7. Layered Knowledge Representations
Summary
TLDR在这段对话中,马文·明斯基(Marvin Minsky)与听众探讨了人工智能、认知心理学、计算机科学以及大脑和学习的本质。明斯基提出了关于人工智能未来研究方向的问题,强调了跨学科研究的重要性,并讨论了弗洛伊德的心理结构模型,将其与人工智能的层级结构进行比较。他深入解释了人类如何处理目标和欲望,以及大脑如何通过不同的机制来减少当前状态和期望状态之间的差异。此外,明斯基还探讨了记忆如何在大脑中存储,特别是短期记忆在杏仁核中的作用,以及睡眠对记忆长期保持的影响。他提出了关于记忆、语言和学习的一些理论,包括K-line理论,并讨论了数学学习的难点,强调了在教育中采用多种表征方式的重要性。整个对话涵盖了广泛的主题,展示了明斯基对人类认知和人工智能的深刻见解。
Takeaways
- 📚 马文·明斯基(Marvin Minsky)讨论了人工智能领域的未来研究方向,强调了跨学科合作的重要性。
- 🤔 明斯基提出了关于人工智能的哲学问题,例如智能的本质和意识的工作原理。
- 🧠 他探讨了人类思维的层次结构,类比于弗洛伊德的本我、自我和超我,以及这些层次如何相互作用。
- 📈 明斯基强调了在科学理论中留出空间以适应新想法的重要性,而不是试图将复杂的思想简化为最基本的机制。
- 💡 他提到了关于人类记忆和学习的理论,包括短期记忆如何转化为长期记忆,以及这一过程中可能涉及的大脑结构。
- 🎓 明斯基讨论了教育系统中如何教授和学习数学,强调了使用多种方法和类比来理解复杂概念的重要性。
- 🎵 通过音乐和艺术的类比,明斯基说明了非语言形式如何帮助人们理解和表达复杂的概念。
- 🧐 他提出了关于语言和思维之间关系的问题,探讨了语言如何影响我们的认知和思考过程。
- 📘 明斯基还提到了他对《情感机器》(The Emotion Machine)一书中关于情感和情绪在人类认知中作用的讨论。
- 🤝 他讨论了团队合作的重要性,以及如何通过团队中不同个体的协作来解决复杂问题。
- 🌐 明斯基强调了人工智能发展中需要考虑的伦理和社会问题,包括隐私、自主性和人类工作的未来。
Q & A
MIT OpenCourseWare是如何提供高质量教育资源的?
-MIT OpenCourseWare通过社会各界的支持和捐赠来继续提供高质量的教育资源。观众可以通过访问ocw.mit.edu来获取来自数百门MIT课程的额外材料并进行捐赠。
Marvin Minsky提到的关于AI研究的下一个方向是什么?
-Marvin Minsky在对话中提到,AI研究者应该关注的问题包括对意识、学习机制、以及如何更好地模拟人类认知过程的深入研究。他还提到了关于目标、记忆和认知表示的理论。
Freud的心理结构理论在AI领域有什么启示?
-Freud的心理结构理论,包括本我、自我和超我,为理解人类复杂行为提供了一个框架。在AI领域,这可以类比为不同层次的计算模型,每一层都处理不同类型的信息和任务,相互之间存在冲突和协调。
Marvin Minsky如何描述他对人类意识的理解?
-Marvin Minsky将人类意识描述为由多个层次组成,包括基本本能、社会学习、理想、反思性思考和自我反思等。他认为这些层次之间存在相互作用,并且提出了“心智社会”理论,即大脑中不同的模块或“代理人”如何相互作用形成复杂的思维和行为。
Lisp语言在AI发展史中的重要性是什么?
-Lisp语言是AI发展史上的里程碑,因为它是第一个能够操作符号和表达式的语言,这使得它在AI研究中用于模拟人类思考和逻辑推理方面具有独特优势。Marvin Minsky和John McCarthy对Lisp的贡献对AI领域产生了深远影响。
Marvin Minsky对于人类记忆的K-line理论是什么?
-Marvin Minsky提出的K-line理论是一种关于人类记忆如何在大脑中存储和检索的假设。他认为记忆可能以一种动态的形式存储在神经元的循环连接中,并且通过睡眠等机制在大脑的不同区域之间转移和固化。
在对话中,Marvin Minsky提到了哪些关于学习数学的看法?
-Marvin Minsky在对话中提到,学习数学时,拥有多种不同的表示方法非常重要。他强调了理解数学概念时使用多种方法和类比的重要性,并且提到了通过不断练习和重复来优化这些表示方法。
Marvin Minsky对于教育系统的看法是什么?
-Marvin Minsky认为教育系统应该鼓励学生使用多种方法解决问题,并且强调了在教育中提供多种表示方法的重要性。他还提到了教育中缺乏对于如何优化学习过程的探讨。
在对话中,Marvin Minsky对于音乐和数学的关系有何见解?
-Marvin Minsky在对话中提到,尽管音乐和数学是两个不同的领域,但它们之间存在某种联系。他认为,擅长数学的人不一定擅长音乐,因为音乐可能需要对声音的表示和模式有深刻的理解,这与数学的逻辑和抽象思维不同。
Marvin Minsky如何看待练习对于学习的影响?
