TUTORIAL MUDAH UJI REGRESI BERGANDA DENGAN SPSS
Summary
TLDRIn this tutorial, the presenter demonstrates how to conduct linear regression testing using SPSS, focusing on the analysis of multiple variables and their impact on performance. The video explains the steps for running regression tests, interpreting key statistics such as correlation and F-values, and understanding model predictions. The tutorial emphasizes the importance of meeting prerequisites like normality and linearity for valid results. It also provides practical insights for students working on research projects or theses, particularly those analyzing the effects of work involvement and job satisfaction on performance.
Takeaways
- 😀 Linear regression analysis is used to evaluate the relationship between two or more variables and their effect on a dependent variable.
- 😀 SPSS is used to perform linear regression analysis, with the tutorial focusing on version 23 of the software.
- 😀 The tutorial uses a sample dataset with variables X1 (employee engagement) and X2 (job satisfaction) to predict performance (Y).
- 😀 Key assumptions for linear regression include normality of data, linearity, and appropriate variable relationships.
- 😀 The significance of regression results is determined by the p-value, with values less than 0.05 indicating a significant relationship.
- 😀 In the regression output, an F-value greater than the critical F-table value suggests that the model is valid for predicting the dependent variable.
- 😀 The correlation coefficient (R) measures the strength of the relationship between variables, with values closer to 1 indicating a stronger relationship.
- 😀 The R² value represents the percentage of variation in the dependent variable explained by the independent variables.
- 😀 Coefficients (β values) show the impact of each independent variable on the dependent variable, with higher values indicating a stronger influence.
- 😀 The tutorial explains how to interpret coefficients for each variable, demonstrating their effect on performance outcomes based on engagement and satisfaction.
- 😀 The regression model is validated by checking for multicollinearity, and if assumptions are met, the model can be used for prediction.
- 😀 The video encourages students working on theses or projects to ask questions about regression analysis in the comments section and provides tips on troubleshooting common issues in SPSS.
Q & A
What is the purpose of the tutorial in the script?
-The purpose of the tutorial is to explain how to perform linear regression testing using SPSS, specifically focusing on the impact of job involvement and job satisfaction on performance.
What are the key variables discussed in the regression analysis?
-The key variables discussed in the regression analysis are 'kinerja' (performance), 'keterlibatan kerja' (job involvement), and 'kepuasan kerja' (job satisfaction).
What software is used for conducting the linear regression analysis in the tutorial?
-SPSS (version 23) is used for conducting the linear regression analysis in the tutorial.
What are the prerequisites for performing linear regression analysis, as mentioned in the script?
-The prerequisites for performing linear regression analysis are that the variables should be normally distributed and have a linear relationship.
What is the significance of the correlation value of 0.76 mentioned in the script?
-The correlation value of 0.76 indicates a strong positive relationship between the independent variables (job involvement and job satisfaction) and the dependent variable (performance).
What is the interpretation of the F-value of 20.2690 mentioned in the script?
-The F-value of 20.2690, with a significance level of 0.0001 (less than 0.05), suggests that the regression model is statistically significant and can be used to predict performance based on the independent variables.
What does the constant value of 11,000 represent in the regression analysis?
-The constant value of 11,000 represents the baseline or intercept value in the regression equation, indicating the predicted performance when both job involvement and job satisfaction are zero.
How does a 1% increase in job involvement (X1) affect performance, based on the regression model?
-A 1% increase in job involvement (X1) leads to a 0.300 increase in performance, as indicated by the coefficient for X1 in the regression equation.
What does the coefficient for job satisfaction (X2) suggest about its impact on performance?
-The coefficient for job satisfaction (X2), which is 0.410949, suggests that a 1% increase in job satisfaction leads to a 0.410949 increase in performance.
What decision-making criteria are used in the regression analysis based on the script?
-The decision-making criteria include ensuring that the p-value is below 0.05 and that the F-value is larger than the F-table value, indicating that the regression model is statistically significant.
