Google Product Manager Execution Interview: YouTube Watch Time Root Cause Analysis
Summary
TLDRIn this mock product management interview, Cherry, a former Google PM, discusses the impact of shipping YouTube comments on mobile. Despite increased comment engagement, watch time has dropped, prompting a strategic analysis. Cherry outlines a structured approach to diagnosing the issue, including setting clear launch criteria, examining user behavior, and considering UI adjustments. The discussion explores potential solutions like A/B testing different comment displays and ensuring a positive user experience, emphasizing the importance of aligning metrics with initial expectations.
Takeaways
- 📱 The scenario involves a decrease in YouTube watch time following the launch of YouTube comments on mobile devices, despite an increase in comment engagement.
- 🤔 Cherry, a former Google product manager, outlines a structured approach to diagnosing and addressing the issue, emphasizing the importance of having clear launch criteria and backstop metrics.
- 📈 Cherry suggests that the increase in comment engagement might be cannibalizing watch time, as users spend more time interacting with comments and less time watching videos.
- 🔍 She recommends analyzing the decline in watch time to determine if it's a one-time event or a progressive trend, and to check for any technical issues or regional/platform-specific problems.
- 🌐 Consideration of internationalization and localization issues is important, as different languages and text densities can affect the user interface and experience.
- 📊 Cherry proposes a series of questions to better understand the context of the decline and to identify potential causes, such as changes in user behavior or issues with the recommendation algorithm.
- 🛠️ A/B testing is suggested as a method to experiment with different UI treatments, such as reducing the number of comments displayed or adjusting the ranking of recommended videos.
- 📝 The importance of defining 'comment engagement' is highlighted, including various metrics like the number of comments created, replies, likes, and time spent on comments.
- 🚫 Assumptions about spam and abuse detection systems being in place are made, to ensure that the increase in comments represents meaningful user engagement.
- 🔄 Cherry discusses the need to continuously monitor and test to find the optimal balance between comment engagement and watch time, using data to guide decision-making.
- 🗣️ The mock interview concludes with a reminder of the importance of considering abuse and bad content growth when analyzing user engagement metrics.
Q & A
What is the main issue discussed in the mock interview?
-The main issue discussed is the decline in YouTube watch time following the launch of YouTube comments on mobile devices, despite an increase in comment engagement.
Who are the hosts and guests involved in the mock interview?
-The hosts and guest are Kevin Way, who is conducting the interview, and Cherry, a former product manager at Google who worked on YouTube and Google Maps.
What is Cherry's approach to handling the scenario of increased comment engagement but decreased watch time?
-Cherry outlines a structured approach involving clarifying terms, listing possible causes, gathering context, establishing a theory of probable cause, and testing this theory to fix the problem.
What was the expected outcome of launching YouTube comments on mobile devices according to Cherry?
-The expected outcome was an increase in comment engagement, with the assumption that if the engagement level was similar to that on desktop, then by launching on mobile, the overall comment engagement should have doubled.
What are the launch criteria and backstop metrics Cherry mentions?
-The launch criteria would be that comment engagement increases by at least 50%. The backstop metric is a maximum acceptable drop of 5% in YouTube watch time.
How does Cherry suggest diagnosing the cause of the decline in watch time?
-Cherry suggests diagnosing by asking questions about the time period of the decline, checking for technical problems, considering regional and platform-specific issues, and examining the ecosystem of YouTube features.
What potential UI treatments does Cherry propose to address the issue?
-Cherry proposes reducing the number of comments displayed, adjusting the ranking of recommended videos to include shorter videos, and adding an educational tooltip to inform users that more recommendations are available below the comments.
How does Cherry define 'comment engagement' in the context of the interview?
-Comment engagement is defined as an aggregate score that encompasses the number of comments created, replies, likes, time spent scrolling on comments, and taps on the comments.
What is Cherry's suggestion for conducting A/B tests to identify the best solution?
-Cherry suggests setting up multiple arms for the experiment, including a control group and test groups with varying numbers of comments displayed, and comparing the impact on watch time across these groups.
How does Cherry address the potential issue of spam and abuse in the comment section?
-Cherry assumes that there is a spam and abuse detection system in place and that the increase in comment engagement is primarily due to meaningful user interactions.
What is Cherry's final recommendation for choosing the best testing arm in an A/B test?
