Reinforcement Fine-Tuning—12 Days of OpenAI: Day 2
Summary
TLDROpenAI's new reinforcement fine-tuning (RFT) for the O1 series models enhances AI's ability to reason over custom domains, enabling breakthrough advancements in complex fields like healthcare and law. By applying reinforcement learning, RFT allows models to learn new ways of reasoning based on expert data sets, improving their performance in specialized tasks. This technique has been successfully used for diagnosing rare genetic diseases and refining legal workflows. OpenAI is expanding access to its RFT program, inviting organizations to customize models for their specific use cases, with broader public availability planned for next year.
Takeaways
- 😀 OpenAI launched reinforcement fine-tuning (RFT) for its 01 model series, enabling more customized AI models for specific domains.
- 😀 RFT allows models to reason in entirely new ways over custom datasets, offering a unique advantage over traditional fine-tuning methods.
- 😀 The technology leverages reinforcement learning algorithms that help models improve by reinforcing correct reasoning and disincentivizing incorrect answers.
- 😀 Researchers and enterprises in fields like law, finance, healthcare, and engineering can now customize AI models for their specific needs using RFT.
- 😀 Reinforcement fine-tuning has shown promising results in domains like rare disease research, where AI models can predict genetic mutations from symptoms.
- 😀 OpenAI's collaboration with Berkeley Lab demonstrates the application of RFT in improving the prediction of rare genetic diseases by enhancing model reasoning.
- 😀 The program is currently in an alpha phase, with plans to expand early access to organizations with complex tasks requiring expert knowledge.
- 😀 Supervised fine-tuning is different from RFT as it focuses on mimicking input data patterns, while RFT enables models to learn complex reasoning over custom domains.
- 😀 OpenAI plans to launch the reinforcement fine-tuning product publicly in 2025, expanding its availability to a broader user base.
- 😀 RFT can drastically improve the performance of smaller, more cost-efficient models like 01 Mini, providing better results without requiring larger, more expensive models.
Q & A
What is reinforcement fine-tuning (RFT) and how does it differ from traditional supervised fine-tuning?
-Reinforcement fine-tuning (RFT) is a model customization technique that uses reinforcement learning algorithms to fine-tune AI models based on feedback. Unlike traditional supervised fine-tuning, which adjusts models by mimicking input data, RFT helps models reason through tasks by reinforcing correct answers and discouraging incorrect ones. This allows for the development of expert models that can excel at specific tasks in specialized domains.
Why is reinforcement fine-tuning important for fields like law, healthcare, and finance?
-Reinforcement fine-tuning is important for these fields because it enables AI models to reason over complex, domain-specific data. In sectors such as law, healthcare, and finance, tasks often require deep expertise, and RFT allows AI models to perform tasks that demand systematic reasoning, making them more efficient and effective at solving specialized problems.
What was the example of reinforcement fine-tuning used in the transcript, and how did it help scientific research?
-One key example was in genomic research, where reinforcement fine-tuning was used to help identify genetic mutations causing rare diseases. The AI model was fine-tuned with data from scientific publications and patient reports to predict which genes might be responsible for a patient's symptoms. This helped researchers in the field of rare diseases better understand genetic causes and speed up the diagnostic process.
How does reinforcement fine-tuning improve model performance with small datasets?
-Reinforcement fine-tuning allows models to learn new reasoning strategies with as little as a few dozen examples. This is much more efficient than traditional fine-tuning methods, which require large amounts of data to adjust models. Through reinforcement learning, the model can improve its reasoning skills even with limited input, making it highly adaptable to specific tasks.
What is the significance of the 'Top-K accuracy' evaluation metric?
-'Top-K accuracy' is a metric used to measure how often the correct answer appears in the top K positions of the model's predicted list. It provides a way to assess the quality of model predictions, especially in tasks like ranking, where the model is expected to predict the most relevant outcomes. In the context of reinforcement fine-tuning, this metric is crucial for determining how well the model is generalizing and improving its predictions over time.
How does the grading system work in reinforcement fine-tuning?
-The grading system in reinforcement fine-tuning involves comparing the model’s output to the correct answer. The grader assigns a score between 0 and 1, with partial credits given depending on how close the model’s answer is to the correct one. This scoring helps reinforce correct reasoning paths and penalizes incorrect ones, ensuring that the model learns to perform tasks more accurately over time.
What was the role of 'graders' in the reinforcement fine-tuning process?
-Graders are used to assess the quality of the model's outputs during training. They compare the model's predictions to the correct answers and assign a score based on how accurate or close the prediction is. This feedback loop helps refine the model's reasoning process, allowing it to improve over multiple training cycles.
