Discrete random variables | Methods 3 and 4 | MaffsGuru
Summary
TLDRThis video introduces the concept of discrete random variables, explaining their difference from continuous variables. Using the example of drawing white and black balls from a jar, it demonstrates how to calculate probabilities for different outcomes, like drawing exactly one white ball in four draws. The video emphasizes understanding probability distributions, creating tables to organize probabilities, and interpreting the results. It also covers key topics like conditional probability and highlights the importance of correct interpretation in probability functions. Overall, it's an essential lesson for understanding basic discrete probability concepts and preparing for more advanced topics.
Takeaways
- 😀 A random variable is a variable whose outcome cannot be predicted in advance.
- 😀 A discrete random variable can only take whole number values, like the number of white balls drawn from a bag.
- 😀 Discrete random variables differ from continuous ones, which can have real numbers with decimals, such as height or weight.
- 😀 The instructor uses an example of drawing balls from a bag with four white and six black balls to demonstrate discrete random variables.
- 😀 Probabilities for discrete random variables can be calculated by listing all possible outcomes and their respective probabilities.
- 😀 In the example, the probability of drawing exactly one white ball out of four draws with replacement is 0.3456.
- 😀 A probability distribution table for a discrete random variable lists all possible outcomes and their associated probabilities.
- 😀 The sum of all probabilities in a discrete probability distribution must equal 1, as it represents all possible outcomes.
- 😀 Conditional probability is important, and it can be calculated by determining the likelihood of an event under certain conditions.
- 😀 When working with probability tables, it's crucial to ensure that the values fall between 0 and 1 and that they add up to 1.
- 😀 The key takeaway is to understand how discrete random variables work, how to create probability distributions, and ensure that probabilities follow the rules.
Q & A
What is a random variable?
-A random variable is an element that can take different values based on the outcome of a random event. It is a variable whose outcome is unpredictable.
What is the difference between a discrete and a continuous random variable?
-A discrete random variable takes only whole number values (e.g., the number of people, or the number of white balls drawn from a bag), whereas a continuous random variable can take any real number value (e.g., height, weight, or time).
What does it mean when a random variable is discrete?
-A discrete random variable is one that takes specific, countable values, typically whole numbers, such as the number of white balls drawn from a bag or the number of people in a group.
What is the probability distribution for a discrete random variable?
-A probability distribution for a discrete random variable lists all possible outcomes of the variable and the probability of each outcome. The sum of all probabilities in the distribution must equal 1.
How do you calculate the probability of drawing exactly one white ball from a bag of 10 balls (4 white and 6 black) when drawing 4 balls with replacement?
-To calculate the probability of drawing exactly one white ball, you multiply the probability of drawing a white ball (0.4) and a black ball (0.6) across all four draws, considering the number of different combinations (outcomes) for one white ball. This results in a probability of 0.3456.
What is the role of combinations when calculating probabilities for discrete random variables?
-Combinations help determine how many different ways an event can occur, such as the different ways to draw one white ball from a set of 4 draws. These combinations are used to adjust the probability calculations based on the number of possible outcomes.
Why is the concept of 'replacement' important in probability calculations?
-Replacement is important because it ensures the probabilities remain constant throughout the trials. In the case of drawing balls from a bag with replacement, the probability of drawing a white or black ball remains unchanged after each draw.
How can we express the probability of a specific outcome using a probability table?
-A probability table can be used to express the probability of each possible outcome of a discrete random variable. For example, if you are drawing balls from a bag, the table can show the probability of drawing 0, 1, 2, 3, or 4 white balls out of 4 total draws.
What should be true about the sum of all probabilities in a probability distribution?
-The sum of all probabilities in a probability distribution for a discrete random variable must equal 1. This ensures that all possible outcomes have been accounted for.
What is conditional probability, and how is it used in the context of discrete random variables?
-Conditional probability is the probability of an event occurring given that another event has already occurred. In the context of discrete random variables, it is used to refine probability calculations when additional information or conditions are provided.
