Building a new tokenizer
Summary
TLDRThis video guides you through the process of building a custom tokenizer from scratch using the Tokenizers library. It covers key steps including normalization, pre-tokenization, model selection (WordPiece for BERT), training, post-processing, and decoding. By following along, viewers will learn how to create a tokenizer tailored to their needs, train it on a dataset, and integrate it with the Transformers library for NLP tasks. The tutorial emphasizes the importance of each step in the tokenization pipeline, making complex concepts accessible for anyone interested in tokenization and NLP workflows.
Takeaways
- 😀 Creating your own tokenizer involves several key operations: normalization, pre-tokenization, model definition, post-processing, and decoding.
- 😀 Normalization refers to cleaning the text by converting it to lowercase and removing accents before tokenization.
- 😀 Pre-tokenization involves splitting the text at spaces and isolating punctuation marks to help in processing.
- 😀 Tokenization models like WordPiece (used by BERT) are central to the tokenizer creation process.
- 😀 Post-processing includes adding special tokens (like CLS and SEP) and generating attention masks and token IDs.
- 😀 Decoding converts token IDs back into a readable sentence, completing the tokenization process.
- 😀 A fast tokenizer combines all these operations, which are grouped under the backend_tokenizer attribute.
- 😀 To create a tokenizer for transformers, you need to create a training dataset, train a tokenizer with the tokenizers library, and load it into transformers.
- 😀 You can use datasets like wikitext-2-raw-v1 to train your tokenizer and use an iterator to process the text.
- 😀 The tokenizer’s model (e.g., WordPiece) can be trained by defining a vocabulary size and choosing special tokens like CLS and SEP for post-processing.
- 😀 Once the tokenizer is trained, you can integrate it into a fast tokenizer and use it in the transformer model, either as a generic or specific class like BertTokenizerFast.
Q & A
What are the main operations involved in tokenization?
-The main operations in tokenization include normalization, pre-tokenization, model creation, post-processing, and decoding.
What is the purpose of post-processing in tokenization?
-Post-processing gathers all the modifications made to the tokenized text, including adding special tokens, creating an attention mask, and generating a list of token IDs.
What is the decoding operation in tokenization?
-Decoding occurs at the end of tokenization and transforms a sequence of token IDs back into a readable sentence.
What is a fast tokenizer in the context of the tokenizers library?
-A fast tokenizer is an optimized implementation of tokenization that uses the 'backend_tokenizer' attribute to combine all the components like normalization, pre-tokenization, model, post-processing, and decoding.
What steps are required to create a tokenizer with the transformers library?
-The steps include creating a training dataset, defining and training a tokenizer using the tokenizers library, and then loading the tokenizer into the transformers library.
What dataset is used in the example to train the tokenizer?
-The dataset used in the example is the 'wikitext-2-raw-v1,' a small English dataset.
Why is the WordPiece model chosen for tokenizer design in the example?
-The WordPiece model is chosen because it is the model used by BERT, making it suitable for recreating a BERT tokenizer.
What normalizations are applied to clean the text during tokenizer creation?
-Two normalizations are used: lowercasing the text and removing accents.
How are the tokens pre-tokenized in the example?
-The pre-tokenization is performed by chaining two pre-tokenizers: the first separates the text at spaces, and the second isolates punctuation marks.
What is the role of the TemplateProcessing class in tokenization?
-The TemplateProcessing class helps add special tokens, like the CLS token at the beginning and the SEP token at the end of sequences, or between sentences in a text pair.
How is the trained tokenizer integrated into the transformers library?
-After training the tokenizer, it is loaded into a fast tokenizer from the transformers library using either the 'PreTrainedTokenizerFast' class or the 'BertTokenizerFast' class, depending on the model type.
What is the significance of choosing a vocabulary size during training?
-The vocabulary size defines the number of unique tokens the tokenizer will recognize. In the example, a vocabulary size of 25,000 is chosen to balance coverage and efficiency.
