[ML News] Jamba, CMD-R+, and other new models (yes, I know this is like a week behind 🙃)
Summary
TLDR在这段视频脚本中,我们探索了最近两周内发布的一些令人兴奋的新型人工智能模型。首先,AI 22 Labs 推出了 Jamba 模型,这是一个混合模型,结合了 Mamba 架构和注意力层,实现了长文本理解而不会显著增加内存需求。Data Bricks 也发布了一个超过100亿参数的大型语言模型,它在自然语言处理、编程和数学方面表现出色。此外,还介绍了 Cohere 的 Command R Plus,这是一个性能更强的模型,专门针对命令优化和检索增强的生成进行了优化。Google Research 展示了 Video Poet,这是一个文本到视频的零样本视频生成模型,以及 Magic Lens,一个使用自然语言检索图像的模型。还有关于合成数据生成的研究,以及 Salesforce AI Research 发布的 MOAI 时间序列基础模型,旨在提供通用的预测能力。最后,还提到了 H2O AI 的 H2O Den 2,一个在多个基准测试中表现出色的1.8亿参数基础语言模型。这些模型的发展不仅展示了人工智能领域的进步,也预示着未来技术应用的广阔前景。
Takeaways
- 🚀 AI 22 labs发布了Jamba模型,这是一个混合模型,结合了Mamba架构和注意力层,能够在不增加内存需求的情况下实现长文本上下文的推理。
- 📚 Databricks推出了新的dbrx模型,这是一个超过100亿参数的大型语言模型,不仅在自然语言理解上表现出色,还在编程和数学方面有优异的性能。
- 🔍 Cohere发布了Command R Plus,这是一个优化的命令检索增强生成模型,支持10种不同的语言,但需要在商业用途中付费。
- 📈 Mistil发布了新的7B模型,这是一个基础模型,用于训练他们的新指令模型,具有32,000个令牌的上下文窗口和指令进行微调。
- 🎥 Google Research的Video Poet是一个文本到视频的零样本视频生成模型,能够处理各种文本和视频混合的任务。
- 🔎 Google Deep Mind的Magic Lens是一个图像检索模型,使用自然语言进行检索,并且大部分使用合成数据进行训练。
- 🧪 Cosmomedia项目是Fi模型的一个开源复制,使用合成数据训练,旨在展示高质量训练数据的重要性。
- 📊 Google Deep Mind的另一份论文探讨了在大型语言模型中进行长篇事实性研究,开发了一种验证方法和模型,以提高事实性评估的准确性。
- 🤖 Snap和特拉维夫大学的研究论文myvLM探讨了如何个性化视觉语言模型,使模型能够从用户的视角理解和推理特定的概念。
- ⏱️ Nvidia的Latte 3D是一个文本到3D模型,能够快速生成高质量的纹理网格,推动了文本到3D领域的研究。
- 🌐 Meta的场景脚本模型旨在理解周围的物理空间,支持增强现实技术,通过合成数据进行训练。
Q & A
Jamba模型是如何结合Mamba架构和注意力层的?
-Jamba模型是一种混合模型,它结合了Mamba架构和一些注意力层。Mamba架构是一种状态空间模型的进化,类似于循环神经网络,但能够一次性完成所有操作。Jamba模型通过这种结合实现了非常长的上下文性能推断,同时没有显著增加内存需求。
dbrx模型在哪些领域表现出色?
-dbrx模型不仅在自然语言理解方面表现出色,还在编程和数学领域也有很好的表现。它是一个大型模型,拥有超过100亿参数,并使用了专家混合架构,总共有1320亿参数,但任何给定输入时只有360亿参数是活跃的。
Command R plus模型的主要特点是什么?
-Command R plus是一个更高效的状态命令优化和检索增强的生成优化模型。它支持10种不同的语言,并且被训练用于引用和使用工具。这个模型不是开源的,而是以开放权重的形式提供,允许个人使用,但在商业环境中使用需要向Cohere支付费用。
视频诗人(Video Poet)模型是如何工作的?
