Has Generative AI Already Peaked? - Computerphile
Summary
TLDR本文探讨了使用生成式人工智能(AI)来理解图像和文本的CLIP嵌入技术。作者质疑了通过增加数据和模型规模就能实现通用智能的观点,指出要达到零样本学习性能,所需的数据量可能是巨大的。文章通过实验发现,对于困难问题,现有模型在数据量不足的情况下表现不佳。此外,数据集中类别的分布不均也影响了模型对稀有类别的识别能力。尽管大型科技公司可能通过增加GPU和使用人类反馈来改进模型,但作者认为,若要处理在互联网文本和搜索中不常见的难题,可能需要寻找新的方法。
Takeaways
- 🧠 讨论了使用生成式AI来生成新句子、新图像等,并理解图像和文本的能力。
- 🔍 通过大量图像和文本配对学习,可以提炼图像内容的语言表示。
- 🚀 有观点认为,随着训练数据和网络规模的增加,AI将发展出跨领域的通用智能。
- 🔬 科学方法强调实验验证而非假设,对于AI性能提升的乐观预测持谨慎态度。
- 📉 近期论文指出,实现通用零样本性能所需的数据量可能非常庞大,难以实现。
- 📈 论文通过实验数据展示了数据量与模型性能之间的关系,通常呈现对数增长而非线性。
- 📊 论文分析了约4000个概念在数据集中的分布,以及它们在下游任务中的表现。
- 🌐 讨论了CLIP嵌入(图像和文本的共享嵌入空间)及其在分类、推荐系统等任务中的应用。
- 🚧 论文指出,对于困难问题,现有数据量不足以有效训练模型,导致性能受限。
- 📚 强调了数据集中类别和概念的不均匀分布问题,常见类别(如猫)过度表示,而特定类别(如某些树种)则表示不足。
- 🔑 暗示了除了收集更多数据之外,可能需要新的数据表示方法或机器学习策略来提升对困难任务的性能。
Q & A
什么是CLIP嵌入(clip embeddings)?
-CLIP嵌入是一种通过大量图像和文本对训练得到的表示方法,能够将图像和文本映射到共享的嵌入空间中,使得描述相同内容的图像和文本在嵌入空间中彼此接近。
为什么有人认为通过增加更多的数据和更大的模型就能实现通用智能(general intelligence)?
-这种观点基于观察到的现象:随着数据和模型规模的增加,AI在图像识别等领域的表现逐渐提升。因此,一些人认为,只要继续增加数据量和模型规模,AI最终能够处理所有类型的任务。
为什么说实验验证比假设更重要?
-在科学领域,实验验证是检验假设正确性的关键。仅仅提出假设而不通过实验来证明它们,无法确保这些假设在实际应用中的有效性。
这篇论文为什么反对仅通过增加数据量和模型规模就能解决所有问题的观点?
-这篇论文通过实验发现,要实现在新任务上的零样本(zero-shot)表现,所需的数据量是极其庞大的,以至于实际上无法实现。这表明仅靠增加数据和模型规模并不能无限提升AI的性能。
什么是下游任务(downstream tasks)?
-下游任务是指在训练好基本模型后,利用这些模型进行的特定应用任务,如分类、推荐系统等。
为什么说在困难问题上应用下游任务需要大量的数据支持?
-因为困难问题往往涉及到更具体的概念,这些概念在数据集中可能非常少见,导致模型无法学习到足够的特征来进行有效识别或分类。
这篇论文是如何测试不同概念在数据集中的分布和模型性能的关系的?
-论文中定义了约4000个不同的概念,并分析了这些概念在数据集中的分布情况,然后测试了在这些概念上的下游任务性能,并将其与对应概念的数据量进行对比。
为什么说数据集中的类别分布不均匀会影响模型性能?
-如果数据集中某些类别(如猫)过度表示,而其他类别(如特定树种)表示不足,模型在常见类别上的性能会很好,但在不常见类别上的性能会较差,因为模型没有足够的数据来学习这些类别的特征。
为什么说仅靠增加数据量可能无法实现AI性能的大幅提升?
