The Shape of Data: Distributions: Crash Course Statistics #7
Summary
TLDRIn this engaging Crash Course Statistics episode, Adriene Hill explores the significance of data sampling and distribution shapes. She explains how samples provide insights into larger datasets, using analogies like a leaf-counting machine to illustrate how distributions operate. The video covers various distribution types, including normal, skewed, bimodal, and uniform distributions, highlighting their characteristics and real-world examples. By understanding the shapes of these distributions, viewers learn to make meaningful comparisons and inferences about data, ultimately showcasing how statistics help reveal underlying patterns in various phenomena.
Takeaways
- π Samples of data help us understand the broader context of a larger dataset without needing to survey everyone.
- π A distribution represents all possible values for a set of data and their frequency of occurrence.
- π Normal distributions are symmetric and unimodal, characterized by the mean and standard deviation.
- π About 68% of data in a normal distribution lies within one standard deviation of the mean.
- π Skewed distributions indicate extreme values, impacting the median and standard deviation.
- π©βπ« Teachers can analyze test score distributions to assess the difficulty level of assessments.
- π Bimodal and multimodal distributions suggest the presence of different underlying groups or processes generating the data.
- π² Uniform distributions indicate that each outcome has an equal probability, such as rolling a fair die.
- π Statistics allow us to infer characteristics of a population based on sample data, despite uncertainty.
- π The shape of data distributions provides insights into real-world phenomena and trends.
Q & A
What is the purpose of collecting samples in statistics?
-Samples are collected because they provide a glimpse of the larger population data, allowing us to estimate characteristics and make inferences about the overall data distribution.
How does the shape of a histogram relate to the underlying data distribution?
-The shape of a histogram represents how frequently values occur in the data, and as the bins become smaller, the histogram can approximate a smooth curve that represents the continuous data distribution.
What distinguishes a normal distribution from other types of distributions?
-A normal distribution is symmetric, unimodal, and defined by its mean and standard deviation, where the mean, median, and mode are all equal.
What role does standard deviation play in a normal distribution?
-The standard deviation indicates the spread of the data around the mean; a smaller standard deviation results in a taller and skinnier distribution, while a larger standard deviation leads to a wider distribution.
What does it mean when a distribution is skewed?
-A skewed distribution has a tail that is longer on one side than the other, indicating that most values cluster on one end while a few extreme values stretch out the opposite tail.
What is bimodal data, and why does it occur?
-Bimodal data has two distinct peaks and often arises when two different populations or processes are being measured together, leading to two unimodal distributions appearing as one.
Can you give an example of a uniform distribution?
-A uniform distribution is where every outcome has an equal chance of occurring, such as the roll of a fair die, where each number from 1 to 6 has the same probability of being rolled.
How does one compare the shapes of two different data samples?
-To compare shapes, one examines whether the distributions that generated the samples are similar or if different settings (knob adjustments in the machine analogy) could have produced the two samples.
What is the significance of understanding the shape of data in statistics?
-Understanding the shape of data helps statisticians make informed decisions and inferences about the underlying processes generating the data, which is crucial for accurate analysis.
How do outliers affect the interpretation of a distribution?
-Outliers can skew the distribution and affect measures of central tendency like the mean, making it crucial to consider them when analyzing data to accurately understand the distribution's shape and spread.
Outlines
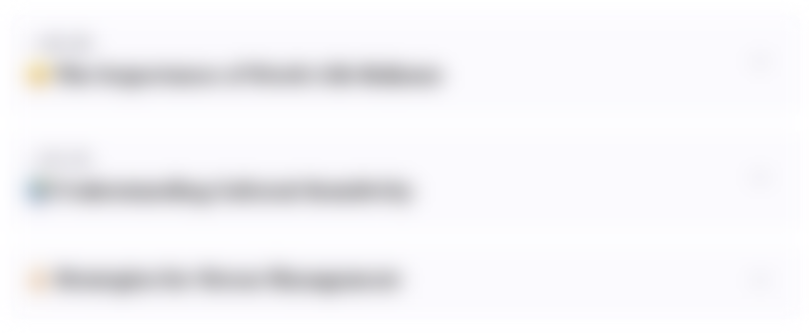
This section is available to paid users only. Please upgrade to access this part.
