K-Means Clustering Explanation and Visualization
Summary
TLDRThe video explains the k-means clustering algorithm, a key technique in unsupervised machine learning. It demonstrates how data points are grouped into clusters based on their similarity, using centroids to represent the center of each cluster. The process starts with randomly placing centroids and assigning data points to the nearest centroid. Iterations follow as centroids shift to the center of their assigned points, and data points are reassigned. The algorithm terminates when centroids stabilize, and data points no longer change clusters, ensuring that similar points are grouped together.
Takeaways
- π The goal of k-means clustering is to group similar data points into predefined clusters.
- π K-means clustering uses centroids, which represent the center of each cluster.
- π The number of centroids corresponds to the number of clusters you wish to create.
- π Each data point is assigned to the closest centroid based on its distance from the centroid.
- π Centroids are initially placed randomly but can be set manually in some cases.
- π After data points are assigned to centroids, the centroids are recalculated as the center of the assigned data points.
- π The algorithm continues iterating, reassigning data points to the nearest centroid after each recalculation.
- π Iterations continue until the centroids no longer shift, and data points stop changing clusters.
- π The process of assigning data points to centroids and recalculating centroids happens in cycles.
- π K-means clustering aims to minimize the distance between data points within a cluster, ensuring that similar points are grouped together.
- π The algorithm is complete when data points no longer change clusters and centroids stabilize.
Q & A
What is the goal of the K-means clustering algorithm?
-The goal of the K-means clustering algorithm is to group similar data points together into a predefined number of clusters based on their distance from each other.
What are centroids in the context of K-means clustering?
-Centroids are the centers of clusters, representing the average position of all the data points in a particular cluster. The number of centroids corresponds to the number of clusters to be formed.
How are centroids initialized in the K-means algorithm?
-Centroids are typically initialized randomly, but for the illustration in the script, the centroids are manually placed in the visualization to start the clustering process.
What happens in the first phase of the K-means algorithm?
-In the first phase, each data point is assigned to the nearest centroid. The data points change color to represent the cluster they belong to, based on the closest centroid.
How are centroids updated in each iteration?
-After the data points are reassigned to centroids, the position of each centroid is recalculated as the center of the new cluster, and the centroids shift to the new calculated centers.
What is the key criteria for assigning a data point to a cluster?
-A data point is assigned to the cluster whose centroid is closest to the data point, typically using Euclidean distance to determine proximity.
What happens after the centroids are updated in the algorithm?
-After the centroids are updated, the data points are reassigned to the new centroids. The process of recalculating centroids and reassigning points repeats until the clusters stabilize.
How can you tell when K-means clustering has finished?
-Clustering is complete when the data points stop changing clusters and the centroids no longer shift in subsequent iterations.
Why does K-means clustering require the number of clusters to be predefined?
-K-means requires the number of clusters to be predefined because the algorithm needs to know how many centroids to initialize and how many groups to form. This number is a parameter set before running the algorithm.
What does the shifting of centroids signify in the K-means process?
-The shifting of centroids signifies that the algorithm is adjusting to better represent the center of the data points in the cluster. This iterative process refines the cluster boundaries until they stabilize.
Outlines
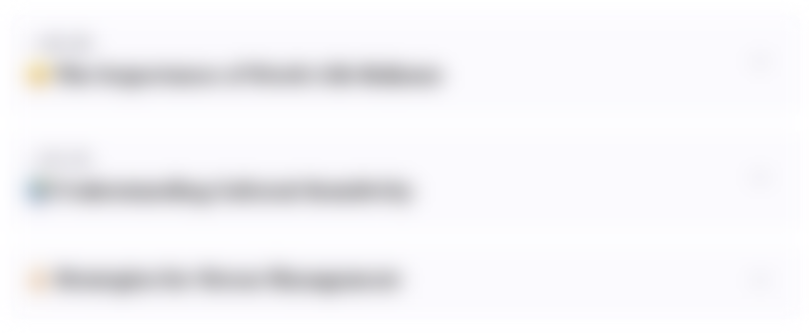
This section is available to paid users only. Please upgrade to access this part.
Upgrade NowMindmap
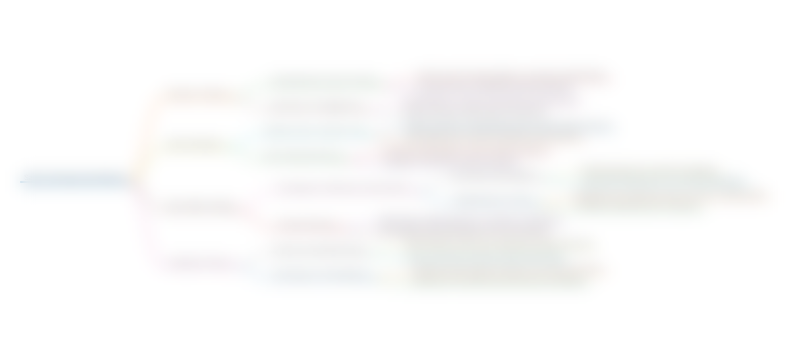
This section is available to paid users only. Please upgrade to access this part.
Upgrade NowKeywords
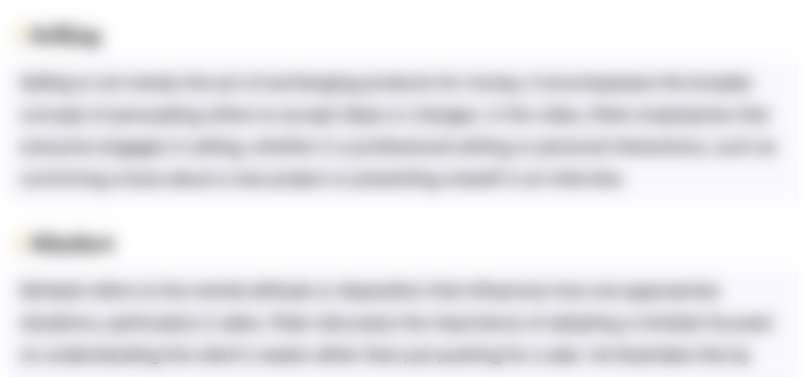
This section is available to paid users only. Please upgrade to access this part.
Upgrade NowHighlights
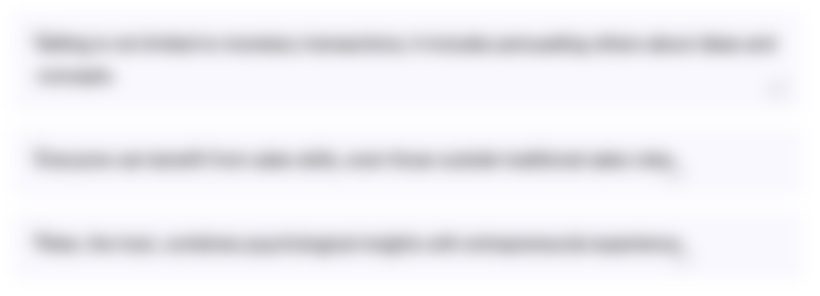
This section is available to paid users only. Please upgrade to access this part.
Upgrade NowTranscripts
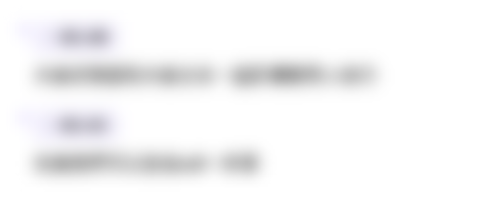
This section is available to paid users only. Please upgrade to access this part.
Upgrade Now5.0 / 5 (0 votes)