-视频诗人模型是一个文本到视频的零样本视频生成模型,它不仅能够进行视频生成,还能够处理任何类型的文本和视频混合。该模型经过多种不同目标的训练,使其能够执行文本到视频、图像到视频的风格化等任务。
Magic Lens模型的主要功能是什么?
-Magic Lens模型是一个图像检索模型,它使用开放式指令,允许用户使用自然语言进行检索,模型会找到相应的内容。它在很大程度上是使用合成数据生成开发的,包括网络抓取、数据集分组、清洁、元数据扩展等步骤。
CosmoPedia项目的目的是什么?
-CosmoPedia项目是尝试使用合成数据重新创建类似于微软的Fi模型的训练过程。Fi模型主要在教科书上进行训练,CosmoPedia项目的目的是通过使用高质量的合成数据,减少训练数据的需求,从而使得模型不必过于庞大也能表现出色。
LLM(大型语言模型)基础事实验证的重要性是什么?
-LLM基础事实验证的重要性在于它可能标志着基于LLM的事实验证时代的开始。通过使用LLM代理作为评估者,开发了一种安全搜索增强的事实性评估方法,该方法在与人群源化注释者的意见不一致时,有76%的情况下自动系统是正确的。
myvLM模型的目的是什么?
-myvLM模型的目的是个性化视觉语言模型。它尝试教授视觉语言模型关于“我”、“我的狗”、“我的房子”和“我的朋友”等概念,使模型能够从第一人称视角回答问题和接收指令。
Latte 3D模型的主要创新点是什么?
-Latte 3D模型的主要创新点在于它能够非常快速地从文本生成高质量的纹理网格。它通过结合3D先验、摊销优化和表面渲染的第二阶段,在仅400毫秒内稳健地生成这些网格。
Dolphin 2.8模型的特点是什么?
-Dolphin 2.8模型是一个微调的mstl 7B模型,它的特点是未审查的,意味着数据集已经被过滤以去除任何偏见和对齐样本。这使得模型更加合规,建议在将模型作为服务公开之前实施自己的对齐层。
Quen 1.5e模型的性能如何?
-Quen 1.5e模型的性能与7亿参数模型相当,但它只有三分之一的激活参数。这表明通过使用混合专家模型,可以在减少活跃参数数量的同时保持模型性能。
Outlines
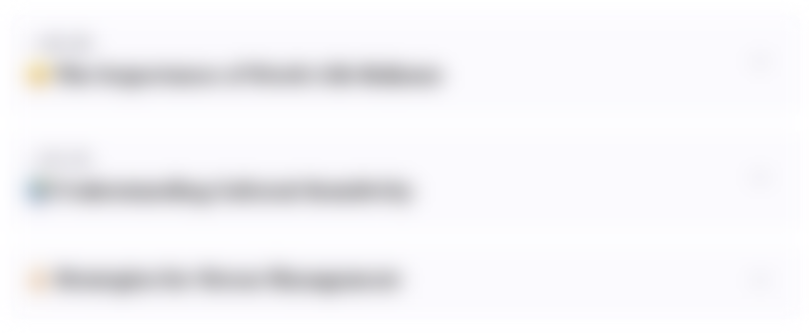
This section is available to paid users only. Please upgrade to access this part.
Upgrade NowMindmap
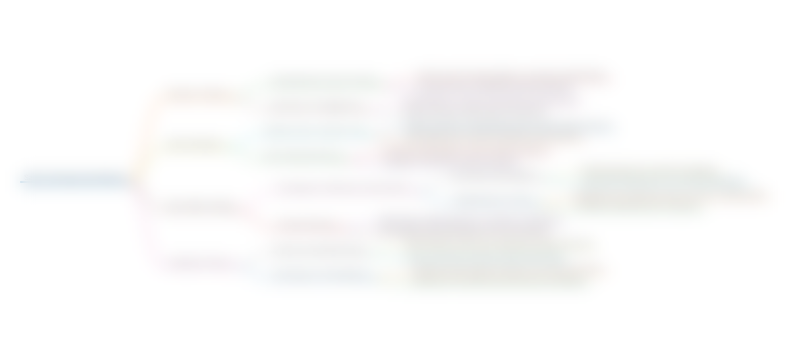
This section is available to paid users only. Please upgrade to access this part.
