Agentic AI: The Future is here?
Summary
TLDR本视频深入探讨了'代理性AI'(即agentic AI)的概念,指出这一术语常被作为营销炒作。视频首先介绍了代理性AI应具备的特质,如自主性、意图性、自我决定和责任感。随后,通过比较分析大型语言模型(LLMs)和真正的代理性系统,明确了LLMs尽管功能强大,但并不具备真正的代理性。视频还讨论了功能调用(function calling)与代理性的区别,并提出未来AI发展可能的方向,包括利用世界知识模型进行规划。最后,视频呼吁观众区分科学术语和营销术语,鼓励关注真正推动AI发展的科学要素。
Takeaways
- 🧠 LLM(大型语言模型)和RAG(检索增强生成模型)目前并不具备代理性(agentic),即它们没有自主性、自我意识和预定的操作参数。
- 🔍 代理性(agency)涉及独立行动、意向性、自我决定和责任感,通常与生物体相关联,而AI代理则是在编程约束下自主操作的计算系统。
- 🤖 AI代理通过传感器感知环境、进行推理并执行任务以实现预定义的目标,而代理性则指一个实体具有独立行动、意向性和自我决定的固有能力。
- 📚 功能调用(function calling)是LLM的一种能力,允许它们访问外部资源和执行超出文本限制的任务,但这并不等同于代理性。
- 🛠️ RAG系统通过信息检索模块和大型语言模型增强了事实准确性和相关性,但它仍然缺乏代理性。
- 🔑 规划(planning)是实现代理性AI系统的关键功能,涉及目标设定、策略发展、行动排序和执行。
- 🚀 未来的AI系统可能需要超越LLM作为逻辑中心,通过世界知识模型进行规划,这可能涉及到科学法则和自然法则。
- 🔄 目前AI研究正在探索的领域包括如何将LLM中的口头世界知识转化为状态知识,以构建更准确的世界模型。
- 📈 规划功能的提升将增加AI系统的效率、效果、适应性,并减少错误,这在逻辑、医疗、股票交易和制造业等领域尤为重要。
- 🔗 功能调用与规划之间存在明显区别,前者是预定义的、结构化的,而后者则涉及动态的、适应性的决策过程。
- 🎯 尽管目前AI系统尚未达到代理性,但了解它们的局限性有助于我们聚焦于未来发展的关键要素。
Q & A
什么是代理性(Agnetic)AI,它与AI代理有何不同?
-代理性AI指的是具有独立行动、自主决策和对世界施加意志的能力的实体,涉及意图性、自主性和责任感。而AI代理是一个设计用来感知环境、推理并执行动作以实现特定目标的计算系统,它在预定义规则和学习数据的基础上自主操作,不直接涉及人类干预。
大型语言模型(LLMs)是否具有代理性?
-根据脚本中的讨论,LLMs虽然功能强大,但它们并不具备自主性、固有目标或动机,也没有自我效能感,因此不被认为具有代理性。
什么是功能调用(Function Calling),它与代理性有何关联?
-功能调用是一种能力,允许模型调用外部函数或API,以与环境交互、接收新信息或执行特定任务。虽然这增强了LLMs的能力,但它并不等同于代理性,因为功能调用并不涉及独立的目标形成、行动选择和自我监控。
RAG系统是什么,它与标准功能调用AI系统有何不同?
-RAG系统是一个结合了信息检索模块和大型语言模型的系统。与标准功能调用AI系统相比,RAG专注于检索信息,而不是执行更广泛的外部操作或与物理世界互动。
为什么规划(planning)在构建真正智能的AI代理中如此重要?
-规划是智能代理设定目标、制定策略、安排行动序列并执行的能力。它对于提高AI系统的效率、效果、适应性,并减少错误至关重要。
如何理解LLMs与世界模型(Word Model)在规划中的作用?
-LLMs提供了丰富的语言和世界知识,但缺乏执行这些知识的能力。世界模型则基于科学法则和自然法则,提供了一种可能的方式来构建一个能够规划和执行复杂任务的智能系统。
目前AI研究中,关于代理性AI系统的规划有哪些新的发展?
-最新的研究正在探索如何将LLMs的知识和世界模型结合起来,创建一个能够进行复杂规划和决策的系统。这包括使用图结构来表示世界状态和可能的行动路径,以及集成实时感官数据来构建虚拟世界模型。
为什么说目前市场上的一些所谓的'代理性策略'实际上是市场营销术语,而非科学术语?
