11. Mind vs. Brain: Confessions of a Defector
Summary
TLDR在这段视频中,David Dalrymple,一位从MIT媒体实验室转至哈佛大学生物物理学博士项目的研究者,分享了他从AI领域转向神经科学的经历和洞见。他讨论了神经科学与AI之间的联系,并提出了一个观点:尽管我们对宇宙的理解已经相当深入,但对于意识和认知的本质,我们知之甚少。Dalrymple将当前神经科学的研究比作哥白尼时代的天文学,认为我们正处在一个即将有重大突破的前夕。他介绍了多种现代神经科学工具,包括光遗传学和多光子显微镜,这些工具使得科学家能够以前所未有的精确度控制和观察大脑活动。此外,他还探讨了关于神经网络的动态和复杂性,以及如何通过这些工具来研究如秀丽隐杆线虫(C. elegans)这样的模型生物的神经系统。Dalrymple的研究目标是建立一个详细的神经网络模型,以解释C. elegans的行为,并希望通过这项工作为理解更复杂的大脑提供基础。
Takeaways
- 📚 大卫·达尔林普尔(David Dalrymple)曾是AI领域的研究者,后转向神经科学领域,目前在哈佛大学生物物理学攻读博士学位,专注于研究线虫(C. elegans)的行为和神经网络。
- 🏛️ 达尔林普尔认为,尽管目前对神经科学的研究还处于初级阶段,但随着新技术的出现,如光遗传学(optogenetics)和多光子显微镜(multiphoton microscopy),未来几十年内有望取得突破性进展。
- 🔬 通过光遗传学技术,科学家可以精确控制特定神经元的活动,这项技术通过基因工程将光敏感的离子通道引入神经元,利用光照来激活或抑制这些神经元。
- 🧬 达尔林普尔提到,通过基因编辑技术,研究者可以在不伤害线虫的情况下,模拟神经元缺失或改变其活动,以观察线虫行为的变化。
- 💡 他强调了数学和计算模型在理解神经系统中的重要性,认为未来可能会有新的数学理论出现,帮助我们更好地理解大脑的工作机制。
- 🌐 达尔林普尔讨论了神经网络的规模自由特性(scale-free networks),这可能与人类意识和认知的某些方面有关。
- 📈 他提到了神经科学和人工智能之间的联系,以及如何通过研究简单的生物模型(如线虫)来理解更复杂的大脑功能。
- 🧠 达尔林普尔认为,要完全理解人类大脑的工作原理,可能需要从单个神经元的活动到整个大脑网络的动态进行全面的研究。
- 🤖 他提到了人工智能的发展,以及如何通过模仿大脑的工作原理来设计更先进的AI系统。
- 🔬 达尔林普尔讨论了神经科学实验中使用的一些技术,包括如何通过操纵神经元来研究动物的行为反应。
- ⚙️ 他还提到了未来技术可能带来的风险,包括生物工程和基因编辑技术可能被滥用的情况。
Q & A
大卫·达尔林普尔(David Dalrymple)在演讲中提到的'Mind vs. Brain--Confessions of a Defector'是什么意思?
-这是他演讲的标题,意在表达他从人工智能(AI)领域转向神经科学领域的过程。他自称是“变节者”,因为他过去是AI领域的研究者,但现在他转而在哈佛大学作为生物物理学的博士生,专注于研究线虫的神经系统,试图了解它们是如何“思考”的。
大卫·达尔林普尔提到他是如何看到神经科学和人工智能之间的关系的?
-他认为神经科学和人工智能都在尝试理解思维是什么。他将科学比作一个塔,底部是物理学,上面是化学、生物学,再上面是神经科学。而在计算机科学领域,从计算理论开始,然后是软件工程和人工智能。这两个领域都在努力理解思维的实质。
大卫·达尔林普尔为什么从AI领域转向研究线虫?
-他提到在AI领域他并没有太多好的想法,虽然别人认为他的想法很好,但这让他感到害怕,所以他稍微退缩了。后来他发现了一个非常酷的神经科学问题,并决定离开MIT的AI领域,转而去哈佛研究线虫。
大卫·达尔林普尔如何看待人类经验的本质?