Outlines
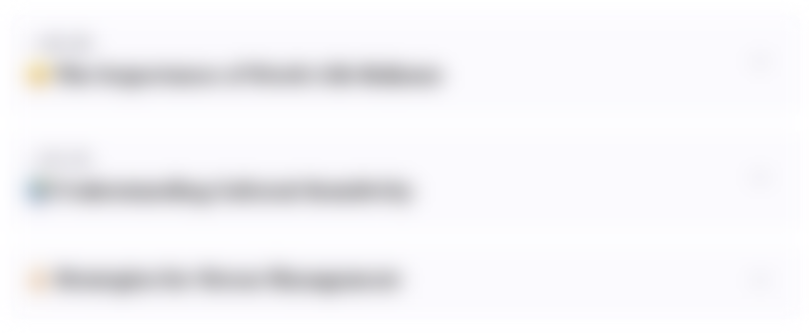
This section is available to paid users only. Please upgrade to access this part.
Upgrade NowMindmap
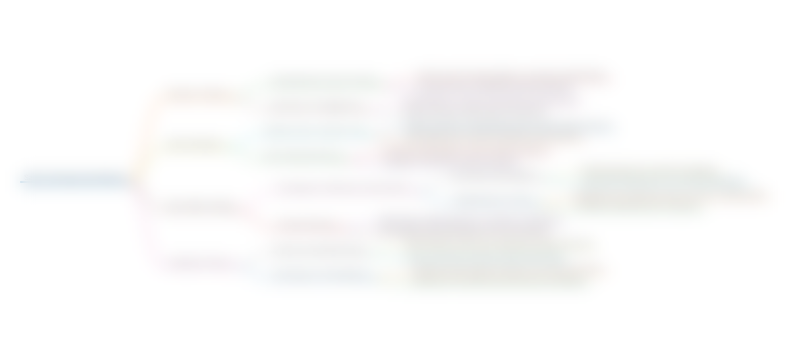
This section is available to paid users only. Please upgrade to access this part.
Upgrade NowKeywords
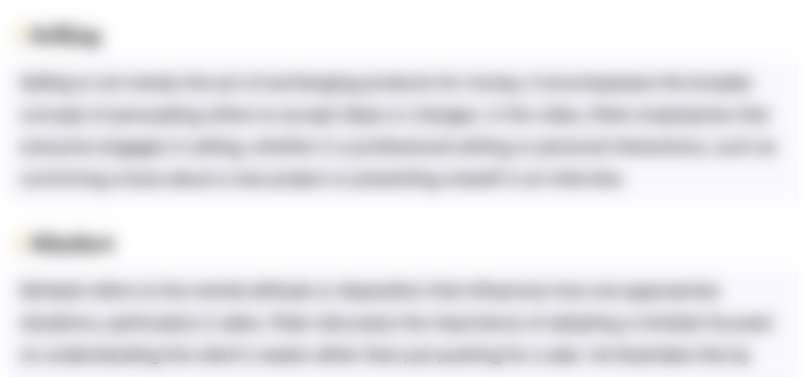
This section is available to paid users only. Please upgrade to access this part.
Upgrade NowHighlights
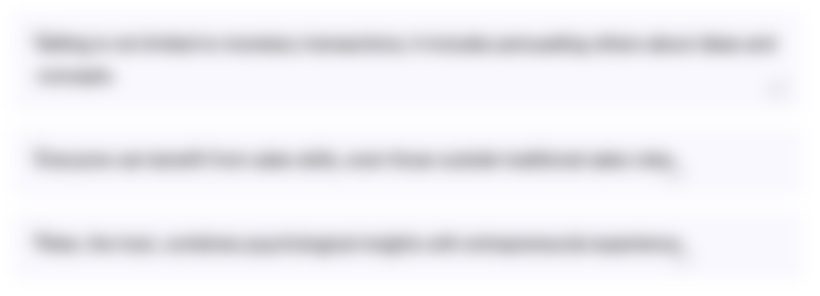
This section is available to paid users only. Please upgrade to access this part.
Upgrade NowTranscripts
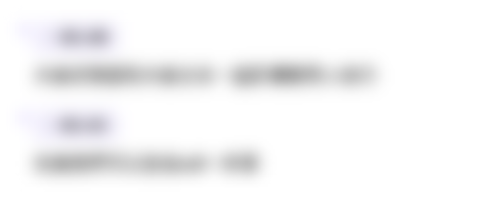
This section is available to paid users only. Please upgrade to access this part.