-论文中的实验结果表明,随着数据量的增加,模型性能的提升会逐渐趋于平缓,即达到一个平台期,这意味着继续增加数据量可能无法带来预期的性能提升。
这篇论文对于未来AI发展的意义是什么?
-这篇论文提供了对当前AI发展模式的批判性思考,提示我们可能需要寻找新的方法或策略来提升AI的性能,而不仅仅是依赖于数据量的增加。
Outlines
🤖 AI与图像文本嵌入的局限性
本段讨论了AI在图像和文本嵌入方面的应用及其局限性。提到了通过大量图像和文本对来训练AI,使其能够将图像内容转化为语言描述的尝试。然而,有观点认为,随着数据量和模型规模的增加,AI将实现通用智能,但最新研究显示,要达到新的未见任务的零样本性能,所需的数据量将是巨大的。论文通过实验表明,对于困难问题,没有足够的数据支持,这些模型无法有效应用。此外,还提到了下游任务,如分类和推荐系统,以及它们如何利用图像和文本的嵌入空间来提高性能。
📈 数据量与AI性能的关系
这段内容通过一个图表形象地展示了数据量与AI性能之间的关系。研究者定义了约4000个核心概念,并分析了这些概念在数据集中的普遍性,以及它们在下游任务中的表现。实验结果表明,随着数据量的增加,AI性能的提升并不是线性的,而是呈现出对数增长,最终趋于平缓。这意味着,尽管增加数据量和模型规模可以提升AI性能,但很快就会达到一个性能提升的瓶颈。此外,数据集中类别和概念的分布不均也是导致性能下降的一个因素,常见类别如猫和狗的数据量远大于特定树种等不常见类别。
🎮 特定任务下AI的挑战与未来展望
最后一段讨论了AI在处理特定任务时遇到的挑战,尤其是在数据量不足的情况下。举例说明了当AI被要求生成不常见物体的图像或解释复杂概念时,性能会下降。同时,提出了对于AI未来发展的一些思考,包括是否需要新的数据表示方法或机器学习策略来突破当前的性能瓶颈。此外,还提到了Jane Street公司提供的技术问题解决项目,鼓励对计算机和问题解决感兴趣的观众参与。
Mindmap
Keywords
💡CLIP嵌入
💡生成式AI
💡通用智能
💡数据集
💡零样本学习
💡下游任务
💡推荐系统
💡概念普及度
💡性能提升
💡模型泛化
💡数据分布
💡机器学习策略
Highlights
讨论了使用生成性AI来生成新句子和图像,并理解图像和文本。
提出了通过足够多的图像和文本配对学习,可以提炼图像内容的语言表示。
提出了随着训练数据和网络规模的增加,AI将发展出跨领域的通用智能。
科学界通常通过实验来验证假设,而不是仅仅基于理论推测。
最近发表的一篇论文认为,要实现零样本学习,需要的数据量将非常庞大。
论文中的数据和图表显示,增加数据量和模型规模并不能无限提高性能。
介绍了CLIP嵌入的概念,包括图像和文本的Transformer编码器。
CLIP嵌入可以用于分类、图像召回和推荐系统等下游任务。
论文指出,对于困难问题,没有大量数据支持,CLIP嵌入的下游任务效果不佳。
论文通过定义核心概念,并分析这些概念在数据集中的普遍性,来测试下游任务的表现。
实验结果表明,对于某些概念,即使数据量增加,性能提升也有限。
论文提出了对于数据集中类别和概念分布不均的问题。
讨论了大型语言模型在处理训练集中不常见的问题时可能出现的准确性下降。
提出了对于困难任务,可能需要寻找除收集更多数据之外的其他方法。
论文的实验结果对于AI领域的未来发展提出了质疑和思考。
讨论了大型科技公司可能对AI发展过于乐观的宣传。
提出了对于AI模型训练成本和效率的考量。
提到了Jane Street公司提供的技术问题解决项目和赞助。
Transcripts
so we looked at clip embeddings right
and we've talked a lot about using
generative AI to produce new sentences
to produce new images and so on and so
to understand images all these kind of
different things and the idea was that
if we look at enough pairs of images and
text we will learn to distill what it is
in an image into that kind of language
so the idea is you have an image you
have some texts and you can find a
representation where they're both the
same the argument has gone that it's
only a matter of time before we have so
many images that we train on and so and
such a big Network and all this kind of
business that we get this kind of
general intelligence or we get some kind
of extremely effective AI that works
across all domains right that's the
implication right the argument is and
you see a lot in the sort of tech sector
from the from some of these sort of um
big tech companies who to be fair want
to sell products right that if you just
keep adding more and more data or bigger
and bigger models or a combination of
both ultimately you will move Beyond
just recognizing cats and you'll be able
to do anything right that's the idea you
show enough cats and dogs and eventually
the elephant just is
implied as someone who works in science
we don't hypothesize about what happens
we experimentally justify it right so I
would say if you're going to if you're
going to say to me that the only upward
trajectory is is going you know the only
trajectory is up it's going to be
amazing I would say go on and prove it
and do it right and then we'll see we'll
sit here for a couple of years and we'll
see what happens but in the meantime
let's look at this paper right which
came out just recently this