-他认为人类经验主要由意识或认知主导,而我们对此知之甚少。他提到认知科学(cog sci)是连接神经科学和人工智能的领域,但目前还相当模糊。
在演讲中,大卫·达尔林普尔提到了哪些科学领域的层级结构?
-他提到了一个层级结构,从基础的物理学开始,然后是化学、生物学,再到神经科学。在计算机科学领域,从计算理论开始,接着是软件工程和人工智能。
大卫·达尔林普尔如何描述他目前的研究工作?
-他目前正在使用多光子显微镜和光遗传学工具来精确控制和测量线虫的神经元活动。他希望能够建立一个模型,模拟线虫的302个神经元如何相互作用以及如何影响行为。
线虫C. elegans的神经系统有什么特别之处?
-线虫C. elegans是唯一已知完整连接组(connectome)的生物,这意味着我们知道它所有302个神经元的连接方式。然而,仅仅知道这些连接并不足以理解线虫的行为或计算方式。
大卫·达尔林普尔提到了哪些技术可以用来研究神经系统?
-他提到了多光子显微镜、光遗传学(使用如channelrhodopsin这样的光敏感通道)和GCaMP这种荧光蛋白,这些技术可以用来激活或抑制特定的神经元,测量神经元的活动和钙浓度或膜电位。
大卫·达尔林普尔如何看待神经科学研究的未来?
-他认为神经科学研究的未来将涉及到更复杂的生物体,如斑马鱼、果蝇、小鼠、猫、狗、猴子,最终是人类。他预见了一种新的数学洞察力的出现,这将类似于微积分的发现,并将帮助我们理解生物系统中复杂网络是如何形成和执行计算的。
在演讲中,大卫·达尔林普尔提到了哪些科学史上的人物?
-他提到了哥白尼(Copernicus)和牛顿(Newton),以及恩斯特·卢瑟福(Ernest Rutherford),这些都是科学史上的重要人物。
大卫·达尔林普尔为什么认为生物学在很大程度上仍然处于“集邮”阶段?
-他认为生物学在很大程度上仍然处于描述和分类的阶段,而不是像物理学那样通过方程式精确地描述事物的工作方式。他指出,当涉及到大脑时,仅仅将事物分类并不足以理解其作为一个动态系统是如何与周围环境互动的。
大卫·达尔林普尔提到了哪些关于线虫行为研究的内容?
-他提到线虫在特定的实验条件下会展现出特定的行为,如omega转或反转等。他的目标是创建一个虚拟的线虫模型,当置于这些条件下时,能够展现出与物理线虫相同的行为。
大卫·达尔林普尔如何看待神经科学的实验方法?
-他认为传统的神经科学实验方法,如使用电极测量大脑对刺激的反应,虽然有价值,但只能提供有限的信息。他正在探索使用光遗传学和多光子显微镜等新技术来进行更精确的神经控制和监测。
大卫·达尔林普尔提到了哪些关于线虫神经系统的基因控制?
-他提到线虫神经系统可能涉及大约100个基因,这些基因控制着如通道和转运蛋白等关键的神经功能。
大卫·达尔林普尔如何看待线虫的生命周期和遗传操作?
-他认为线虫的生命周期非常短,只有四天,而且它们是自育的雌雄同体生物,这意味着它们可以自我克隆。此外,线虫对低温冷冻的抵抗力使其可以在液氮中冷冻多年后仍能存活。
大卫·达尔林普尔提到了哪些关于线虫行为的变异?
-他提到,当线虫的某些基因发生突变时,会导致网络结构的变化,有时这会导致某些神经元无法激发,从而成为信号传递的障碍。
大卫·达尔林普尔如何看待线虫神经系统的复杂性?
-他认为线虫的神经系统虽然只有302个神经元,但每个神经元都非常专业化,执行特定的任务。他希望通过研究线虫的神经系统来找到控制行为的基本神经机制。
大卫·达尔林普尔提到了哪些关于线虫神经系统的实验挑战?