-一些所谓的'代理性策略',如在Llama指数中提到的,实际上只是使用了LLMs进行决策制定,并没有涉及到真正的代理性行为,如自主性、意图性、自我决定和责任感。因此,这些术语更多是市场营销用语,而非科学上对代理性的准确描述。
AI系统在执行任务时,如何从功能调用转变为更高级的规划能力?
-AI系统可以通过集成更复杂的世界模型和增强的推理能力,从简单的功能调用转变为能够进行高级规划的系统。这可能包括自编码新功能、动态集成新功能以及与环境的实时交互。
目前的AI系统在规划和决策方面存在哪些限制?
-当前的AI系统,特别是基于LLMs的系统,缺乏真正的自主性、意图性、自我决定能力和责任感。它们在适应新任务、学习新情境以及自我监控和调整方面的能力有限。
为什么理解和规划在AI的发展中如此关键?
-理解和规划是实现真正智能代理的关键因素。它们使AI系统能够设定目标、制定策略、安排行动并执行,这对于解决复杂问题和适应不断变化的环境至关重要。
Outlines
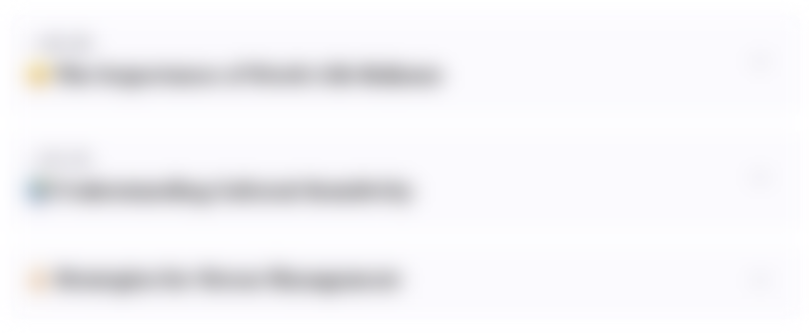
This section is available to paid users only. Please upgrade to access this part.
Upgrade NowMindmap
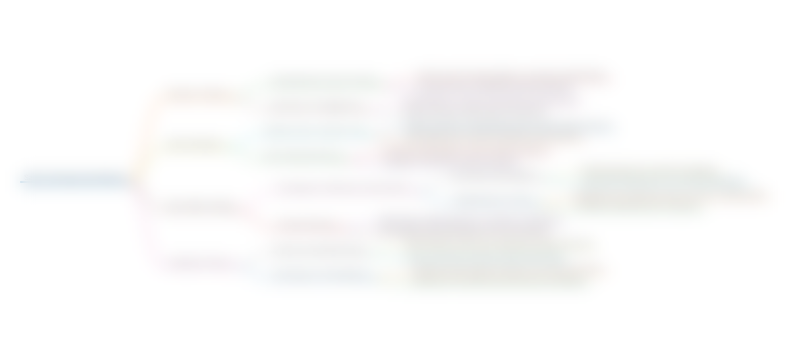
This section is available to paid users only. Please upgrade to access this part.
Upgrade NowKeywords
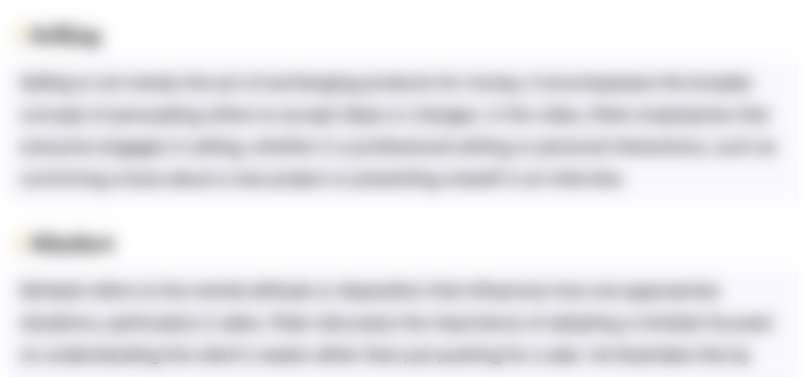
This section is available to paid users only. Please upgrade to access this part.