Can reinforcement fine-tuning be applied to non-scientific tasks, and if so, how?
-Yes, reinforcement fine-tuning is a general-purpose technique that can be applied to a wide range of tasks beyond scientific research. It has shown promising results in fields like AI safety, legal processes, and business applications. By customizing AI models to specific tasks within these domains, reinforcement fine-tuning can help optimize performance, improve reasoning, and automate complex workflows.
How does the reinforcement fine-tuning process help researchers and developers?
-The process allows researchers and developers to leverage OpenAI's reinforcement learning algorithms and model training infrastructure, enabling them to customize AI models for their specific needs. By providing their own datasets and grading criteria, they can tailor models to excel at specialized tasks without needing to build complex machine learning systems from scratch.
What future developments are expected in reinforcement fine-tuning?
-Future developments include expanding access to the reinforcement fine-tuning program for more organizations, allowing them to apply this technique to a wider variety of tasks. Additionally, OpenAI plans to allow users to create custom graders, providing even greater flexibility for model customization. The product is expected to be publicly available by early 2025.
Outlines
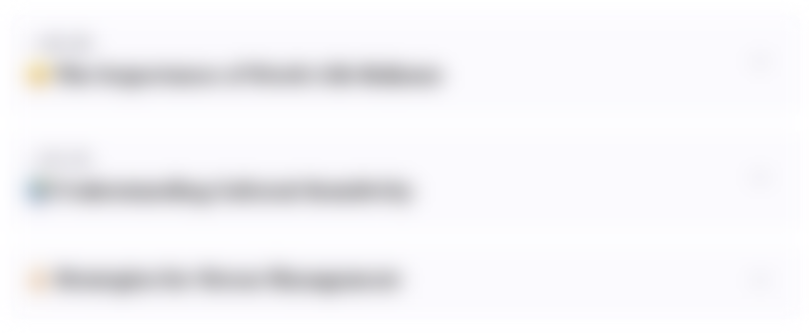
This section is available to paid users only. Please upgrade to access this part.
Upgrade NowMindmap
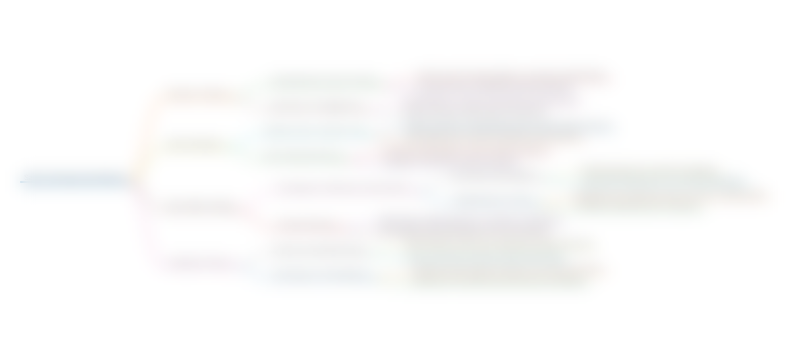
This section is available to paid users only. Please upgrade to access this part.
Upgrade NowKeywords
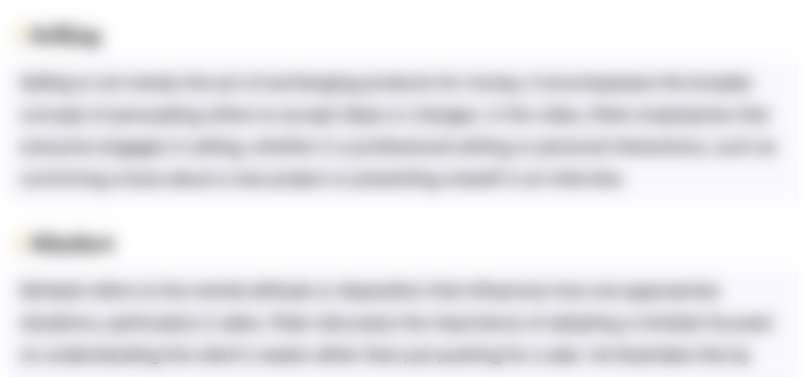
This section is available to paid users only. Please upgrade to access this part.
