The Normal Distribution: Crash Course Statistics #19
Summary
TLDRThis Crash Course episode explores the significance of the normal distribution in statistics, explaining its widespread use in analyzing data from various fields such as height, IQ, and even cereal box weights. The video emphasizes the Central Limit Theorem, which states that the distribution of sample means tends to become normal regardless of the original population's shape as sample size increases. Through simulations and examples, it illustrates how this principle simplifies statistical analysis by making sample means easier to compare. The episode also highlights the importance of understanding standard errors and their role in determining the likelihood of statistical differences.
Takeaways
- 😀 The normal distribution is symmetric, with the mean, median, and mode all at the center, and it's commonly found in natural phenomena like height, IQ, and test scores.
- 😀 Sampling distributions of means follow a normal distribution, even if the original population distribution is not normal, especially when the sample size is large.
- 😀 The Central Limit Theorem (CLT) states that as sample sizes grow, the distribution of sample means approaches normality, regardless of the population distribution.
- 😀 The mean of the sampling distribution of sample means is always equal to the population mean, even when the population itself isn't normally distributed.
- 😀 The standard deviation of the sampling distribution of sample means, called the standard error, decreases as the sample size increases, making sample means more concentrated around the population mean.
- 😀 Larger sample sizes reduce the likelihood of extreme values in the distribution of sample means, making the distribution more tightly grouped around the population mean.
- 😀 For example, when rolling dice, the distribution of sample means moves toward normal as the number of dice rolls increases, even though the original distribution is uniform.
- 😀 The Central Limit Theorem can be applied to various statistical parameters like proportions, regression coefficients, and standard deviations, not just means.
- 😀 Standard error measures how much sample means typically differ from the population mean, and it helps to assess the significance of differences between sample means.
- 😀 By understanding the distribution of sample means, we can assess whether observed differences, like in the example of strawberry box weights, are likely due to random variation or if there's a systematic cause behind them.
Q & A
What is the normal distribution and why is it important in statistics?
-The normal distribution is a symmetric, bell-shaped distribution where the mean, median, and mode are the same. It is important because many natural phenomena, like height, IQ, and test scores, follow this distribution. Additionally, the Central Limit Theorem explains that even if a population isn’t normally distributed, the distribution of sample means will approach normality as sample size increases.
What is the Central Limit Theorem?
-The Central Limit Theorem states that the distribution of sample means will approach a normal distribution as the sample size increases, even if the original population distribution is not normal. This is crucial in inferential statistics, as it allows us to make predictions and comparisons based on sample data.
Why do we focus on the distribution of sample means rather than individual scores?
-In statistics, we are often concerned with comparing groups or samples rather than individual data points. The distribution of sample means allows us to make more accurate and generalizable comparisons between groups by providing a more reliable estimate of the population mean.
What happens to the distribution of sample means as the sample size increases?
-As the sample size increases, the distribution of sample means becomes more concentrated around the population mean, and the distribution itself becomes narrower. This happens because larger sample sizes make extreme values less likely and provide a better approximation of the true population mean.
What is the significance of the shape of the distribution of sample means?
-The shape of the distribution of sample means is typically normal, even if the original population is not. This is significant because it simplifies statistical analysis, making it easier to calculate probabilities, percentiles, and differences between means.
How does the standard deviation of the distribution of sample means differ from the population standard deviation?
-The standard deviation of the distribution of sample means, known as the standard error, is smaller than the population standard deviation. This is because larger sample sizes lead to less variability in sample means, and the standard error decreases as the sample size increases.
What is the standard error and how is it calculated?
-The standard error is the standard deviation of the sampling distribution of sample means. It is calculated by dividing the population standard deviation by the square root of the sample size (n). This adjustment accounts for the reduced variability in sample means as the sample size increases.
Why are sample means less likely to be extreme compared to individual values?
-Sample means are less likely to be extreme because the means of random samples are averages, and extreme values are diluted when combined with other values. As a result, the distribution of sample means tends to be more centered around the true population mean.
How does the Central Limit Theorem apply to various types of distributions?
-The Central Limit Theorem applies to all types of distributions, including uniform, normal, and skewed distributions. Regardless of the original distribution, as long as the sample size is large enough, the distribution of sample means will approximate a normal distribution.
How can the Central Limit Theorem help in real-world situations like quality control?
-In real-world situations like quality control, the Central Limit Theorem helps by allowing us to make decisions based on sample data rather than measuring entire populations. For instance, by understanding the distribution of sample means, companies can assess whether their products meet quality standards and detect potential issues with production.