-Cherry recommends choosing the arm that best meets the launch criteria, considering both the impact on watch time and the level of comment engagement, ensuring that the solution aligns with the initial objectives.
Outlines
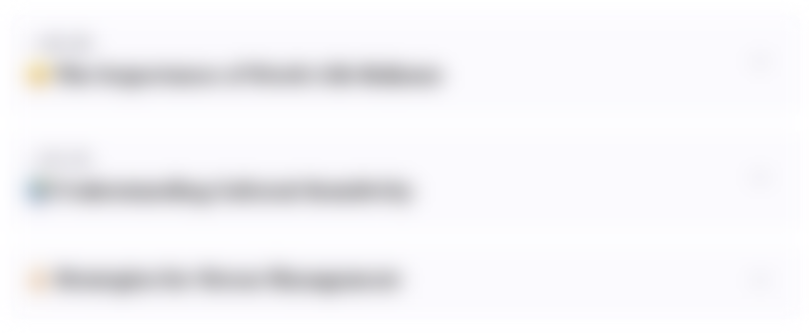
This section is available to paid users only. Please upgrade to access this part.
Upgrade NowMindmap
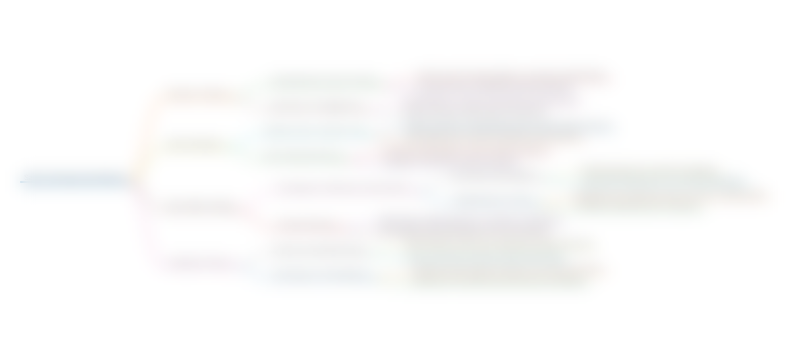
This section is available to paid users only. Please upgrade to access this part.
Upgrade NowKeywords
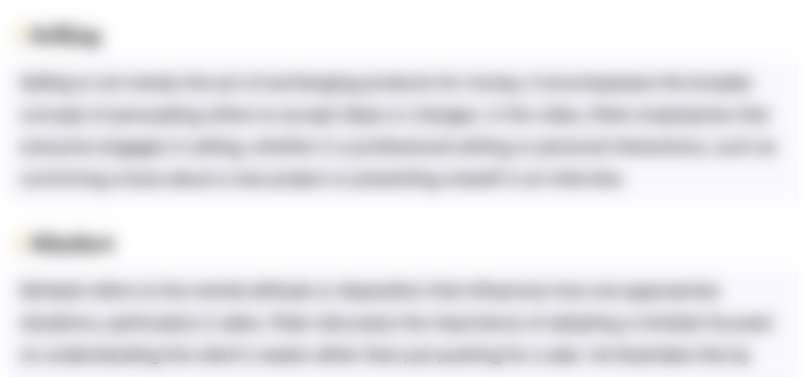
This section is available to paid users only. Please upgrade to access this part.
Upgrade NowHighlights
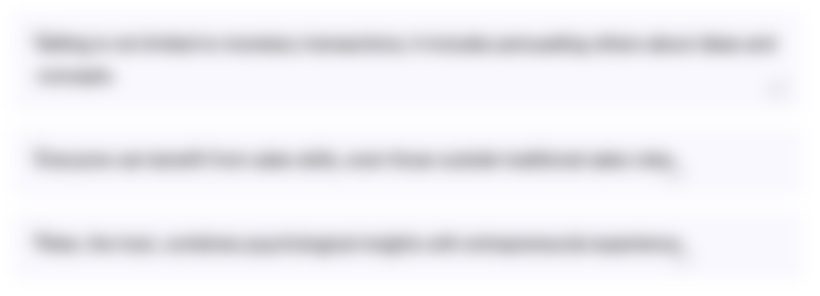
This section is available to paid users only. Please upgrade to access this part.
