Complete Sufficiency
Summary
TLDRThis lecture introduces the concepts of complete and sufficient statistics within statistical inference, focusing on determining complete sufficient statistics in exponential families of distributions. It explores results for one and multiple parameter families, providing several examples such as the normal and beta distributions. The lecture further discusses cases outside the exponential family, emphasizing the intricacies of identifying complete sufficient statistics in these scenarios. Notably, it concludes with key remarks highlighting that a complete statistic may not always be sufficient and vice versa, illustrating the nuanced relationships between these statistical properties.
Takeaways
- 📊 A statistic T is called complete sufficient for an unknown parameter θ if it is both sufficient for θ and complete.
- 🔍 The lecture introduces the concept of complete sufficient statistics within the context of exponential family distributions.
- 📈 For a one-parameter exponential family, if the PMF or PDF is of a specific form, the statistic T is complete sufficient for θ.
- 🧮 The factorization theorem is crucial for proving sufficiency in exponential families, allowing us to express PMF/PDF in a specific format.
- 🔗 Examples of complete sufficient statistics include the sum of random samples from Poisson and normal distributions.
- 🎲 When distributions do not belong to the exponential family, determining complete sufficient statistics is more complex.
- 🌟 For a uniform distribution, the maximum statistic serves as a complete sufficient statistic when expressed in a specific factorized form.
- 🏗️ In cases with K-parameter exponential families, both the sum of observations and the sum of squares can be complete sufficient for the parameters.
- 💡 A complete statistic may not be sufficient, and a sufficient statistic may not be complete; understanding these distinctions is essential in statistical inference.
- 📚 The lecture emphasizes the importance of recognizing the nuances of sufficiency and completeness in different statistical contexts.
Q & A
What is the main focus of the lecture?
-The lecture primarily focuses on the concept of a complete sufficient statistic, specifically in the context of statistical inference. It discusses how to determine complete sufficient statistics for distributions belonging to the exponential family and for those that do not.
What are sufficiency and completeness in statistical inference?
-In statistical inference, sufficiency refers to a statistic that captures all necessary information about a parameter, while completeness refers to a statistic that contains no redundant information. A statistic is complete sufficient if it is both sufficient and complete.
What is the definition of a complete sufficient statistic?
-A statistic T is called complete sufficient for an unknown parameter θ if and only if T is sufficient for θ and T is complete.
What result determines the complete sufficient statistic for the exponential family of distributions?
-For a one-parameter exponential family with the form f(θ, x) = e^(Q(θ)T(x) + u(θ) + v(x)), the statistic T is complete sufficient for θ. This is based on the factorization theorem and the fact that T is sufficient for θ.
What is the significance of the factorization theorem in determining sufficiency?
-The factorization theorem is used to determine whether a statistic is sufficient for a parameter. It states that a statistic T is sufficient for θ if the joint probability function can be factored into a product of a function of T and θ and a function of the data that does not depend on θ.
What is an example of a complete sufficient statistic for a normal distribution with known variance?
-For a random sample from a normal distribution with mean θ and variance 1, the sum of the sample values, ΣXi, is a complete sufficient statistic for θ.
How can complete sufficient statistics be determined for a k-parameter exponential family?
-For a k-parameter exponential family, if the joint distribution has the form f(θ, x) = e^(ΣQi(θ)Ti(x) + u(θ) + v(x)), the statistics T1, T2, ..., Tk are complete sufficient for θ1, θ2, ..., θk.
How does the joint PMF of a random sample from a Boyce population illustrate a counterexample of sufficiency?
-In the case of a random sample from a Boyce population with mean θ, X1 is complete for θ but not sufficient. This shows that completeness does not necessarily imply sufficiency.
What is the difficulty in determining a complete sufficient statistic for distributions not in the exponential family?
-When the underlying distribution does not belong to the exponential family, it becomes significantly more challenging to determine a complete sufficient statistic. This complexity arises due to the lack of a straightforward factorization method.
What are the key takeaways regarding the relationship between sufficiency and completeness?
-The lecture concludes that a sufficient statistic may not always be complete, and a complete statistic may not always be sufficient. These two concepts are distinct and may not necessarily coincide in all cases.