Upgrade NowHighlights
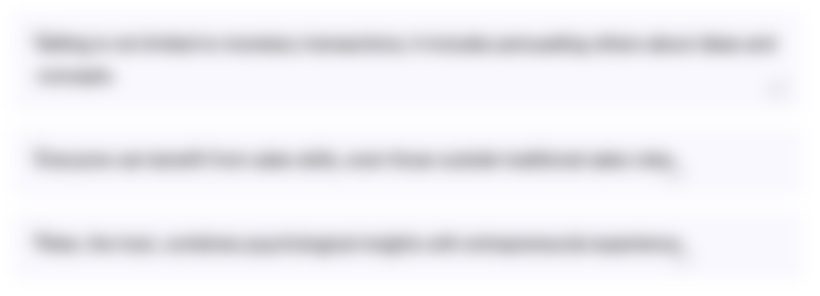
This section is available to paid users only. Please upgrade to access this part.
Upgrade NowTranscripts
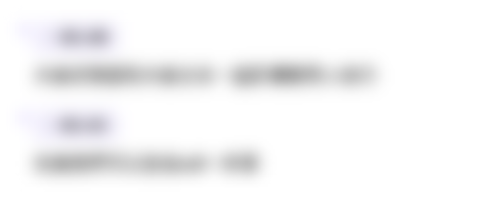
This section is available to paid users only. Please upgrade to access this part.