paper is saying that that is not true
right this paper is saying that the
amount of data you will need to get that
kind of General zero shot performance
that is to say performance on new tasks
that you've never
seen is going to be astronomically vast
to the point where we cannot do it right
that's the idea so it basically is
arguing against the idea that we can
just add more data and more models and
we we'll solve it right now this is only
one p
and of course you know your mileage may
vary if you have a bigger GPU than these
people and so on but I think that this
is actual numbers right which is what I
like because I want to see tables of
data that show a trend actually
happening or not happening I think
that's much more interesting than
someone's blog post that says I think
this is going what's going to happen so
let's talk about what this paper does
and why it's interesting we have clip
embeddings right so we have an image we
have a big Vision Transformer and we
have a big text encoder which is another
Transformer bit like the sort of you
would see in a large language model
right which takes text strings my text
string today and we have some shared
embedded space and that embedded space
is just a numerical fingerprint for the
meaning in these two items and they're
trained remember across many many images
such that when you put the same image
and the text that describes that image
in you get something in the middle that
matches and the idea then is you can use
that for other tasks like you can use
that for classification you can use it
for image recall if you use a streaming
service like Spotify or Netflix right
they have this thing called a recom
recommended system a recommended system
is where you've watched this program
this program this program what should
you watch next right and you you might
have noticed that your mileage may vary
on how effective that is but actually I
think they're pretty impressive what
they have to do but you could use this
for a recommender system because you
could say basically what programs have I
got that embed into the same space of
all the things I just watched and and
recommend them that way right so there
are Downstream tasks like classification
and recommendations that we could use
based on a system like this what this
paper is showing is that you cannot
apply these effectively these Downstream
tasks for difficult problems without
massive amounts of data to back it up
right and so and the idea that you can
apply you know this kind of
classification on hard things so not
just cats and dogs but specific cats and
specific dogs or subspecies of tree
right or difficult problems where the
the answer is more difficult than just
the broad category that there isn't
enough data on those things to train
these models and way I've got one of
those apps that tells you what specific
species a tree is so is it not just
similar to that no because they're just
doing classification right or some other
problem they're not using this kind of
generative giant AI right the argument
has been why do that silly little
problem where you can do a general
problem and solve all your problems
right and the response is because it
didn't work right that's that's that's
