Introduction to Random Variables Probability Distribution
Summary
TLDRIn this video, Amal Kumar explains the concept of random variables in probability theory, addressing common misconceptions. He clarifies that random variables are not truly random or variables in the traditional sense, but rather functions that link outcomes from a sample space to real numbers. Using examples like tossing two coins, he demonstrates how to define and calculate random variables. The video further explores the distinction between discrete and continuous random variables, explaining their applications in different contexts. Overall, the video provides a clear and approachable understanding of random variables and their role in probability distribution.
Takeaways
- π Random variables are not truly random; they are functions that map outcomes from a sample space to real numbers.
- π The term 'random variable' is misleading since the variable is not random and functions are defined by the user.
- π A random variable is a function that associates each outcome in the sample space with a real number, making data easier to work with.
- π In the example of tossing two coins, the random variable counts the number of heads in the outcome, demonstrating how to define a random variable.
- π Random variables are defined using capital letters (e.g., X), and the function part assigns real numbers to outcomes based on defined criteria.
- π There are two main types of random variables: discrete and continuous.
- π Discrete random variables have countable outcomes, which could be finite or infinite, like the number of heads in coin tosses.
- π Continuous random variables have uncountable outcomes, represented by real numbers, such as the height of individuals, where any real number within a range is possible.
- π If continuous data is rounded (e.g., to one decimal place), it may be treated as discrete, showing an overlap between discrete and continuous data types.
- π The function of a random variable simplifies the analysis of probability distributions, allowing for further exploration of events in experiments.
- π Random variables help move beyond simple events and allow for deeper analysis and conclusions based on grouped data from experiments.
Q & A
What is the primary confusion about random variables that the speaker addresses?
-The speaker clarifies that random variables are not truly 'random' nor are they traditional 'variables.' Instead, they are functions that map outcomes to real numbers.
What is the key idea behind defining a random variable?
-A random variable is defined as a function that associates each outcome in the sample space with a real number, which makes it easier to analyze probability distributions.
Why are random variables described as 'functions' instead of being random or variables?
-Random variables are functions because they systematically map outcomes from an experiment (sample space) to real numbers, which provides structure and clarity for probability analysis.
What is a sample space, and how does it relate to random variables?
-A sample space is the set of all possible outcomes of an experiment. Random variables associate each of these outcomes with a real number.
Can a random variable be both discrete and continuous?
-Yes, a random variable can be either discrete (with countable outcomes, like coin tosses) or continuous (with uncountable outcomes, like people's heights).
What is the difference between discrete and continuous random variables?
-Discrete random variables have countable outcomes, such as the number of heads in a coin toss, while continuous random variables have uncountable outcomes, like height, where any real number within a range is possible.
How does the speaker illustrate the concept of a random variable with an example?
-The speaker uses the example of tossing two fair coins. The random variable in this case is defined as the number of heads that result from the toss, which is a discrete random variable.
What happens when you round a continuous random variable to a certain number of decimal places?
-Rounding a continuous random variable, such as height, to a specific decimal place makes it discrete, as the range of possible outcomes is now limited to a set of countable values.
What does the term 'real number' signify in the context of random variables?
-In the context of random variables, a 'real number' refers to the value that the random variable assigns to each outcome in the sample space, making it easier to work with in probability and statistical analysis.
Why is the mapping of outcomes to real numbers useful in probability theory?
-Mapping outcomes to real numbers simplifies calculations and analysis in probability theory, as it allows the use of mathematical operations and models to work with events and their associated probabilities.
Outlines
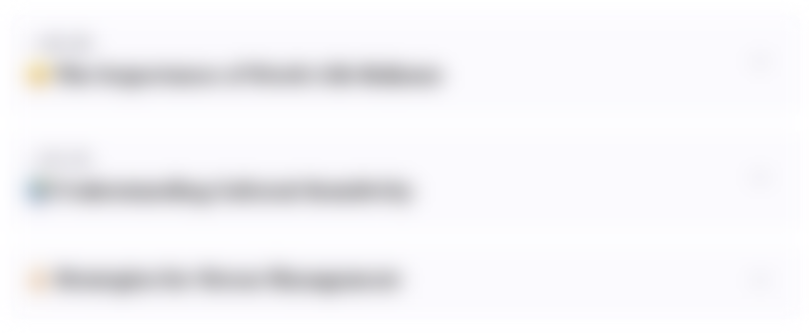
This section is available to paid users only. Please upgrade to access this part.
