MAX UnMix - Fitting and Optimization
Summary
TLDRThis video walks through the process of fitting coercivity spectra data using a model. It covers the key steps of setting up the model, adjusting parameters like mean coercivity, dispersion, and skewness, and adding components to the model. The video also explains how to interpret the data physically, including how to consider natural sample characteristics when selecting components. It then delves into optimization, where the model is refined, and how to statistically evaluate the fit using residual sum squares and an F-test to compare simpler and more complex models. The focus is on balancing statistical accuracy with physical interpretability.
Takeaways
- ๐ Make sure the data is correctly plotted with gray points representing the raw coercivity spectra and the dark line representing the model fit.
- ๐ The modelโs fit is initially built with one component, which can be adjusted by adding more components to refine the model for better coercivity fitting.
- ๐ Adjust the maximum field and maximum log field parameters to ensure that the mean coercivity reaches sufficient fields for accurate modeling.
- ๐ Components can be saved and renamed for later use, allowing you to reuse common components when fitting multiple datasets in a single session.
- ๐ The parameters in the model include mean coercivity (affects peak position), dispersion (controls distribution width), relative proportion (scaling of components), and skewness (shapes the distribution).
- ๐ Skewness values can indicate physical phenomena, such as thermal effects or grain interactions, which cause a skewed distribution in coercivity spectra.
- ๐ Right-skewed distributions (skewness > 1) are not allowed in the model by default, but may indicate a mixed mineralogy scenario.
- ๐ Common magnetic components like hematite, magnetite, and their various forms can serve as a basis for fitting, but should be interpreted based on the physical context of the sample.
- ๐ After fitting the model, optimization calculates the best-fitting parameters, and residual sum squared (RSS) can be used to assess model accuracy.
- ๐ An F-test can be used to statistically evaluate model complexity. If the p-value is below 0.05, the more complex model may be preferred; otherwise, the simpler model is favored.
Q & A
What is the primary objective of building an initial model on the fitting page?
-The primary objective is to fit the coercivity spectra data by building an initial model, ensuring that the model aligns well with the observed coercivity data and correctly represents the physical properties of the sample.
How do you adjust the maximum field and maximum log field parameters for the model?
-To adjust these parameters, you can set the maximum log field to a desired value, ensuring that the coercivity data extends to the required fields. For example, setting the maximum log field to 3 ensures the model captures higher coercivity values.
What does the relative proportion parameter control in the model?
-The relative proportion controls the normalized amplitude of individual components in the model. It adjusts the weight of each component but does not need to add up to one. The total contribution of each component is calculated separately.
What is the significance of skewness in the model?
-Skewness in the model controls the shape of the coercivity distribution. A skewness of 1 indicates a normal distribution, while values less than 1 (skew left) suggest effects like thermal relaxation or grain interactions. Skewness greater than 1 (skew right) is less commonly observed and may indicate mixed mineralogy.
What are common components included in the model, and why are they important?
-Common components include pedogenic magnetite, tribal magnetite, and various types of magnetofossils. These components serve as initial guides for fitting the model, as they represent well-understood magnetic minerals with specific physical properties, which help in interpreting the data.
How do you save a component within the model for future use?
-To save a component, check the option to save, name the component (e.g., 'test component'), and then click close. This saved component can be reused for fitting other datasets with similar components.
What is the role of the optimization page in fitting the model?
-The optimization page fine-tunes the model by calculating optimized values for each parameter, such as mean coercivity, dispersion, and skewness, to improve the fit between the model and the data. It outputs optimized parameter values, contributing to a better model fit.
What does the residual sum squared (RSS) represent in the model optimization?
-The residual sum squared (RSS) measures the difference between the observed data and the model's predictions. A lower RSS indicates a better fit. The optimization process aims to minimize this value, improving the model's accuracy.
What is the purpose of performing an F-test during model comparison?
-An F-test compares the fit of different models (e.g., two-component vs. three-component models) by calculating a p-value. A p-value less than 0.05 suggests that the more complex model (e.g., three components) is statistically better. If the p-value is higher, the simpler model is preferred.
How does the interpretation of results change when skewness values are above 1?
-Skewness values above 1, indicating a skew right distribution, may suggest mixed mineralogy, which is uncommon and may require further interpretation. This could mean that the model might not be fitting the sample's magnetic properties accurately, prompting a reconsideration of the components used.
