Cara Analisis Regresi Berganda dengan JASP
Summary
TLDRIn this tutorial, the presenter walks viewers through performing multiple linear regression analysis using Jazz software, comparing it to SPSS. The analysis explores how motivation and IQ predict academic performance (prestasi). Key steps include checking for assumptions like normality, linearity, multicollinearity, and homoscedasticity. The tutorial also covers interpreting regression outputs such as R-squared, coefficients, and the significance of the independent variables. Viewers learn how to create a predictive model for academic performance and how to analyze the contribution of each predictor. The session provides clear, practical insights for anyone looking to perform regression analysis using Jazz.
Takeaways
- π The tutorial demonstrates how to conduct multiple regression analysis using Jazz software.
- π The dataset used for this analysis is the same as in a previous SPSS regression tutorial, with motivation and IQ as predictors for academic achievement.
- π Four key assumptions for regression analysis are tested: normality, linearity, multicollinearity, and homoscedasticity.
- π Normality of residuals is checked using a histogram, where the distribution is confirmed to be normal.
- π Multicollinearity is evaluated using collinearity statistics, confirming that motivation and IQ are not highly correlated with each other.
- π Linearity is assessed using scatter plots to check the relationship between the dependent variable (academic achievement) and independent variables (motivation, IQ).
- π Homoscedasticity is verified by ensuring that residuals are equally spread across predicted values, confirmed through scatter plots.
- π The multiple regression analysis shows that motivation and IQ together explain 76.8% of the variance in academic achievement.
- π A significant F-test (p-value < 0.001) indicates that the regression model is significant and that motivation and IQ significantly predict achievement.
- π The regression coefficients for motivation and IQ are individually significant, showing that each predictor has a substantial impact on academic achievement.
- π The final regression equation allows for the prediction of academic achievement based on values of motivation and IQ, with clear examples provided.
Q & A
What is the main focus of the video tutorial?
-The main focus of the video is to demonstrate how to conduct a multiple regression analysis using the Jazz software, specifically to predict the 'prestasi' (performance) variable based on 'motivasi' (motivation) and 'Icu' (academic score).
What are the four key assumptions tested in regression analysis?
-The four key assumptions tested in regression analysis are normality, linearity, multicollinearity, and homoscedasticity.
How is the normality assumption tested in the video?
-The normality assumption is tested by inspecting the residuals through a histogram in Jazz, which shows whether the residuals are normally distributed.
What does it mean if the residuals are normally distributed?
-If the residuals are normally distributed, it indicates that the assumption of normality is met, meaning the residuals are spread symmetrically around the mean, which is essential for valid regression results.
How is linearity tested in the analysis?
-Linearity is tested using scatter plots of residuals versus predicted values. If the plot shows a random, non-patterned distribution, the assumption of linearity is met.
What is multicollinearity, and how is it tested?
-Multicollinearity occurs when the independent variables are highly correlated with each other. It is tested by checking the Variance Inflation Factor (VIF) values; a VIF greater than 10 would indicate multicollinearity.
What does a VIF value less than 10 indicate?
-A VIF value less than 10 indicates that there is no multicollinearity, meaning the independent variables are not highly correlated with each other.
How is homoscedasticity tested in the regression analysis?
-Homoscedasticity is tested by inspecting a scatter plot of residuals versus predicted values. If the plot shows no discernible pattern, it suggests that the variance of residuals is constant across all levels of the independent variables.
What does the R-squared value represent in regression analysis?
-The R-squared value represents the proportion of the variance in the dependent variable ('prestasi') that is explained by the independent variables ('motivasi' and 'Icu'). In this case, an R-squared value of 0.768 means that 76.8% of the variation in performance is explained by the independent variables.
What is the significance of the F-test in the regression analysis?
-The F-test in regression analysis checks whether the independent variables, taken together, significantly predict the dependent variable. A p-value less than 0.01, as shown in the video, indicates that the independent variables significantly predict the performance ('prestasi').