Upgrade NowBrowse More Related Video
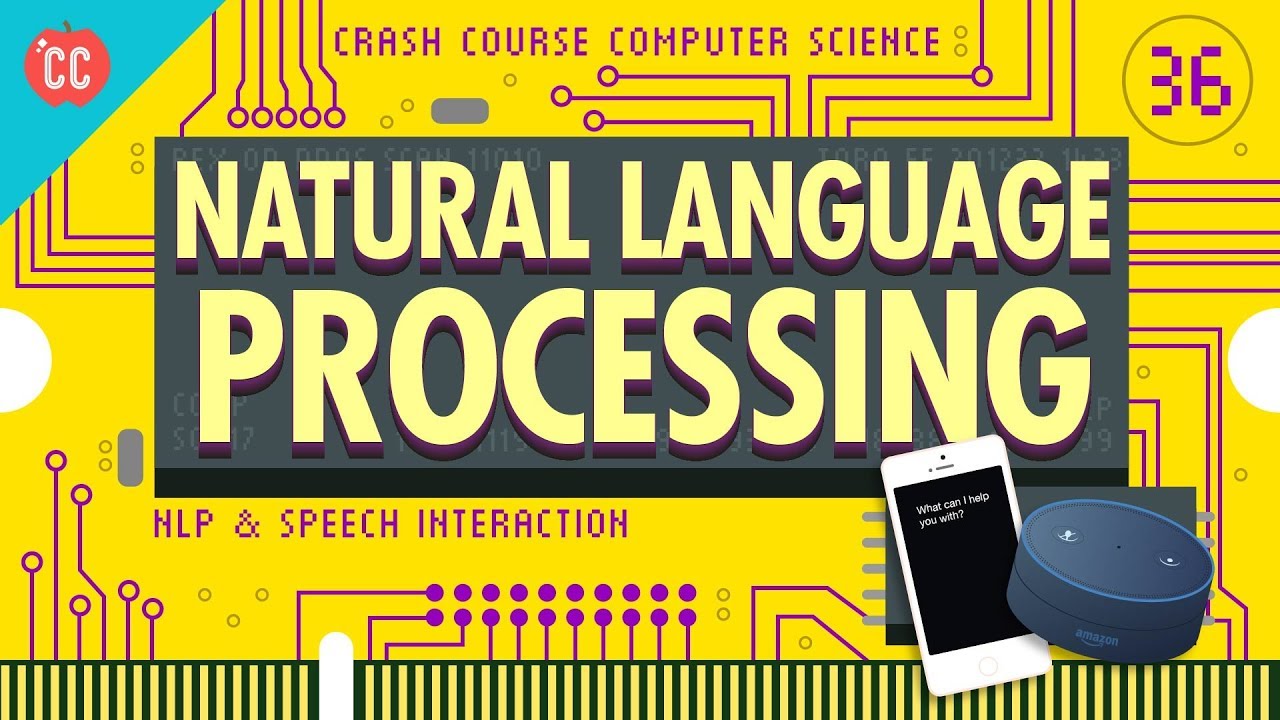
Natural Language Processing: Crash Course Computer Science #36
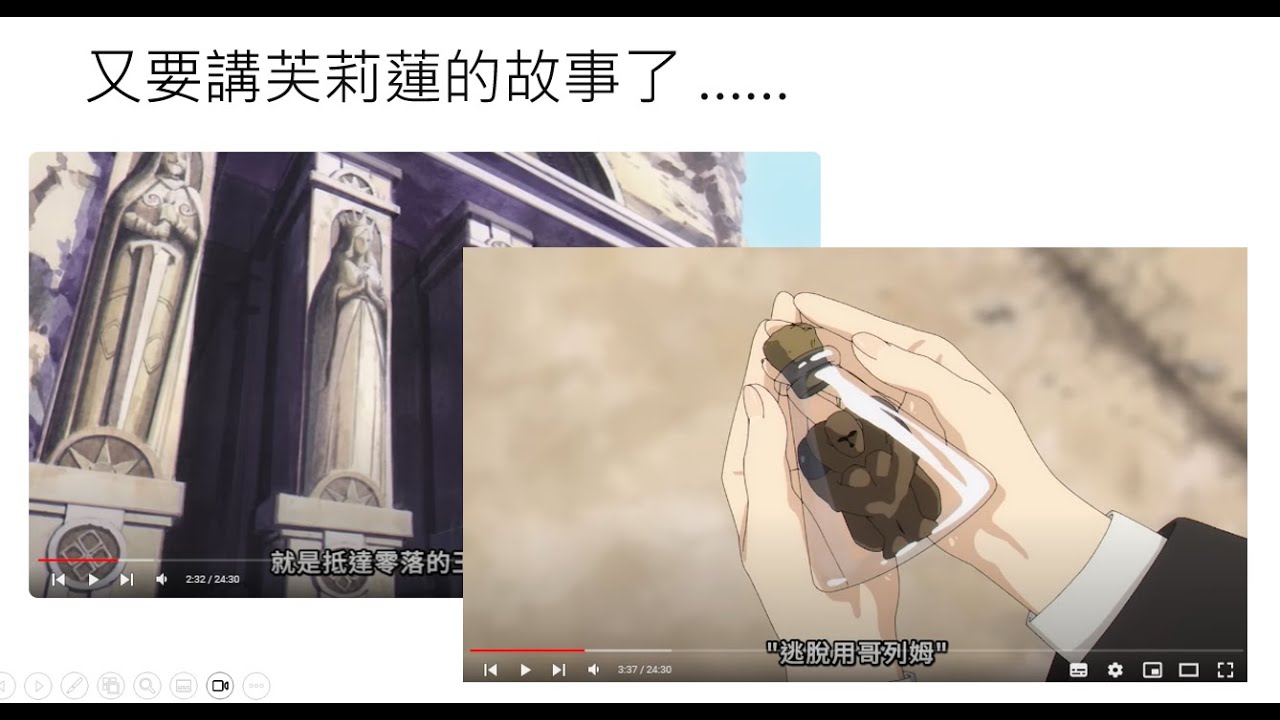
【生成式AI導論 2024】第9講:以大型語言模型打造的AI Agent (14:50 教你怎麼打造芙莉蓮一級魔法使考試中出現的泥人哥列姆)