Outlines
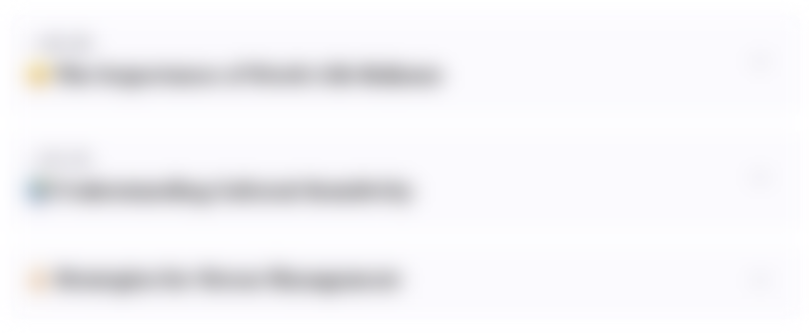
This section is available to paid users only. Please upgrade to access this part.
Upgrade NowMindmap
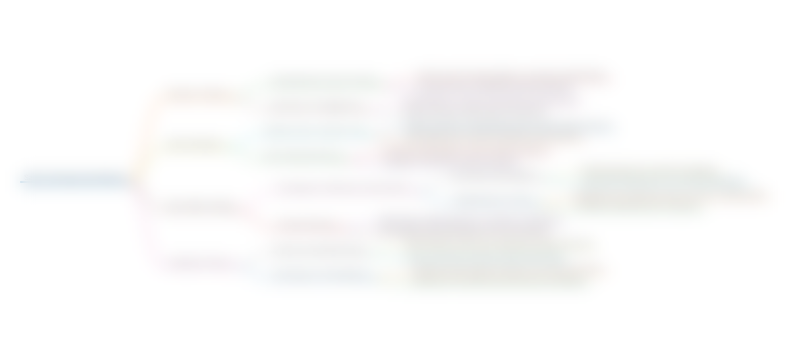
This section is available to paid users only. Please upgrade to access this part.
Upgrade NowKeywords
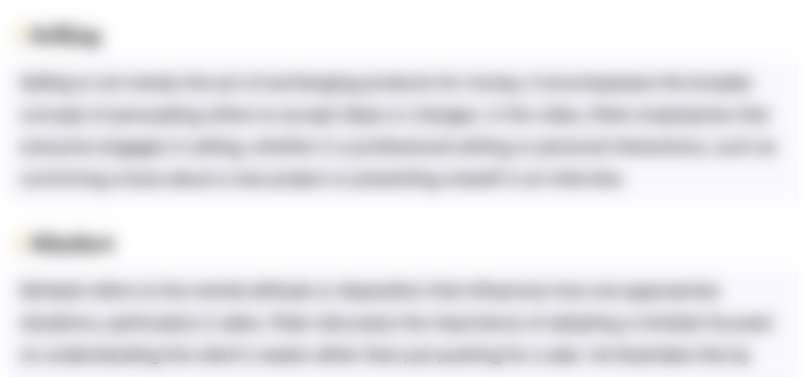
This section is available to paid users only. Please upgrade to access this part.
Upgrade NowHighlights
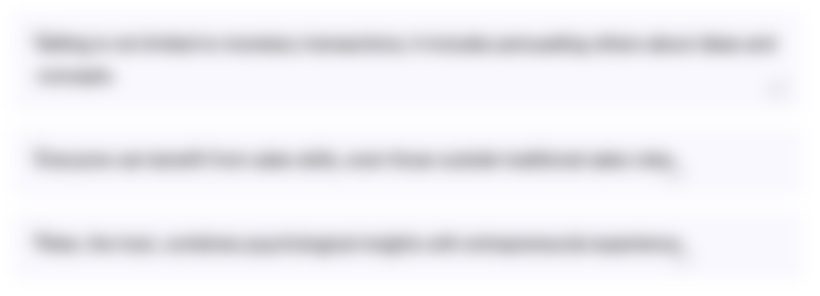
This section is available to paid users only. Please upgrade to access this part.
Upgrade NowTranscripts
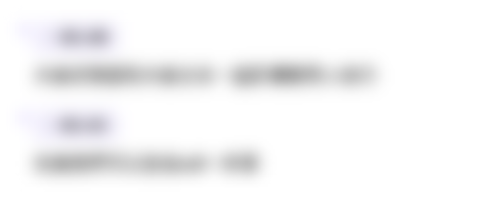
This section is available to paid users only. Please upgrade to access this part.