Upgrade NowTranscripts
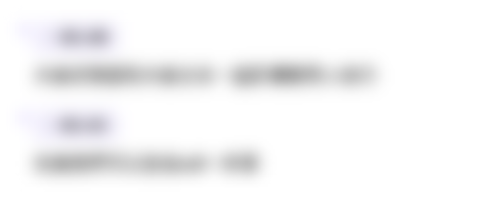
This section is available to paid users only. Please upgrade to access this part.
Upgrade NowBrowse More Related Video
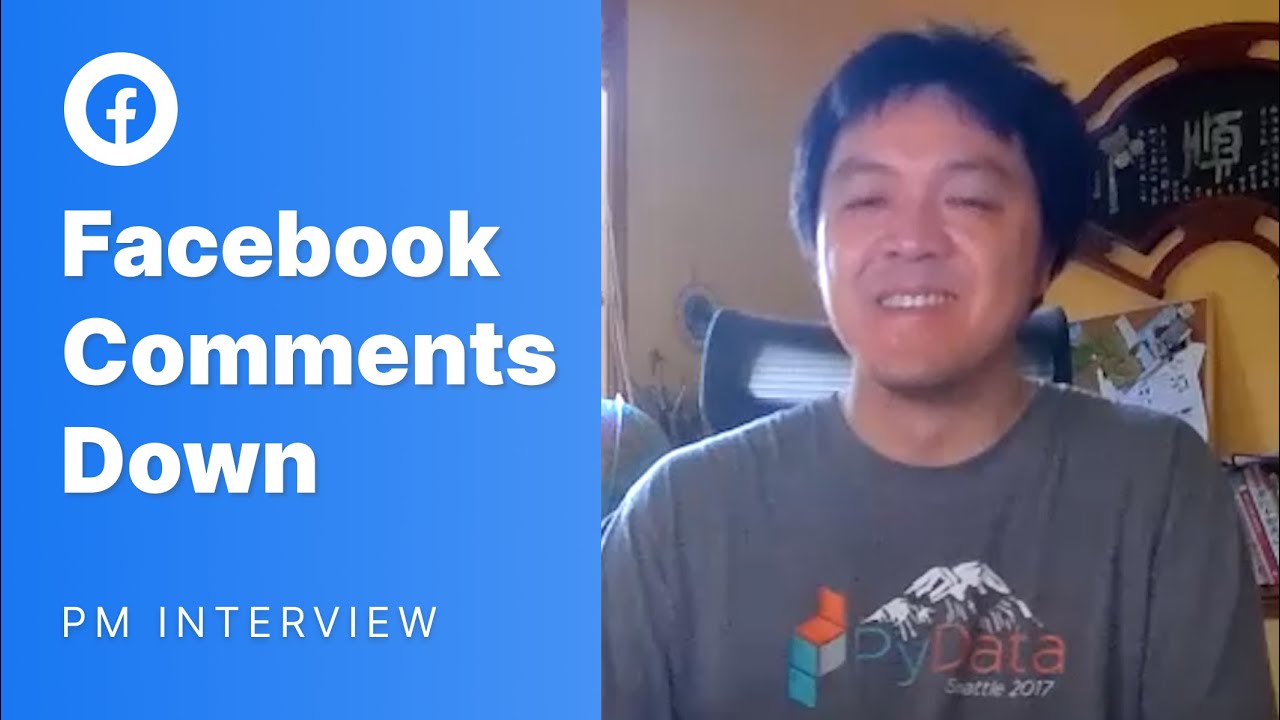