Outlines
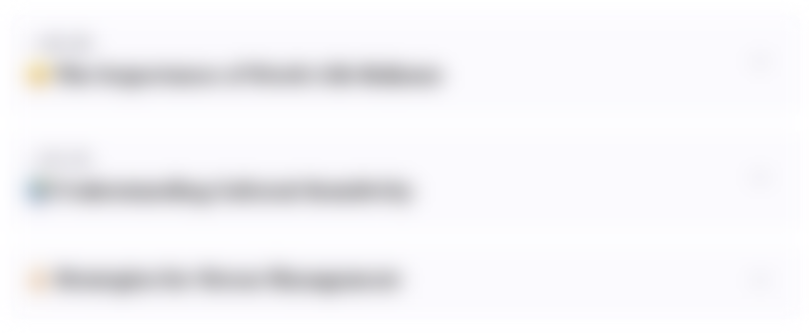
This section is available to paid users only. Please upgrade to access this part.
Upgrade NowMindmap
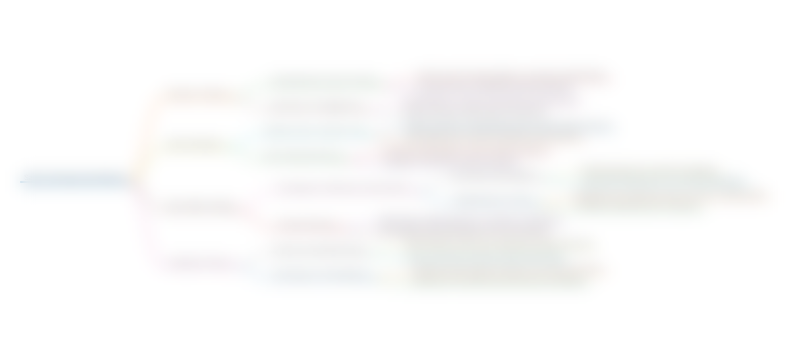
This section is available to paid users only. Please upgrade to access this part.
Upgrade NowKeywords
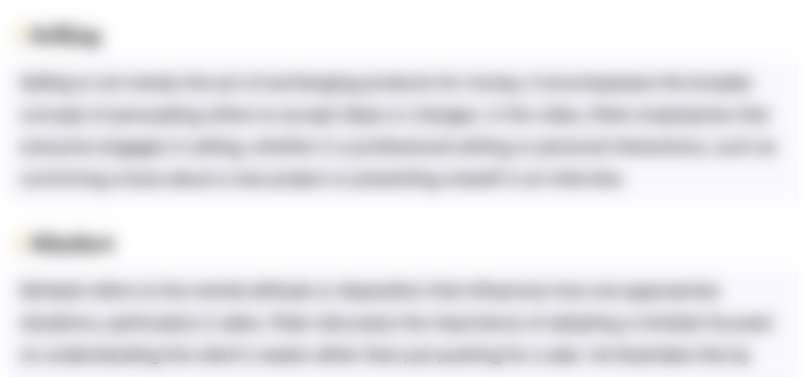
This section is available to paid users only. Please upgrade to access this part.
Upgrade NowHighlights
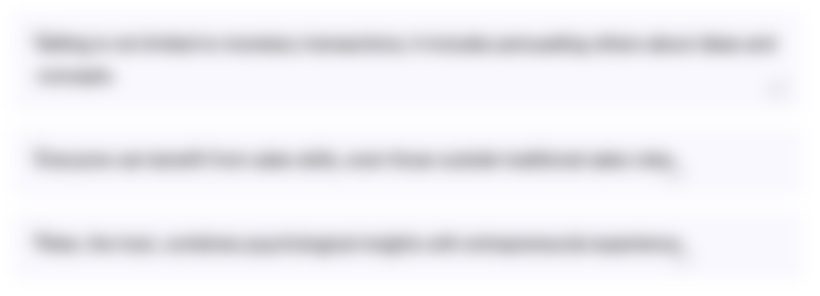
This section is available to paid users only. Please upgrade to access this part.
Upgrade NowTranscripts
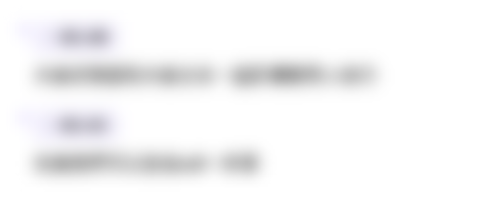
This section is available to paid users only. Please upgrade to access this part.
