Liquid Neural Networks
Summary
TLDRThe video script features a CBMM talk with Daniela Rus, director of CSAIL, and Dr. Ramin Hasani, where they introduce the concept of 'liquid neural networks.' These networks, inspired by neuroscience, aim to improve upon traditional deep neural networks by offering more compact, sustainable, and explainable models. Hasani discusses the limitations of current AI systems, which rely heavily on computation and data without fully capturing the causal structure of tasks. He presents a new approach that integrates biological insights into machine learning, resulting in models that are more expressive, robust to perturbations, and capable of extrapolation. The talk also covers the implementation of these models using continuous-time processes and the potential applications in real-world robotics and autonomous driving.
Takeaways
- 📚 Daniela Rus introduced the concept of bridging the natural world with engineering, focusing on intelligence in both biological brains and artificial intelligence (AI).
- 🤖 Ramin Hasani presented Liquid Neural Networks, inspired by neuroscience, aiming to improve upon deep neural networks in terms of compactness, sustainability, and explainability.
- 🧠 The natural brain's interaction with the environment and its ability to understand causality were highlighted as areas where AI could benefit from biological insights.
- 🚗 Attention maps from AI systems were discussed, noting differences in focus when driving decisions are made, with an emphasis on the importance of capturing the true causal structure.
- 🔬 Hasani's research involved looking at neural circuits and dynamics at the cellular level to understand the building blocks of intelligence.
- 🌐 Continuous time neural networks (Neural ODEs) were explored for their ability to model sequential behavior and their potential advantages over discrete representations.
- 🔍 The importance of using numerical ODE solvers for implementing these models and the trade-offs between different solvers in terms of accuracy and memory complexity were discussed.
- 🤝 The integration of biological principles, such as leaky integrators and conductance-based synapse models, into AI networks to improve representation learning and robustness was emphasized.
- 📉 The expressivity of different network types was compared, demonstrating that liquid neural networks could produce more complex trajectories, indicating higher expressivity.
- 🚀 Applications of these networks were shown in real-world scenarios like autonomous driving, where they outperformed traditional deep learning models in terms of parameter efficiency and robustness to perturbations.
- ⚖️ The potential of liquid neural networks to serve as a bridge between statistical and physical models, offering a more causal and interpretable approach to machine learning, was highlighted.
Q & A
Who is the presenter of today's CBMM talk?
-The presenter of today's CBMM talk is Daniela Rus, the director of CSAIL.
What is the main focus of Daniela Rus' research?
-Daniela Rus' research focuses on bridging the gap between the natural world and engineering, specifically by drawing inspiration from the natural world to create more compact, sustainable, and explainable machine learning models.
What is the name of the artificial intelligence algorithm that Ramin Hasani is presenting?
-Ramin Hasani is presenting Liquid Neural Networks, a class of AI algorithms.
How do Liquid Neural Networks differ from traditional deep neural networks?
-Liquid Neural Networks differ from traditional deep neural networks by incorporating principles from neuroscience, such as continuous dynamics, synaptic release mechanisms, and conductance-based synapse models, leading to more expressive and causally structured models.
What are the advantages of using continuous time models in machine learning?
-Continuous time models offer advantages such as a larger space of possible functions, arbitrary computation steps, the ability to model sequential behavior more naturally, and improved expressivity and robustness to perturbations.
How do Liquid Neural Networks capture the causal structure of data?
-Liquid Neural Networks capture the causal structure of data by using dynamical systems that are inspired by biological neural activity, allowing them to understand and predict the outcomes of interventions and to perform better in out-of-distribution scenarios.
What is the significance of the unique solution property in the context of Liquid Neural Networks?
-The unique solution property, derived from the Picard-Lindelof theorem, ensures that the differential equations describing the network's dynamics have a unique solution under certain conditions, which is crucial for the network's ability to make deterministic predictions and maintain stability.
How do Liquid Neural Networks improve upon the limitations of standard neural networks?
-Liquid Neural Networks improve upon standard neural networks by providing a more expressive representation, better handling of memory and temporal aspects of tasks, enhanced robustness to input noise, and a more interpretable model structure due to their biological inspiration.
What are some potential applications of Liquid Neural Networks?