Upgrade NowKeywords
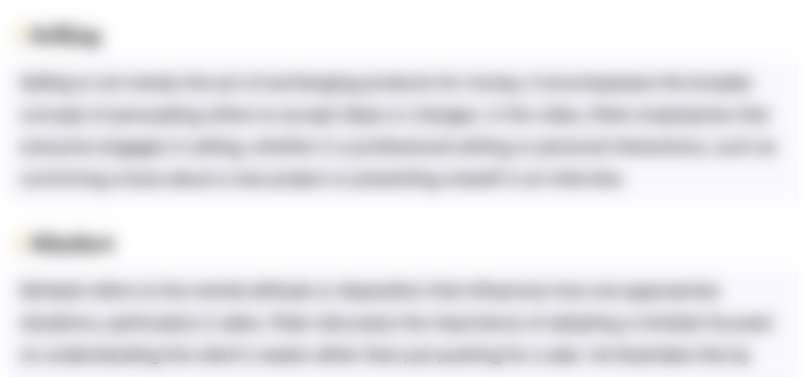
This section is available to paid users only. Please upgrade to access this part.
Upgrade NowHighlights
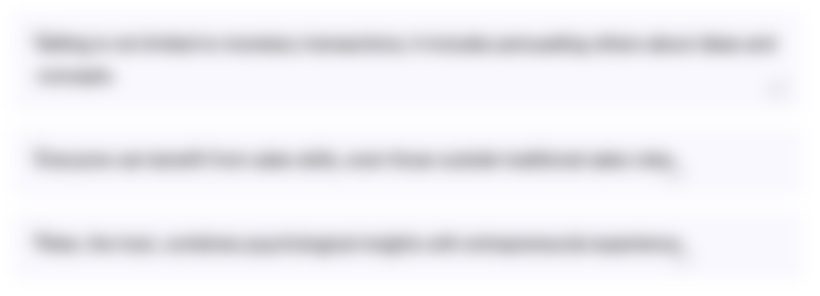
This section is available to paid users only. Please upgrade to access this part.
Upgrade NowTranscripts
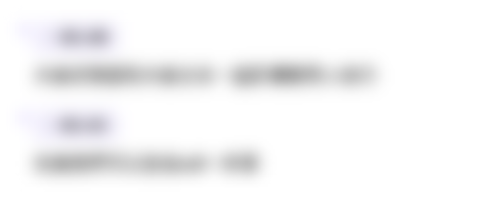
This section is available to paid users only. Please upgrade to access this part.