Upgrade NowMindmap
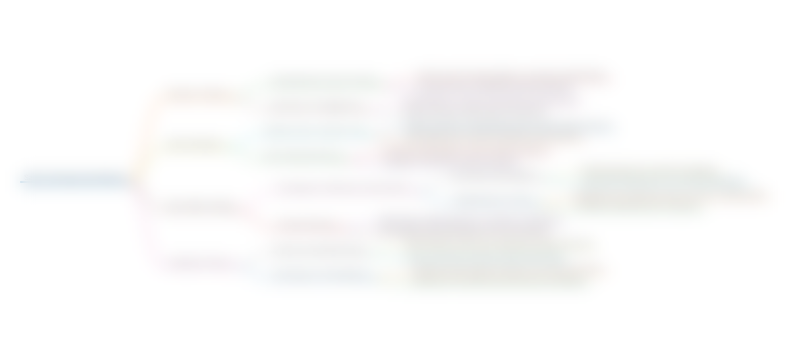
This section is available to paid users only. Please upgrade to access this part.
Upgrade NowKeywords
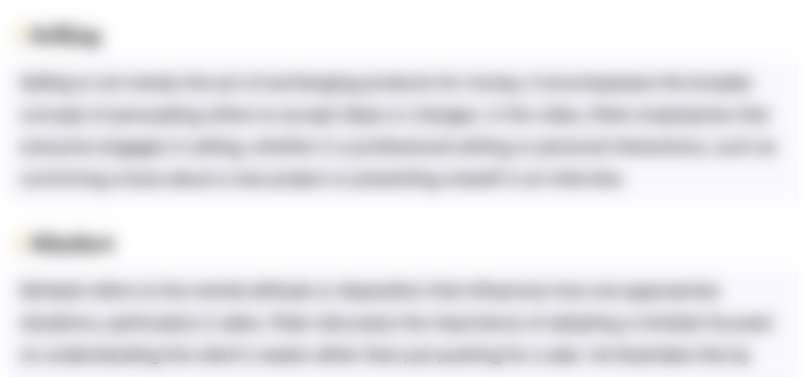
This section is available to paid users only. Please upgrade to access this part.
Upgrade NowHighlights
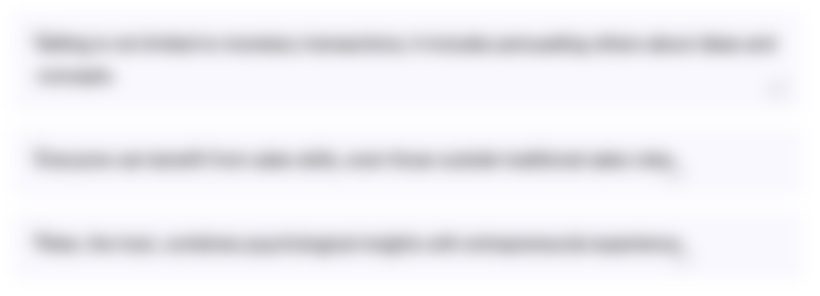
This section is available to paid users only. Please upgrade to access this part.
Upgrade NowTranscripts
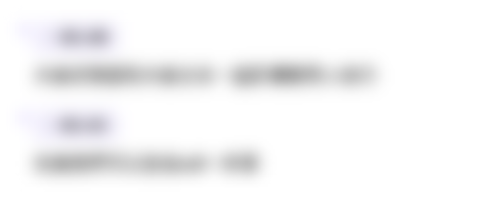
This section is available to paid users only. Please upgrade to access this part.
