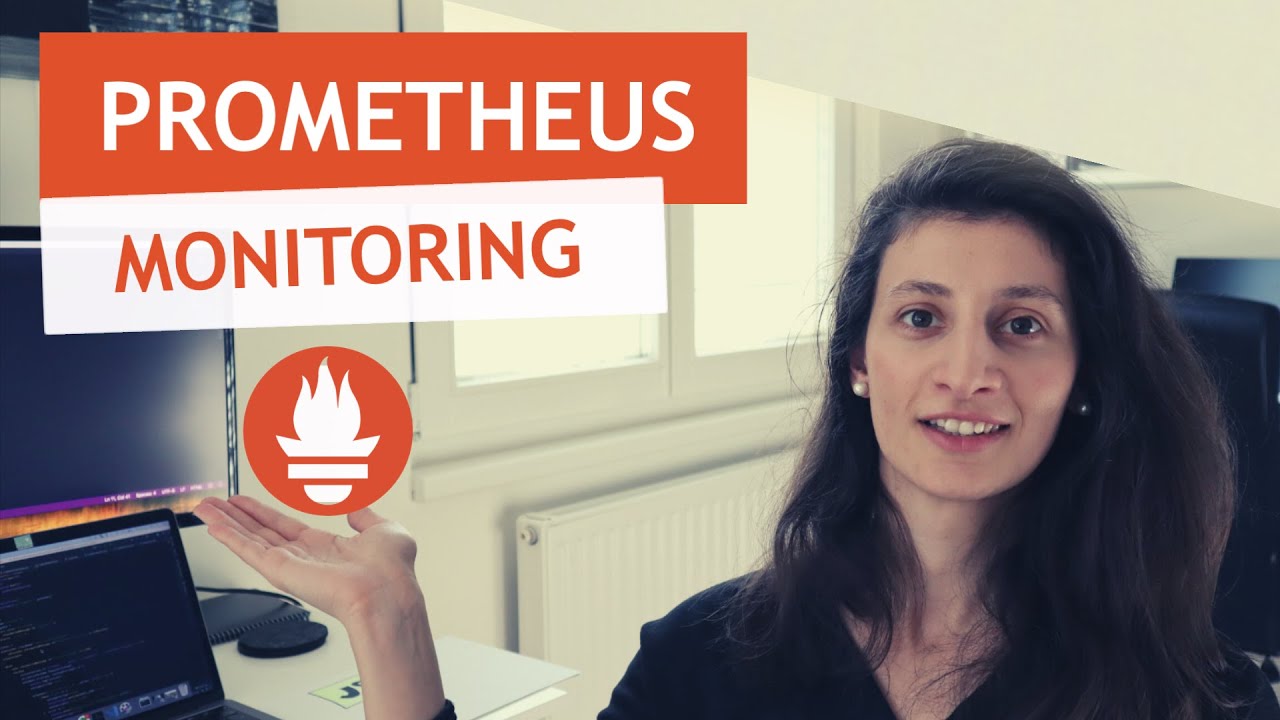
How Prometheus Monitoring works | Prometheus Architecture explained
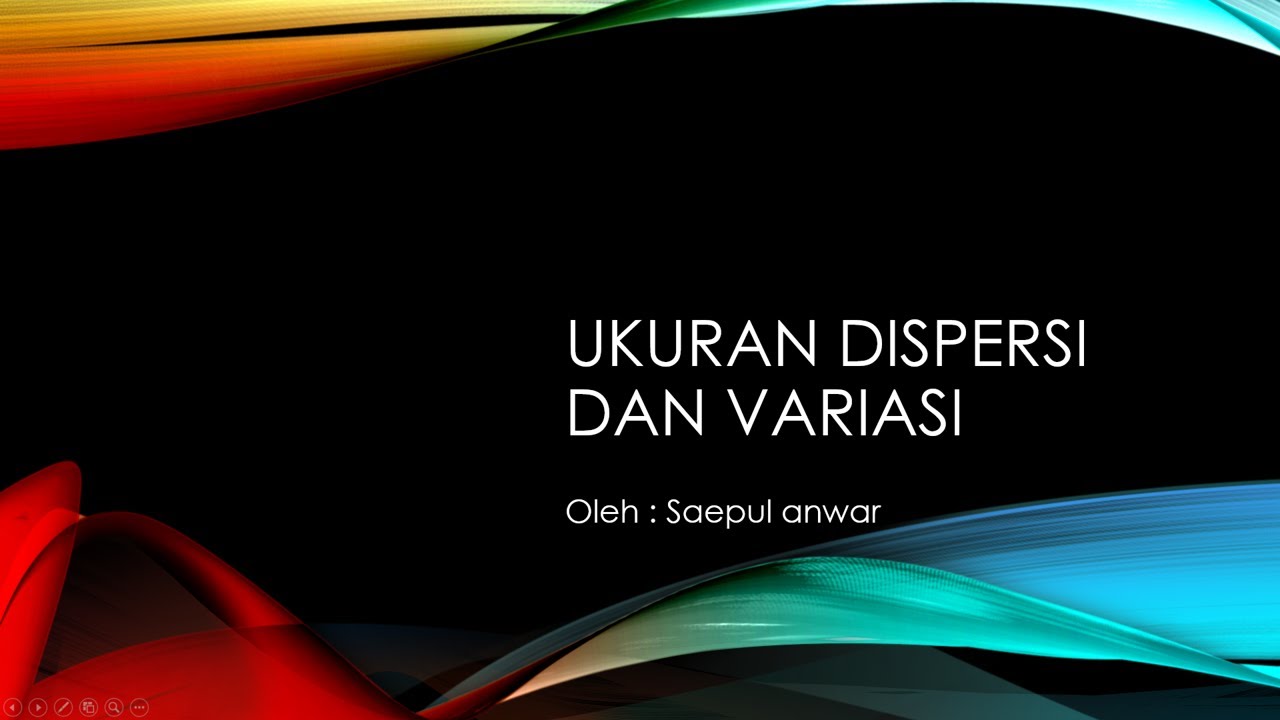
Ukuran Dispersi dan Variasi