Upgrade NowMindmap
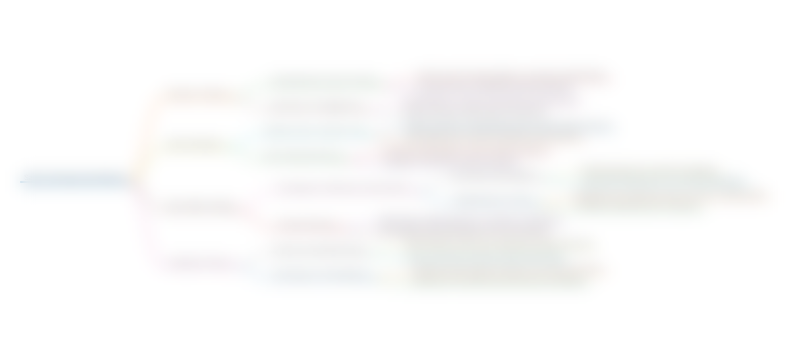
This section is available to paid users only. Please upgrade to access this part.
Upgrade NowKeywords
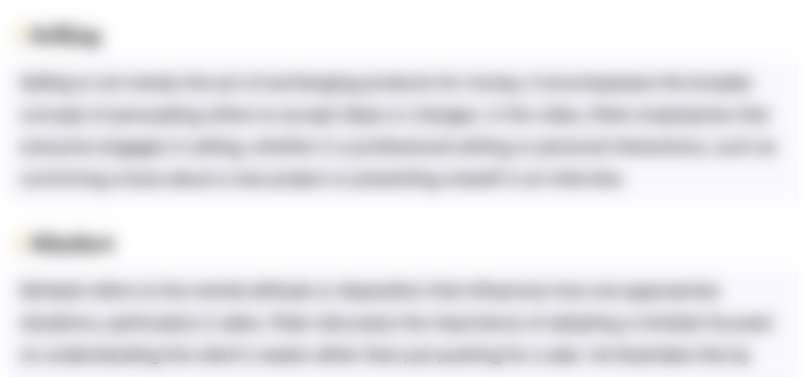
This section is available to paid users only. Please upgrade to access this part.
Upgrade NowHighlights
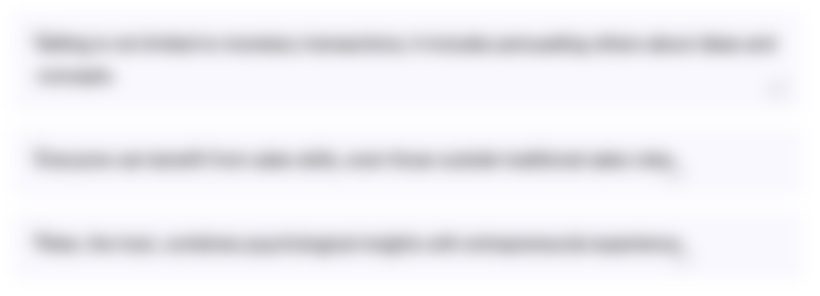
This section is available to paid users only. Please upgrade to access this part.
Upgrade NowTranscripts
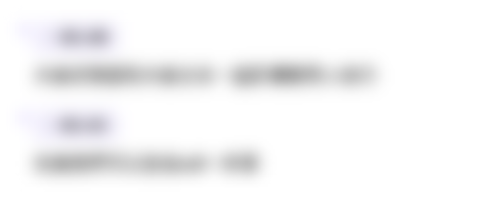
This section is available to paid users only. Please upgrade to access this part.