Facebook Product Manager Execution Interview: Comments & Reactions
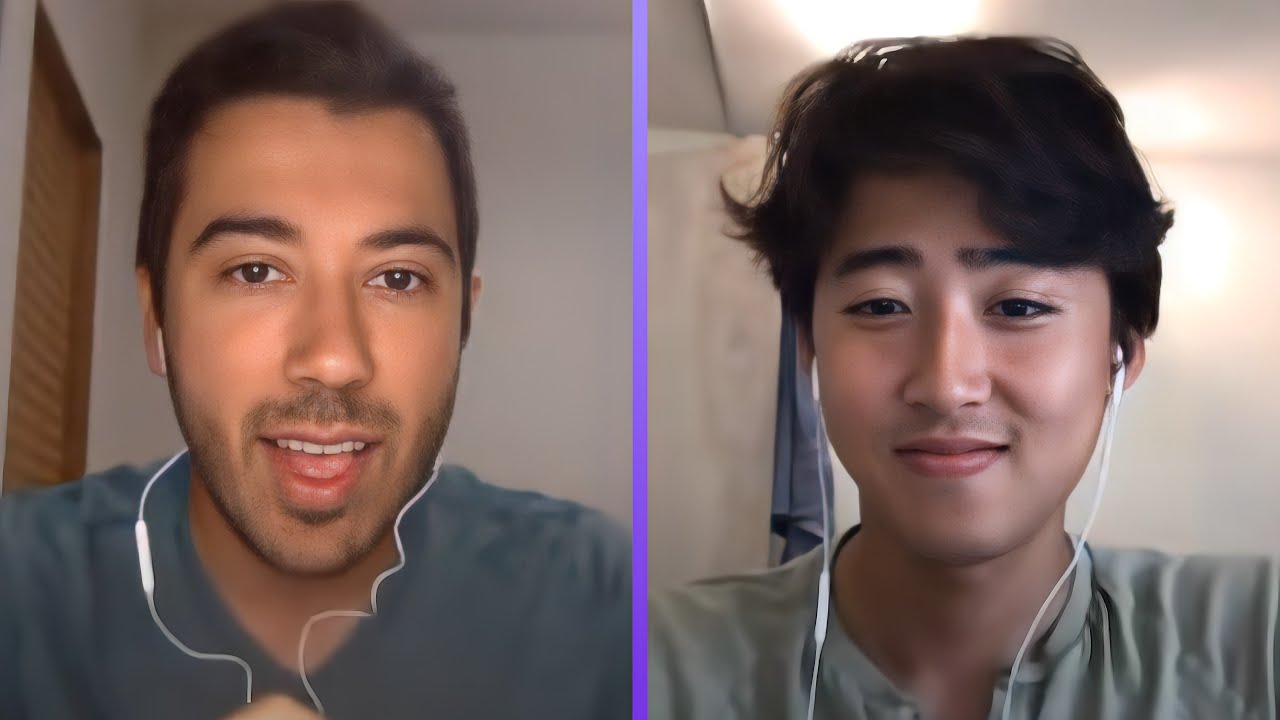
Curriculum - Design Product To Encourage Voting (with LinkedIn PM)
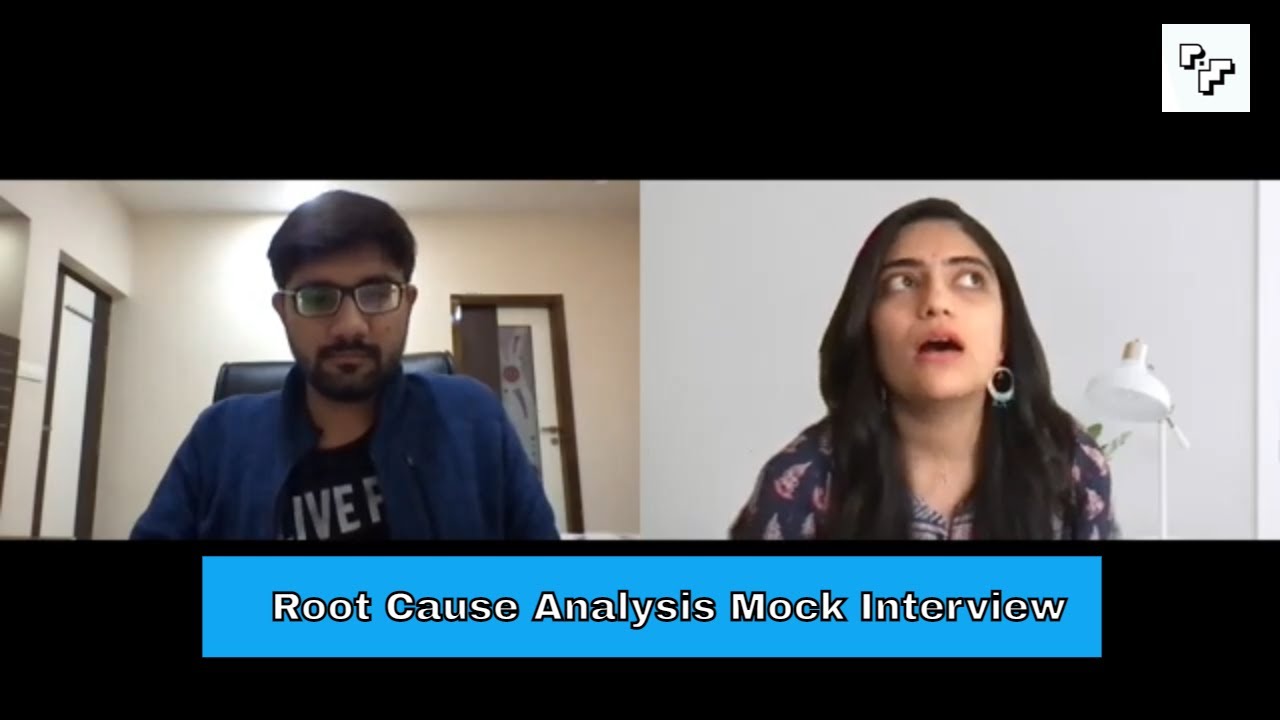
Root Cause Analysis Mock Interview with Piyush Sharma, Product Manager at Cure.Fit