-Marvin Minsky认为练习不仅是重复,而是通过每次练习中的变化来深化对概念的理解和掌握。他提到,通过变化练习可以帮助学习者从不同的角度理解和解决问题,这有助于扩展和优化他们的认知表示。
在对话中,Marvin Minsky对于双语学习者有什么有趣的观察?
-Marvin Minsky分享了一个关于他女儿学习颜色名称的故事,展示了儿童如何通过组合现有概念来形成新的概念。他还提到了双语学习者可能会遇到的一些现象,比如在回忆特定对话时忘记了使用的是哪种语言。
Outlines
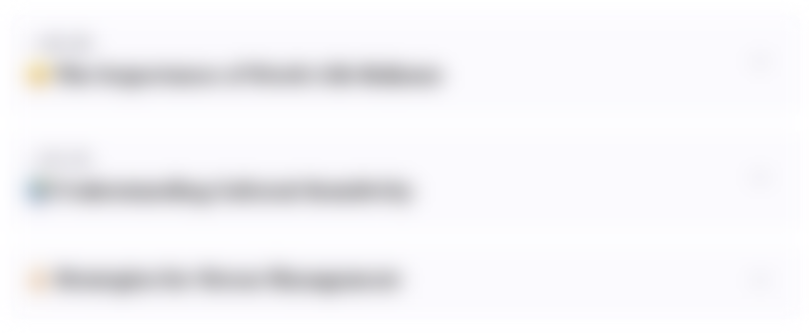
This section is available to paid users only. Please upgrade to access this part.
Upgrade NowMindmap
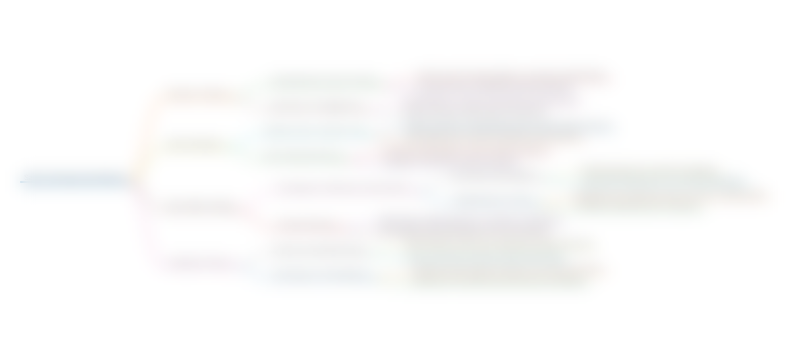
This section is available to paid users only. Please upgrade to access this part.
Upgrade NowKeywords
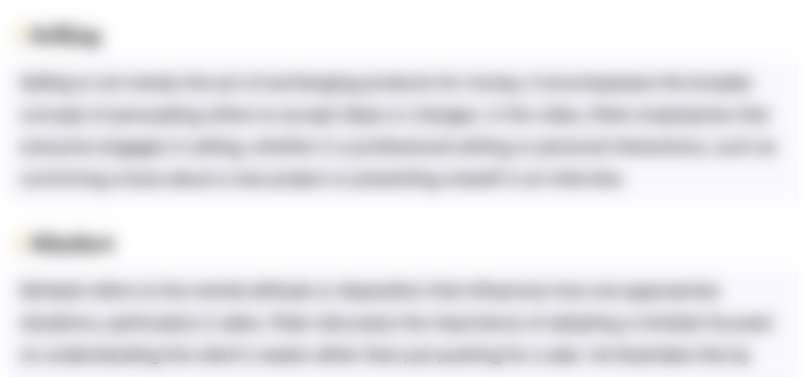
This section is available to paid users only. Please upgrade to access this part.
Upgrade NowHighlights
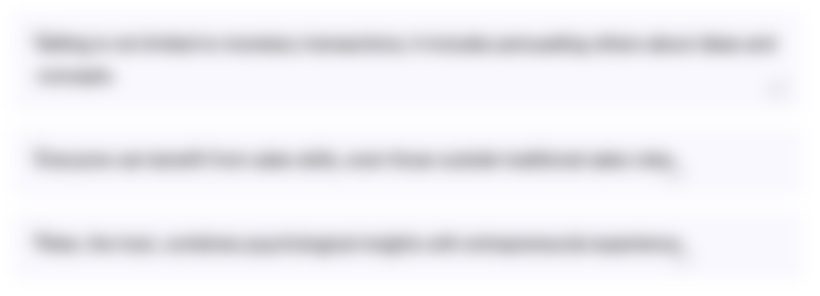
This section is available to paid users only. Please upgrade to access this part.
Upgrade NowTranscripts
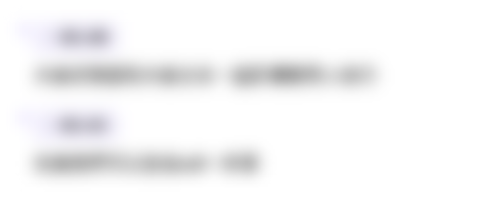
This section is available to paid users only. Please upgrade to access this part.
Upgrade Now5.0 / 5 (0 votes)