Upgrade NowBrowse More Related Video
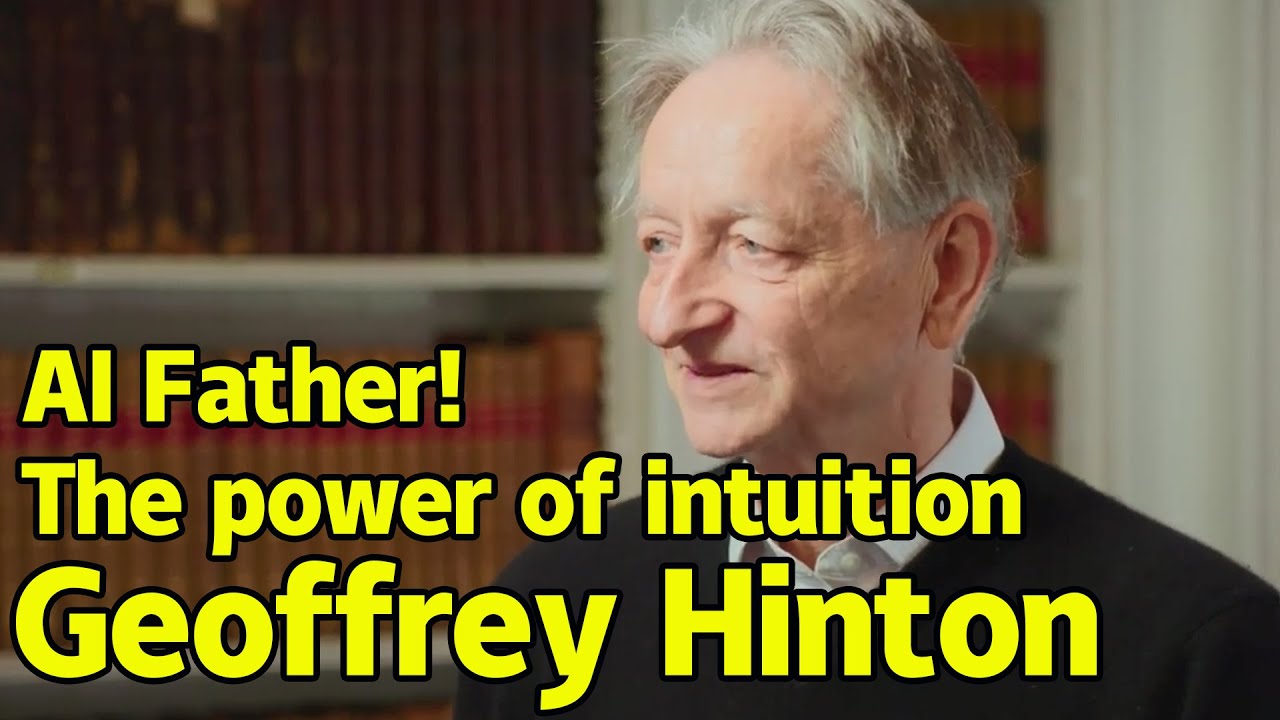
Geoffrey Hinton | Ilya's AI tutor Talent and intuition have already led to today’s large AI models
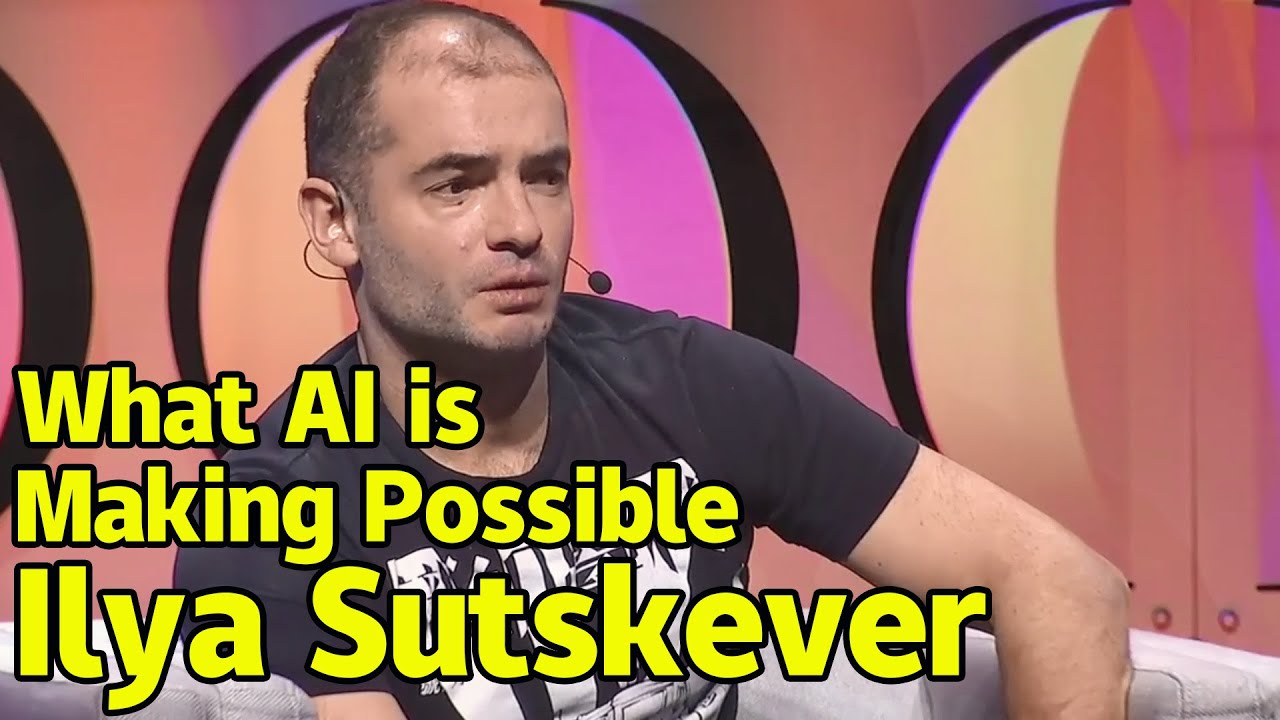