Upgrade NowBrowse More Related Video
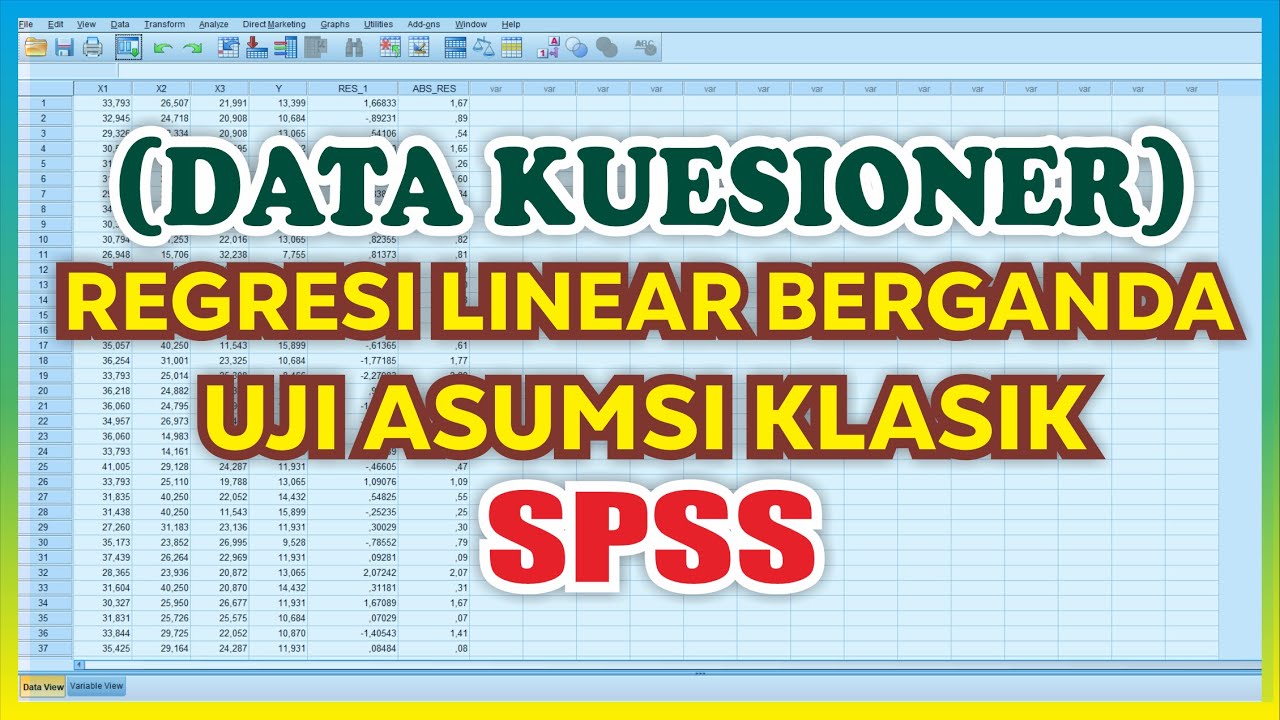
Uji Asumsi Klasik SPSS Data Kuesioner beserta Analisis Regresi Linear Berganda

Tutorial Uji Asumsi Klasik dengan SPSS serta Interpretasi Output
