The future of AI looks like THIS (& it can learn infinitely)
Summary
TLDRThis video script explores the limitations of current AI models, which are static and energy-intensive, and introduces the next generation of AI with liquid and spiking neural networks. These networks aim to mimic the human brain's adaptability and efficiency, offering real-time learning and reduced computational needs. Applications range from autonomous vehicles to healthcare, with the potential for AI to become smarter over time. However, these concepts are still in early research phases, facing challenges in implementation and training.
Takeaways
- 🧠 Current AI models, including GPT and Stable Diffusion, are limited by their inability to learn or adapt after training, much like a brain that has stopped growing.
- 🤖 AI operates on neural networks with nodes and layers, where each node filters information to the next layer, akin to dials and knobs controlling data flow.
- 🔄 The training process for AI models involves millions of iterations, using backpropagation to adjust weights and minimize errors, but once trained, the model's intelligence is fixed.
- 🔋 AI models are highly energy-intensive, with GPT-3's training alone requiring as much energy as 1,500 US homes use in a month, highlighting a need for more efficient AI.
- 🌟 The next generation of AI should ideally mimic the human brain's neuroplasticity, allowing for continuous learning and adaptation to new information.
- 💧 Liquid neural networks are an emerging architecture designed to be flexible and adaptive, with a 'reservoir' layer that can change dynamically in response to new data.
- 📉 Liquid neural networks require less computational power for training since only the output layer is trained, making them potentially more efficient than traditional networks.
- 🚀 Applications for liquid neural networks include autonomous robots, self-driving cars, and real-time data processing, where adaptability is crucial.
- 🌐 Spiking neural networks are another potential next-gen AI architecture, mimicking the brain's neuron communication through discrete spikes and timing.
- 🕒 Spiking networks incorporate time into their processing, which can lead to more efficient learning and adaptation, especially suitable for temporal data.
- 🚧 Both liquid and spiking neural networks are in early stages of research with challenges such as complexity in training and lack of standardized support, but they offer promising potential for AI evolution.
Q & A
What is the current state of AI technology as described in the video script?
-The current state of AI technology, as described in the script, is that while it is impressive, it is also quite limited. AI models like chat GPT, stable diffusion, and others are based on neural networks that are fixed in their intelligence and capabilities after training, and they require significant computational power to function.
What is a neural network and how does it function in the context of AI?
-A neural network is a series of interconnected nodes, or neurons, arranged in layers, that process information by adjusting weights and biases to determine how much information flows through to the next layer. It functions in AI by receiving input data, processing it through these layers, and outputting a result after the data has passed through the network and been interpreted by the final layer.
What is the concept of 'neuroplasticity' in the context of the human brain and how does it differ from current AI models?
-Neuroplasticity refers to the brain's ability to reorganize and reconfigure itself by forming new neural connections over time to adapt to new environments or learn new things. This is different from current AI models, which are static after training and cannot continue to learn or adapt without being retrained with new data.
How are AI models like GPT and stable diffusion trained?
-AI models like GPT and stable diffusion undergo millions of rounds of training. They process input data, and if the output is incorrect, a penalty is incurred which causes the weights in the neural network to be updated through a process called backpropagation. This continues until the model can accurately perform its task.
What are the two major limitations of the current generation of AI models?
-The two major limitations of the current generation of AI models are that they are fixed in their intelligence and capabilities after training and cannot learn or improve further, and they are extremely energy-intensive and inefficient compared to the human brain.
What is the concept of liquid neural networks and how do they differ from traditional neural networks?
-Liquid neural networks are designed to mimic the flexibility and plasticity of the human brain. They have a 'reservoir' layer that can change dynamically over time in response to new data, unlike traditional neural networks which have fixed weights and connections after training.
How are liquid neural networks trained and why is this process more efficient?
-Liquid neural networks are trained by setting up random connections in the reservoir layer, feeding data into the input layer, and training only the output layer to map the reservoir states to the desired output. This process is more efficient because it requires optimizing fewer parameters, reducing computational requirements.
What are some potential real-world applications of liquid neural networks?
-Potential applications of liquid neural networks include autonomous AI robots that can adapt to new tasks, self-driving cars that can navigate dynamic environments, healthcare monitoring for real-time patient analysis, stock trading optimization, and smart city management for traffic flow and energy management.
What is a spiking neural network and how does it differ from other neural networks?
-A spiking neural network is a type of neural network that mimics the way neurons in the human brain communicate using discrete spikes or action potentials. Unlike other neural networks that use continuous signals, spiking neural networks process information based on the timing and frequency of these spikes.
What are the main benefits of spiking neural networks?
-The main benefits of spiking neural networks include their efficiency, as they only use energy where spikes occur, making them more energy-efficient than traditional neural networks. They are also well-suited for neuromorphic chips and can process temporal data effectively, making them ideal for adaptive and autonomous systems.