-他提到了在实验中需要同时控制线虫的感觉神经元和运动神经元,以便在虚拟环境中模拟线虫的行为。他还提到了需要开发新的算法来追踪和解析在快速变化中的线虫神经系统的活动。
大卫·达尔林普尔如何看待神经科学研究的未来趋势?
-他认为未来的神经科学研究将更多地依赖于新的技术和方法,如光遗传学和多光子显微镜,这些技术将使我们能够以前所未有的精度和控制力来研究神经系统。
Outlines
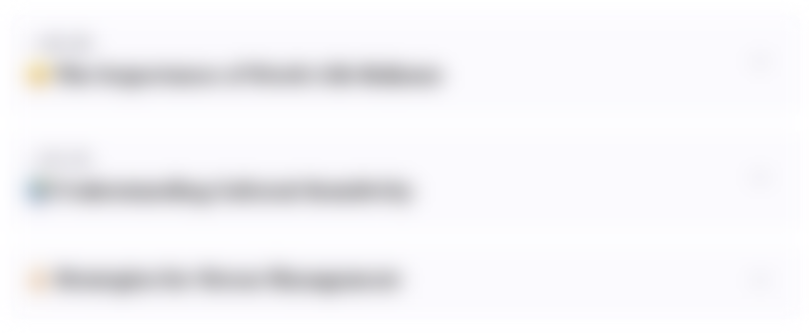
This section is available to paid users only. Please upgrade to access this part.
Upgrade NowMindmap
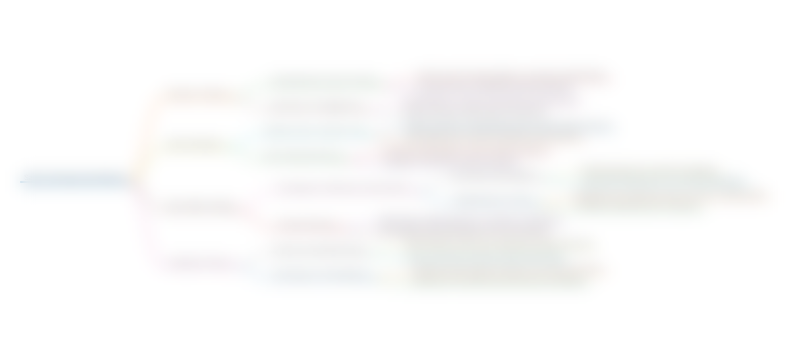
This section is available to paid users only. Please upgrade to access this part.
Upgrade NowKeywords
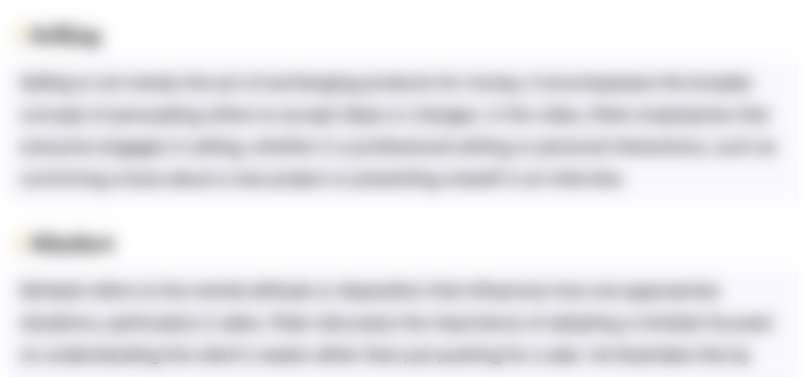
This section is available to paid users only. Please upgrade to access this part.
