Feedforward Neural Networks and Backpropagation - Part 1
Summary
TLDRThis video lecture delves into the workings of multi-layer perceptrons (MLPs), a type of feedforward neural network. It explains how these networks, composed of layers of neurons, can approximate complex functions by mapping input to output. The lecture covers the process of training MLPs using gradient descent, focusing on how the loss function is minimized through iterative updates of weights and biases. By employing backpropagation and the chain rule, the network learns to adjust its parameters to improve performance. Key concepts like loss functions, gradient descent, and the importance of the learning rate are also discussed.
Please replace the link and try again.
Please replace the link and try again.
Outlines
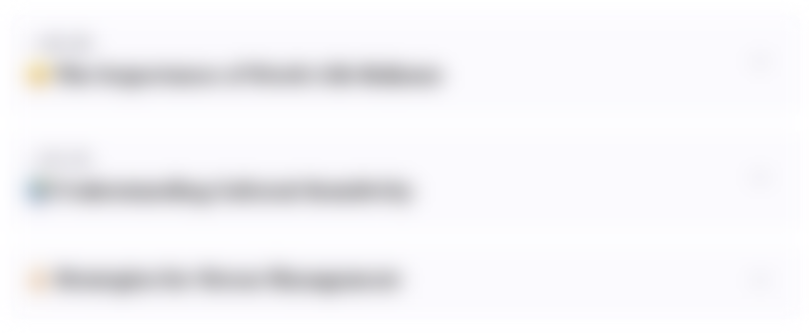
This section is available to paid users only. Please upgrade to access this part.
Upgrade NowMindmap
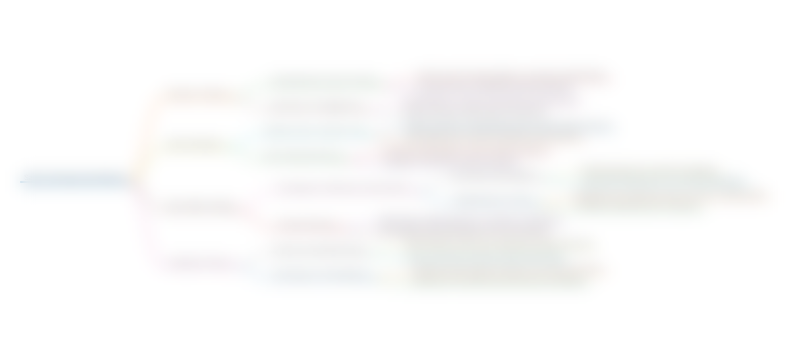
This section is available to paid users only. Please upgrade to access this part.
Upgrade NowKeywords
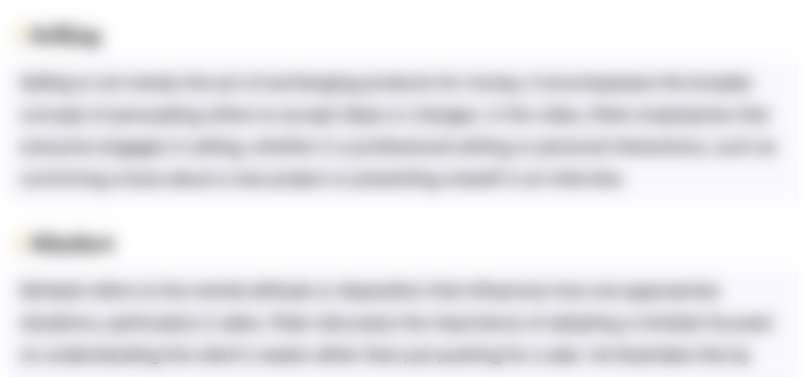
This section is available to paid users only. Please upgrade to access this part.
Upgrade NowHighlights
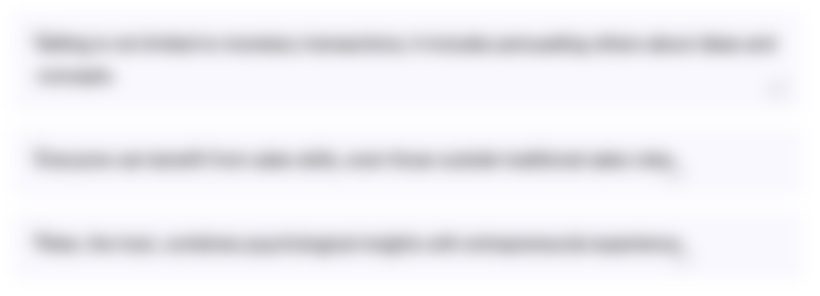
This section is available to paid users only. Please upgrade to access this part.