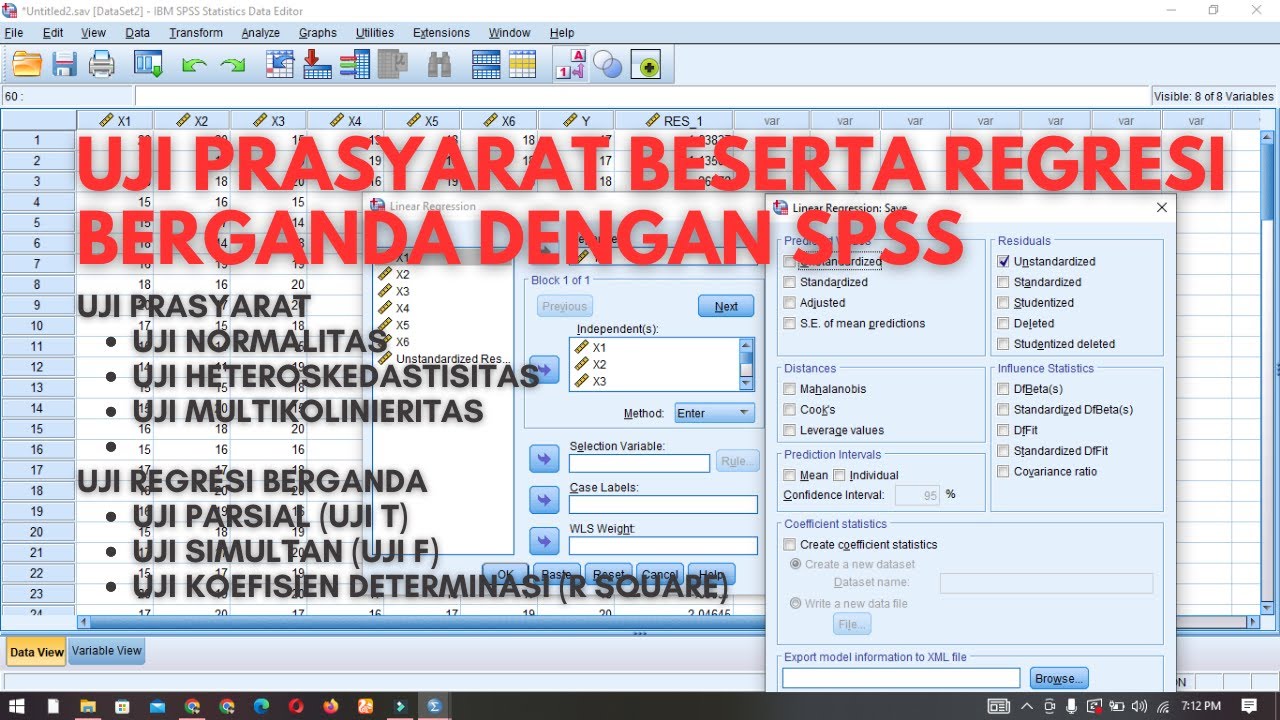
Tutorial Uji Asumsi Klasik (Prasyarat) Beserta Uji Regresi Berganda Dengan SPSS
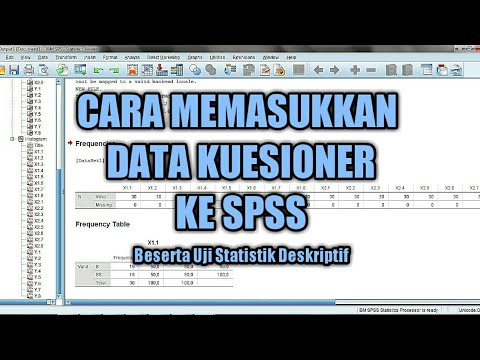
CARA MEMASUKKAN DATA KUESIONER KE SPSS - Beserta Uji Statistik Deskriptif