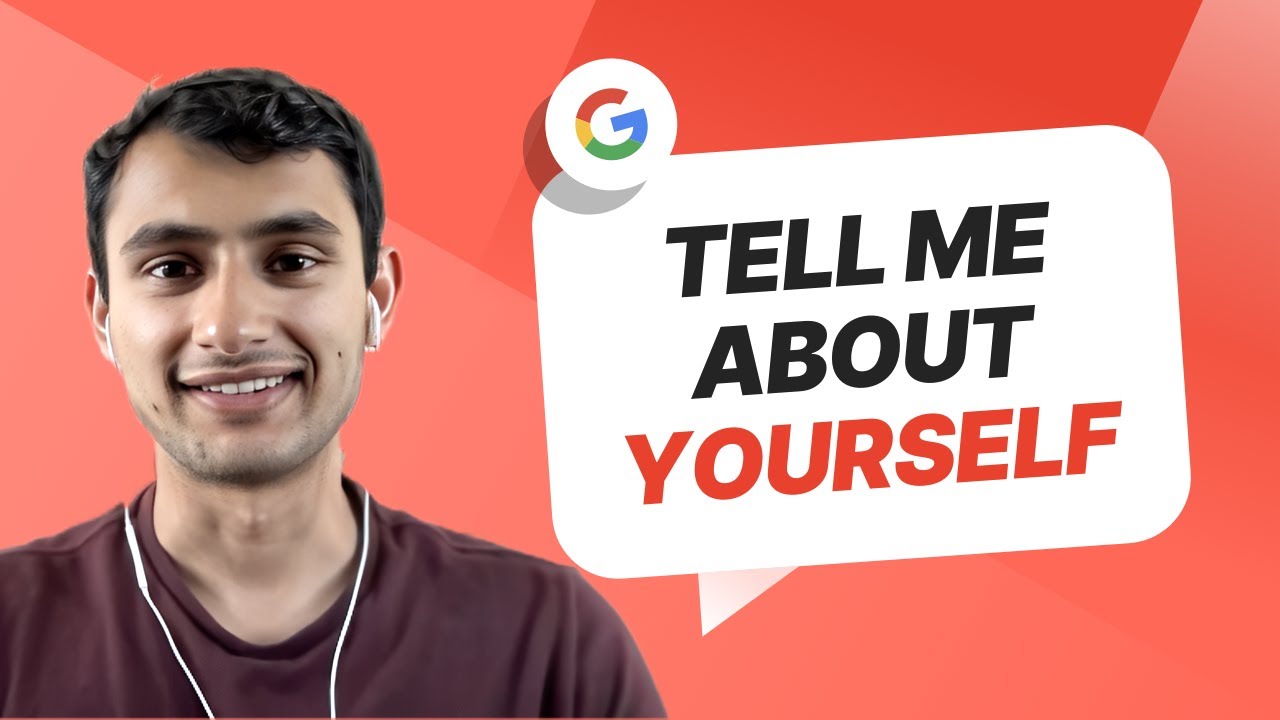
Google product manager behavioral interview (Top 5 questions)

Grow Google Cloud 10x - Strategy Mock Interview (with Google Product Manager)

Live Product Manager Interview: Slack
5.0 / 5 (0 votes)