that's why we're doing it um so there
are pros and cons for both right I'm not
going to say that no generative AI is
useful or no or these these models are
incredibly effective for what they do
but I'm perhaps suggesting that it may
not be reasonable to expect them to do
very difficult medical diagnosis because
you haven't got the data set to back
that up right so how does this paper do
this well what they do is they def they
Define these Core Concepts right so some
of the concepts are going to be simple
ones like a cat or a person some of them
are going to be slightly more difficult
like a specific species of cat or a
specific disease in an image or
something like this and they they come
up about
4,000 different concepts right and these
are simple text Concepts right these are
not complicated philosophical ideas
right I don't know how well it embeds
those and and what they do is they look
at the prevalence of these Concepts in
these data sets and then they sh they
they test how well the downstream task
of let's say one zero shot
classification or recall recommended
systems works on all of these different
concepts and they plot that against the
amount of data that they had for that
specific concept right so let's draw a
graph and that will help me make it more
clear right so let's imagine we have a
graph here like this and this is the
number of
examples in our training set of a
specific concept right so let's say a
cat a dog something more difficult and
this is the performance on the actual
task of let's say recommend a system or
recall of an object or the ability to
actually classify as a cat right
remember we talked about how you could
use this for zero shck classification by
just seeing if it embeds to the same
place as a picture of a cat the text a
picture of a cat that kind of process so
this is performance right the best case
scenario if you want to have an all
powerful AI that can solve all the
world's problems is that this line goes
very steeply upwards right this is the
exciting case it goes like like this
right that's the exciting case this is
the kind of AI explosion argument that
basically says we're on the Custer
something that's about to happen
whatever that may be where the scale is
going to be such that this can just do
anything right okay then there the
perhaps slightly more reasonable should
we say pragmatic interpretation which is
like just call it balanced right which
is but there a sort of linear movement
right so the idea is that we have to add
a lot of examples but we are going to
get a decent performance Boost from it
right so we just keep adding examples
we'll keep getting better and that's
going to be great and remember that if
we ended up up here we have something
that could take any image and tell you
exactly what's in it under any
circumstance right that's that's kind of
what we're aiming for and similarly for
large language models this would be
something that could write with
Incredible accuracy on lots of different
topics or for image generation it would
be something that could take your prompt
and generate a photorealistic image of
that with almost no coercion at all
that's kind of the goal this paper has
done a lot of experiments on a lot of