Upgrade NowBrowse More Related Video
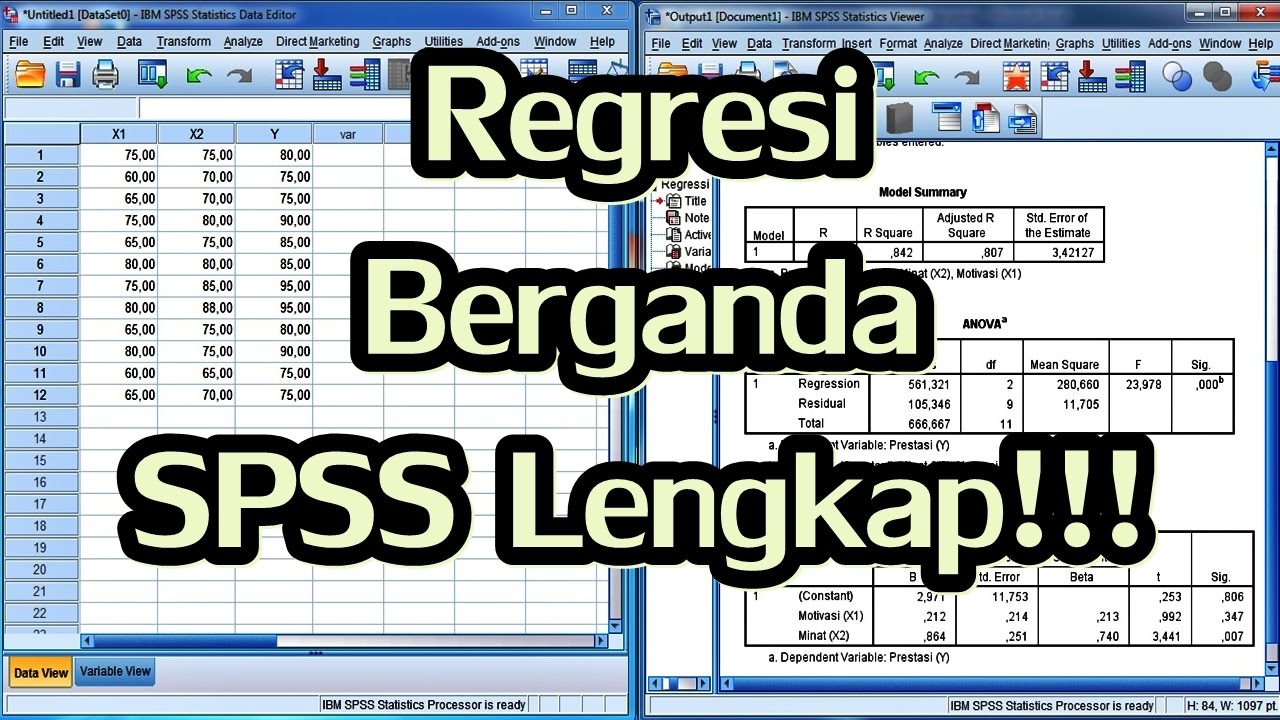
Uji t dan Uji F dalam Analisis Regresi Berganda dengan SPSS Lengkap
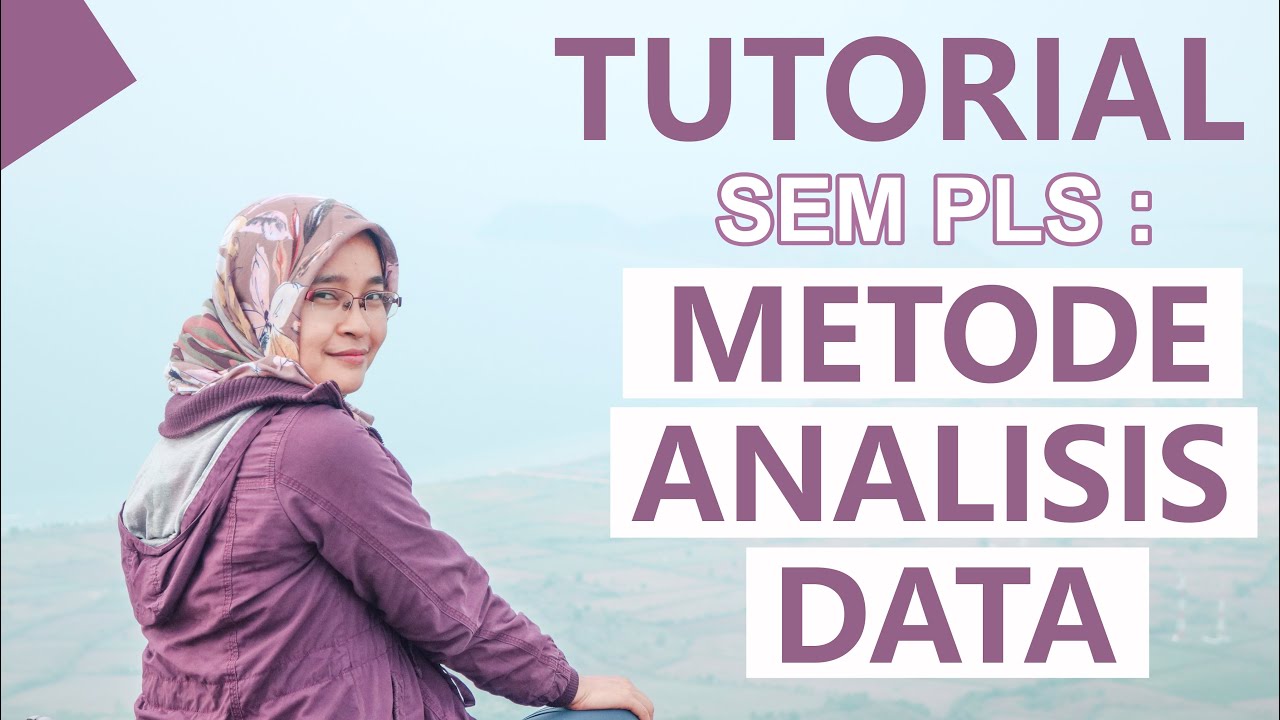