Upgrade NowBrowse More Related Video
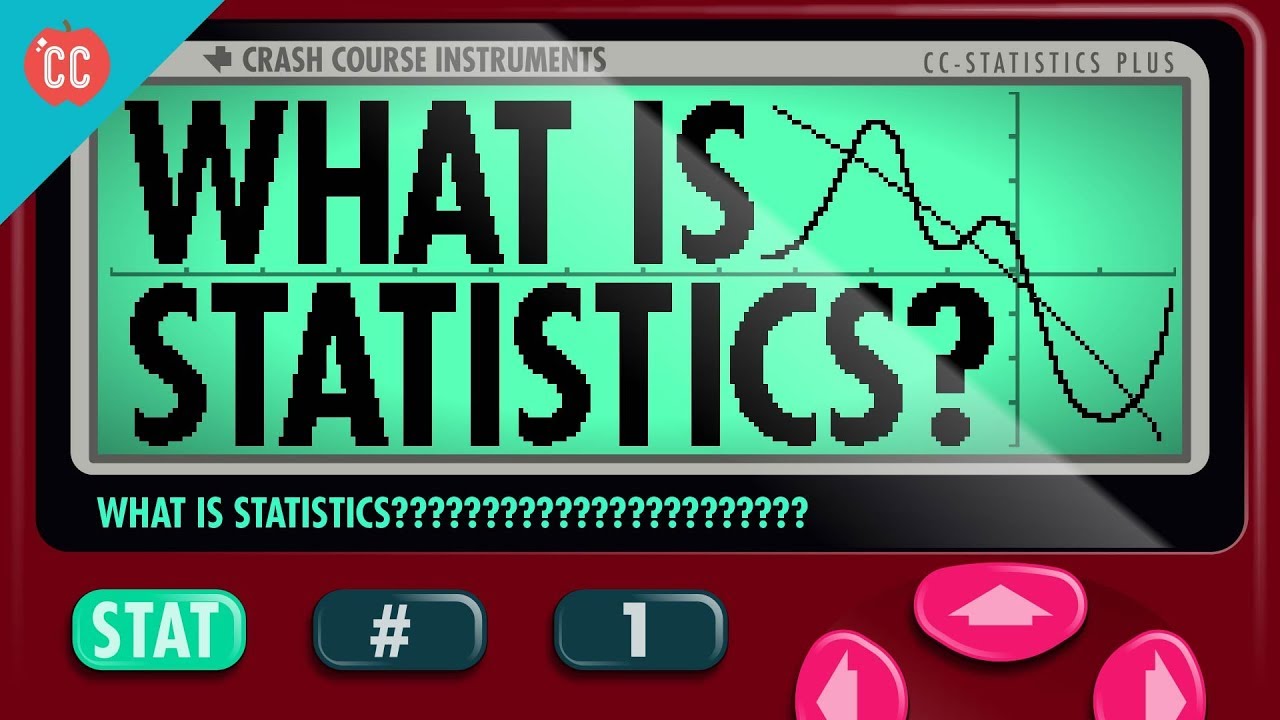
What Is Statistics: Crash Course Statistics #1
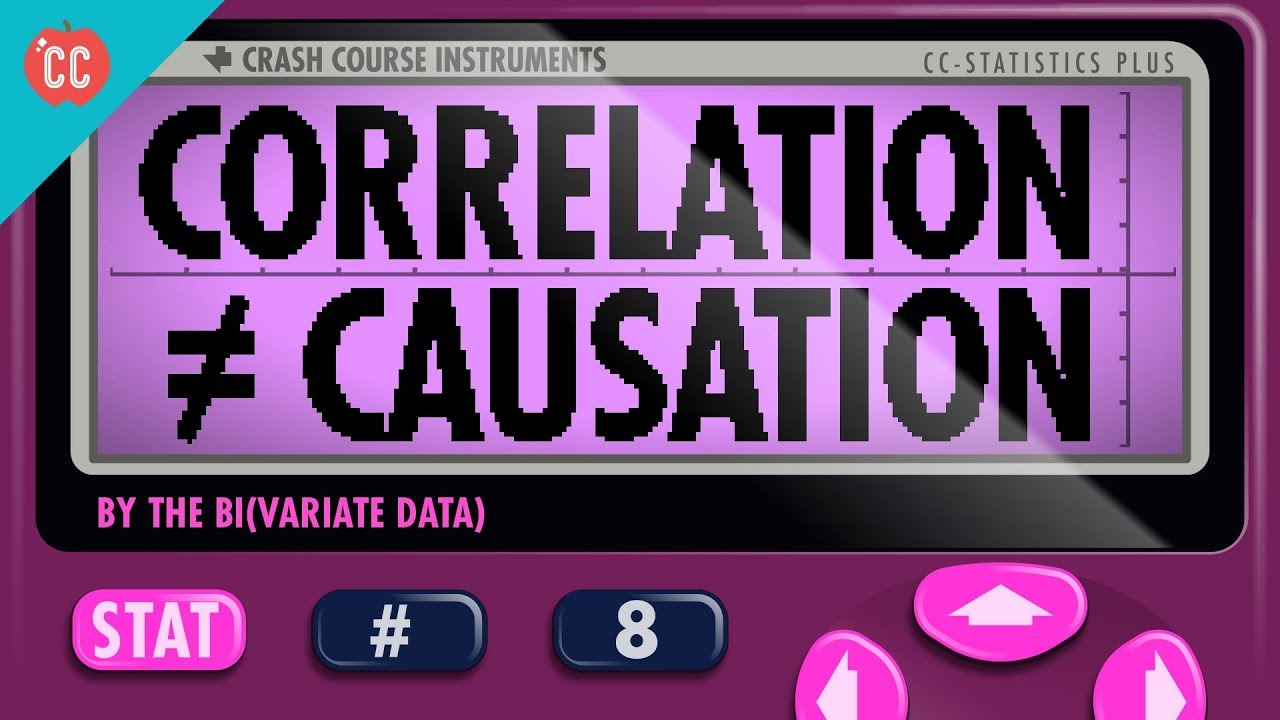