-Potential applications of Liquid Neural Networks include autonomous driving, robotics, generative modeling, and any task that requires capturing causal relationships, temporal dynamics, or making decisions based on complex data.
What are the challenges or limitations associated with implementing Liquid Neural Networks?
-Challenges or limitations associated with Liquid Neural Networks include potentially longer training and testing times due to the complexity of ODE solvers, the possibility of vanishing gradients for learning long-term dependencies, and the need for careful initialization and parameter tuning.
How does the research presented by Ramin Hasani contribute to the broader field of artificial intelligence?
-The research contributes to the broader field of artificial intelligence by proposing a new class of algorithms that are inspired by neuroscience, which can lead to more efficient, robust, and interpretable AI models. It also opens up new avenues for research in understanding intelligence and developing advanced machine learning frameworks.
Outlines
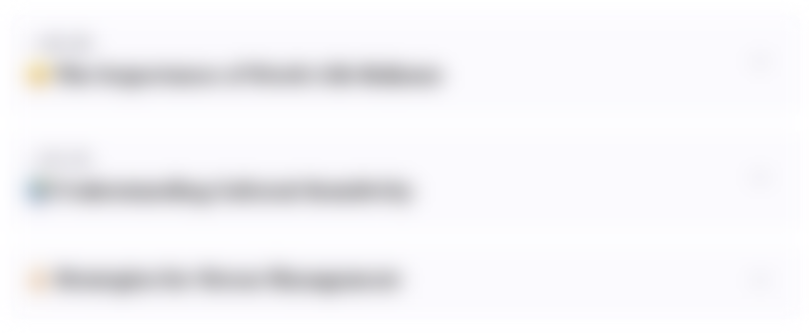
This section is available to paid users only. Please upgrade to access this part.
Upgrade NowMindmap
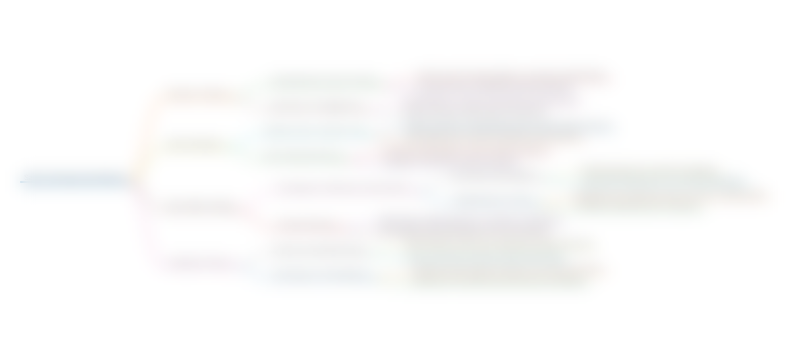
This section is available to paid users only. Please upgrade to access this part.
Upgrade NowKeywords
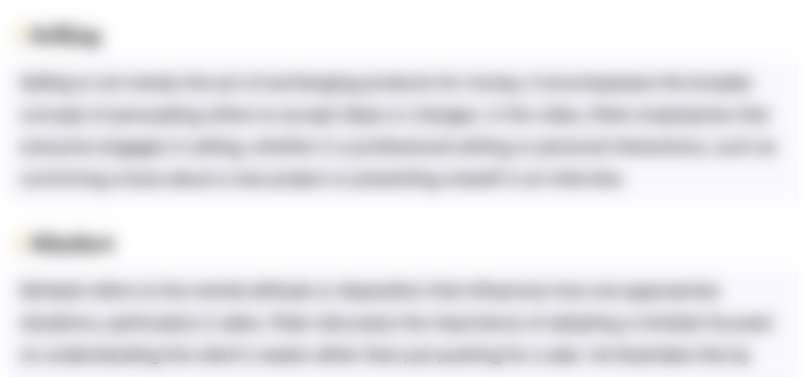
This section is available to paid users only. Please upgrade to access this part.