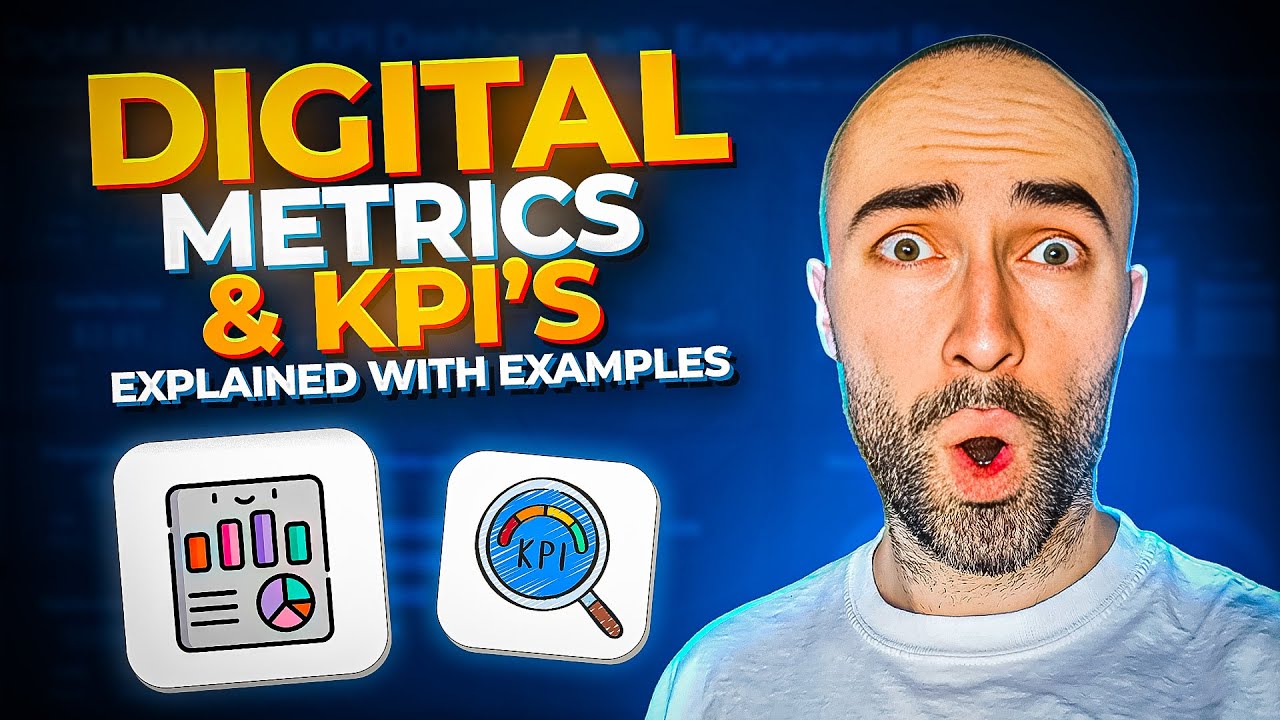
Digital Marketing Metrics & KPI's Explained (With Examples)
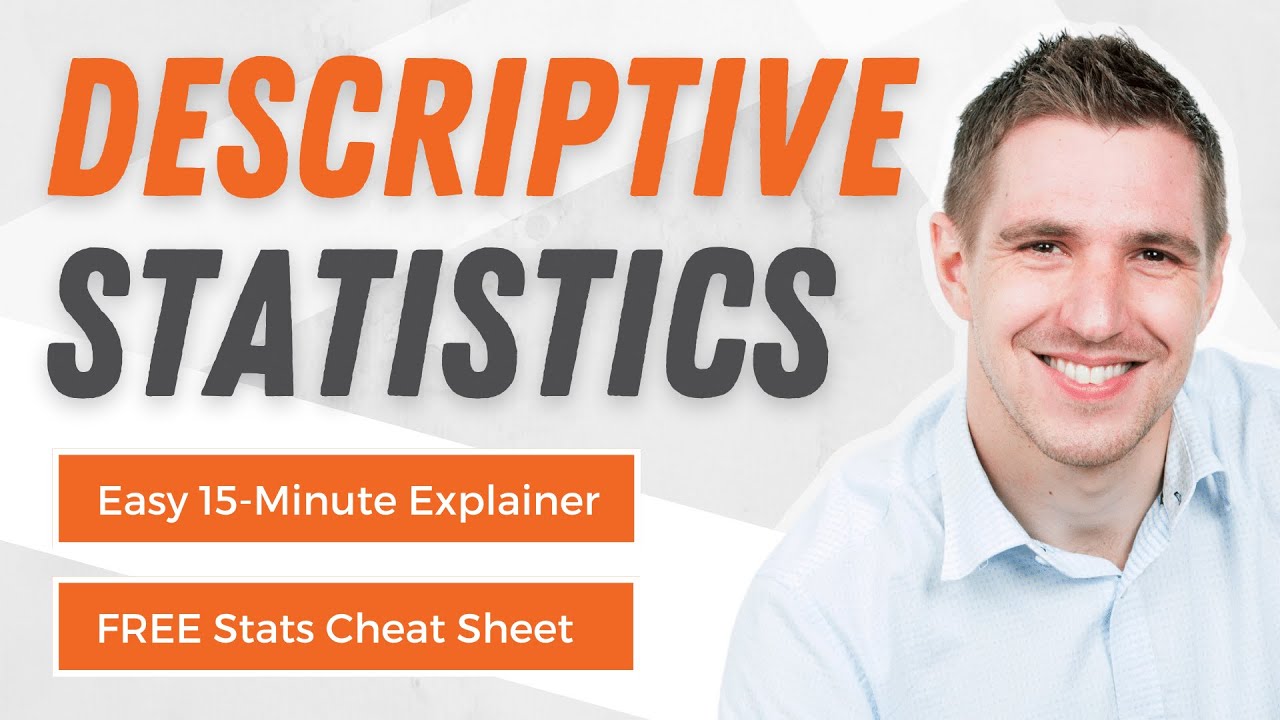