Upgrade NowHighlights
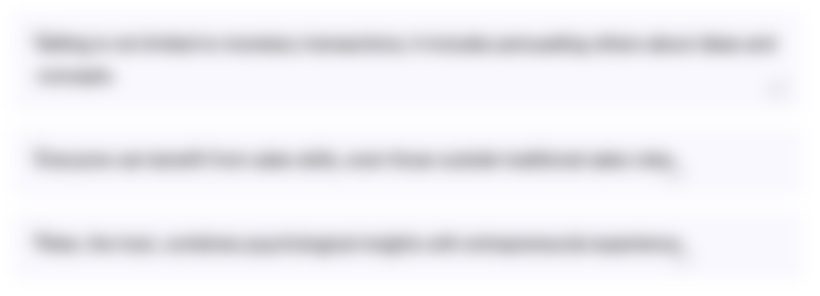
This section is available to paid users only. Please upgrade to access this part.
Upgrade NowTranscripts
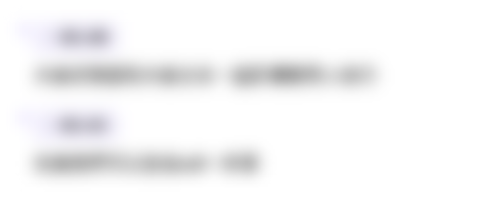
This section is available to paid users only. Please upgrade to access this part.