Upgrade NowHighlights
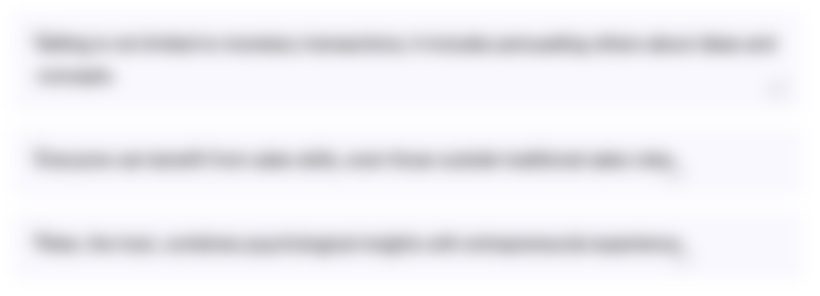
This section is available to paid users only. Please upgrade to access this part.
Upgrade NowTranscripts
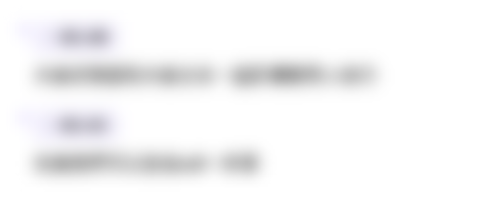