Upgrade NowTranscripts
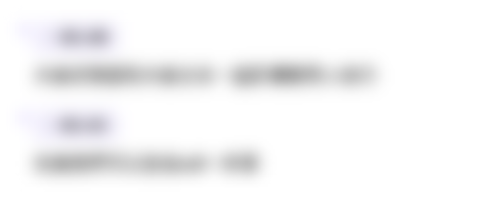
This section is available to paid users only. Please upgrade to access this part.
Upgrade NowBrowse More Related Video

Feedforward and Feedback Artificial Neural Networks
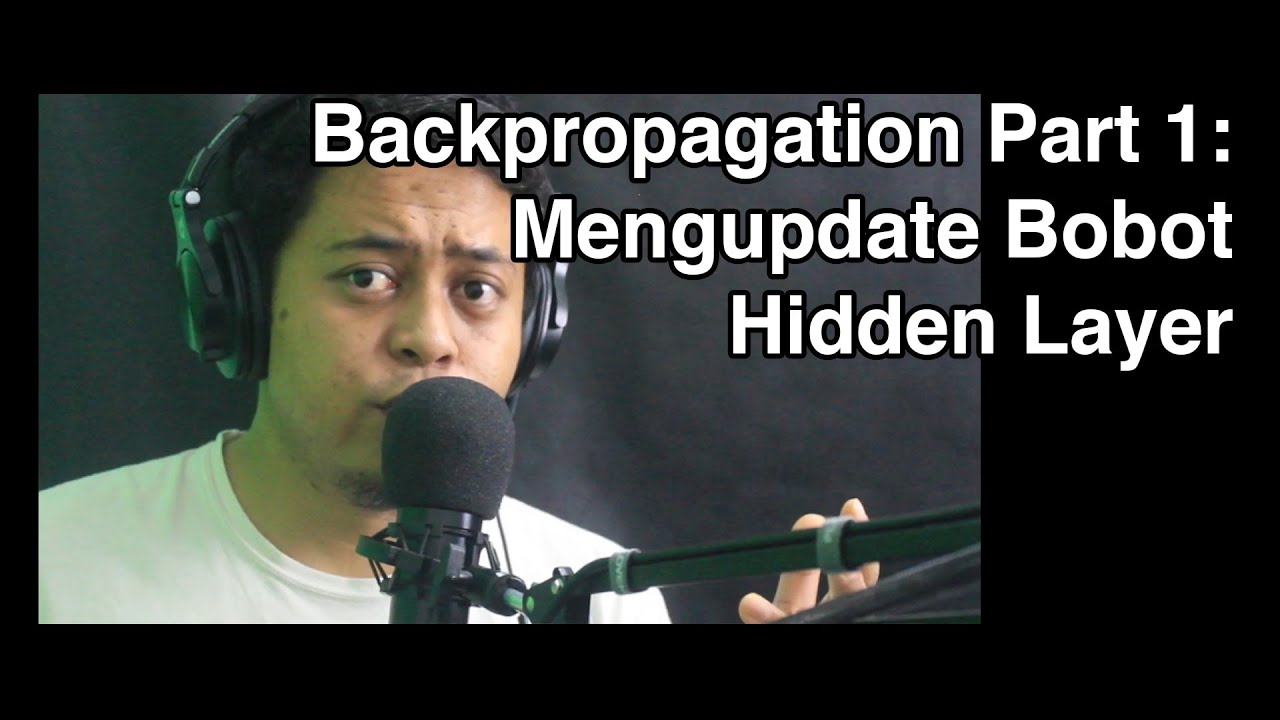
Backpropagation Part 1: Mengupdate Bobot Hidden Layer | Machine Learning 101 | Eps 15
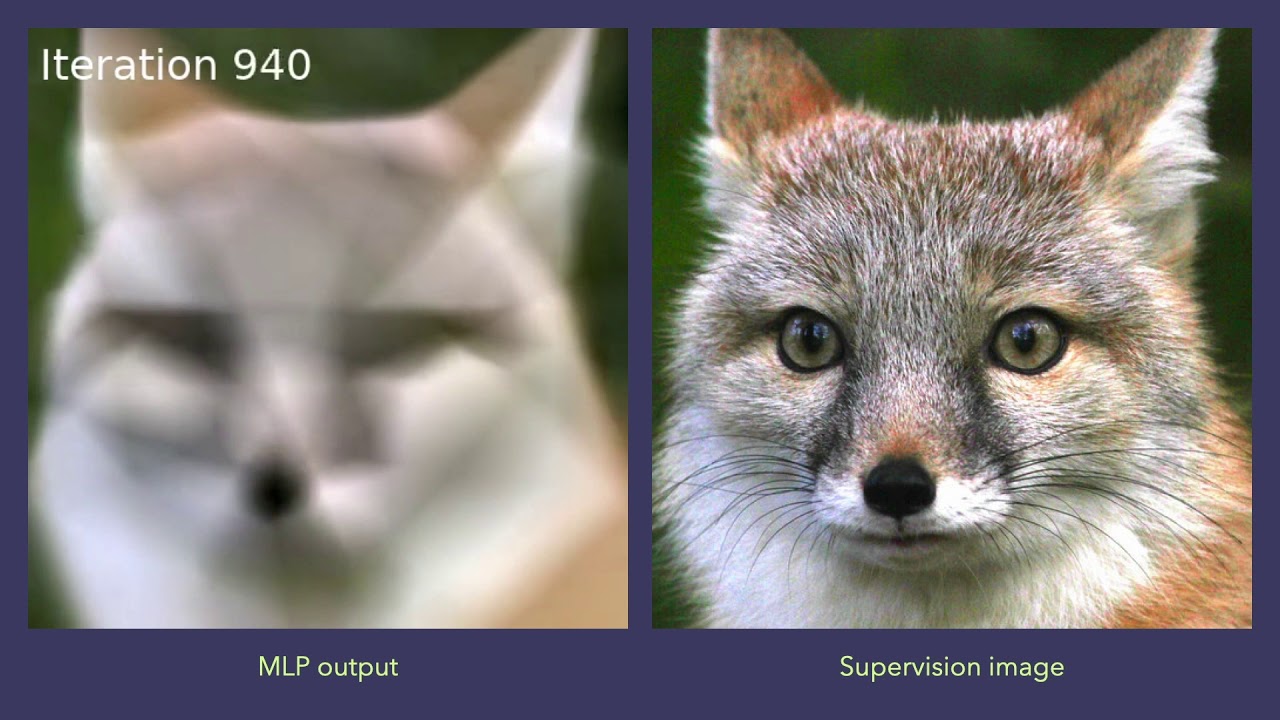
Fourier Features Let Networks Learn High Frequency Functions in Low Dimensional Domains