Upgrade NowBrowse More Related Video
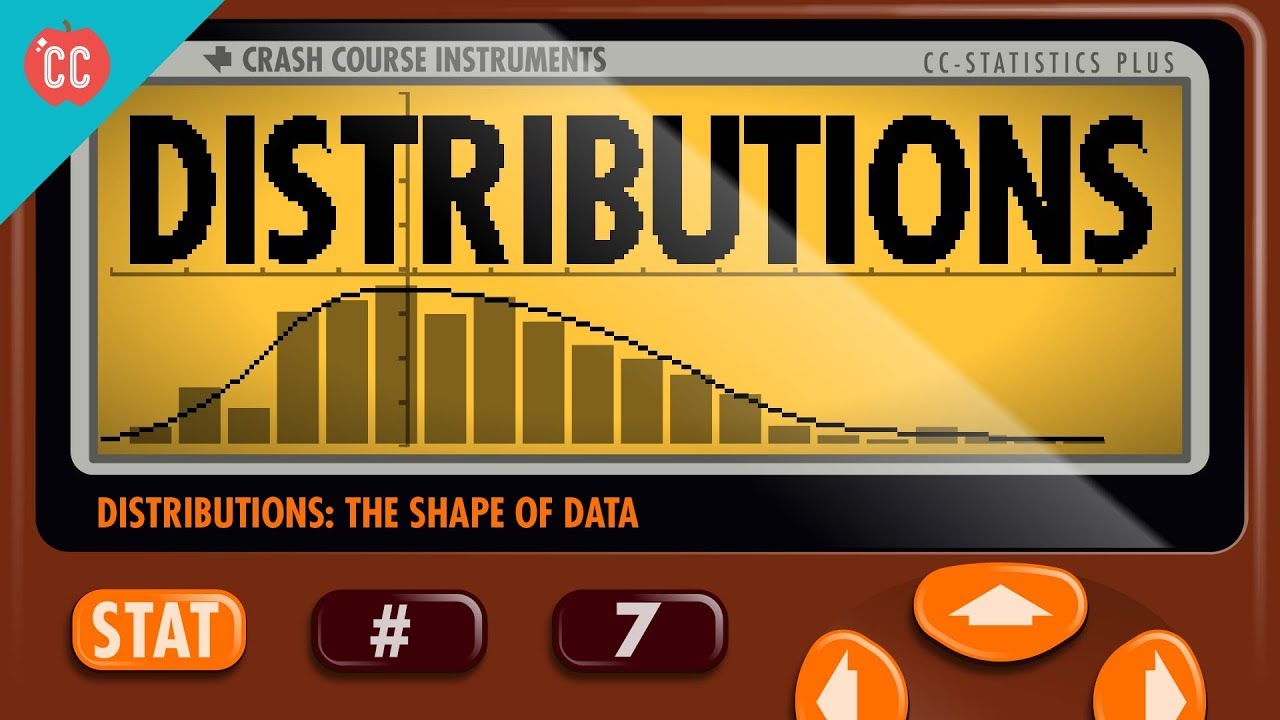
The Shape of Data: Distributions: Crash Course Statistics #7

The Normal Distribution and Shoplifting (DeSTRESS Film 12)

Normal Curve - Bell Curve - Standard Deviation - What Does It All Mean? Statistics Help