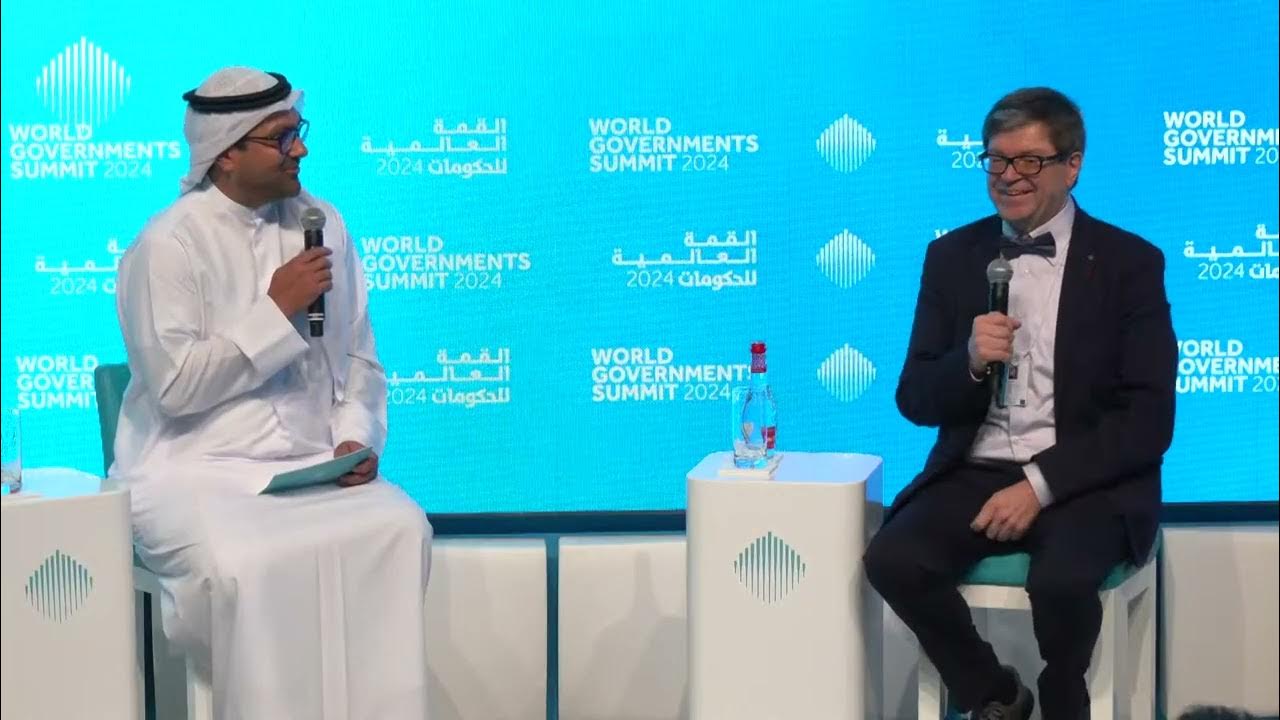
《與楊立昆的對話:人工智能是生命線還是地雷?》- World Governments Summit
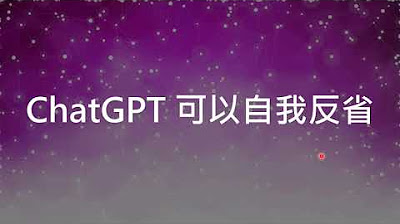
【生成式AI】ChatGPT 可以自我反省!
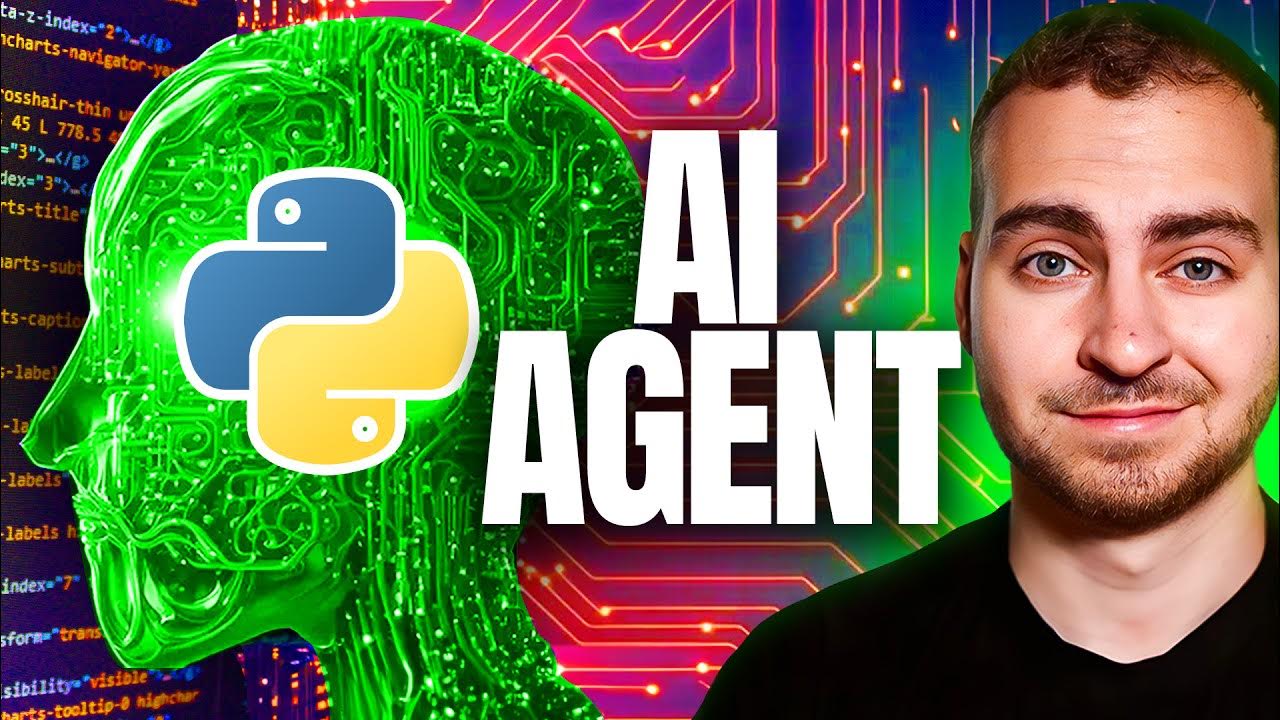
Python Advanced AI Agent Tutorial - LlamaIndex, Ollama and Multi-LLM!