Correlation Doesn't Equal Causation: Crash Course Statistics #8
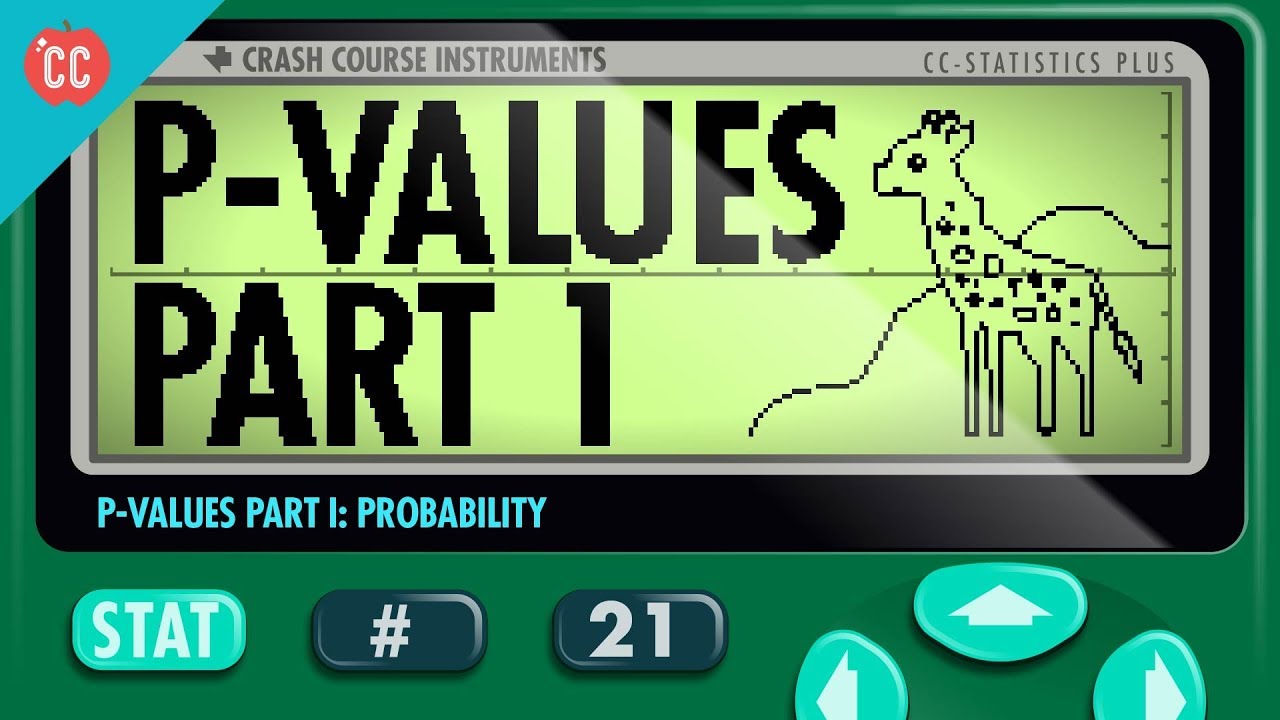
How P-Values Help Us Test Hypotheses: Crash Course Statistics #21