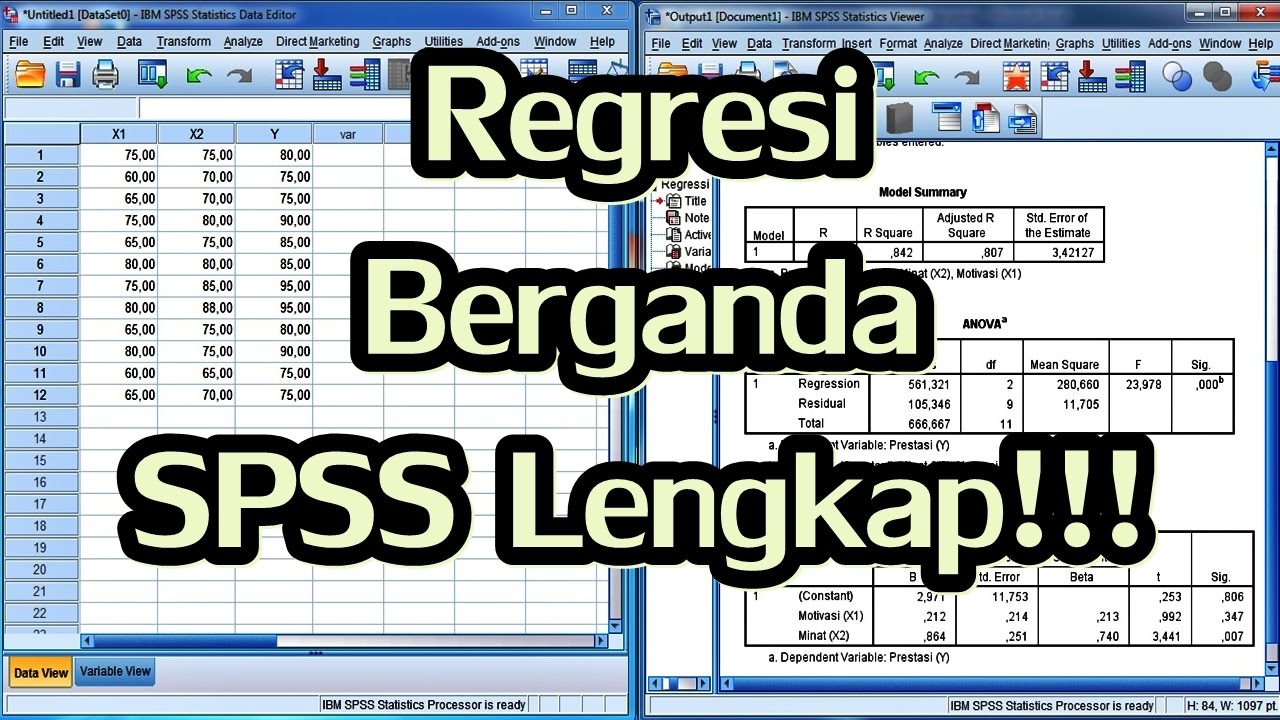
Uji t dan Uji F dalam Analisis Regresi Berganda dengan SPSS Lengkap
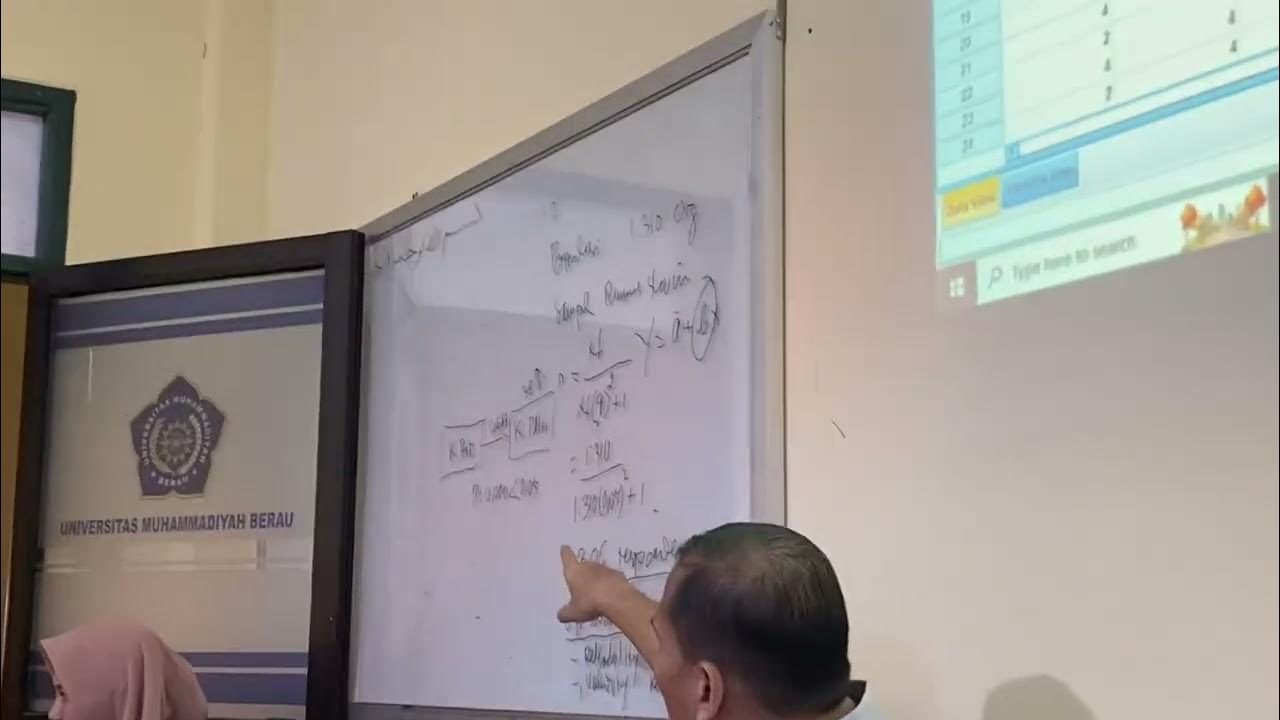
19 Oktober 2024
5.0 / 5 (0 votes)