Upgrade NowBrowse More Related Video
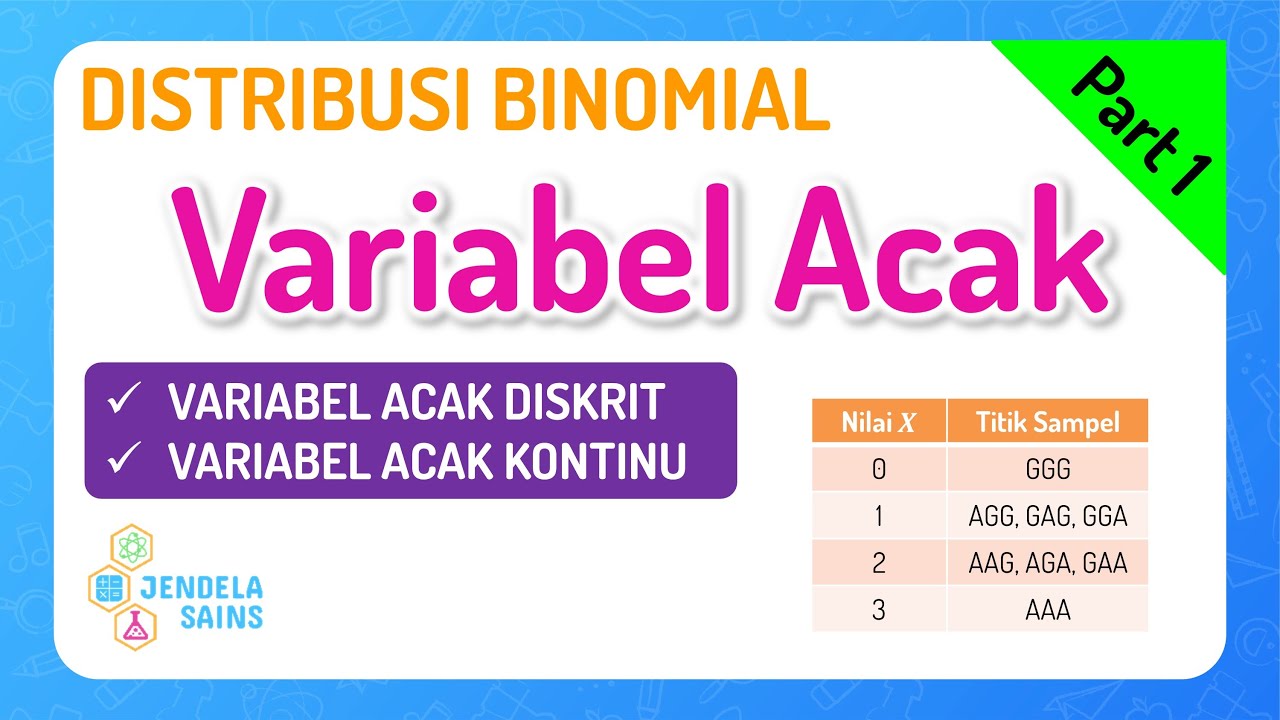
Distribusi Binomial β’ Part 1: Variabel Acak

EXPLORING RANDOM VARIABLES | DISCRETE AND CONTINUOUS | PROF D