What are some challenges associated with the development and implementation of spiking neural networks?
-Challenges with spiking neural networks include the complexity of setting up and programming them, the difficulty in training them due to the discrete nature of spikes, the need for specialized hardware like neuromorphic chips, and their current underperformance for non-time-based data compared to traditional neural networks.
Outlines
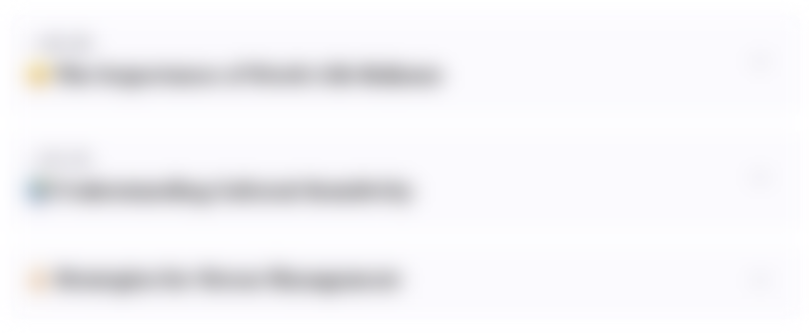
This section is available to paid users only. Please upgrade to access this part.
Upgrade NowMindmap
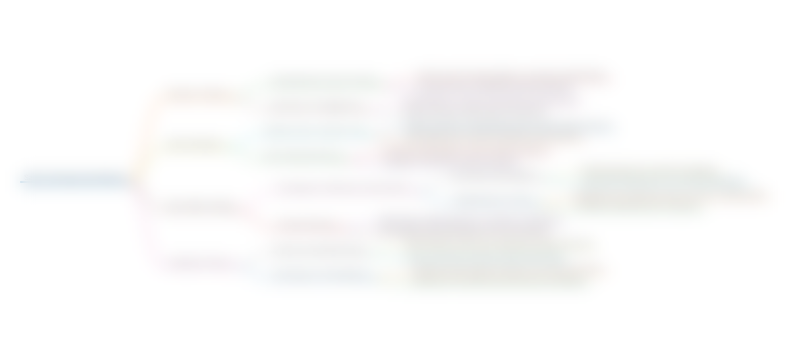
This section is available to paid users only. Please upgrade to access this part.
Upgrade NowKeywords
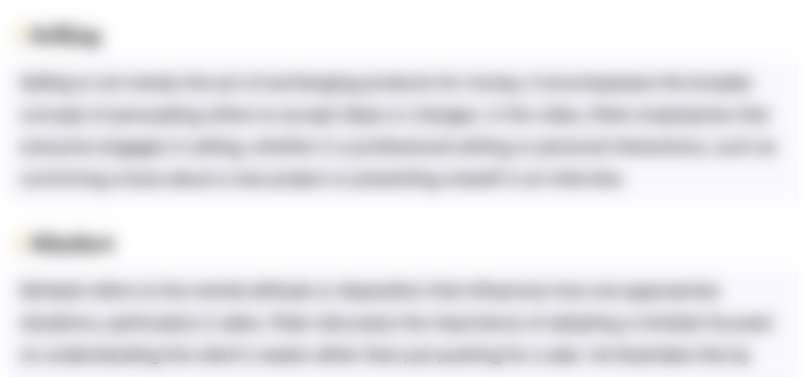
This section is available to paid users only. Please upgrade to access this part.
Upgrade NowHighlights
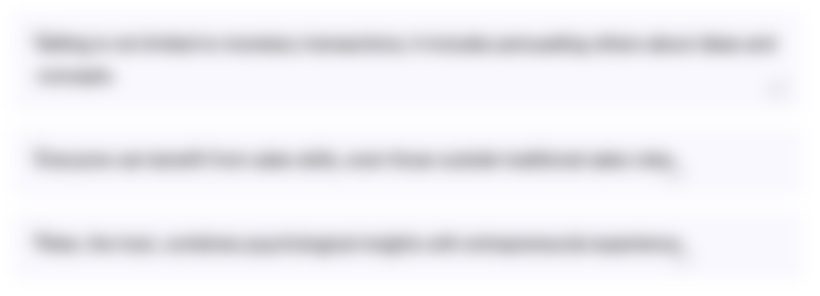
This section is available to paid users only. Please upgrade to access this part.
Upgrade NowTranscripts
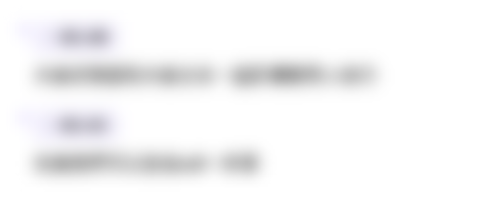
This section is available to paid users only. Please upgrade to access this part.
Upgrade NowBrowse More Related Video
5.0 / 5 (0 votes)