Upgrade NowBrowse More Related Video
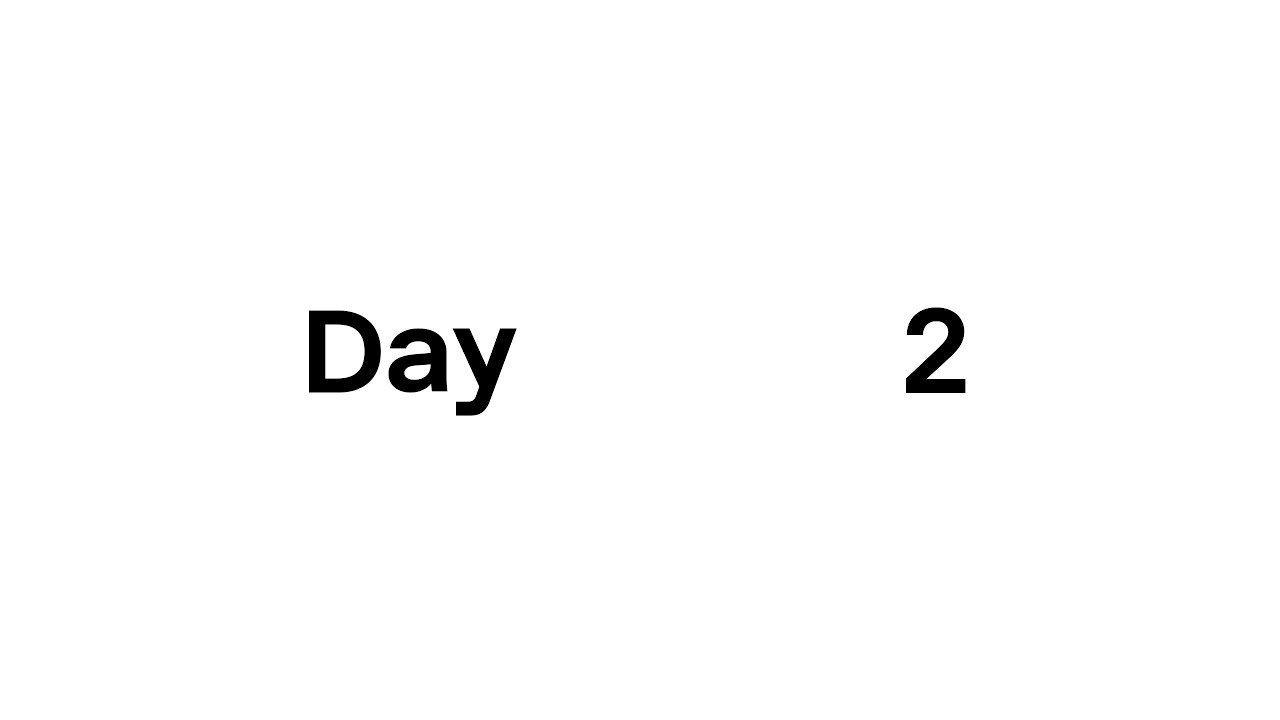
12 Days of OpenAI: Day 2
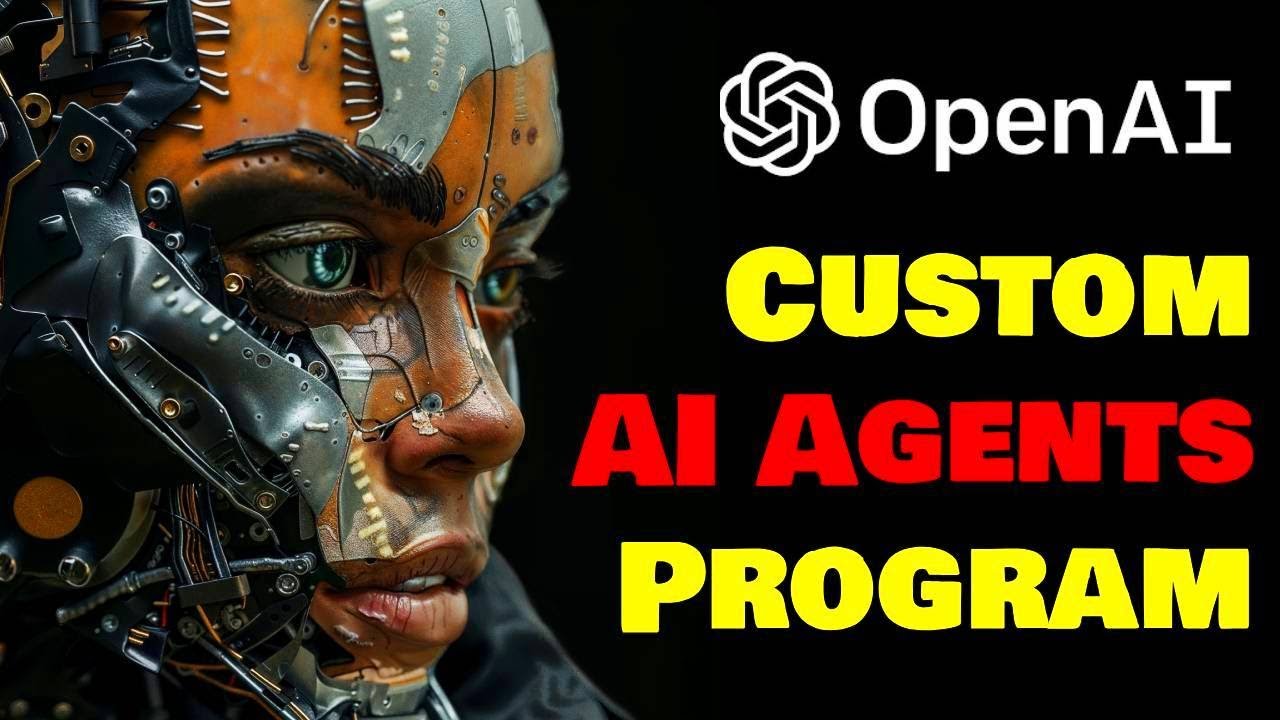
OpenAI's STUNNING "GPT-based agents" for Businesses | Custom Models for Industries | AI Flywheels
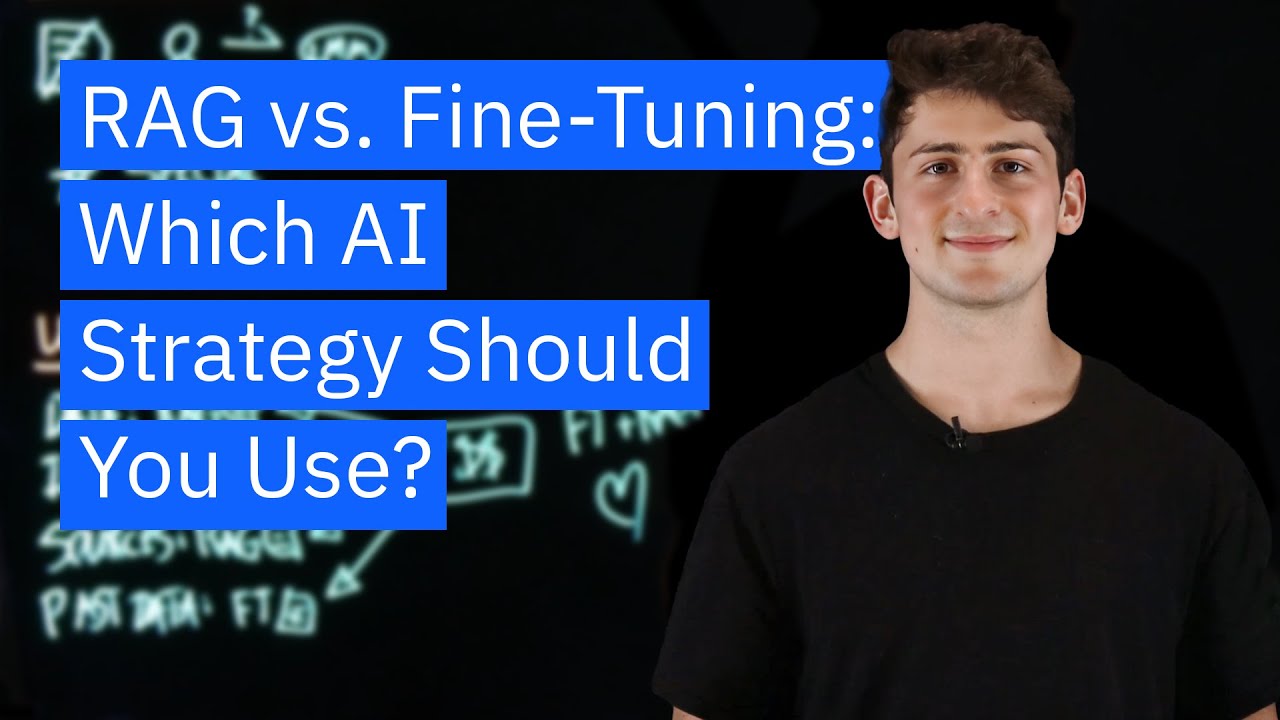