Descriptive Statistics: FULL Tutorial - Mean, Median, Mode, Variance & SD (With Examples)
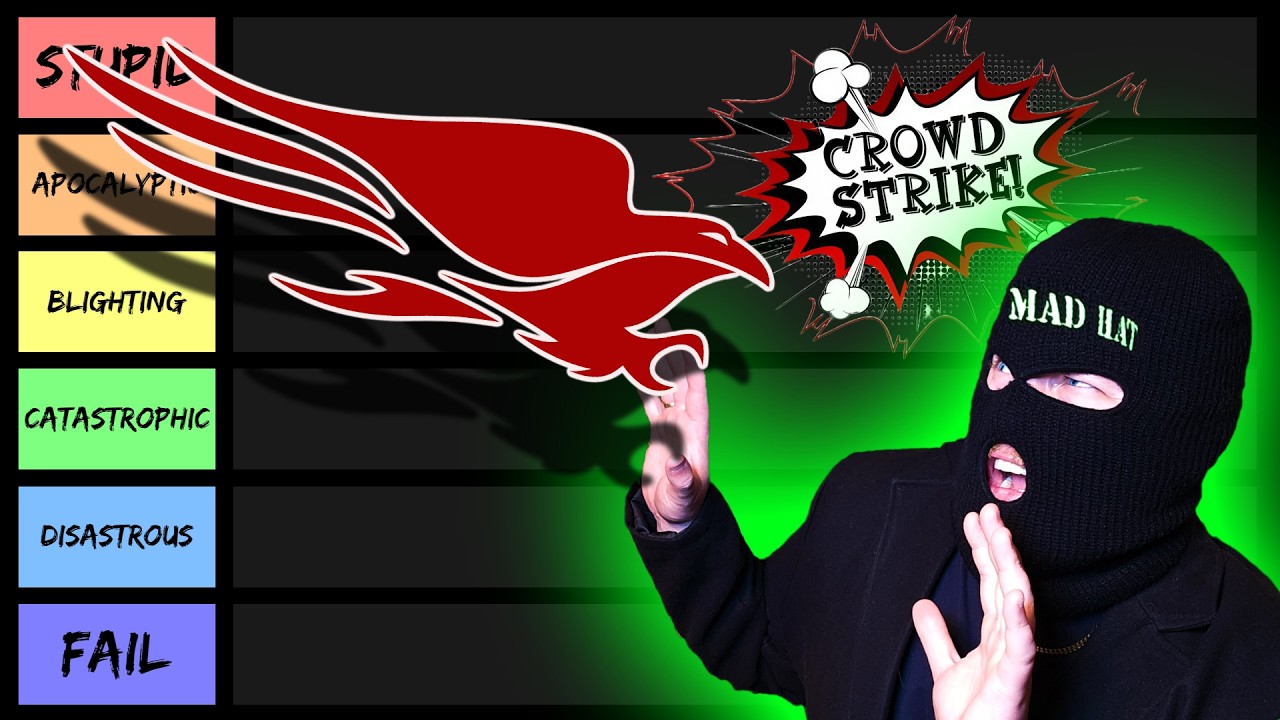
Cybersecurity Breach Tier List 2024
5.0 / 5 (0 votes)