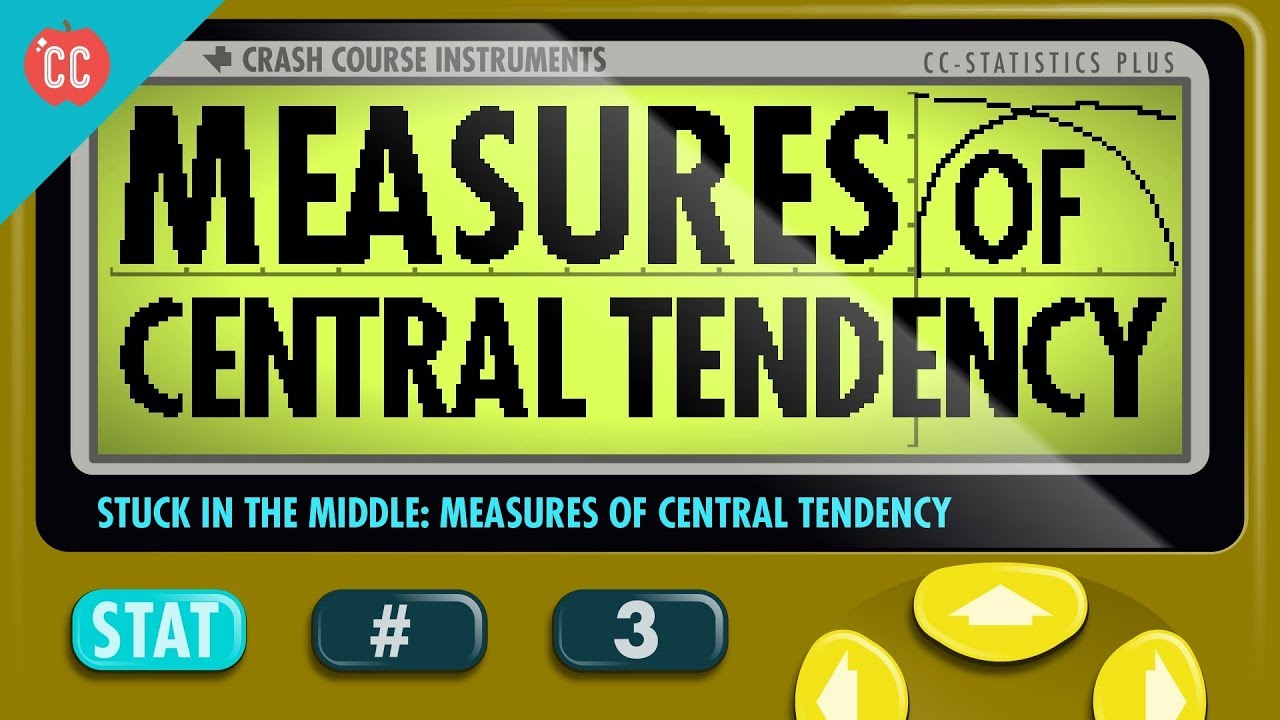
Mean, Median, and Mode: Measures of Central Tendency: Crash Course Statistics #3

ANOVA: Crash Course Statistics #33