This section is available to paid users only. Please upgrade to access this part.
Upgrade NowBrowse More Related Video

The future of AI looks like THIS (& it can learn infinitely)
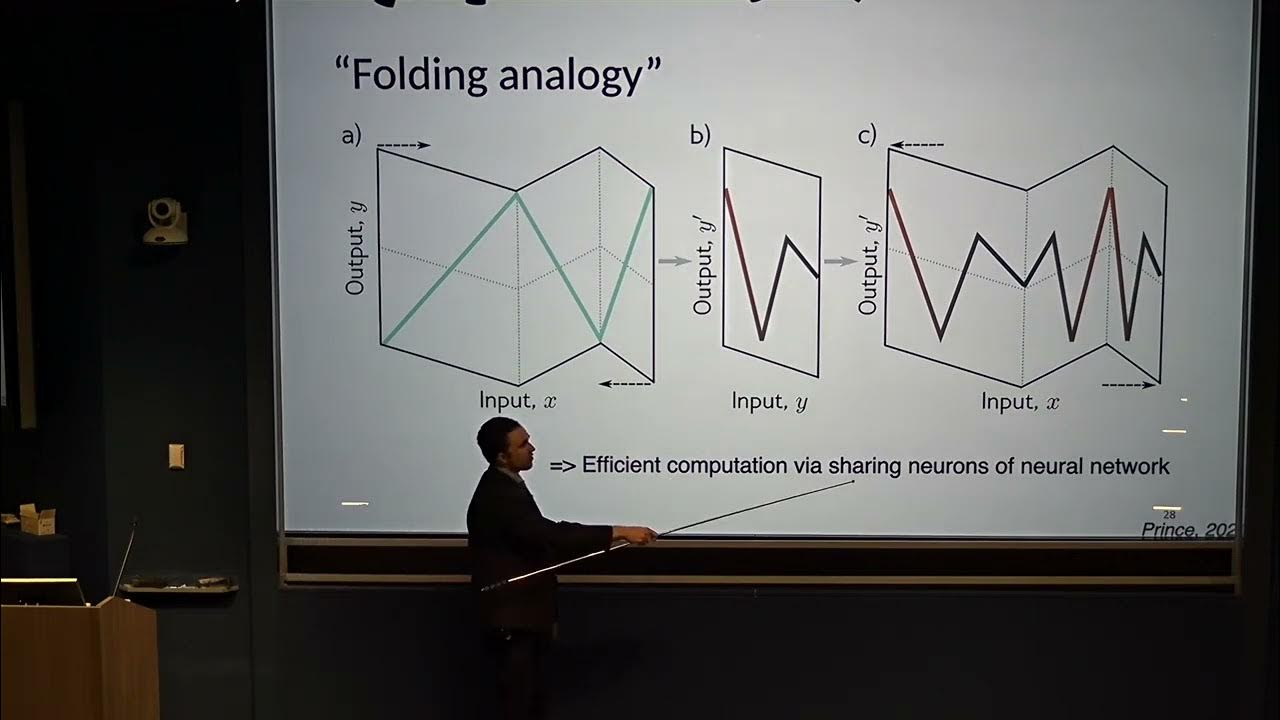