these Concepts across a lot of models
across a lot of Downstream tasks and
let's call this the evidence what you're
going to call it pessimistic now it is
pessimistic also right it's logarithmic
so it basically goes like this right
flattens out it flattens out now this is
just one paper right it doesn't
necessarily mean that it will always
flatten out but the argument is I think
that and it's not an argument they
necessarily make in in the paper but you
know the paper's very reasonable I'm
being a bit more Cavalier with my
wording the suggestion is that you can
keep adding more examples you can keep
making your models bigger but we are
soon about to hit a plateau where we
don't get any better and it's costing
you millions and millions of dollars to
train this at what point do you go well
that's probably about as good as we're
going to get with technology right and
then the argument goes we need something
else we need something in the
Transformer or some other way of
representing data or some other machine
learning strategy or some other strategy
that's better than this in the long term
if we want to have this line G up here
or this line gar up here that's that's
kind of the argument and so this is
essentially
evidence I would argue against the kind
of
explosion you know possibility of but
just you just add a bit more data and we
were on the cusp of something we might
come back here in a couple of years you
know if you're still allow me on
computer file after this absolute
embarrassment of of these claims that I
made um and we say okay actually the
performan has improve improved massively
right or we might say we've doubled the
number of data sets to 10 billion images
and we've got 1% more right on the on on
the classification to which is good but
is it worth it I don't know this is a
really interesting paper because it's
very very fough right if there's a lot
of evidence there's a lot of Curves and
they all look exactly the same it
doesn't doesn't matter what method you
use it doesn't matter what data set you
train on it doesn't matter what your
Downstream task is the vast majority of
them show this kind of problem and the
other problem is that we don't have a a
nice even distribution of classes and
Concepts within our data set so for
example cats you can imagine are over um
emphasized or over represented over
represented yeah over represented in the
data set by an order of magnitude right
whereas specific planes or specific
trees are incredibly under represented
because you just have tree right so I
mean trees are probably going to be less
represented than cats anyway but then
specific species of tree very very
underrepresented which is why when you
ask one of these models what kind of cat
is this or what kind of tree is this it
performs worse than when you ask it what
animal is this because it's a much
easier problem and you see the same
thing in image generation if you ask it
to draw a picture of something really
obvious like a castle where that comes
up a lot in the training set it can draw
you a Fant fantastic castle in the style
of Monet and it can do all this other
stuff but if you ask it to draw some
obscure artifact from a video game