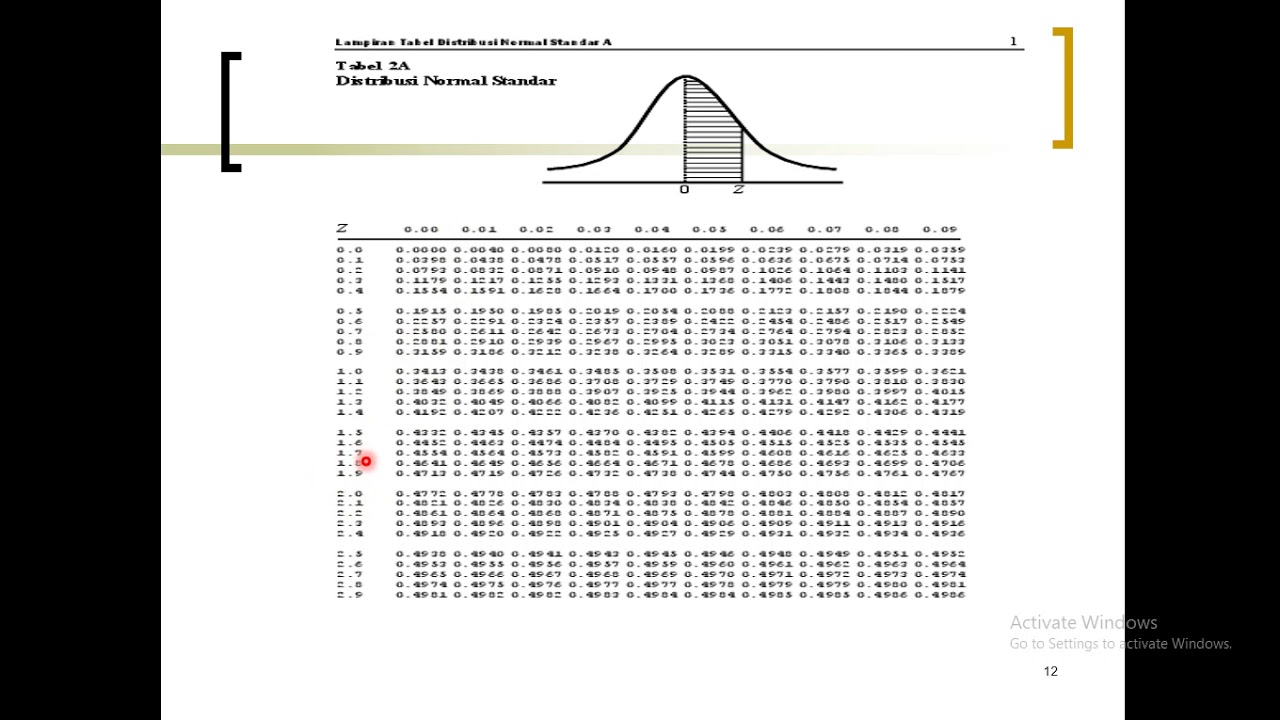
Distribusi Probabilitas Normal
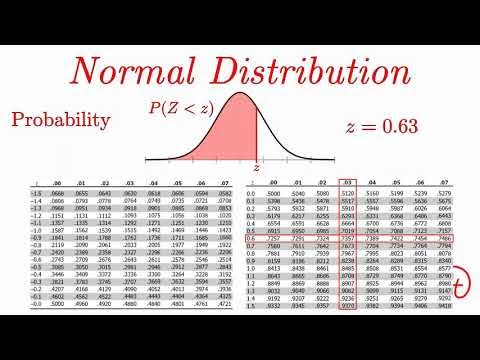
Normal Distribution EXPLAINED with Examples
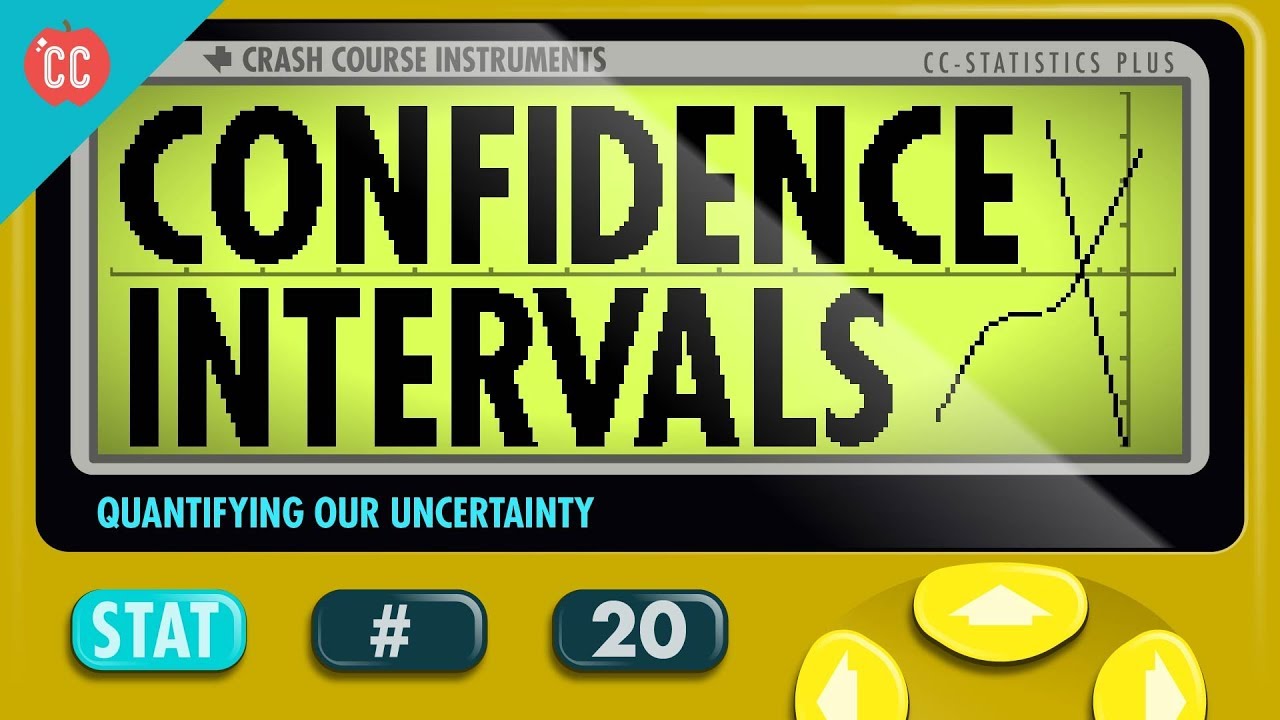
Confidence Intervals: Crash Course Statistics #20
5.0 / 5 (0 votes)