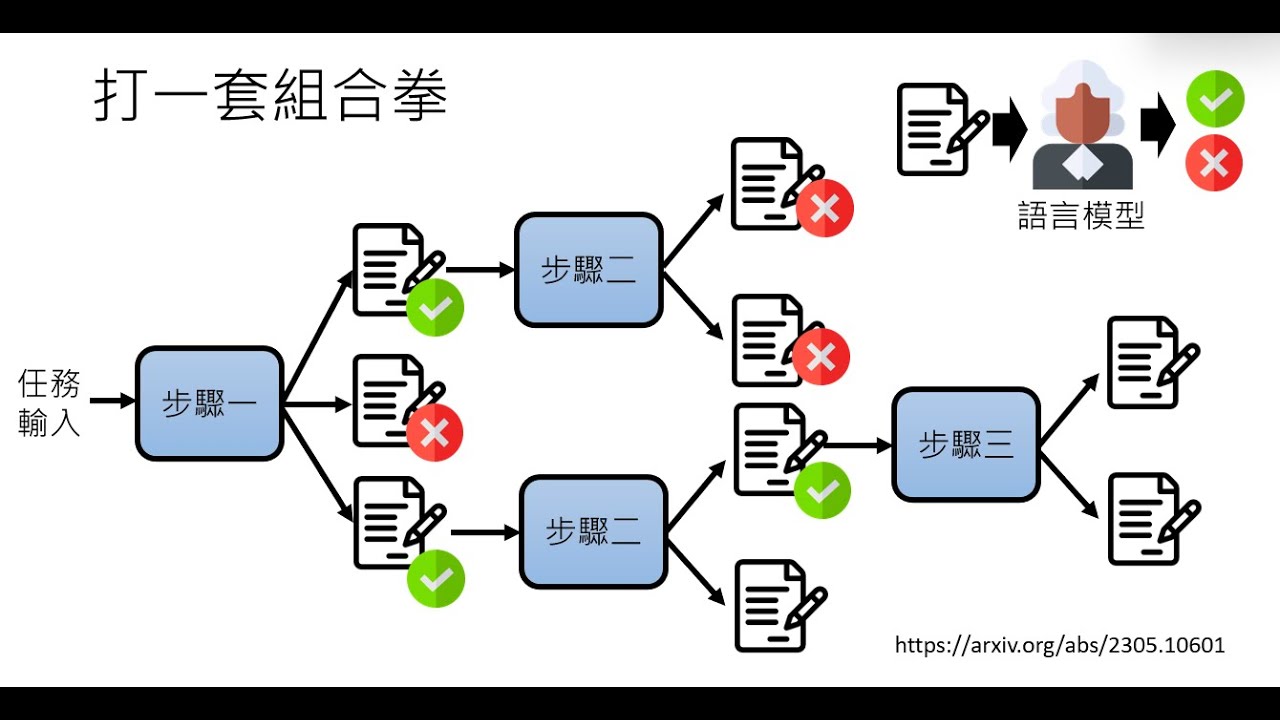
【生成式AI導論 2024】第4講:訓練不了人工智慧?你可以訓練你自己 (中) — 拆解問題與使用工具
5.0 / 5 (0 votes)