that's barely even made it into the
training set suddenly it's starting to
draw something a little bit less quality
and the same with large language models
this paper isn't about large language
models but the same process you can see
actually already happening if you talk
to something like chap GPT when you ask
it about a really important topic from
physics or something like this it will
usually give you a pretty good
explanation of that thing because that
in the training set but the question is
what happens when you ask it about
something more difficult right when you
ask it to write that code which is
actually quite difficult to write and it
starts to make things up it starts to
hallucinate and it starts to be less
accurate and that is essentially the
performance degrading because it's under
represented in the training set the
argument I think is at least it's the
argument that I'm starting to come
around to thinking if you want
performance on hard tasks tasks that are
under represented on just general
internet text and searches we have to
find some other way of doing it than
just is collecting more and more data
right particularly because it's
incredibly inefficient to do this right
on the other hand we they you know these
companies will they've got a lot more
gpus than me right they're going to
train on on bigger and bigger corpuses
better quality data they're going to use
human feedback to better train their
language models and things so they may
find ways to improve this you know up
this way a little bit as we go forward
but it's going to be really interesting
see what happens because you know will
it Plateau out will we see trap GPT 7
or8 or 9 be roughly the same as chat
dpt4 or will we see another
state-of-the-art performance boost every
time I'm kind of trending this way but
you know it'll be excited to see if it
goes this way take a look at this puzzle
devised by today's episode sponsor Jane
straight it's called bug bite inspired
by debugging code that world we're all
too familiar with where solving one
problem might lead to a whole chain of
others we'll link to the puzzle in the
video description let me know how you to
get on and speaking of Jane Street we're
also going to link to some programs that
they're running at the moment these
events are all expenses paid and give a
little taste of the tech and problem
solving used at trading firms like Jane
Street are you curious are you Problem
Solver are you into computers I think
maybe you are if so well you may well be
eligible to apply for one of these
programs check out the links below or
visit the Jane Street website and follow
the these links there are some deadlines
coming up for ones you might want to
look at and there are always more on the
horizon our thanks to Jane Street for
running great programs like this and
also supporting our Channel and don't
forget to check out that bug bite puzzle
Browse More Related Video
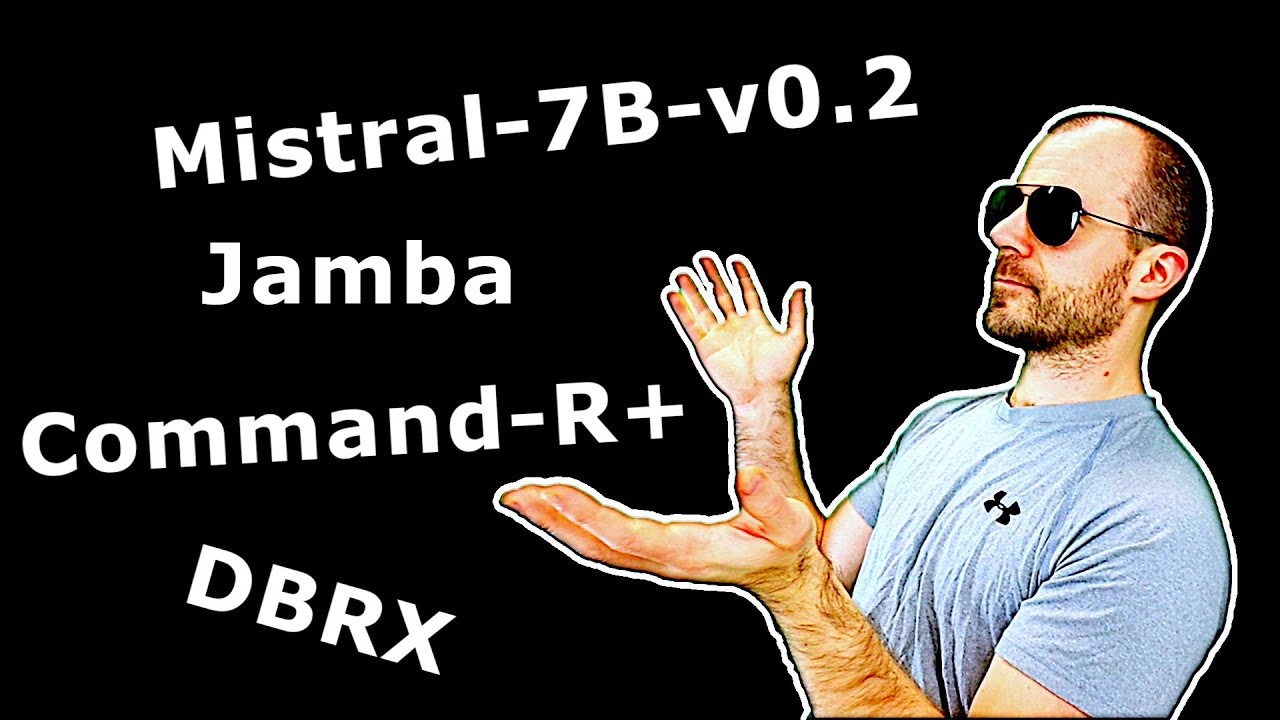
[ML News] Jamba, CMD-R+, and other new models (yes, I know this is like a week behind 🙃)
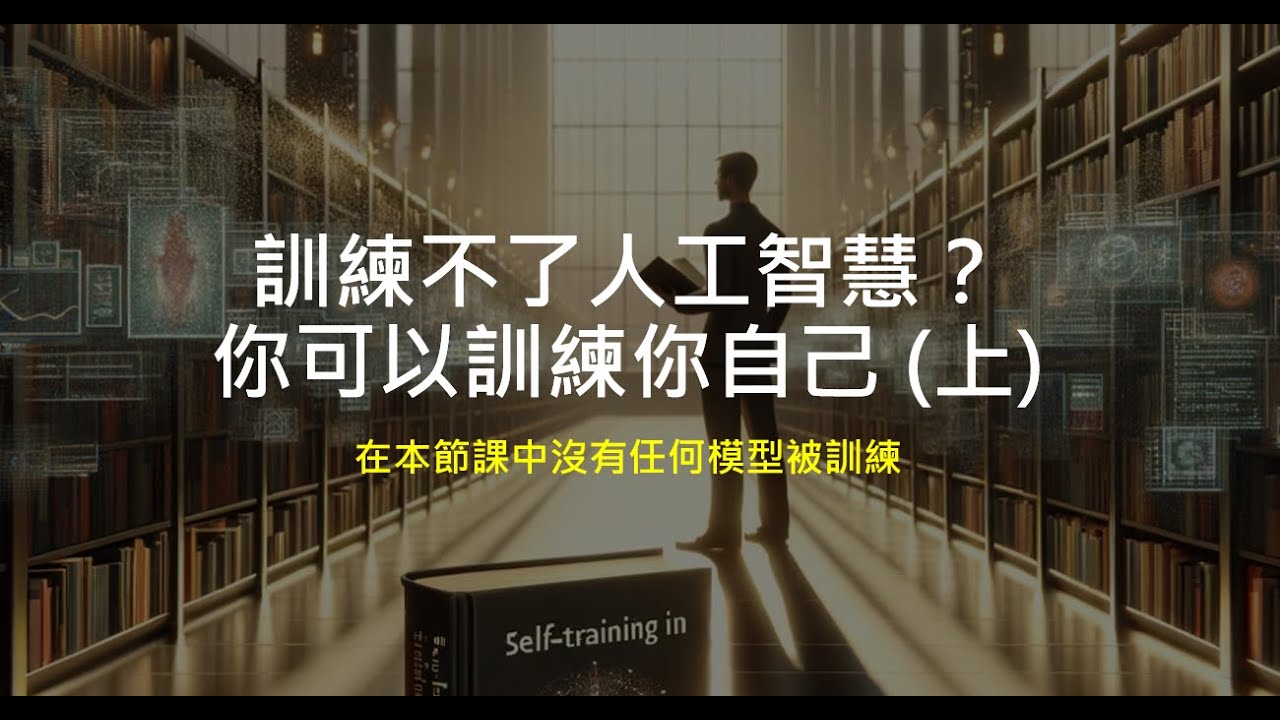
【生成式AI導論 2024】第3講:訓練不了人工智慧?你可以訓練你自己 (上) — 神奇咒語與提供更多資訊

[ML2021] Pytorch Tutorial 2

How Did Dario & Ilya Know LLMs Could Lead to AGI?