TUTORIAL SEM PLS: METODE ANALISIS DATA
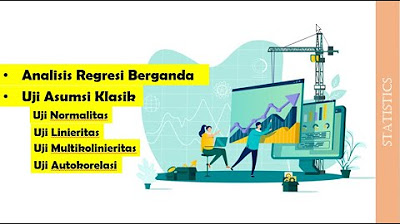
(1/4) Analisis Regresi : Uji asumsi Klasik

Uji t dan Uji F Lengkap dengan Penjelasanya Menggunakan SPSS
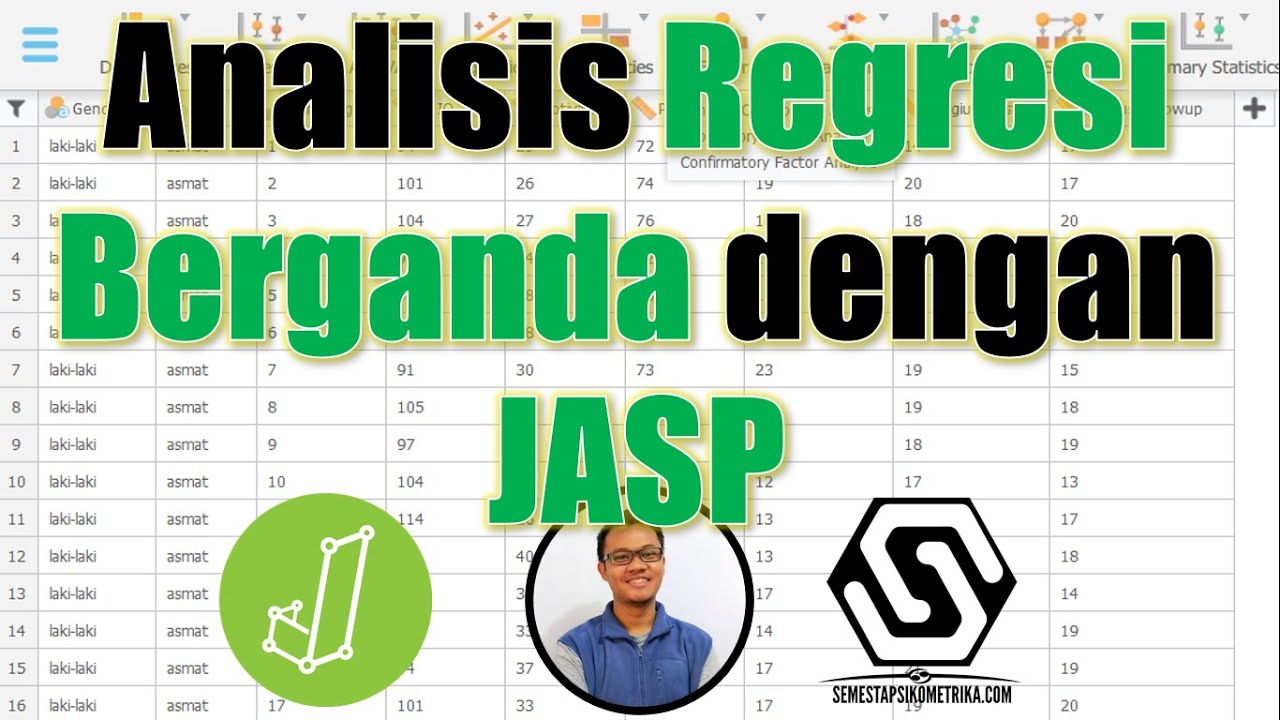
Cara Analisis Regresi Berganda dengan JASP
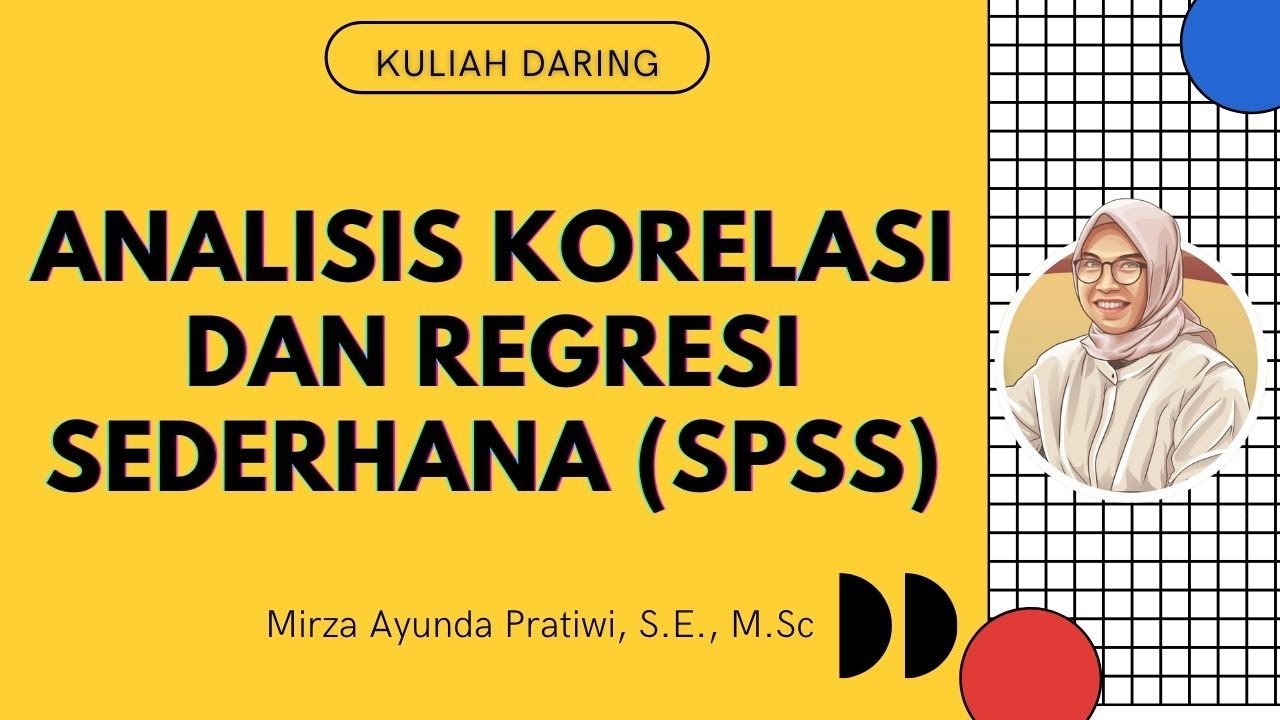
Statistika: Uji Korelasi-Regresi sederhana menggunakan SPSS
5.0 / 5 (0 votes)