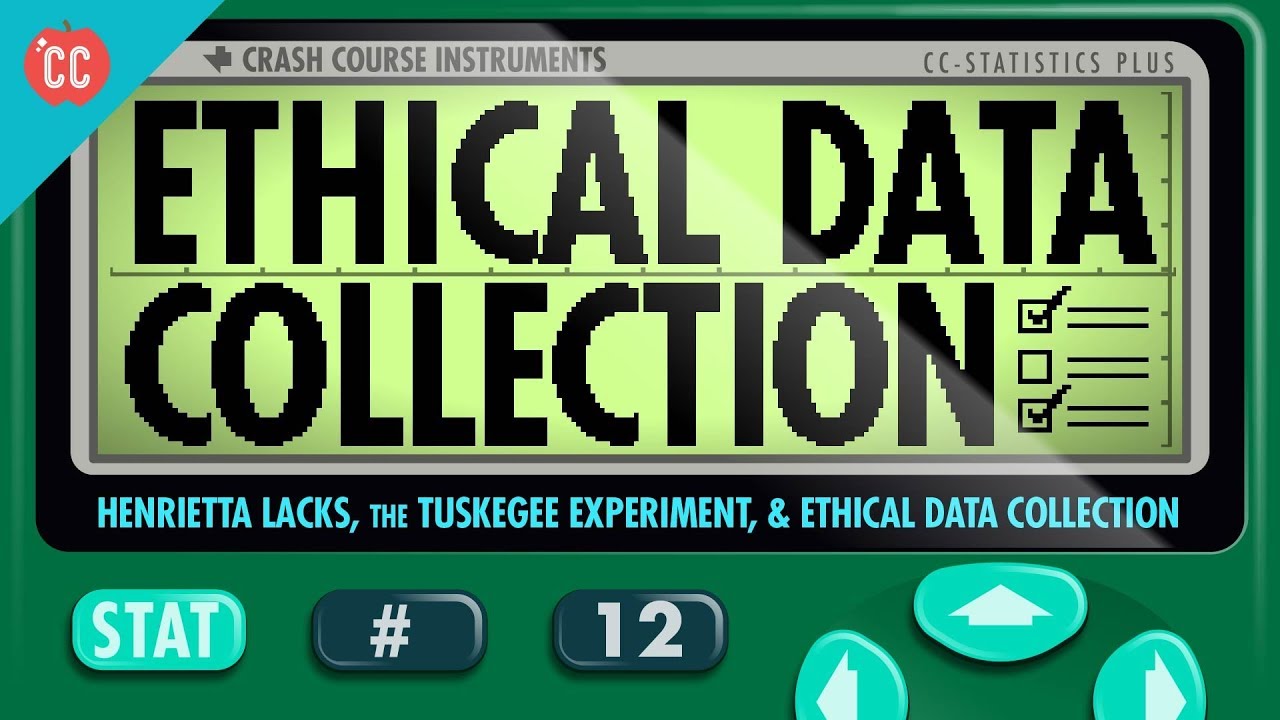
Henrietta Lacks, the Tuskegee Experiment, and Ethical Data Collection: Crash Course Statistics #12
5.0 / 5 (0 votes)