Outlines
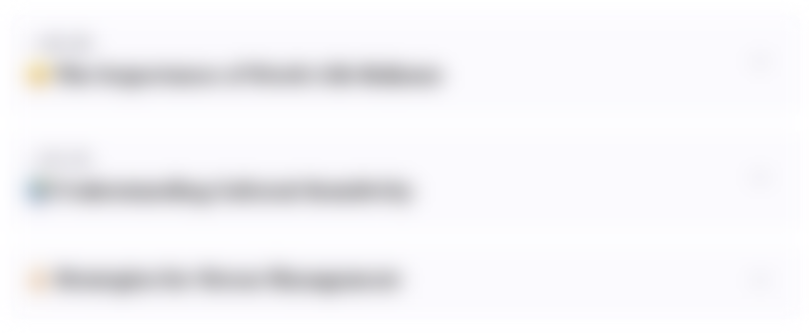
This section is available to paid users only. Please upgrade to access this part.
Upgrade NowMindmap
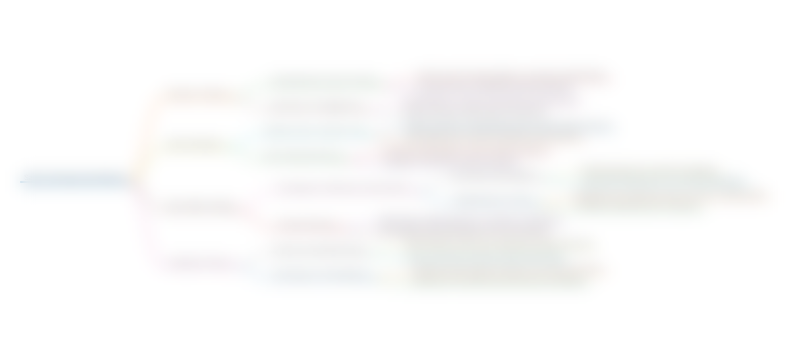
This section is available to paid users only. Please upgrade to access this part.
Upgrade NowKeywords
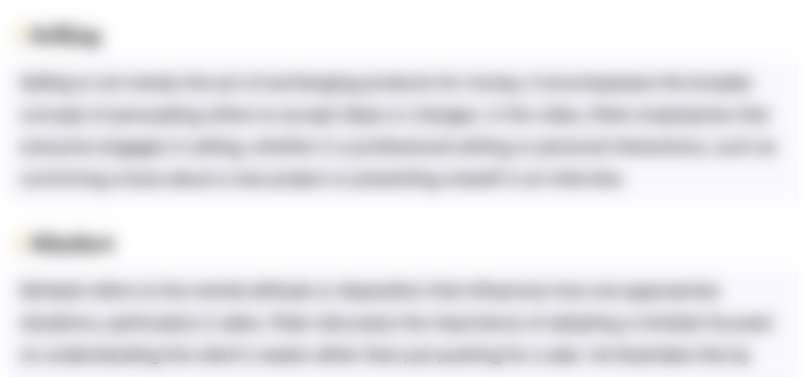
This section is available to paid users only. Please upgrade to access this part.
Upgrade NowHighlights
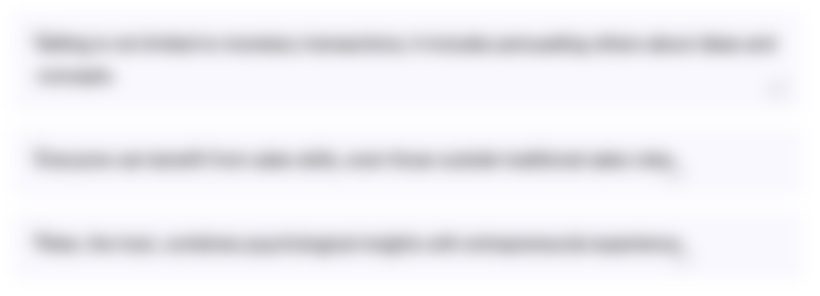
This section is available to paid users only. Please upgrade to access this part.
Upgrade NowTranscripts
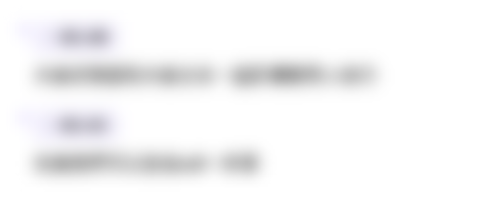
This section is available to paid users only. Please upgrade to access this part.