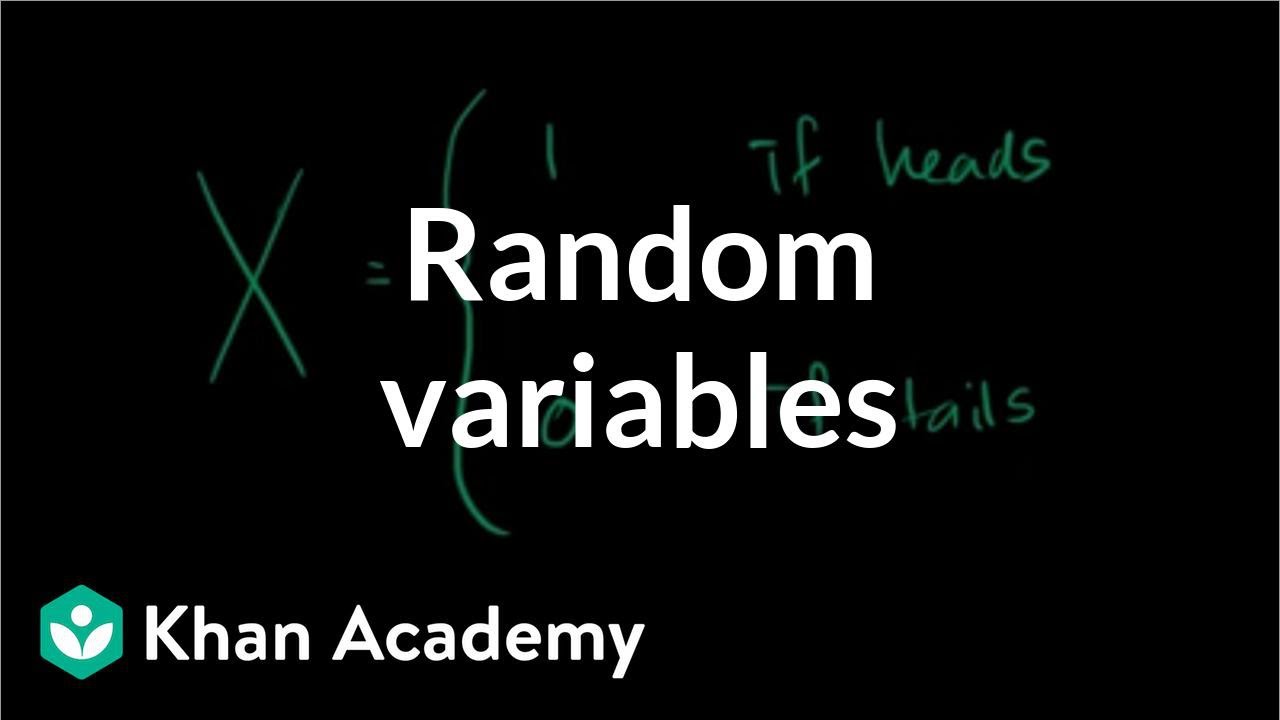
Random variables | Probability and Statistics | Khan Academy
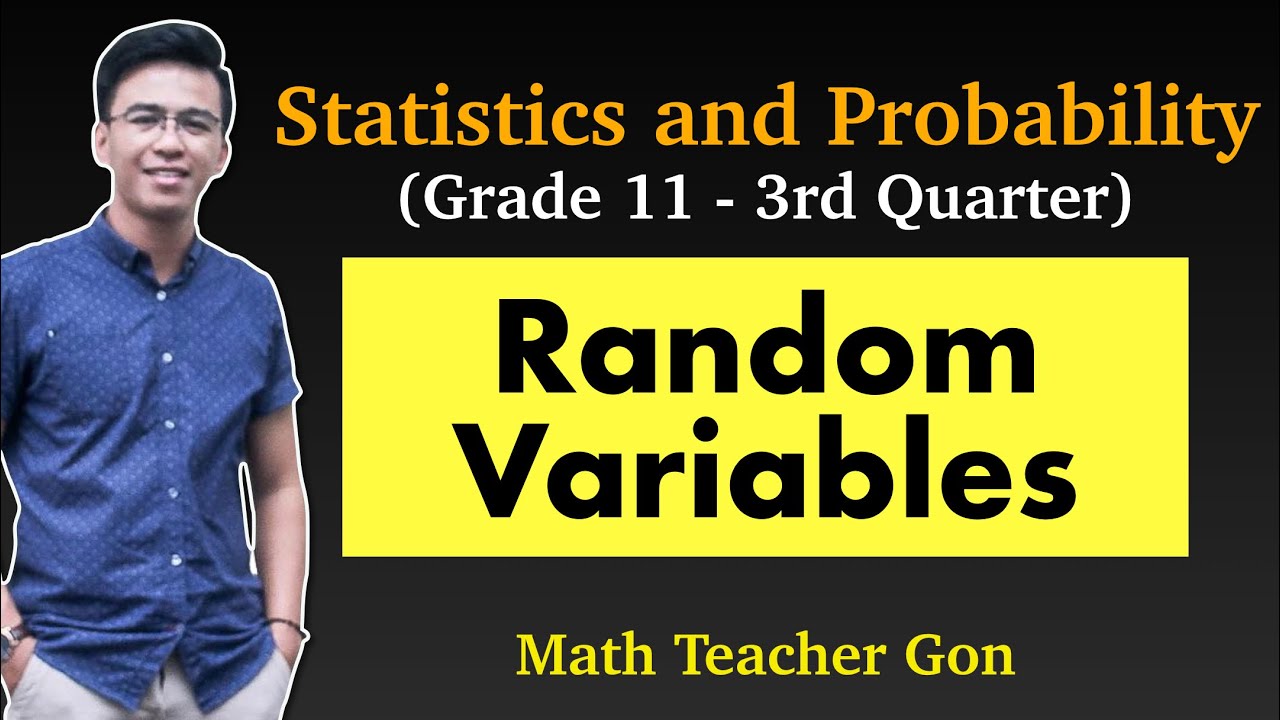
Random Variables - Grade 11 (Statistics and Probability) @MathTeacherGon