Miles Cranmer - The Next Great Scientific Theory is Hiding Inside a Neural Network (April 3, 2024)
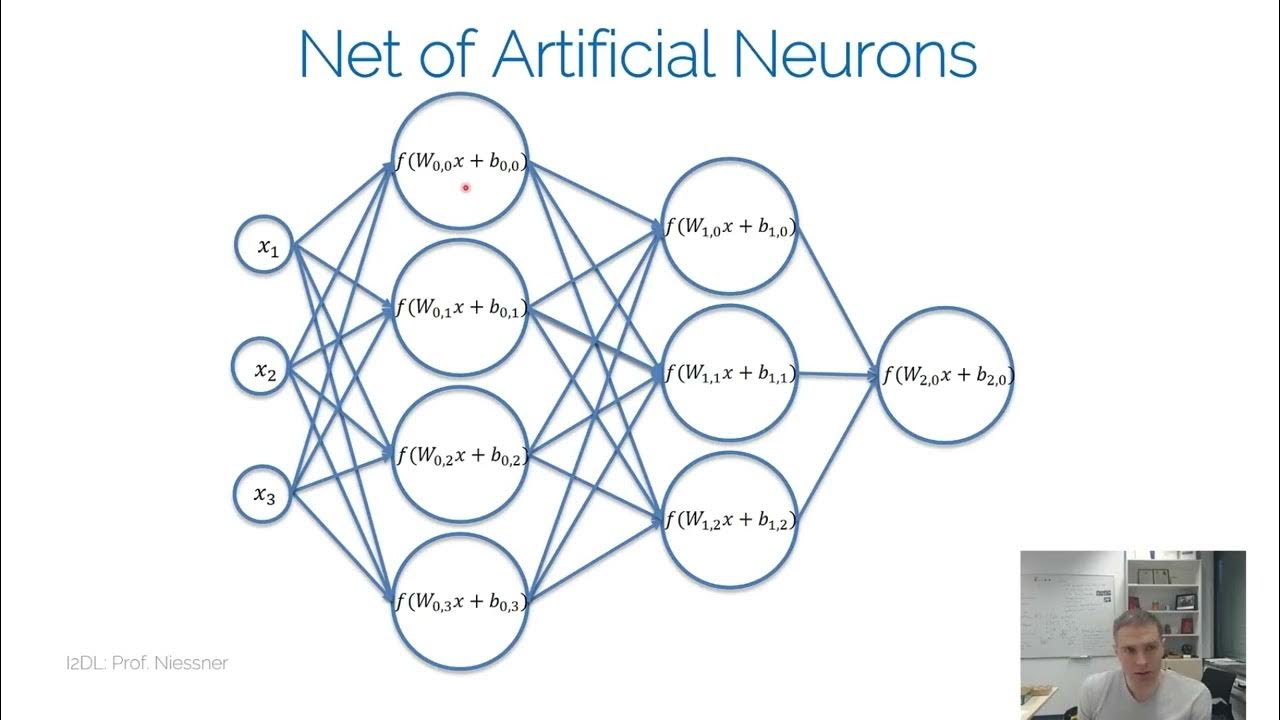
I2DL NN
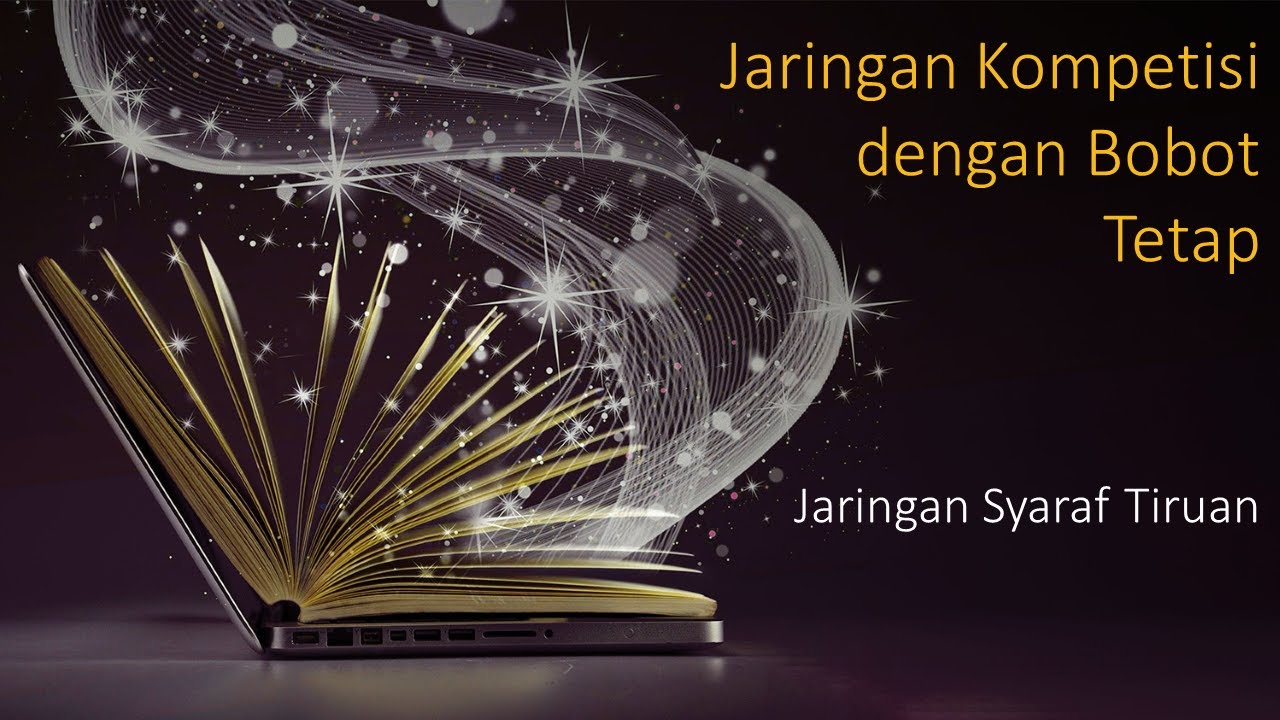
Jaringan Kompetisi dengan Bobot Tetap | Jaringan Syaraf Tiruan Pertemuan 10