Outlines
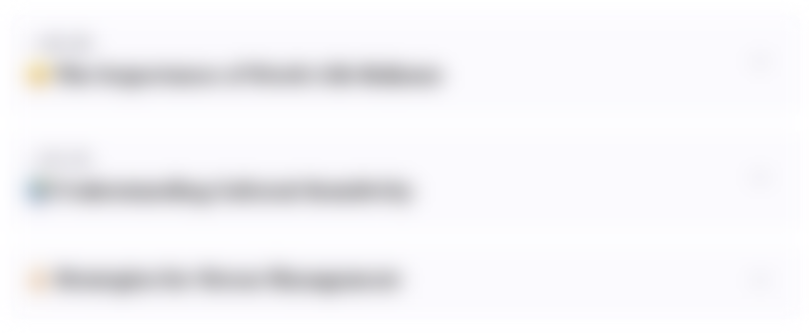
This section is available to paid users only. Please upgrade to access this part.
Upgrade NowMindmap
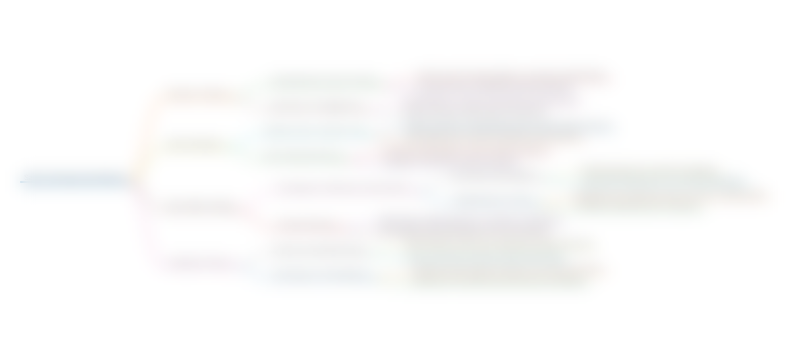
This section is available to paid users only. Please upgrade to access this part.
Upgrade NowKeywords
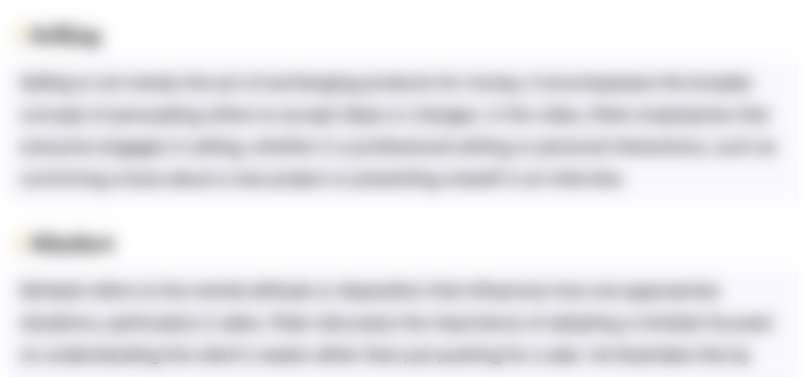
This section is available to paid users only. Please upgrade to access this part.
Upgrade NowHighlights
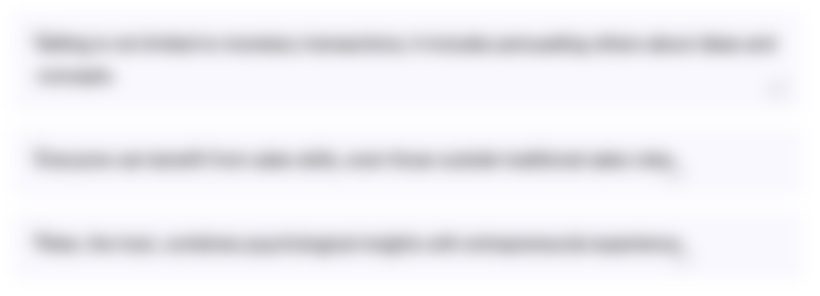
This section is available to paid users only. Please upgrade to access this part.
Upgrade NowTranscripts
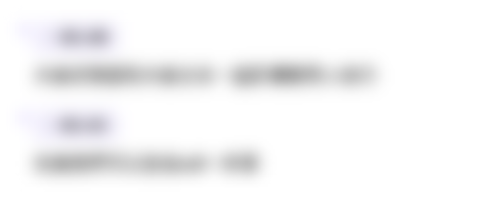
This section is available to paid users only. Please upgrade to access this part.