Upgrade NowHighlights
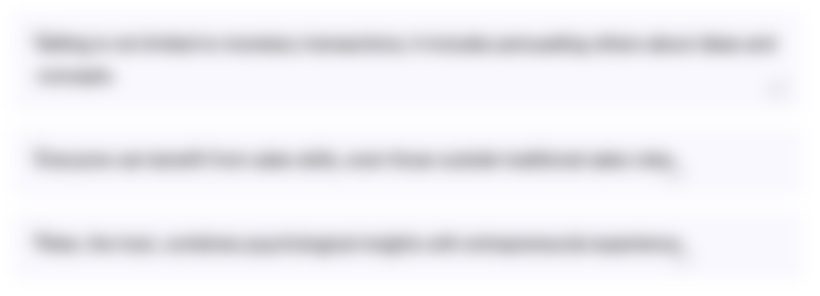
This section is available to paid users only. Please upgrade to access this part.
Upgrade NowTranscripts
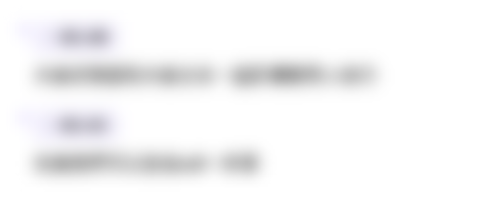
This section is available to paid users only. Please upgrade to access this part.
Upgrade NowBrowse More Related Video
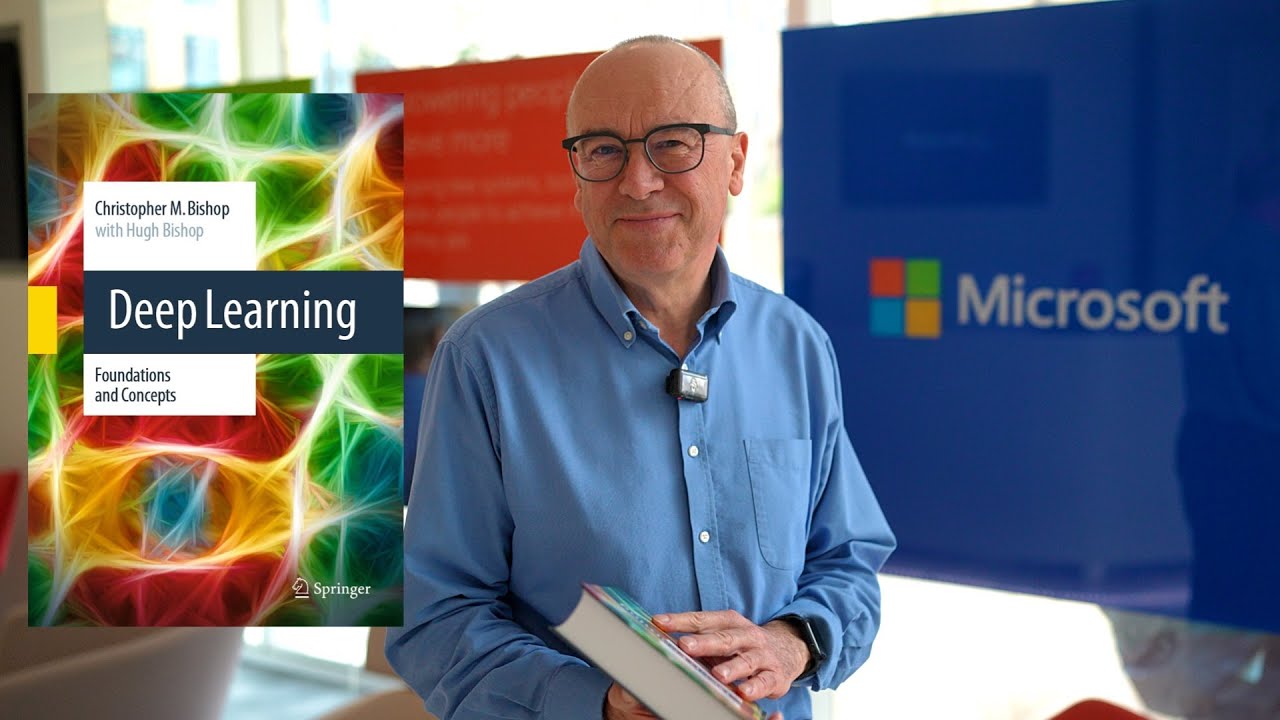
Prof. Chris Bishop's NEW Deep Learning Textbook!