Outlines
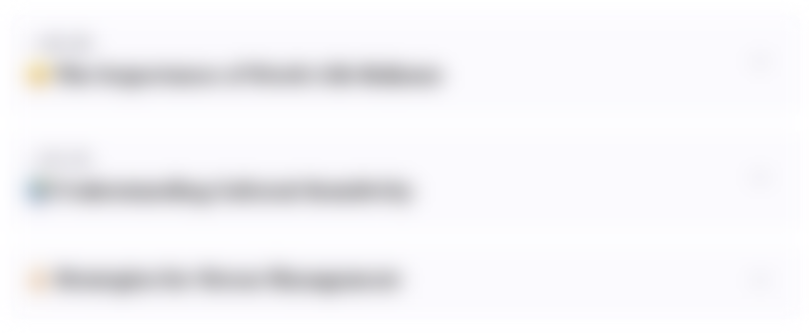
This section is available to paid users only. Please upgrade to access this part.
Upgrade NowMindmap
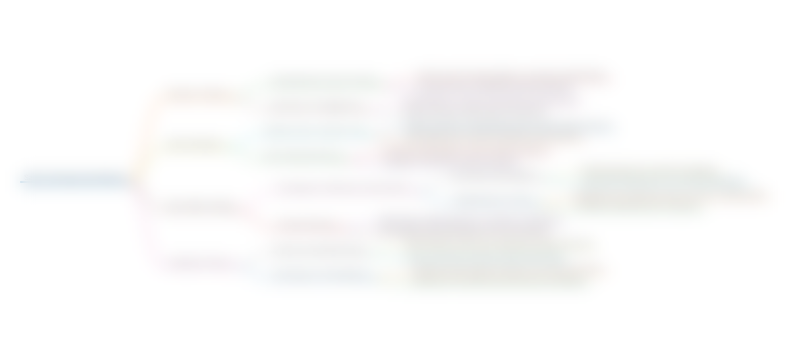
This section is available to paid users only. Please upgrade to access this part.
Upgrade NowKeywords
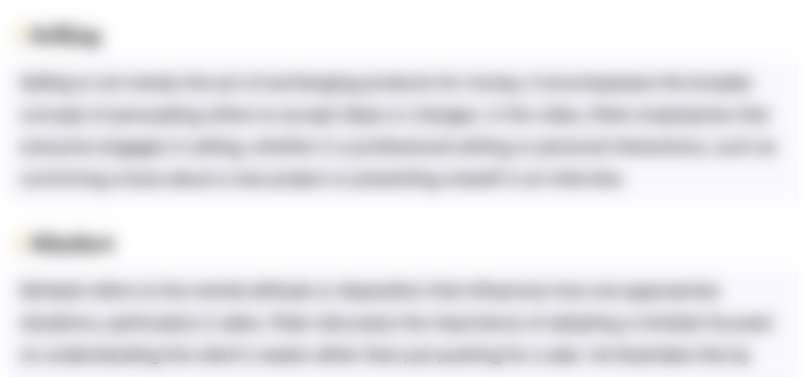
This section is available to paid users only. Please upgrade to access this part.
Upgrade NowHighlights
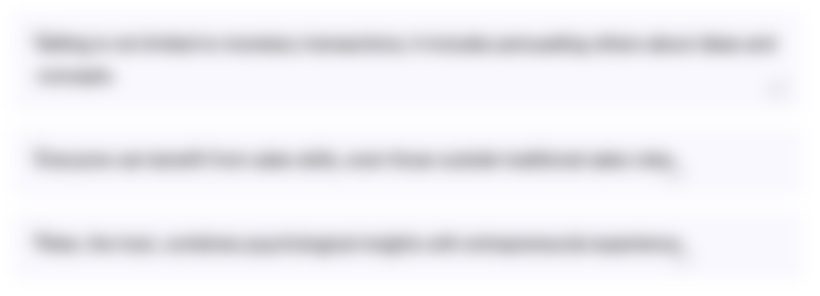
This section is available to paid users only. Please upgrade to access this part.