Ilya Sutskever | AI will be omnipotent in the future | Everything is impossible becomes possible

Stable Diffusion Best Model For Interior Designing And Home designing | #stablediffusion Best Model

OpenAI o3 and o3-mini—12 Days of OpenAI: Day 12
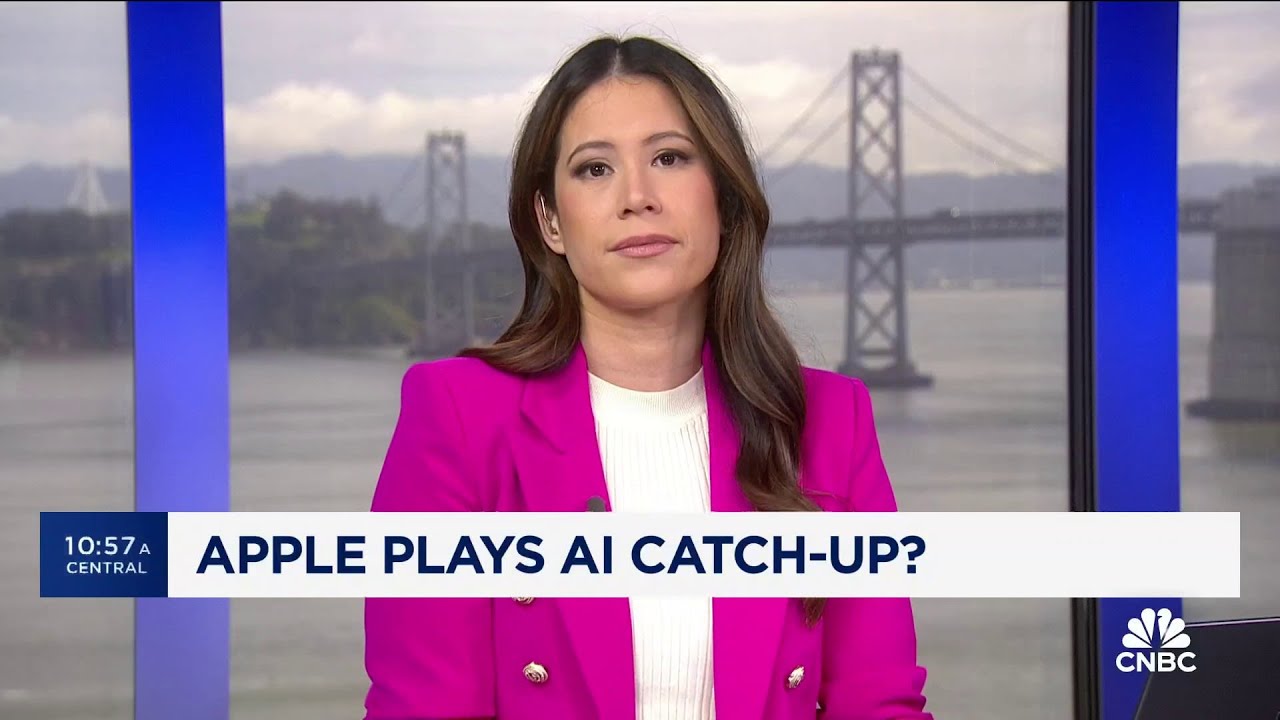
Apple unveils 'world's best consumer laptop for AI'
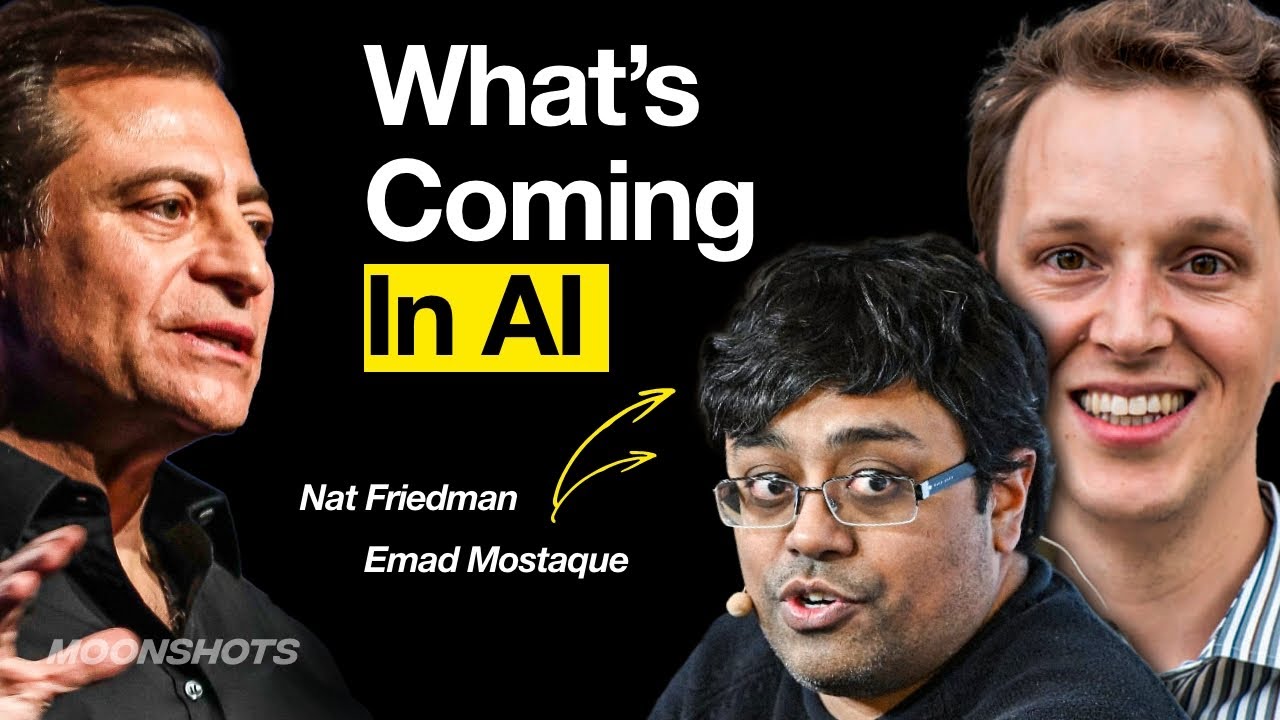
2 Ex-AI CEOs Debate the Future of AI w/ Emad Mostaque & Nat Friedman | EP #98
5.0 / 5 (0 votes)