Upgrade NowBrowse More Related Video

EXPLORING RANDOM VARIABLES | DISCRETE AND CONTINUOUS | PROF D
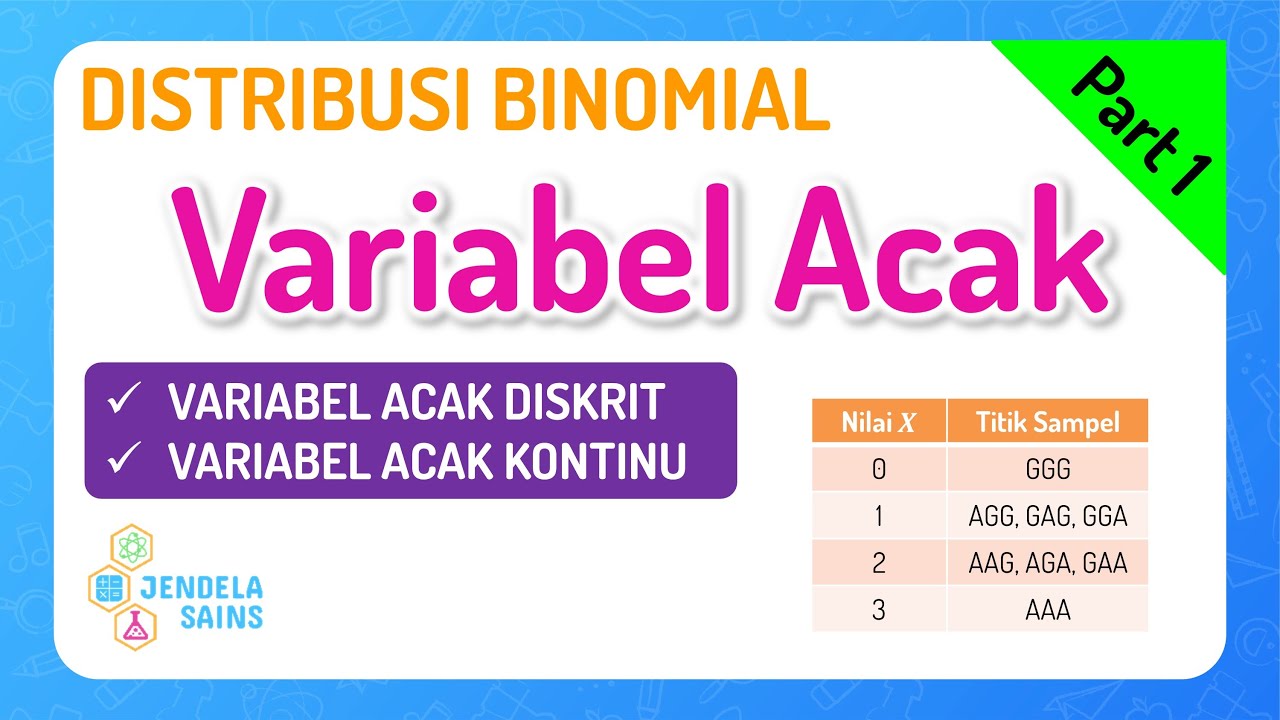
Distribusi Binomial • Part 1: Variabel Acak