Upgrade NowTranscripts
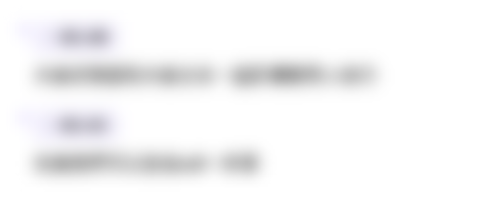
This section is available to paid users only. Please upgrade to access this part.
Upgrade NowBrowse More Related Video
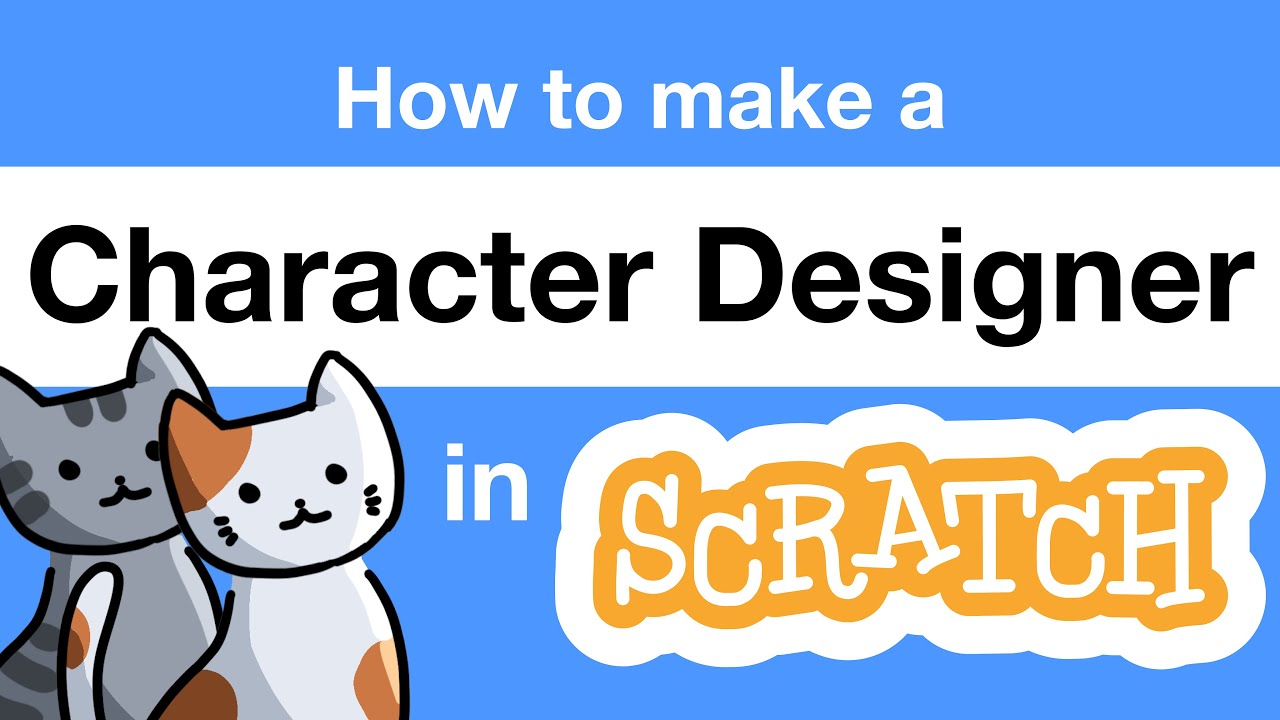
How to Make a Character Designer in Scratch | Tutorial
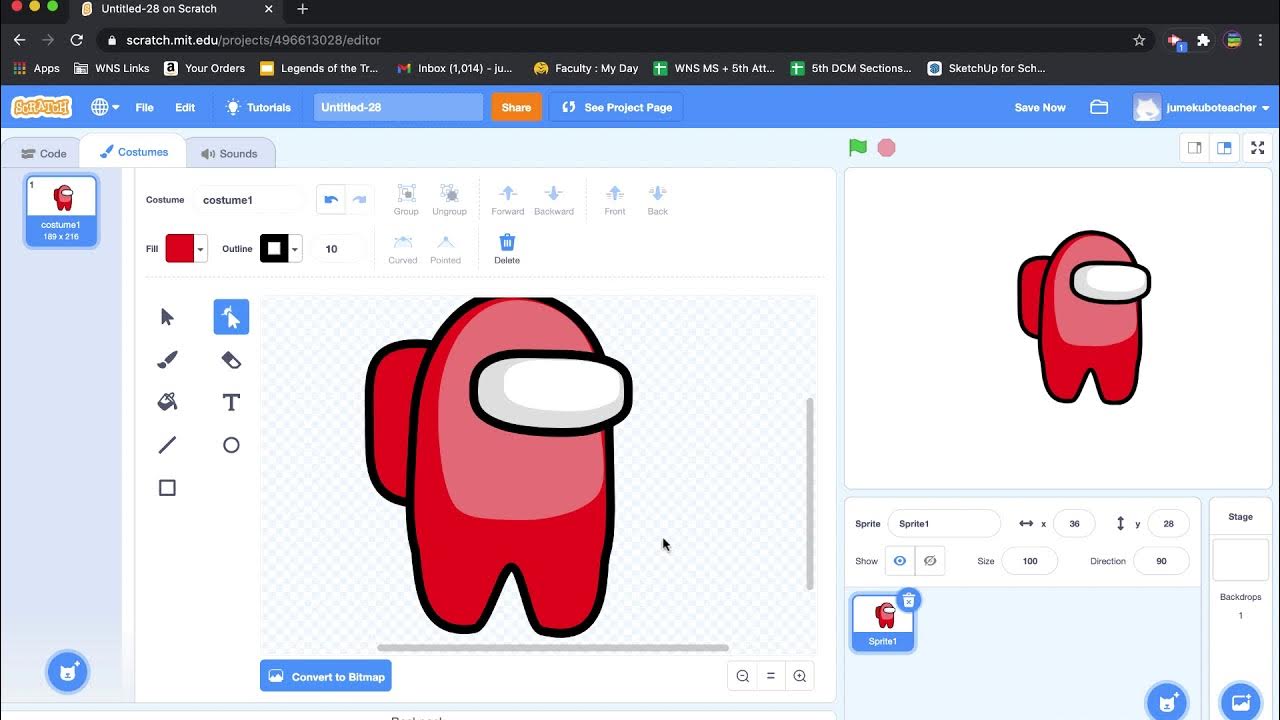
07. Scratch - Drawing and Animating a Sprite