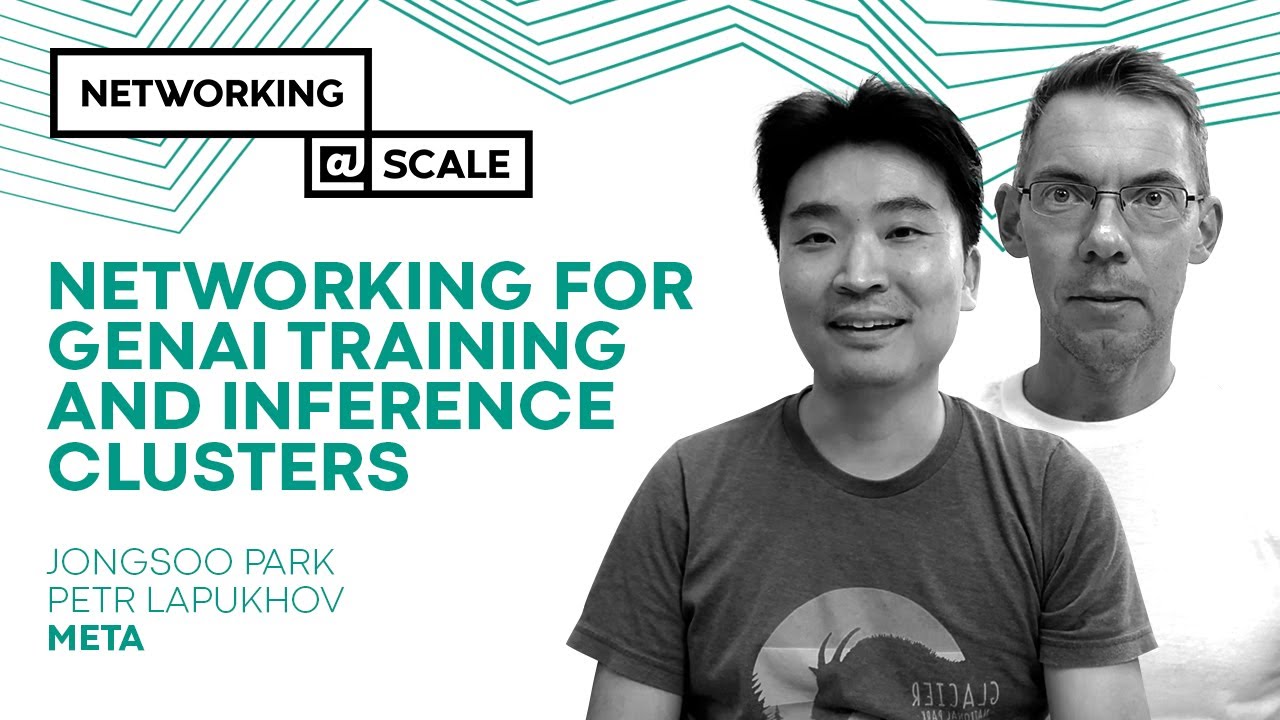
Networking for GenAI Training and Inference Clusters | Jongsoo Park & Petr Lapukhov

【生成式AI導論 2024】第18講:有關影像的生成式AI (下) — 快速導讀經典影像生成方法 (VAE, Flow, Diffusion, GAN) 以及與生成的影片互動
5.0 / 5 (0 votes)