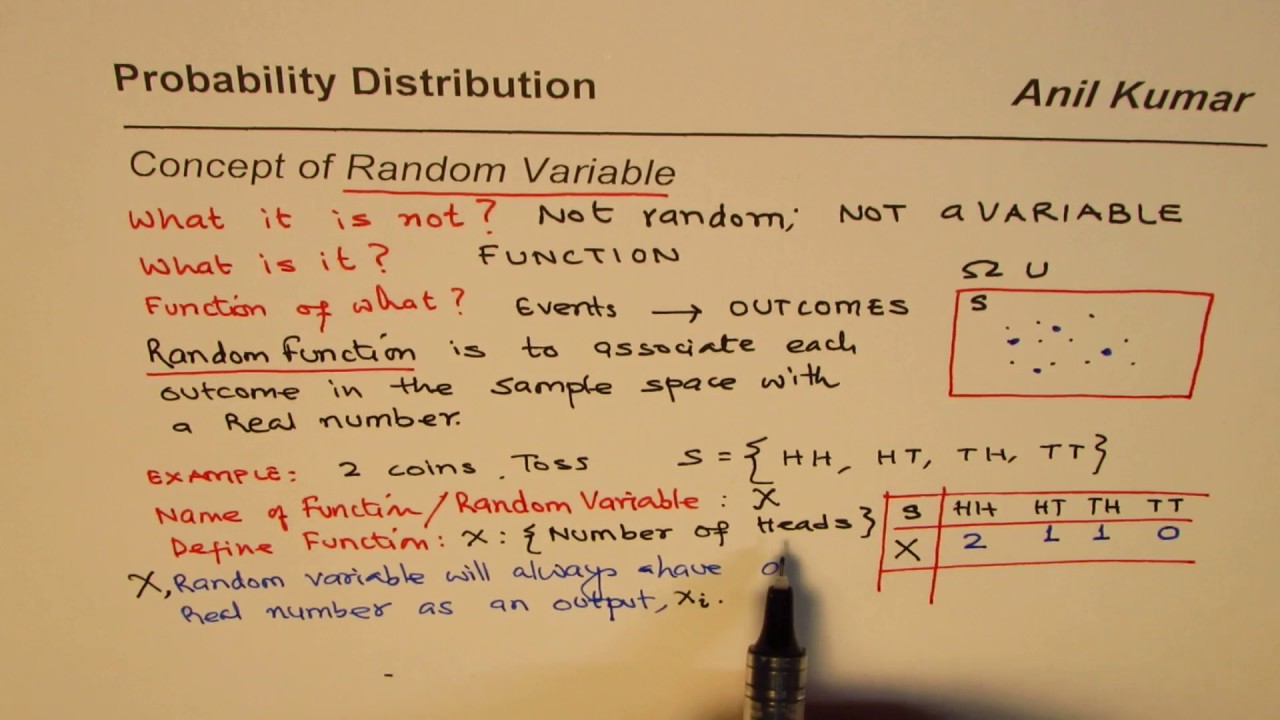
Introduction to Random Variables Probability Distribution
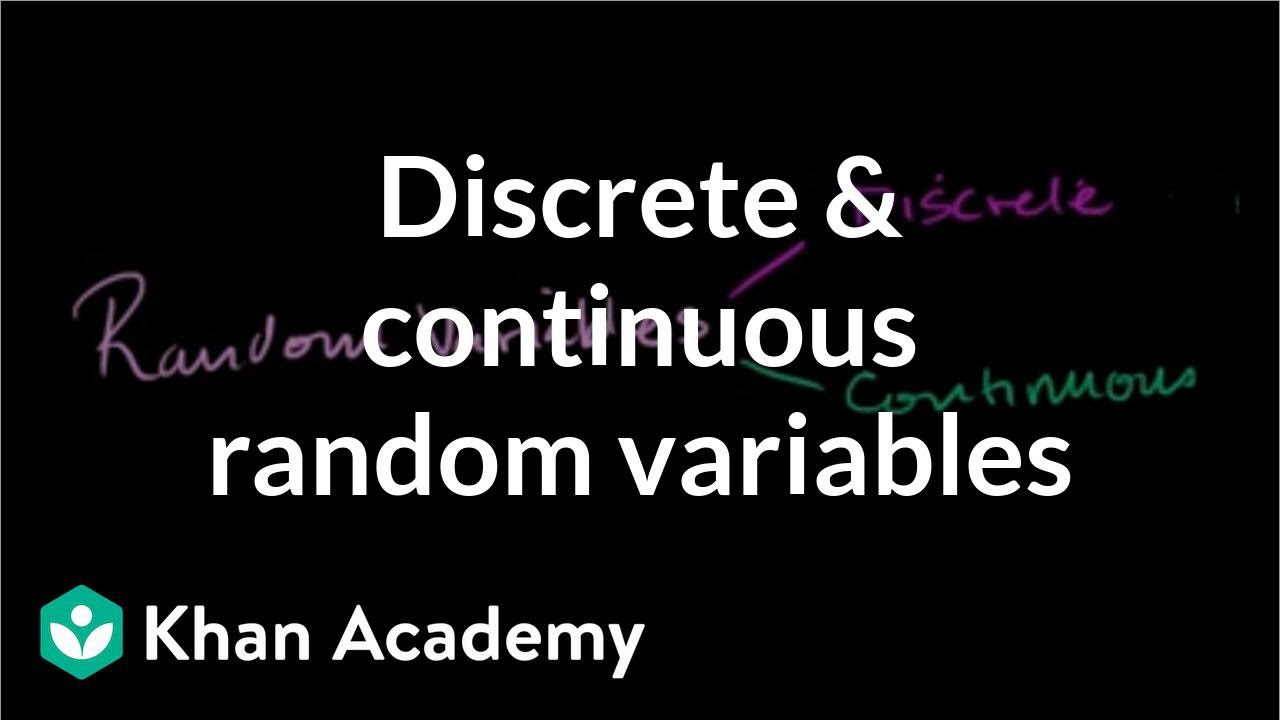
Discrete and continuous random variables | Probability and Statistics | Khan Academy