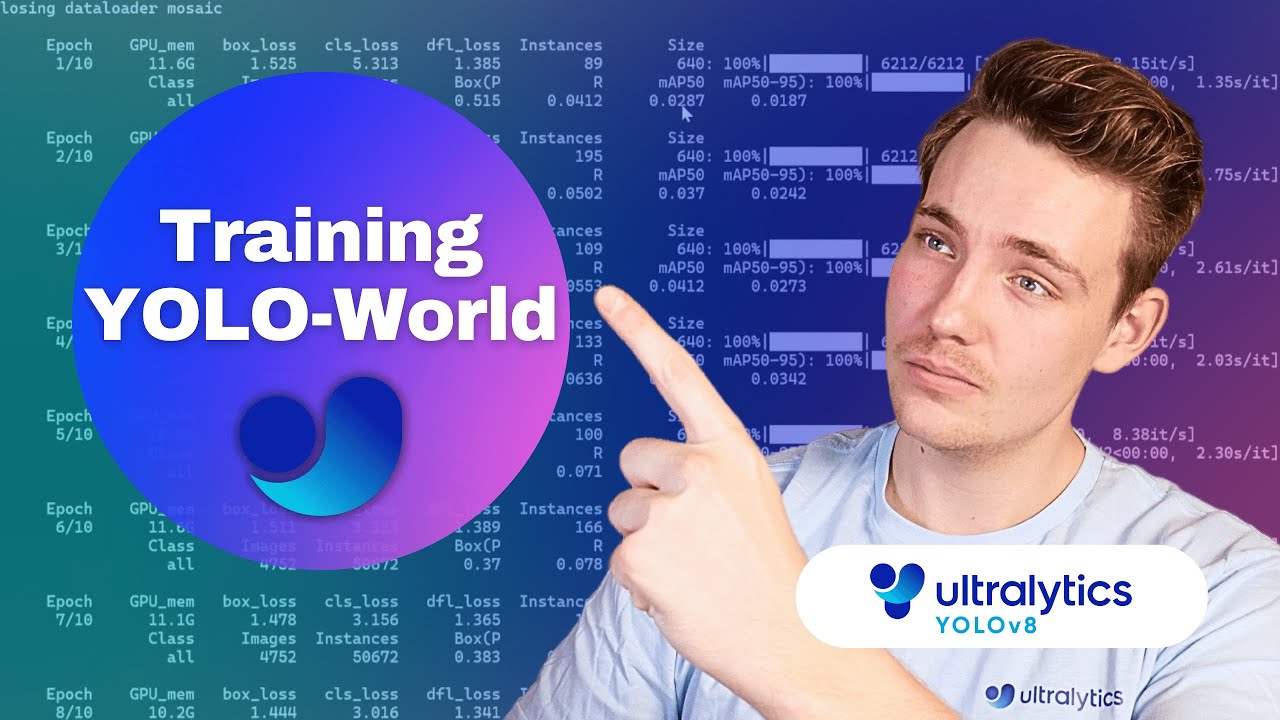
YOLO World Training Workflow with LVIS Dataset and Guide Walkthrough | Episode 46

Dagster Crash Course: develop data assets in under ten minutes