Upgrade NowBrowse More Related Video
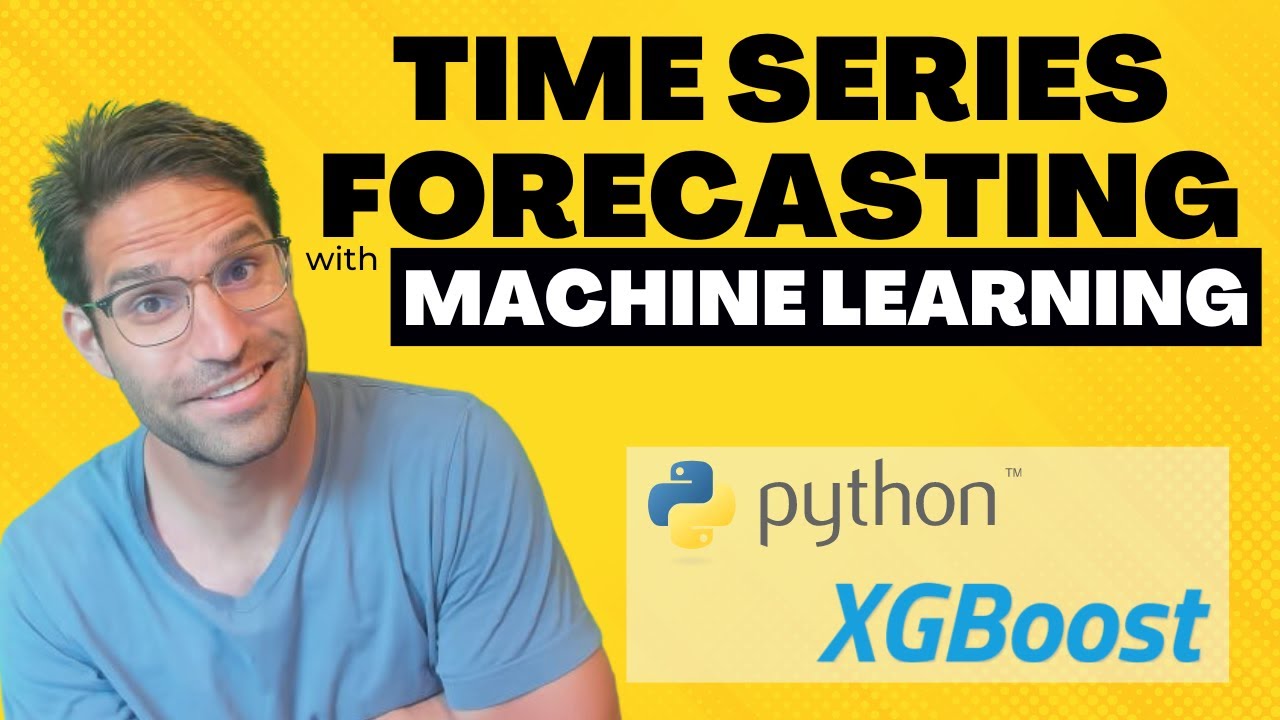
Time Series Forecasting with XGBoost - Use python and machine learning to predict energy consumption
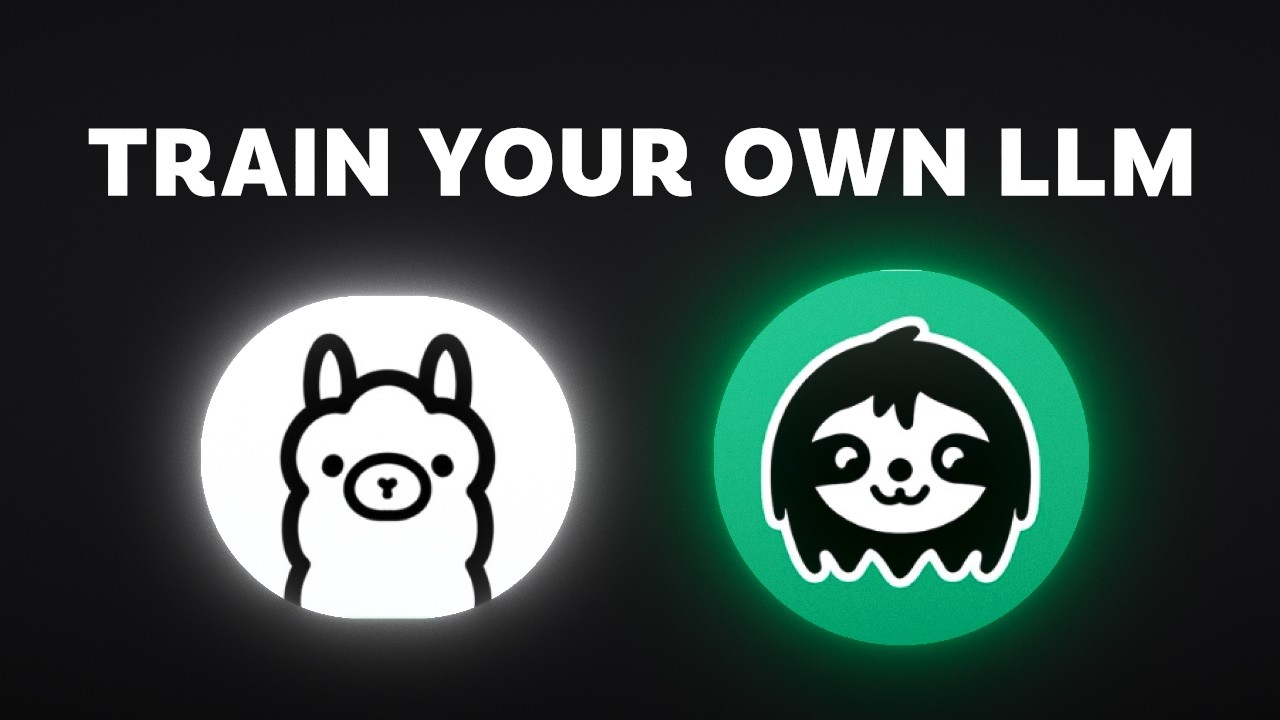
EASIEST Way to Fine-Tune a LLM and Use It With Ollama
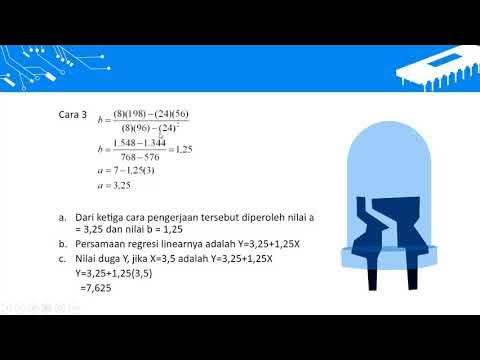