RAG vs. Fine Tuning
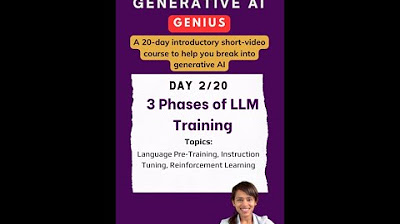
Introduction to Generative AI (Day 2/20) How are LLMs Trained?

5 MINUTES AGO: OpenAI Just Released GPT-o1 the Most Powerful AI Model Yet
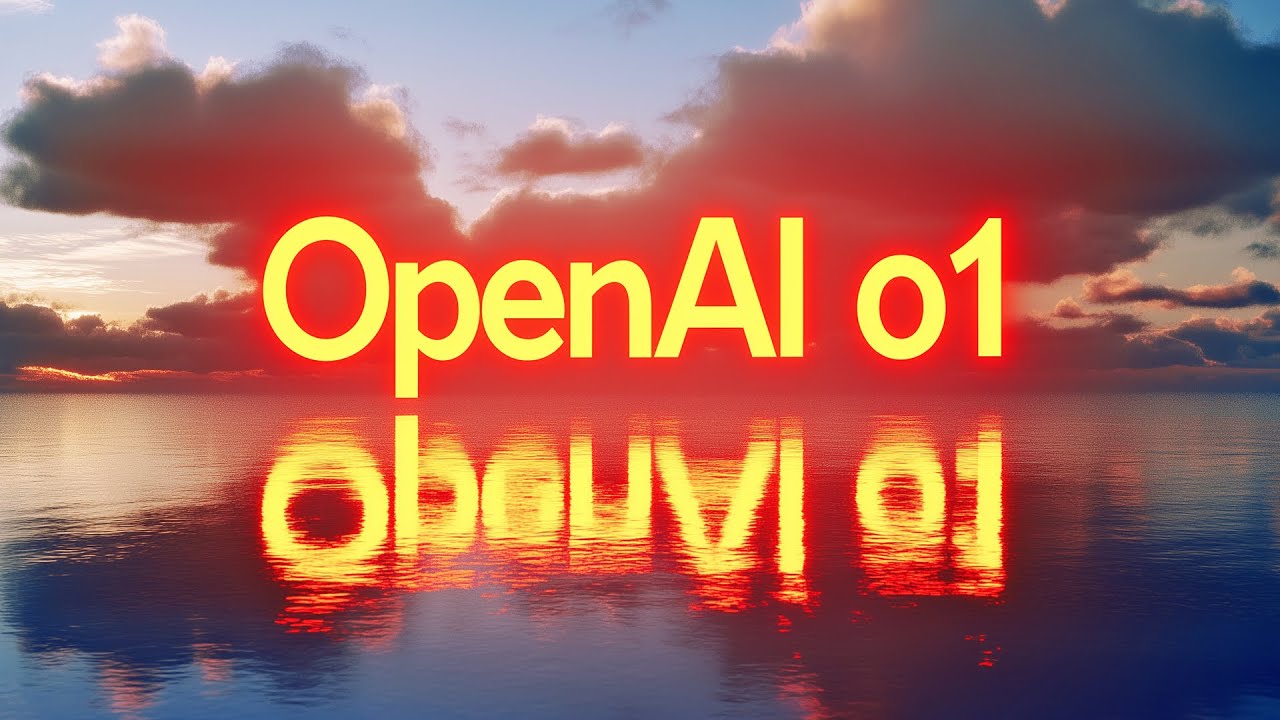
OpenAI o1: ChatGPT Supercharged!
5.0 / 5 (0 votes)