Taxonomy of Neural Network
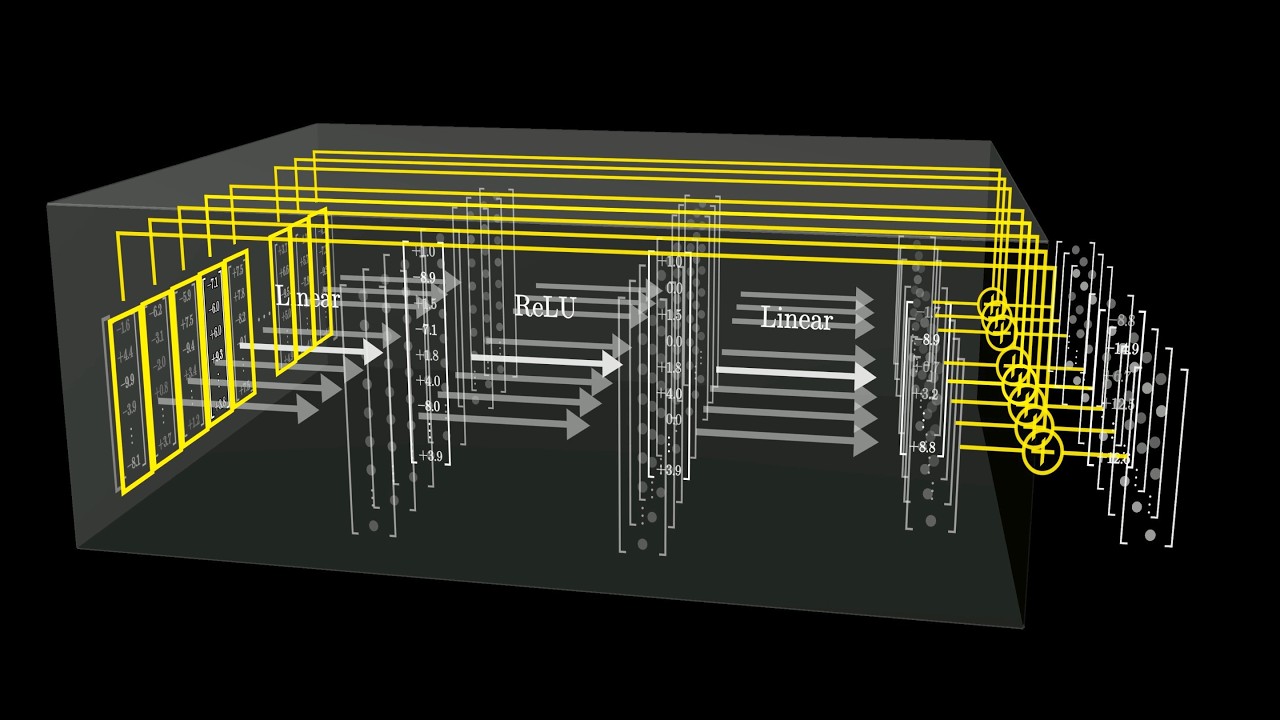
How might LLMs store facts | Chapter 7, Deep Learning
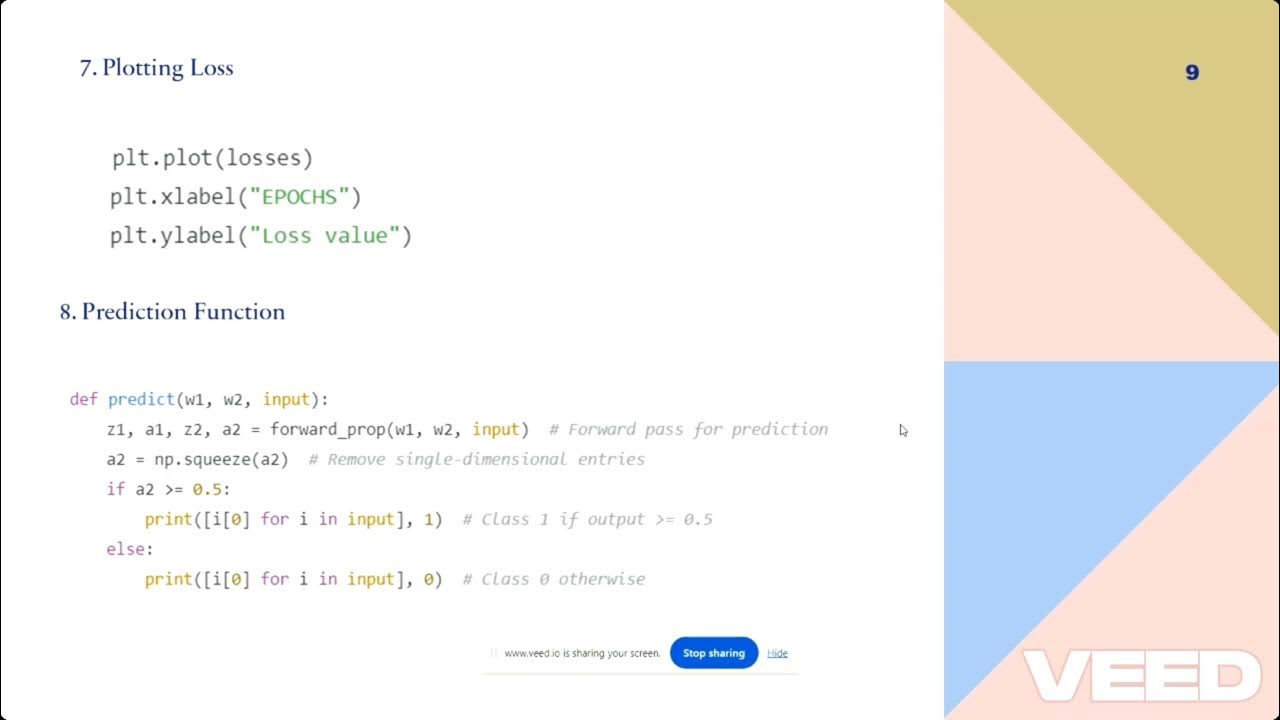
Multi-layer perceptron on any non-linearly separable data
5.0 / 5 (0 votes)