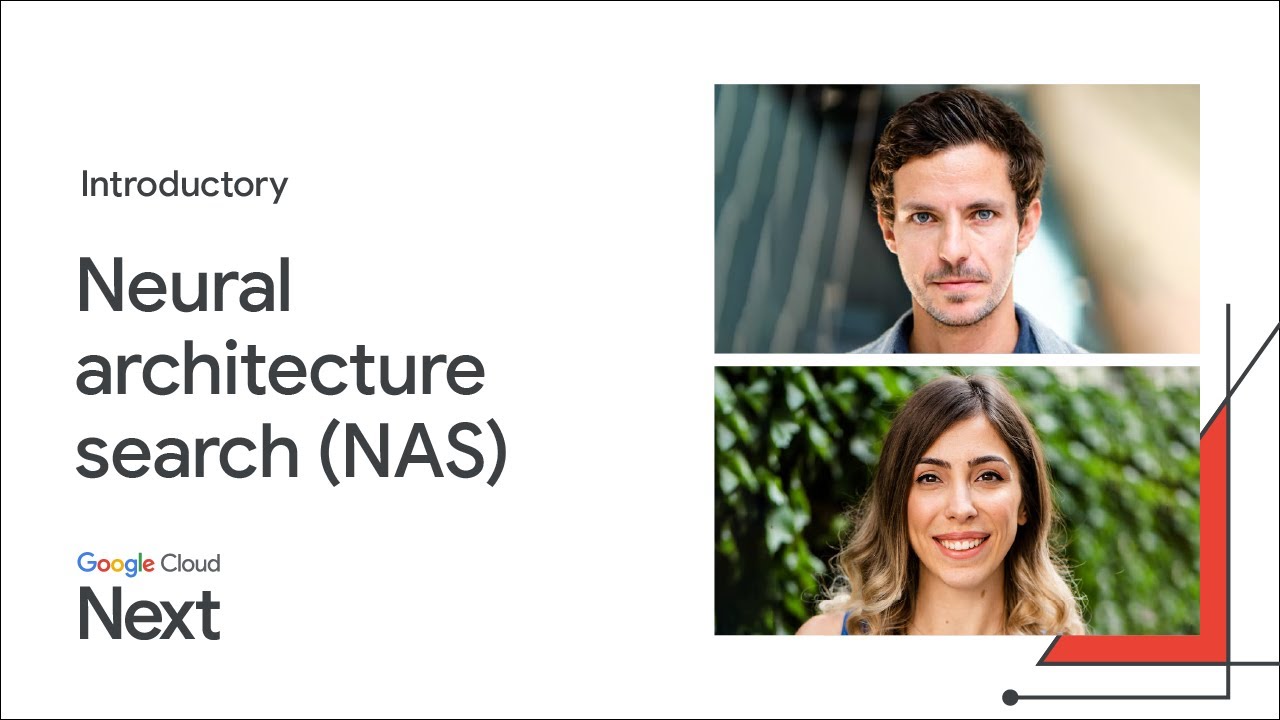
State of the Art Neural Networks - Neural architecture search (NAS)
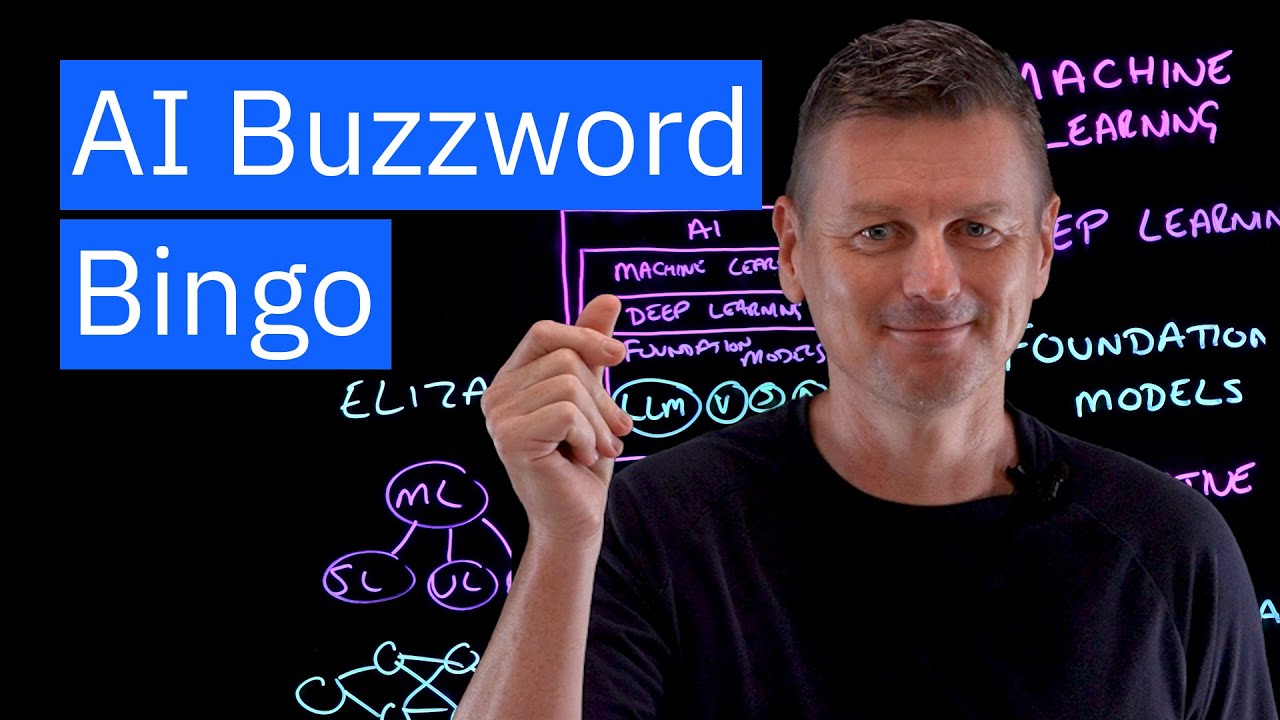
Machine Learning vs. Deep Learning vs. Foundation Models
5.0 / 5 (0 votes)