Outlines
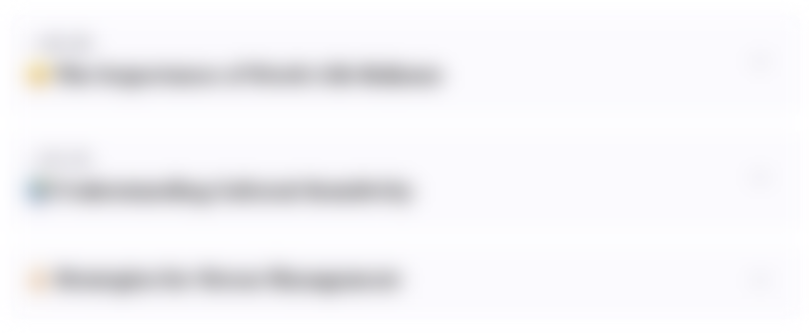
This section is available to paid users only. Please upgrade to access this part.
Upgrade NowMindmap
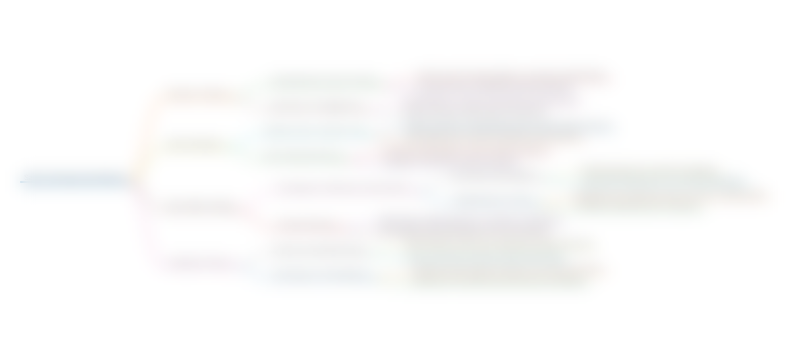
This section is available to paid users only. Please upgrade to access this part.
Upgrade NowKeywords
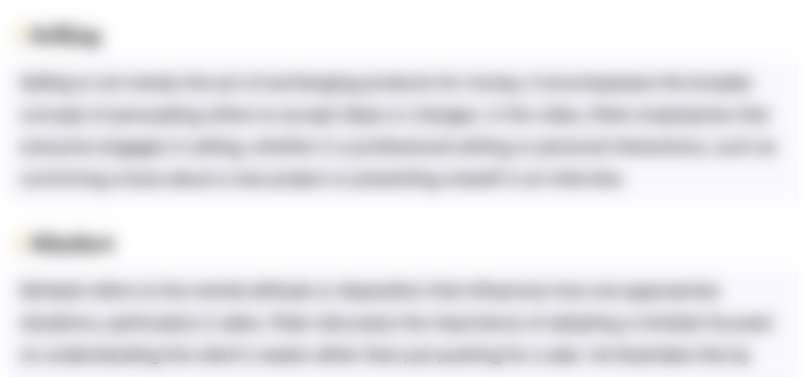
This section is available to paid users only. Please upgrade to access this part.
Upgrade NowHighlights
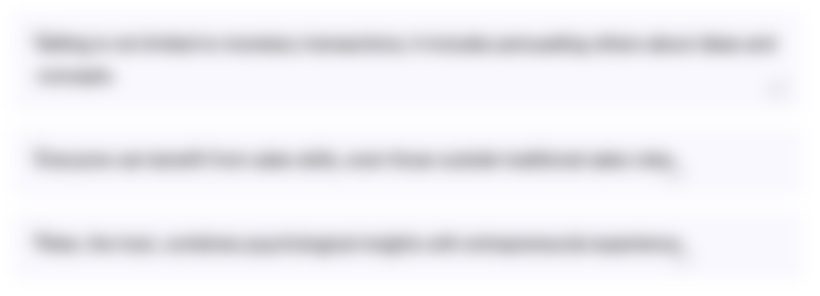
This section is available to paid users only. Please upgrade to access this part.
Upgrade NowTranscripts
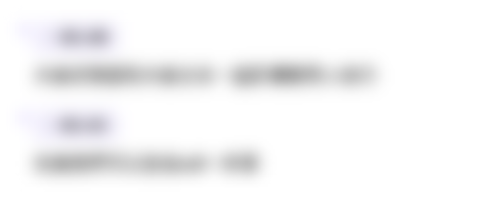
This section is available to paid users only. Please upgrade to access this part.
Upgrade Now5.0 / 5 (0 votes)