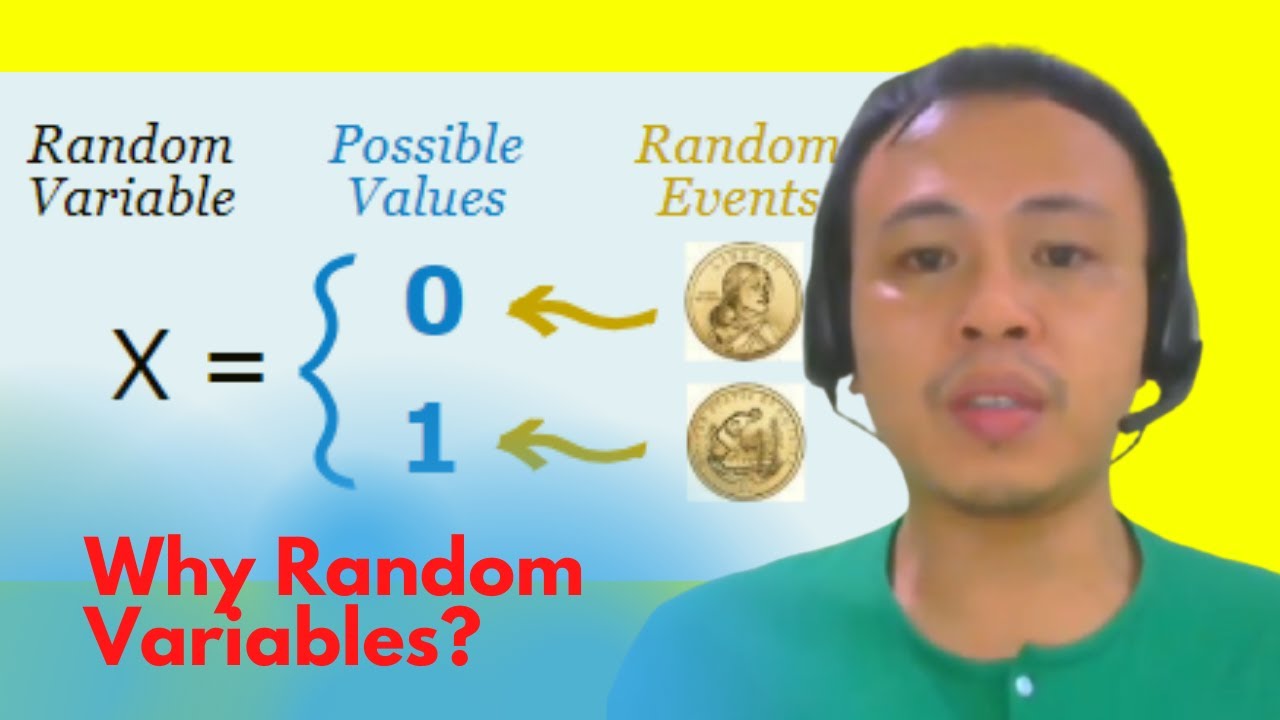
LESSON 1: MACHINE LEARNING ALGORITHM ESSENTIALS: Random Variables and Measurable Function

Konsep Variabel Acak Diskrit dan Kontinu
5.0 / 5 (0 votes)