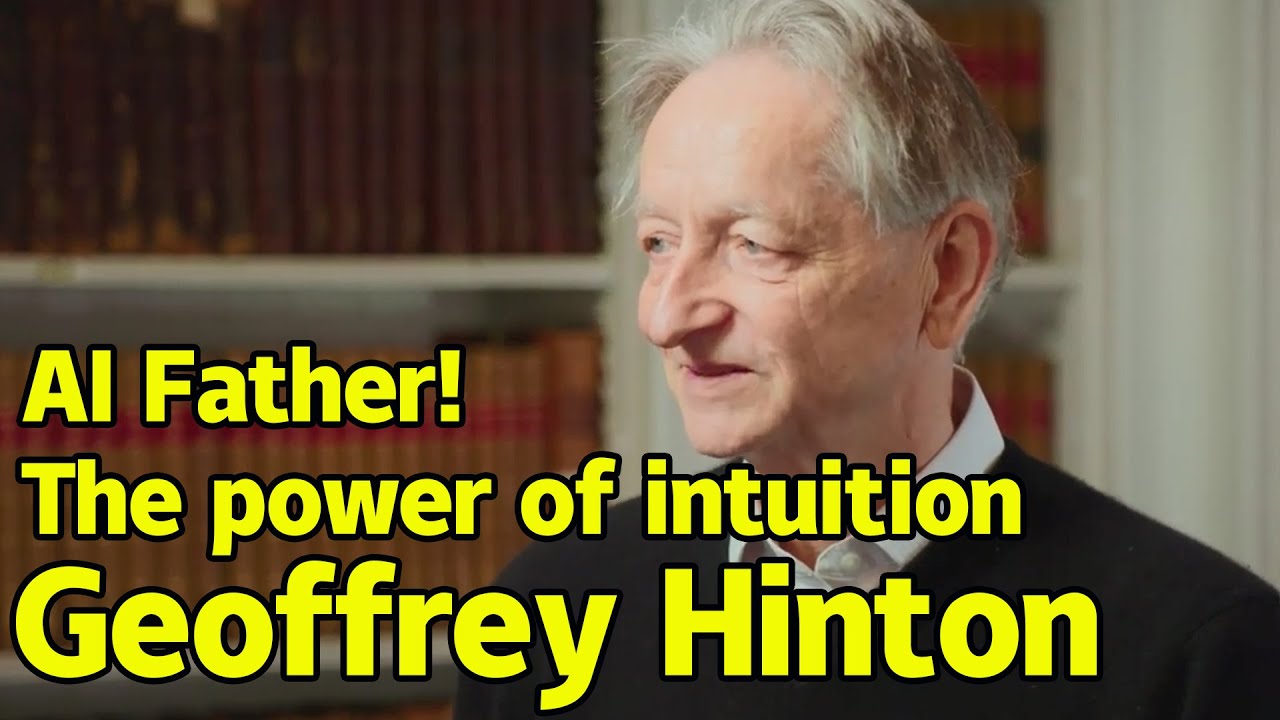
Geoffrey Hinton | Ilya's AI tutor Talent and intuition have already led to today’s large AI models

Heroes of Deep Learning: Andrew Ng interviews Geoffrey Hinton

Recréer le passé grâce à la réalité virtuelle - Dans les coulisses de "Mondes disparus"

Your Brain: Who's in Control? | Full Documentary | NOVA | PBS
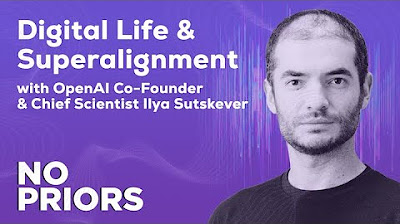
No Priors Ep. 39 | With OpenAI Co-Founder & Chief Scientist Ilya Sutskever
5.0 / 5 (0 votes)