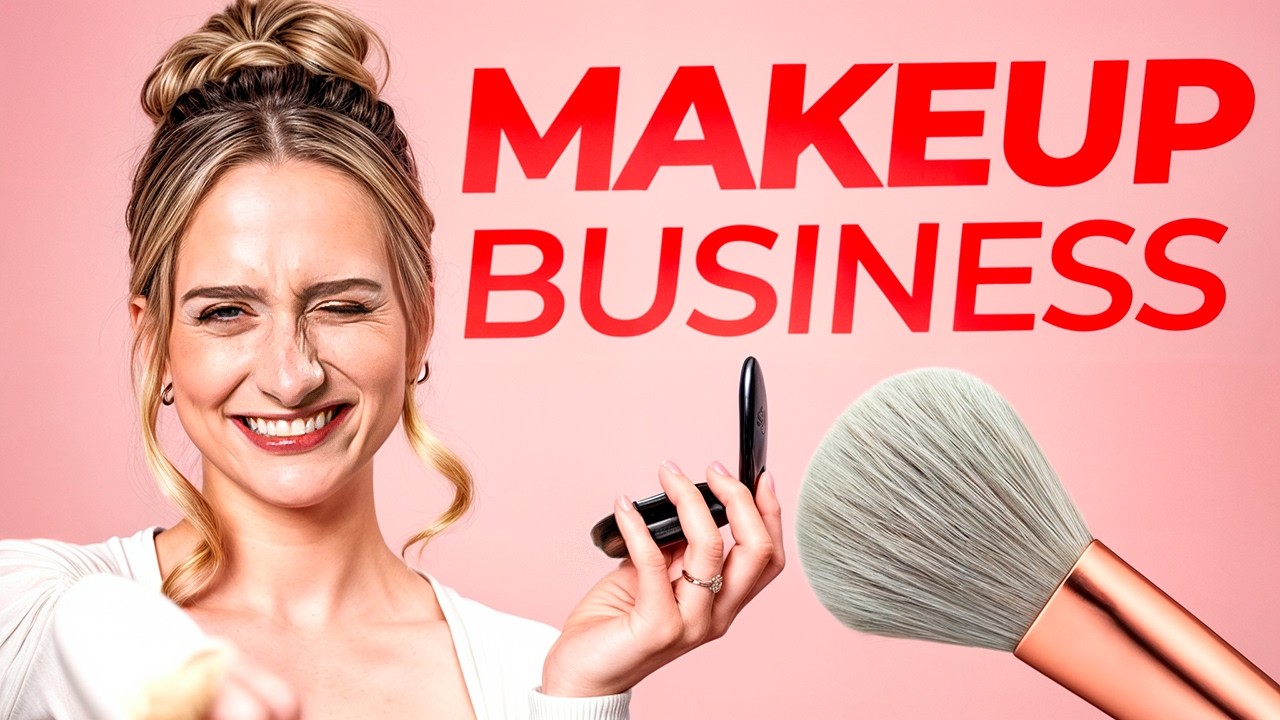
How To Launch A Makeup Brand & Sell Online: The Ultimate Guide
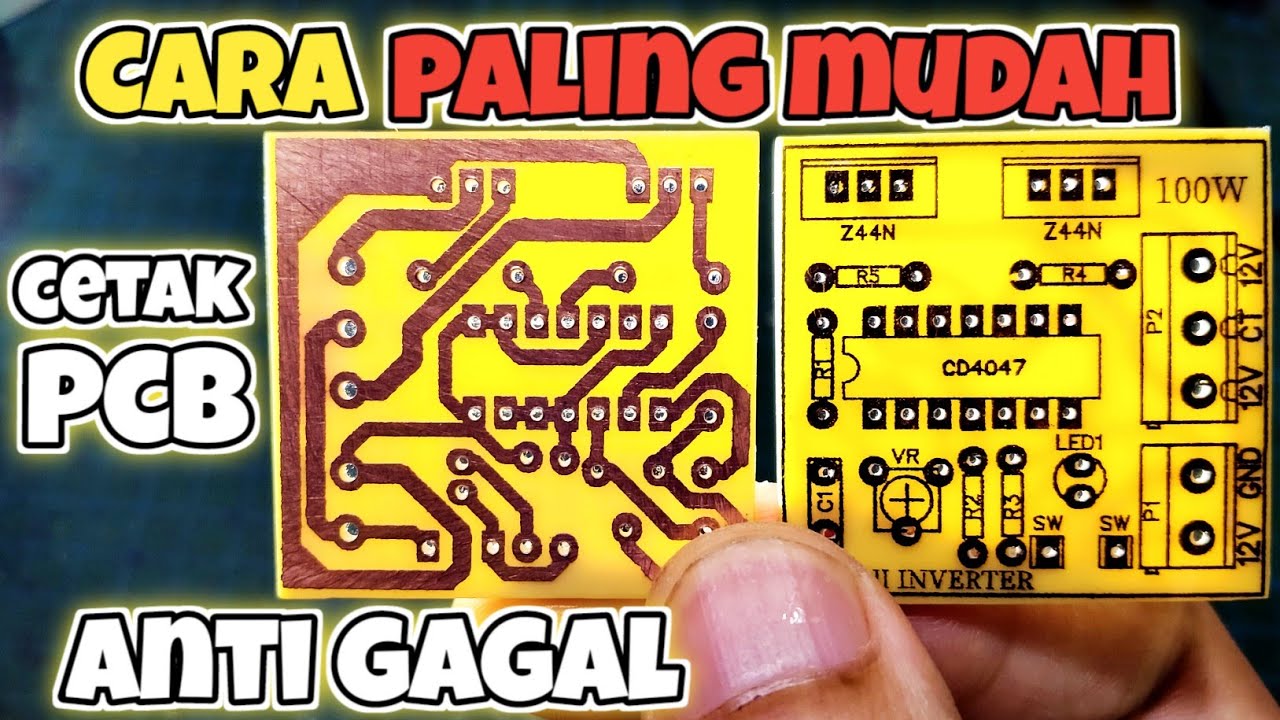
CARA CETAK PCB MANUAL Paling mudah anti gagal
5.0 / 5 (0 votes)