METODE NUMERIK 11 REGRESI LINIER

MAX UnMix - Data Upload
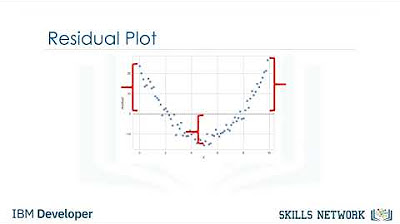
Model Evaluation using Visualization #datascience #datascience #technology #subscribeformore
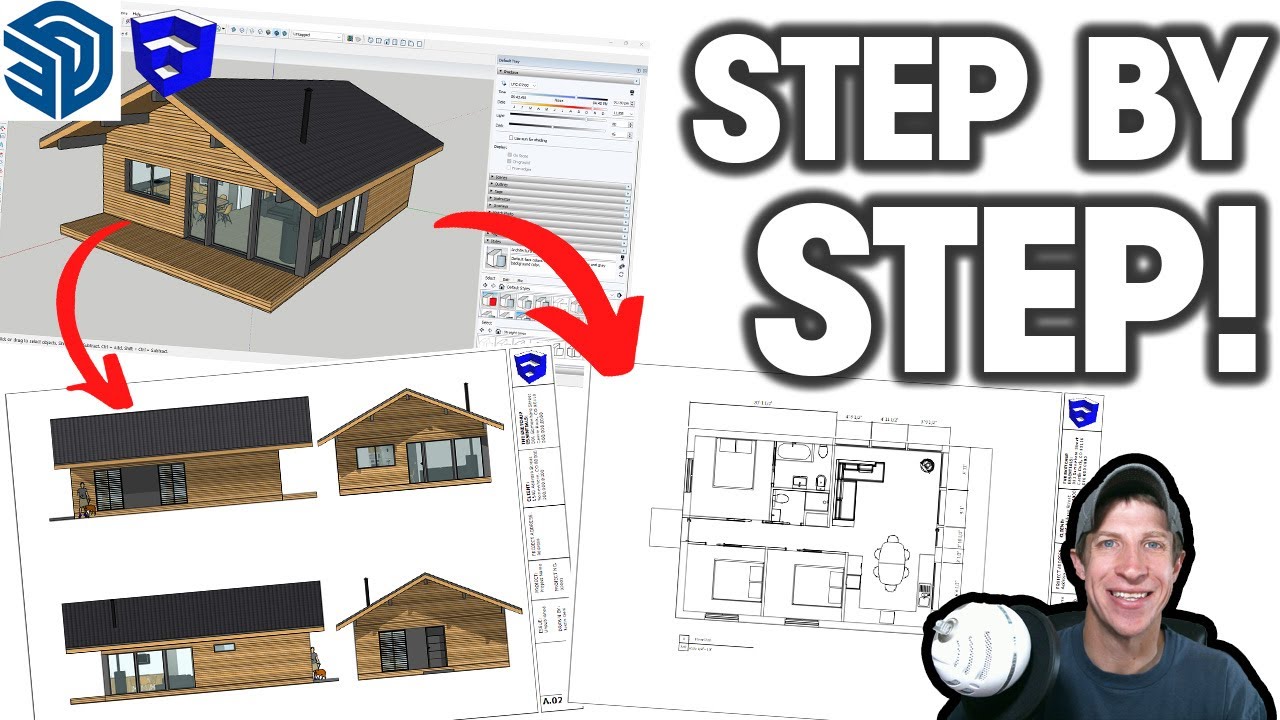
Creating PLANS IN LAYOUT from a SketchUp Model - COMPLETE PROCESS!
5.0 / 5 (0 votes)