Statistical Inference Definition with Example | Statistics Tutorial #18 | MarinStatsLectures
Summary
TLDRThis video provides a comprehensive overview of statistical inference, focusing on the estimation of a single mean. It introduces key concepts such as sampling distribution, standard error, confidence intervals, and hypothesis testing, emphasizing the consistent principles that apply across different statistical tests, whether parametric or non-parametric. The speaker highlights the significance of p-values in understanding the likelihood of observing sample estimates under the null hypothesis and encourages viewers to engage with the material as they prepare to utilize software for advanced statistical analysis.
Takeaways
- 📊 Takeaway 1: Statistical inference lays a foundation for understanding population means through sampling distribution and standard error.
- 📉 Takeaway 2: The standard error indicates how much an estimate may vary from the true population value.
- 🔍 Takeaway 3: Confidence intervals are created by adding and subtracting a margin of error from an estimate, providing a range likely to contain the true mean.
- ✅ Takeaway 4: Hypothesis testing involves comparing sample estimates to hypothesized values using test statistics.
- 📈 Takeaway 5: P-values reflect the probability of obtaining an observed estimate or one more extreme under the null hypothesis.
- ⚖️ Takeaway 6: Confidence intervals and hypothesis tests remain consistent concepts across various statistical methods, whether parametric or nonparametric.
- 🔄 Takeaway 7: Parametric tests, like the one-sample t-test, and nonparametric approaches, such as bootstrapping, serve different purposes but share fundamental ideas.
- 💻 Takeaway 8: Future coursework will focus more on software for statistical calculations rather than manual methods, enhancing efficiency and accuracy.
- 📚 Takeaway 9: Understanding the foundational concepts of statistics is essential for applying more advanced analytical techniques in the course.
- 🎉 Takeaway 10: Engaging with statistical inference can be enjoyable, making it easier to grasp complex concepts in data analysis.
Q & A
What is the purpose of the sampling distribution?
-The sampling distribution describes the theoretical set of all possible estimates (such as the mean) that can be derived from a sample, helping to understand the variability and reliability of our estimates.
What is standard error, and why is it important?
-Standard error indicates how far an estimate, on average, will deviate from the true population value. It is crucial for assessing the precision of the estimate and constructing confidence intervals.
How are confidence intervals constructed?
-Confidence intervals are constructed using the formula: estimate ± (T-value × standard error), which provides a range likely to contain the true population mean.
What does a p-value represent in hypothesis testing?
-A p-value represents the probability of obtaining the observed estimate, or a more extreme value, assuming the null hypothesis is true. It helps determine the significance of the results.
How does hypothesis testing relate to the null hypothesis?
-Hypothesis testing involves calculating a test statistic that compares the sample estimate to the hypothesized value from the null hypothesis, determining how far the sample data is from what would be expected if the null hypothesis were true.
What is the difference between parametric and nonparametric tests?
-Parametric tests assume underlying statistical distributions (like normality), while nonparametric tests do not rely on these assumptions and can be used for data that do not meet parametric criteria.
What role do bootstrapping methods play in statistical inference?
-Bootstrapping methods involve resampling from the observed data to create an empirical distribution of the estimate, allowing for the estimation of standard errors and confidence intervals without relying on parametric assumptions.
Why is understanding the underlying concepts of statistical inference essential?
-Understanding the foundational concepts of statistical inference is essential because it equips students with the knowledge to apply more advanced statistical methods effectively, regardless of the specific tests used.
What can be expected as the course progresses regarding calculations?
-As the course progresses, students will shift from manual calculations to using software for statistical analysis, although the foundational concepts will still guide their understanding of the methods.
What is the speaker's overall message about statistics?
-The speaker conveys that statistics is an exciting field, emphasizing the importance of the foundational concepts discussed, and encourages viewers to engage further with the material.