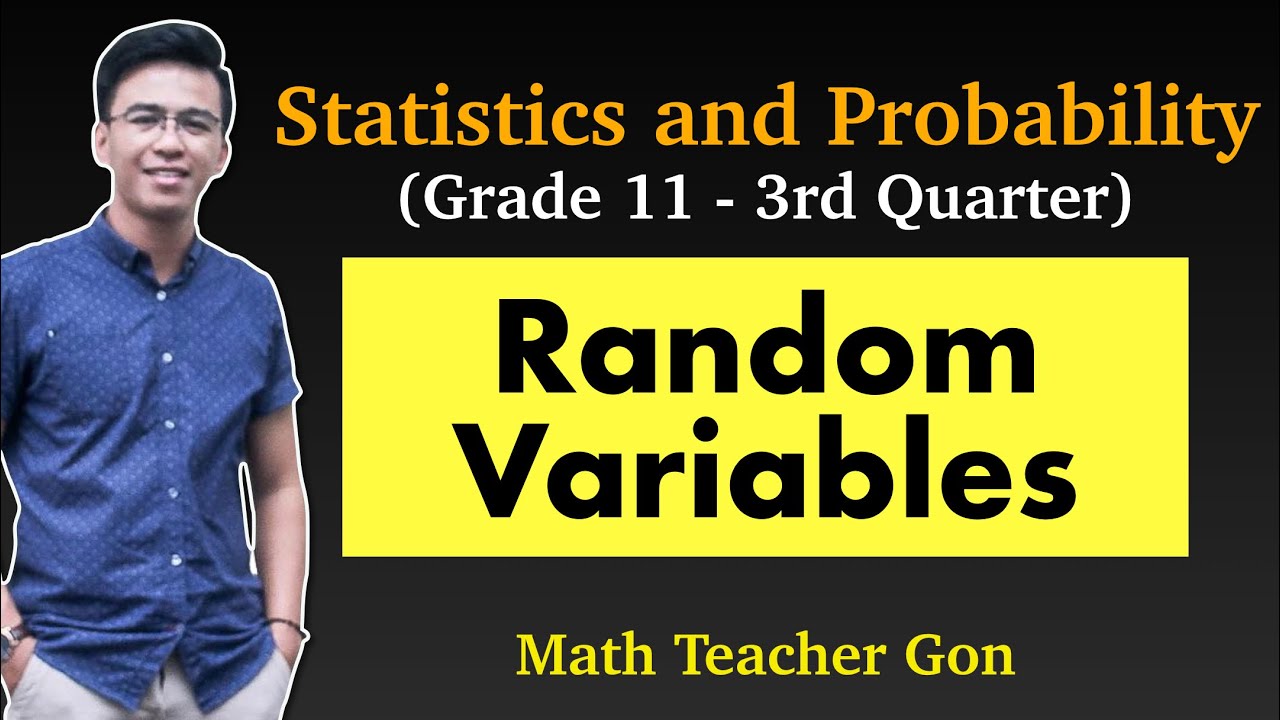
Random Variables - Grade 11 (Statistics and Probability) @MathTeacherGon

Distribusi Normal • Part 1: Distribusi Peluang Variabel Acak Kontinu
5.0 / 5 (0 votes)