Outlines
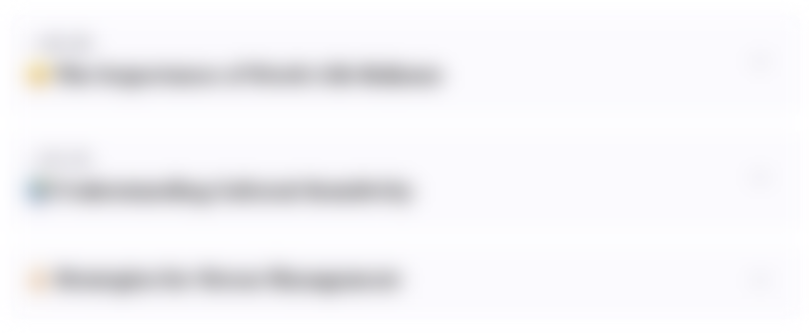
This section is available to paid users only. Please upgrade to access this part.
Upgrade NowMindmap
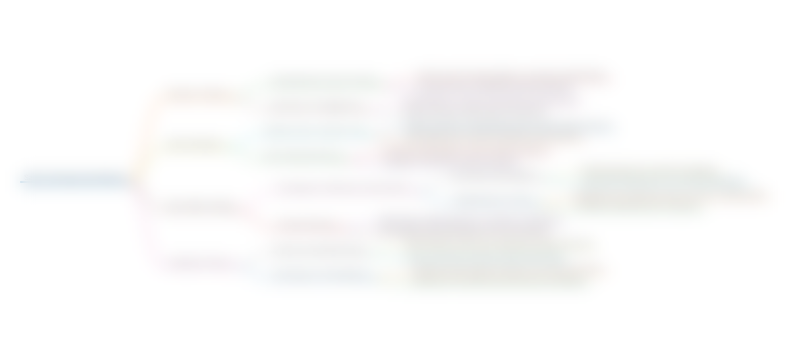
This section is available to paid users only. Please upgrade to access this part.
Upgrade NowKeywords
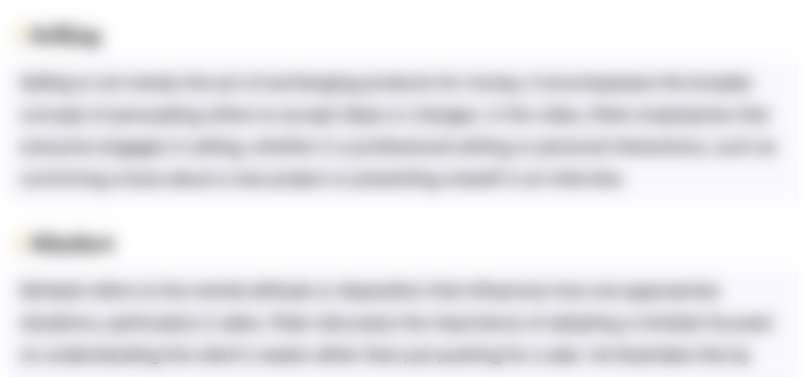
This section is available to paid users only. Please upgrade to access this part.
Upgrade NowHighlights
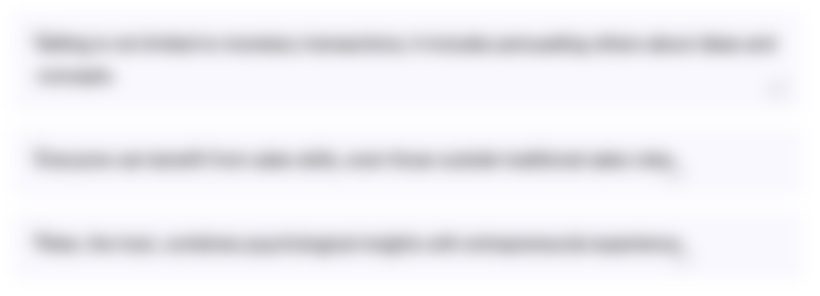
This section is available to paid users only. Please upgrade to access this part.
Upgrade NowTranscripts
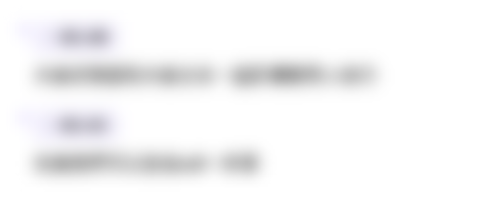
This section is available to paid users only. Please upgrade to access this part.
Upgrade NowBrowse More Related Video
5.0 / 5 (0 votes)